- 2022-04-09 发布 |
- 37.5 KB |
- 18页
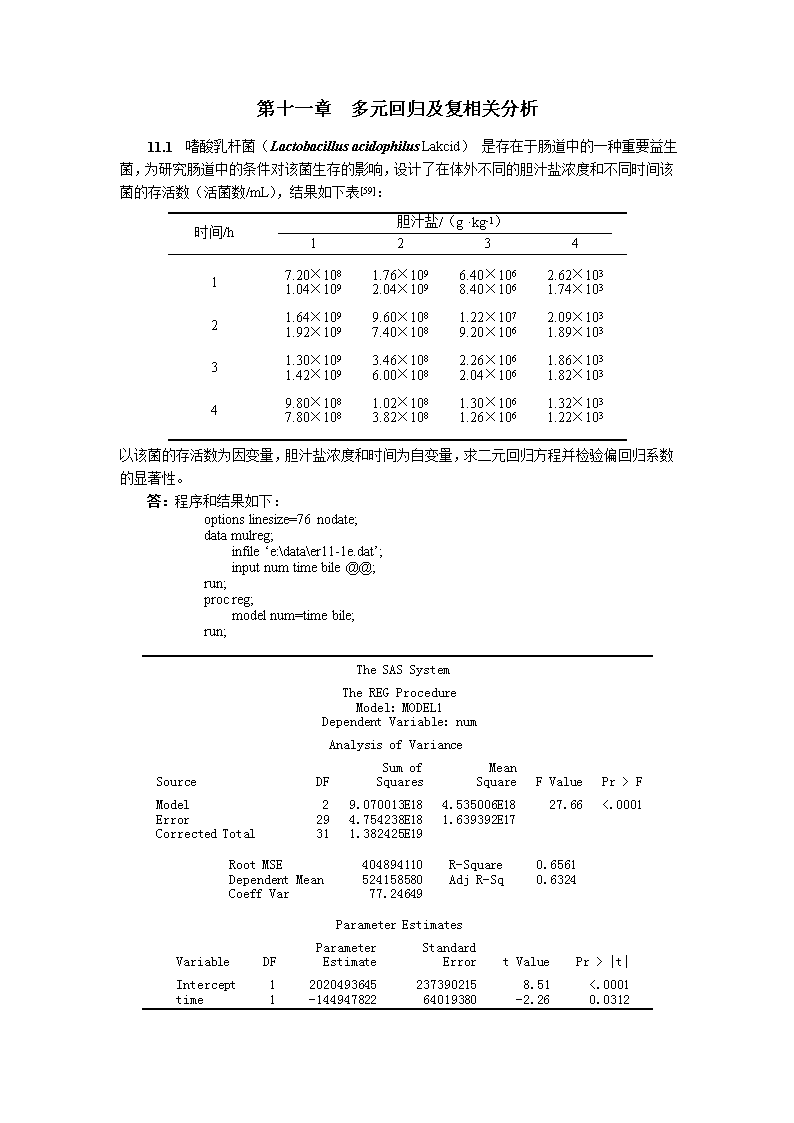
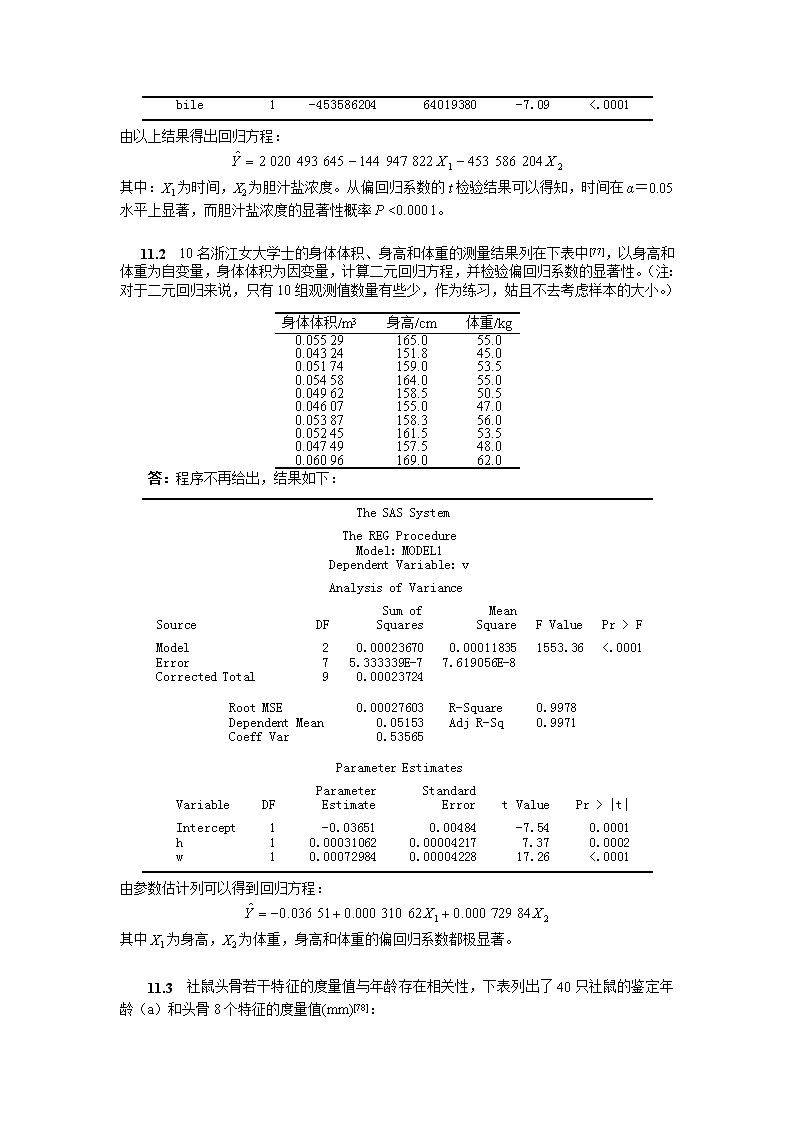
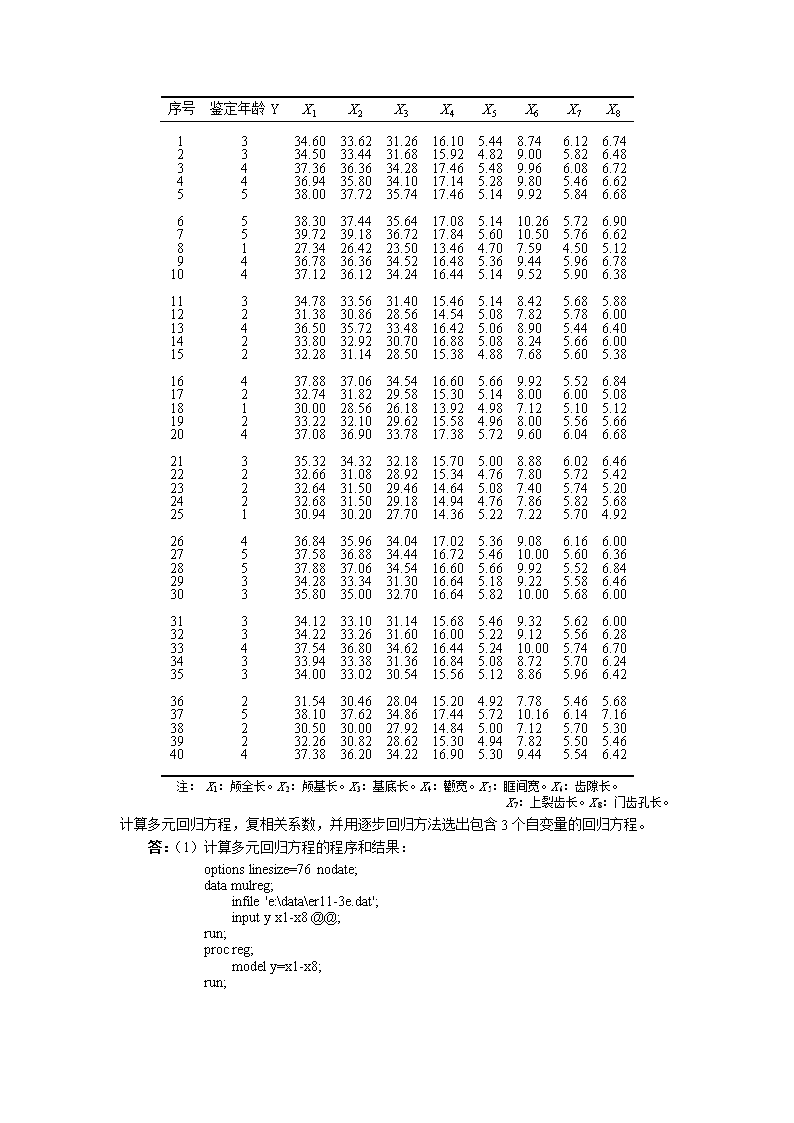
申明敬告: 本站不保证该用户上传的文档完整性,不预览、不比对内容而直接下载产生的反悔问题本站不予受理。
文档介绍
生物统计学(第3版)杜荣骞 课后习题答案 第十一章 多元
第十一章多元回归及复相关分析11.1嗜酸乳杆菌(LactobacillusacidophilusLakcid)是存在于肠道中的一种重要益生菌,为研究肠道中的条件对该菌生存的影响,设计了在体外不同的胆汁盐浓度和不同时间该菌的存活数(活菌数/mL),结果如下表[59]:时间/h胆汁盐/(g·kg-1)123417.20×1081.04×1091.76×1092.04×1096.40×1068.40×1062.62×1031.74×10321.64×1091.92×1099.60×1087.40×1081.22×1079.20×1062.09×1031.89×10331.30×1091.42×1093.46×1086.00×1082.26×1062.04×1061.86×1031.82×10349.80×1087.80×1081.02×1083.82×1081.30×1061.26×1061.32×1031.22×103以该菌的存活数为因变量,胆汁盐浓度和时间为自变量,求二元回归方程并检验偏回归系数的显著性。答:程序和结果如下:optionslinesize=76nodate;datamulreg;infile‘e:dataer11-1e.dat’;inputnumtimebile@@;run;procreg;modelnum=timebile;run;TheSASSystemTheREGProcedureModel:MODEL1DependentVariable:numAnalysisofVarianceSumofMeanSourceDFSquaresSquareFValuePr>FModel29.070013E184.535006E1827.66<.0001Error294.754238E181.639392E17CorrectedTotal311.382425E19RootMSE404894110R-Square0.6561DependentMean524158580AdjR-Sq0.6324CoeffVar77.24649ParameterEstimatesParameterStandardVariableDFEstimateErrortValuePr>|t|Intercept120204936452373902158.51<.0001time1-14494782264019380-2.260.0312nbile1-45358620464019380-7.09<.0001由以上结果得出回归方程:其中:X1为时间,X2为胆汁盐浓度。从偏回归系数的t检验结果可以得知,时间在α=0.05水平上显著,而胆汁盐浓度的显著性概率P<0.0001。11.210名浙江女大学士的身体体积、身高和体重的测量结果列在下表中[77],以身高和体重为自变量,身体体积为因变量,计算二元回归方程,并检验偏回归系数的显著性。(注:对于二元回归来说,只有10组观测值数量有些少,作为练习,姑且不去考虑样本的大小。)身体体积/m3身高/cm体重/kg0.05529165.055.00.04324151.845.00.05174159.053.50.05458164.055.00.04962158.550.50.04607155.047.00.05387158.356.00.05245161.553.50.04749157.548.00.06096169.062.0答:程序不再给出,结果如下:TheSASSystemTheREGProcedureModel:MODEL1DependentVariable:vAnalysisofVarianceSumofMeanSourceDFSquaresSquareFValuePr>FModel20.000236700.000118351553.36<.0001Error75.333339E-77.619056E-8CorrectedTotal90.00023724RootMSE0.00027603R-Square0.9978DependentMean0.05153AdjR-Sq0.9971CoeffVar0.53565ParameterEstimatesParameterStandardVariableDFEstimateErrortValuePr>|t|Intercept1-0.036510.00484-7.540.0001h10.000310620.000042177.370.0002w10.000729840.0000422817.26<.0001由参数估计列可以得到回归方程:其中X1为身高,X2为体重,身高和体重的偏回归系数都极显著。11.3社鼠头骨若干特征的度量值与年龄存在相关性,下表列出了40只社鼠的鉴定年龄(a)和头骨8个特征的度量值(mm)[78]:n序号鉴定年龄YX1X2X3X4X5X6X7X81334.6033.6231.2616.105.448.746.126.742334.5033.4431.6815.924.829.005.826.483437.3636.3634.2817.465.489.966.086.724436.9435.8034.1017.145.289.805.466.625538.0037.7235.7417.465.149.925.846.686538.3037.4435.6417.085.1410.265.726.907539.7239.1836.7217.845.6010.505.766.628127.3426.4223.5013.464.707.594.505.129436.7836.3634.5216.485.369.445.966.7810437.1236.1234.2416.445.149.525.906.3811334.7833.5631.4015.465.148.425.685.8812231.3830.8628.5614.545.087.825.786.0013436.5035.7233.4816.425.068.905.446.4014233.8032.9230.7016.885.088.245.666.0015232.2831.1428.5015.384.887.685.605.3816437.8837.0634.5416.605.669.925.526.8417232.7431.8229.5815.305.148.006.005.0818130.0028.5626.1813.924.987.125.105.1219233.2232.1029.6215.584.968.005.565.6620437.0836.9033.7817.385.729.606.046.6821335.3234.3232.1815.705.008.886.026.4622232.6631.0828.9215.344.767.805.725.4223232.6431.5029.4614.645.087.405.745.2024232.6831.5029.1814.944.767.865.825.6825130.9430.2027.7014.365.227.225.704.9226436.8435.9634.0417.025.369.086.166.0027537.5836.8834.4416.725.4610.005.606.3628537.8837.0634.5416.605.669.925.526.8429334.2833.3431.3016.645.189.225.586.4630335.8035.0032.7016.645.8210.005.686.0031334.1233.1031.1415.685.469.325.626.0032334.2233.2631.6016.005.229.125.566.2833437.5436.8034.6216.445.2410.005.746.7034333.9433.3831.3616.845.088.725.706.2435334.0033.0230.5415.565.128.865.966.4236231.5430.4628.0415.204.927.785.465.6837538.1037.6234.8617.445.7210.166.147.1638230.5030.0027.9214.845.007.125.705.3039232.2630.8228.6215.304.947.825.505.4640437.3836.2034.2216.905.309.445.546.42注:X1:颅全长。X2:颅基长。X3:基底长。X4:颧宽。X5:眶间宽。X6:齿隙长。X7:上裂齿长。X8:门齿孔长。计算多元回归方程,复相关系数,并用逐步回归方法选出包含3个自变量的回归方程。答:(1)计算多元回归方程的程序和结果:optionslinesize=76nodate;datamulreg;infile'e:dataer11-3e.dat';inputyx1-x8@@;run;procreg;modely=x1-x8;run;nTheSASSystemTheREGProcedureModel:MODEL1DependentVariable:yAnalysisofVarianceSumofMeanSourceDFSquaresSquareFValuePr>FModel853.172316.6465464.33<.0001Error313.202690.10331CorrectedTotal3956.37500RootMSE0.32142R-Square0.9432DependentMean3.12500AdjR-Sq0.9285CoeffVar10.28553ParameterEstimatesParameterStandardVariableDFEstimateErrortValuePr>|t|Intercept1-6.149271.68879-3.640.0010x11-0.222960.20853-1.070.2932x210.568130.250382.270.0304x310.017710.192070.090.9271x41-0.120070.12562-0.960.3466x51-0.397540.31415-1.270.2151x610.209350.193461.080.2875x71-0.341980.23671-1.440.1586x810.214640.200761.070.2932从参数估计列可以得到回归方程:复相关系数:(2)逐步回归分析:optionslinesize=76nodate;datastepreg;infile'e:dataer11-3e.dat';inputyx1-x8;run;procreg;modely=x1-x8/selection=stepwiseslentry=0.05slstay=0.05;run;TheSASSystemTheREGProcedureModel:MODEL1DependentVariable:yStepwiseSelection:Step1Variablex2Entered:R-Square=0.9188andC(p)=8.2905AnalysisofVariancenSumofMeanSourceDFSquaresSquareFValuePr>FModel151.7992351.79923430.17<.0001Error384.575770.12041CorrectedTotal3956.37500ParameterStandardVariableEstimateErrorTypeIISSFValuePr>FIntercept-10.245790.6470030.19713250.78<.0001x20.394830.0190451.79923430.17<.0001Boundsonconditionnumber:1,1---------------------------------------------------------------------------StepwiseSelection:Step2Variablex7Entered:R-Square=0.9294andC(p)=4.5012AnalysisofVarianceSumofMeanSourceDFSquaresSquareFValuePr>FModel252.3973426.19867243.70<.0001Error373.977660.10750CorrectedTotal3956.37500ParameterStandardVariableEstimateErrorTypeIISSFValuePr>FIntercept-8.339021.013527.2776767.70<.0001x20.418890.0206844.11123410.32<.0001x7-0.477510.202450.598115.560.0237Boundsonconditionnumber:1.3218,5.2873----------------------------------------------------------------------------StepwiseSelection:Step3Variablex8Entered:R-Square=0.9369andC(p)=2.4570AnalysisofVarianceSumofMeanSourceDFSquaresSquareFValuePr>FModel352.8151617.60505178.04<.0001Error363.559840.09888CorrectedTotal3956.37500ParameterStandardVariableEstimateErrorTypeIISSFValuePr>FIntercept-8.426720.972977.4172675.01<.0001x20.357660.035799.8751399.87<.0001x7-0.459880.194350.553675.600.0235x80.336390.163650.417824.230.0471Boundsonconditionnumber:4.3043,28.581----------------------------------------------------------------------------Allvariablesleftinthemodelaresignificantatthe0.0500level.Noothervariablemetthe0.0500significancelevelforentryintothenmodel.SummaryofStepwiseSelectionVariableVariableNumberPartialModelStepEnteredRemovedVarsInR-SquareR-SquareC(p)FValuePr>F1x210.91880.91888.2905430.17<.00012x720.01060.92944.50125.560.02373x830.00740.93692.45704.230.0471引入方程中的三个变量没有剔除,最终保留在方程中的三个变量,在α=0.05水平上全都是显著的。方程如下:11.4下表给出了高山姬鼠头骨8个特征的测量值和鉴定年龄[79],用逐步回归方法从8个特征中选出与鉴定年龄关系最密切的变量,并对结果做回归的方差分析。序号鉴定年龄/a头骨特征/mmX1X2X3X4X5X6X7X81530.6430.0028.3414.324.308.784.525.662328.7828.5626.7814.004.568.064.345.463328.0027.1225.0413.864.487.564.345.024226.6426.1624.5213.144.687.064.464.865226.0825.5023.7613.284.526.944.364.946429.4028.7027.8614.144.868.244.685.487124.8224.0422.0612.444.526.384.344.748226.5625.7423.7813.024.587.164.185.149227.1826.2624.4413.064.747.344.205.2010226.4625.8224.1213.064.587.064.204.5011429.6228.8227.0413.524.448.284.345.4812530.1029.8828.2414.024.668.824.385.4613531.1830.6229.0614.604.868.864.825.9214327.5426.9225.3014.144.587.544.525.1615328.4027.9426.3013.844.467.844.545.6816328.1227.6425.9613.764.427.964.365.1417227.5027.0025.3613.164.447.684.325.4418429.1828.3626.4614.704.707.864.605.4619530.3429.9228.2415.004.789.264.386.0420532.5032.0230.1415.345.148.964.786.1021531.2830.9629.0215.084.729.184.626.0022227.3826.8825.1413.384.587.244.425.2023124.4223.8822.1212.404.626.284.204.4624226.8826.2224.4413.344.627.564.165.0025227.5027.0025.3613.164.447.684.325.4426328.3427.6625.7813.824.887.764.525.6027328.5827.7225.7814.584.767.004.085.2428328.4828.0426.2813.784.767.804.345.6829328.8028.0826.3014.004.827.264.605.92注:X1:颅全长。X2:颅基长。X3:基底长。X4:颧宽。X5:眶间距。X6:齿隙长。X7:上裂齿长。X8:门齿孔长。答:结果如下:TheSASSystemnTheREGProcedureModel:MODEL1DependentVariable:yStepwiseSelection:Step1Variablex1Entered:R-Square=0.9111andC(p)=11.3797AnalysisofVarianceSumofMeanSourceDFSquaresSquareFValuePr>FModel139.9626539.96265276.71<.0001Error273.899420.14442CorrectedTotal2843.86207ParameterStandardVariableEstimateErrorTypeIISSFValuePr>FIntercept-14.876811.0811427.34609189.35<.0001x10.634130.0381239.96265276.71<.0001Boundsonconditionnumber:1,1----------------------------------------------------------------------------StepwiseSelection:Step2Variablex6Entered:R-Square=0.9259andC(p)=7.3289AnalysisofVarianceSumofMeanSourceDFSquaresSquareFValuePr>FModel240.6112220.30561162.40<.0001Error263.250850.12503CorrectedTotal2843.86207ParameterStandardVariableEstimateErrorTypeIISSFValuePr>FIntercept-13.313311.2178614.94162119.50<.0001x10.440660.092052.8653022.92<.0001x60.503250.220960.648575.190.0312Boundsonconditionnumber:6.7351,26.941StepwiseSelection:Step3Variablex8Entered:R-Square=0.9375andC(p)=4.5706AnalysisofVarianceSumofMeanSourceDFSquaresSquareFValuePr>FModel341.1212513.70708125.03<.0001Error252.740820.10963CorrectedTotal2843.86207ParameterStandardVariableEstimateErrorTypeIISSFValuePr>FIntercept-13.506691.1439215.28437139.41<.0001x10.566480.104083.2477229.62<.0001x60.513470.206960.674826.160.0202nx8-0.643090.298160.510034.650.0408Boundsonconditionnumber:9.8194,62.516----------------------------------------------------------------------------Allvariablesleftinthemodelaresignificantatthe0.0500level.Noothervariablemetthe0.0500significancelevelforentryintothemodel.SummaryofStepwiseSelectionVariableVariableNumberPartialModelStepEnteredRemovedVarsInR-SquareR-SquareC(p)FValuePr>F1x110.91110.911111.3797276.71<.00012x620.01480.92597.32895.190.03123x830.01160.93754.57064.650.0408在α=0.05水平上筛选出三个变量,它们分别是:X1,X6和X8。回归方程为:方差分析表:变差来源平方和自由度均方FP回归41.12125313.70708125.03<0.0001误差2.74082250.10963总和43.862072811.5土壤根际微生物的生物量氮与季节变化有如下关联[80]:月份生物量氮/(10-4mg·100g-1)56.5767.4478.72810.68911.55109.15115.87124.42生物量氮与月份之间存在怎样的回归关系?求出回归方程。答:先绘出散点图,然后求回归方程。n从散点图上可见,生物量氮与月份呈抛物线关系,应当用一元二次方程拟合。程序与结果如下:optionslinesize=76nodate;datastepreg;infile'e:dataer11-5e.dat';inputx1y;x2=x1**2;run;procreg;modely=x1x2;run;TheSASSystemTheREGProcedureModel:MODEL1DependentVariable:yAnalysisofVarianceSumofMeanSourceDFSquaresSquareFValuePr>FModel235.6153517.8076715.610.0071Error55.702251.14045CorrectedTotal741.31760RootMSE1.06792R-Square0.8620DependentMean8.05000AdjR-Sq0.8068CoeffVar13.26607ParameterEstimatesParameterStandardVariableDFEstimateErrortValuePr>|t|Intercept1-19.570605.70767-3.430.0187x117.293811.410325.170.0035x21-0.443570.08239-5.380.0030回归方程为:一次项和二次项的回归系数都是极显著的。11.6两种农药“呋喃丹”和“铁灭克”,在不同pHn条件下对土壤磷酸酶活性(mg/g)的影响如下表所示[81]:缓冲液pH呋喃丹(Y1)铁灭克(Y2)7.90.190.108.31.370.798.71.311.099.11.651.219.31.491.299.61.120.8710.01.070.7810.50.310.2211.00.120.10分别绘出呋喃丹和铁灭克对pH的散点图,计算出回归方程并求出磷酸酶活性达到最大值时的pH值,以及在该pH时磷酸酶的活性值。答:计算程序与上题一样,不再给出,只给出结果。(1)呋喃丹:TheSASSystemTheREGProcedureModel:MODEL1DependentVariable:y1AnalysisofVarianceSumofMeanSourceDFSquaresSquareFValuePr>FModel22.258591.1292912.370.0074Error60.547700.09128CorrectedTotal82.80629RootMSE0.30213R-Square0.8048DependentMean0.95889AdjR-Sq0.7398CoeffVar31.50847ParameterEstimatesParameterStandardVariableDFEstimateErrortValuePr>|t|Intercept1-41.710199.89391-4.220.0056x119.343952.108524.430.0044x21-0.505950.11147-4.540.0039n回归方程为:一次项和二次项的回归系数都是极显著的。最大值的计算:1.0119X=9.34395X=9.23406Y=1.43113故当pH9.23406时磷酸酶活性有最大值,其最大值为1.43113。(2)铁灭克:TheSASSystemTheREGProcedureModel:MODEL2DependentVariable:y2AnalysisofVarianceSumofMeanSourceDFSquaresSquareFValuePr>FModel21.465640.7328215.380.0044Error60.285960.04766CorrectedTotal81.75160RootMSE0.21831R-Square0.8367DependentMean0.71667AdjR-Sq0.7823CoeffVar30.46194ParameterEstimatesParameterStandardVariableDFEstimateErrortValuePr>|t|Intercept1-35.503327.14903-4.970.0025x117.881571.523555.170.0021x21-0.424190.08054-5.270.0019回归方程为:一次项和二次项的回归系数都是极显著的。最大值的计算:0.84838X=7.88157X=9.29014Y=1.10713故当pH9.29014时磷酸酶活性有最大值,其最大值为1.10713。11.7“武运粳7号”考种相关数据见下表[82]:n序号产量/(kg·hm-2)千粒重/g每穗总粒数/粒亩有效穗/(104·hm-2)株高/cm19787.525.9125.7372.30102.529390.025.8131.3363.75105.639607.526.3122.5370.8099.349547.525.9128.3377.7098.959237.026.5127.8358.65103.568947.525.8137.5340.05100.378277.525.7118.2372.9098.888475.526.2113.6373.9597.698415.025.9118.9373.0597.3108040.025.4118.5356.7095.3118167.526.1121.3333.6095.6127845.025.3124.7345.7595.1137927.525.8121.6343.5094.7147327.525.6112.5343.2094.5157305.025.9103.8362.4093.6167125.025.4123.1319.2092.5177140.026.1113.8308.5589.6186945.026.4111.5306.4590.5以产量为因变量,计算多元回归方程,通过逐步回归筛选出对产量影响的重要因素。答:(1)多元回归方程见下表:TheSASSystemTheREGProcedureModel:MODEL1DependentVariable:yAnalysisofVarianceSumofMeanSourceDFSquaresSquareFValuePr>FModel414038414350960350.79<.0001Error1389823969095CorrectedTotal1714936652RootMSE262.85981R-Square0.9399DependentMean8305.97222AdjR-Sq0.9214CoeffVar3.16471ParameterEstimatesParameterStandardVariableDFEstimateErrortValuePr>|t|Intercept1-312455522.29553-5.66<.0001x11839.98368215.863583.890.0019x2165.8977014.605974.510.0006x3123.223495.130904.530.0006x4117.3875637.836320.460.6534从参数估计列可以得出回归方程:(2)用逐步回归方法筛选最优回归方程:①首先以sle=0.25和sls=0.25显著水平进行筛选,结果见下表:TheSASSystemnTheREGProcedureModel:MODEL1DependentVariable:yStepwiseSelection:Step1Variablex4Entered:R-Square=0.8102andC(p)=27.0270AnalysisofVarianceSumofMeanSourceDFSquaresSquareFValuePr>FModel1121018801210188068.31<.0001Error162834772177173CorrectedTotal1714936652ParameterStandardVariableEstimateErrorTypeIISSFValuePr>FIntercept-105872288.08824379286121.410.0003x4194.8582223.577161210188068.31<.0001Boundsonconditionnumber:1,1----------------------------------------------------------------------------Allvariablesleftinthemodelaresignificantatthe0.2500level.Noothervariablemetthe0.2500significancelevelforentryintothemodel.SummaryofStepwiseSelectionVariableVariableNumberPartialModelStepEnteredRemovedVarsInR-SquareR-SquareC(p)FValuePr>F1x410.81020.810227.027068.31<.0001筛选的结果,只有X4一个变量保留在方程中。②进一步提高显著水平,这次使用sle=0.01和sls=0.01筛选,结果见下表:TheSASSystemTheREGProcedureModel:MODEL1DependentVariable:yStepwiseSelection:Step1Variablex4Entered:R-Square=0.8102andC(p)=27.0270AnalysisofVarianceSumofMeanSourceDFSquaresSquareFValuePr>FModel1121018801210188068.31<.0001Error162834772177173CorrectedTotal1714936652ParameterStandardVariableEstimateErrorTypeIISSFValuePr>FIntercept-105872288.08824379286121.410.0003nx4194.8582223.577161210188068.31<.0001Boundsonconditionnumber:1,1----------------------------------------------------------------------------Allvariablesleftinthemodelaresignificantatthe0.0100level.Noothervariablemetthe0.0100significancelevelforentryintothemodel.SummaryofStepwiseSelectionVariableVariableNumberPartialModelStepEnteredRemovedVarsInR-SquareR-SquareC(p)FValuePr>F1x410.81020.810227.027068.31<.0001其结果X4仍保留在方程中。实际上,在第一步的结果中已经给出X4的显著性概率P<0.0001,这一步完全可以省略。③选择sle=0.30和sls=0.25。TheSASSystemTheREGProcedureModel:MODEL1DependentVariable:yStepwiseSelection:Step1Variablex4Entered:R-Square=0.8102andC(p)=27.0270AnalysisofVarianceSumofMeanSourceDFSquaresSquareFValuePr>FModel1121018801210188068.31<.0001Error162834772177173CorrectedTotal1714936652ParameterStandardVariableEstimateErrorTypeIISSFValuePr>FIntercept-105872288.08824379286121.410.0003x4194.8582223.577161210188068.31<.0001Boundsonconditionnumber:1,1----------------------------------------------------------------------------StepwiseSelection:Step2Variablex3Entered:R-Square=0.8262andC(p)=25.5717AnalysisofVarianceSumofMeanSourceDFSquaresSquareFValuePr>FModel212340628617031435.65<.0001Error152596025173068CorrectedTotal1714936652ParameterStandardVariableEstimateErrorTypeIISSFValuePr>FIntercept-105612261.52833377445621.810.0003nx37.257796.179362387481.380.2585x4168.3044032.46742465064226.870.0001Boundsonconditionnumber:1.9413,7.7652----------------------------------------------------------------------------StepwiseSelection:Step3Variablex3Removed:R-Square=0.8102andC(p)=27.0270AnalysisofVarianceSumofMeanSourceDFSquaresSquareFValuePr>FModel1121018801210188068.31<.0001Error162834772177173CorrectedTotal1714936652ParameterStandardVariableEstimateErrorTypeIISSFValuePr>FIntercept-105872288.08824379286121.410.0003x4194.8582223.577161210188068.31<.0001Boundsonconditionnumber:1,1----------------------------------------------------------------------------Allvariablesleftinthemodelaresignificantatthe0.2500level.Thestepwisemethodterminatedbecausethenextvariabletobeenteredwasjustremoved.SummaryofStepwiseSelectionVariableVariableNumberPartialModelStepEnteredRemovedVarsInR-SquareR-SquareC(p)FValuePr>F1x410.81020.810227.027068.31<.00012x320.01600.826225.57171.380.25853x310.01600.810227.02701.380.2585其结果虽然X3被引进,由于sls=0.25,与上一步是相同的,最后仍被剔除,方程中仍然只有X4一个变量。④以下是sle=0.30和sls=0.30的结果:TheSASSystemTheREGProcedureModel:MODEL1DependentVariable:yStepwiseSelection:Step1Variablex4Entered:R-Square=0.8102andC(p)=27.0270AnalysisofVarianceSumofMeanSourceDFSquaresSquareFValuePr>FModel1121018801210188068.31<.0001Error162834772177173CorrectedTotal1714936652ParameterStandardVariableEstimateErrorTypeIISSFValuePr>FnIntercept-105872288.08824379286121.410.0003x4194.8582223.577161210188068.31<.0001Boundsonconditionnumber:1,1----------------------------------------------------------------------------StepwiseSelection:Step2Variablex3Entered:R-Square=0.8262andC(p)=25.5717AnalysisofVarianceSumofMeanSourceDFSquaresSquareFValuePr>FModel212340628617031435.65<.0001Error152596025173068CorrectedTotal1714936652ParameterStandardVariableEstimateErrorTypeIISSFValuePr>FIntercept-105612261.52833377445621.810.0003x37.257796.179362387481.380.2585x4168.3044032.46742465064226.870.0001Boundsonconditionnumber:1.9413,7.7652----------------------------------------------------------------------------StepwiseSelection:Step3Variablex2Entered:R-Square=0.8698andC(p)=18.1420AnalysisofVarianceSumofMeanSourceDFSquaresSquareFValuePr>FModel312992174433072531.18<.0001Error141944479138891CorrectedTotal1714936652ParameterStandardVariableEstimateErrorTypeIISSFValuePr>FIntercept-104882026.24419372104326.790.0001x239.8737818.409946515464.690.0481x315.412596.694787361295.300.0372x488.3183847.008414902603.530.0813Boundsonconditionnumber:5.071,31.81----------------------------------------------------------------------------StepwiseSelection:Step4Variablex1Entered:R-Square=0.9399andC(p)=5.0000AnalysisofVarianceSumofMeanSourceDFSquaresSquareFValuePr>FModel414038414350960350.79<.0001Error1389823969095CorrectedTotal1714936652nParameterStandardVariableEstimateErrorTypeIISSFValuePr>FIntercept-312455522.29553221185532.01<.0001x1839.98368215.86358104624015.140.0019x265.8977014.60597140646320.360.0006x323.223495.13090141552020.490.0006x417.3875637.83632145920.210.6534Boundsonconditionnumber:6.6037,58.735----------------------------------------------------------------------------StepwiseSelection:Step5Variablex4Removed:R-Square=0.9389andC(p)=3.2112AnalysisofVarianceSumofMeanSourceDFSquaresSquareFValuePr>FModel314023822467460771.69<.0001Error1491283065202CorrectedTotal1714936652ParameterStandardVariableEstimateErrorTypeIISSFValuePr>FIntercept-321605003.04317269421641.32<.0001x1887.77442183.75513152190823.340.0003x271.486287.85845539552182.75<.0001x325.185452.76451541159083.00<.0001Boundsonconditionnumber:1.0452,9.2712----------------------------------------------------------------------------Allvariablesleftinthemodelaresignificantatthe0.3000level.Noothervariablemetthe0.3000significancelevelforentryintothemodel.SummaryofStepwiseSelectionVariableVariableNumberPartialModelStepEnteredRemovedVarsInR-SquareR-SquareC(p)FValuePr>F1x410.81020.810227.027068.31<.00012x320.01600.826225.57171.380.25853x230.04360.869818.14204.690.04814x140.07000.93995.000015.140.00195x430.00100.93893.21120.210.6534这次变量X4首先被引进,然后逐次引进X3,X2和X1,引进X1后,X4反倒变得不显著,从方程中被剔除了。为了说明其原因,我们先看一看下面的相关系数矩阵表。从该表中可以看出,X4与另外三个自变量的相关系数,除X1外都达到了极显著,而另外的三个自变量间的相关都不显著。这就说明在把X1,X2和X3引进方程中之后,X4的对Y的贡献在很大程度上被另外的三个自变量取代,使X4变得不那么重要了。换句话说,变量X4在很大程度上代表了另外三个自变量对Y的贡献。因此,在方程中只要保留X4已经是一个很有代表性的方程了。根据以上的分析,最优方程应当是:PearsonCorrelationCoefficients,N=18nProb>|r|underH0:Rho=0yx1x2x3x4y1.000000.250260.683280.717520.900120.31650.00180.0008<.0001x10.250261.00000-0.11527-0.000530.146160.31650.64880.99830.5628x20.68328-0.115271.000000.173200.675920.00180.64880.49190.0021x30.71752-0.000530.173201.000000.696330.00080.99830.49190.0013x40.900120.146160.675920.696331.00000<.00010.56280.00210.0013查看更多