- 2022-08-29 发布 |
- 37.5 KB |
- 86页
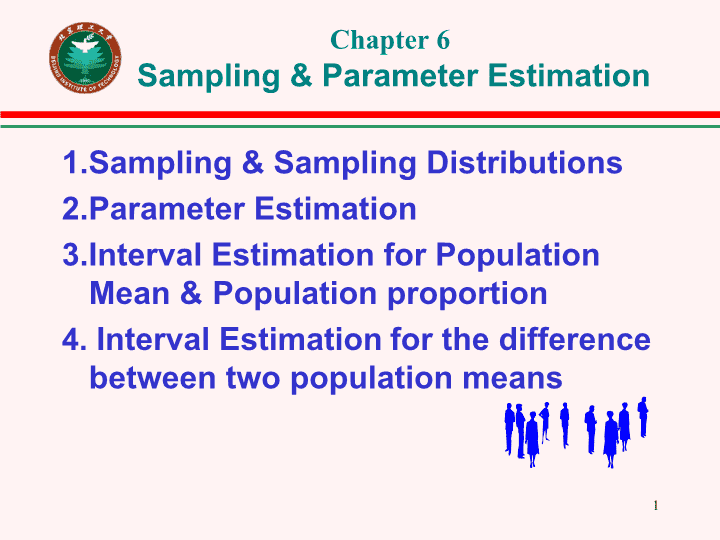
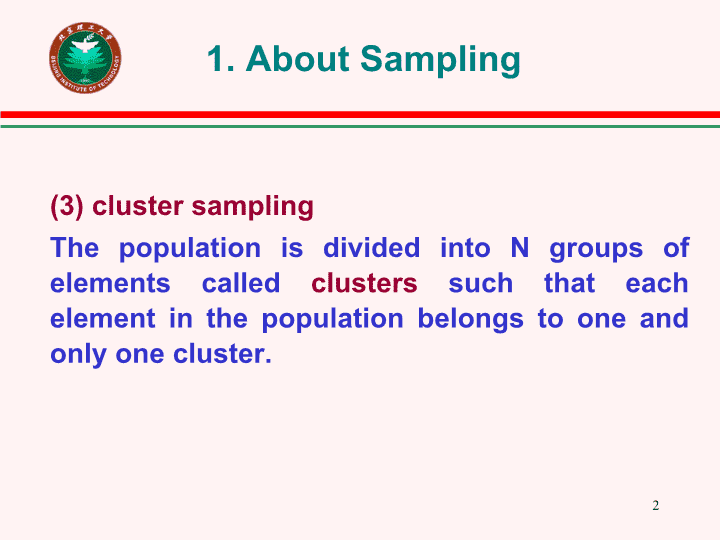
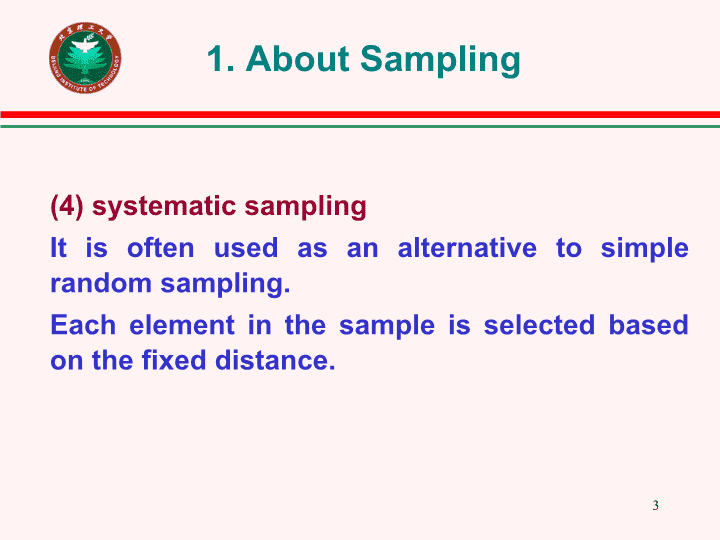
申明敬告: 本站不保证该用户上传的文档完整性,不预览、不比对内容而直接下载产生的反悔问题本站不予受理。
文档介绍
MBA统计学第6章
1.Sampling&SamplingDistributions2.ParameterEstimation3.IntervalEstimationforPopulationMean&Populationproportion4.IntervalEstimationforthedifferencebetweentwopopulationmeansChapter6Sampling&ParameterEstimation1\n(3)clustersamplingThepopulationisdividedintoNgroupsofelementscalledclusterssuchthateachelementinthepopulationbelongstooneandonlyonecluster.1.AboutSampling2\n(4)systematicsamplingItisoftenusedasanalternativetosimplerandomsampling.Eachelementinthesampleisselectedbasedonthefixeddistance.1.AboutSampling3\n1.3TheSampleStatisticItisthefunctionofsample(Forexample,,S2,etc.)。Statisticalsohasthetwofoldproperty.ThedistributionthatStatisticfollowsissamplingdistribution.Wewillexplaintheconceptfurther.1.AboutSampling4\nThereisasampleconsistedof30employeesinacompany,theirincomeandratioofmanagementtrainingareinvestigated.=2000yuan,s=200yuan,ratiop=0.70Now,selectanother30employees,(asimplerandomsample):=2010yuan,s=202yuan,ratiop=0.722.SamplingDistribution5\nRepeatthisprocedure,wecanobtainalargeamountofestimatorsabout,s,pWecansketchhistograminaccordancewiththeseoutcomes.,s,pcanbetakenasrandomvariable.Fromtheseoutcomes,wecanalsoestimatethemean,varianceandprobabilitydistribution,i.e.,samplingdistribution.2.SamplingDistribution6\n2.SamplingDistributionSamplingdistributionisgenerallycomplicated,butitiseasyforaNormalpopulation.2.1X1…..XniidfollowN(μ,σ2),then∽N(μ,)(Whendealingwithalargesample,(n≥30),thenormalassumptionofthepopulationdoesnotneeded.)seep1257\nTheCentralLimitTheorem(seep126)Inselectingsimplerandomsamplesofsizenfromapopulation,thesamplingdistributionofthesamplemeancanbeapproximatedbyanormalprobabilitydistributionasthesamplesizebecomeslarge(n>30).Wecanseeafigurewhichistheillustrationofthetheoremforthreedifferentpopulations.Seep127,example6.28\n2.SamplingDistribution2.2TheChi-squareDistribution(seep127)(AsymmetricDistribution)X1…….XniidfollowN(0,1),2=∑Xi2∽2(n)n—df(degreeoffreedom)Generallycompute2:P(2(n)>2)=,(criticalvalue2)seethedistributiontableatpage370Example:=0.05,n=10,then20.05(10)=18.307seep128,densityfunctionof2(n)9\n2.3studenttDistribution(symmetricdistributiom)Whenn≥30,itapproximatestheNormalDistributionItalsohasthedegreeoffreedom.(p130)Weoftencompute:t/2two-tailedpercentile:P(T>t/2)=(criticalvaluet/2)tone-tailedpercentile:P(T>t)=seethetableatpage368Forexample:=0.1t/2(21)=1.721,t(21)=1.3232.SamplingDistribution10\nThetDistribution(p130)Zt0t(df=5)TheNormalDistributiont(df=13)Bell-ShapedSymmetryLarge-Tailed11\nStudent’stdistributiontable(p368)Tailareaontherightside.df.25.10.0511.0003.0786.31420.8171.8862.92030.7651.6382.353t0Suppose:n=3df=n-1=2=.10/2=.052.920Criticalvalueoft./2.0512\nFdistributionF(n1-1,n2-1)distribution,thefirstdfn1-1,theseconddfn2-1,seethedensityofFstatisticonp129seethetableofcriticalvalueFponp37313\n3.1PointEstimatorMomentEstimation:Substitutesamplemomentsforpopulationmoments.(Populationmomentsincludemean,variance,etc.)Substituterelativefrequencyforprobability3.ParameterEstimation14\nNotice:①Whenusingthismethod,therelationshipbetweenpopulationcharacteristicsandparametersmustbeknown.②SamplemeanSamplevariance3.ParameterEstimation15\nExample:X∽N(μ,σ2),ThisindicatesthatXisthepointestimatorofparameterμ,othersituationscanbeexplainedbythesameway.Example:X∽F(x)=1-e-λx,Mean=1/λThen∴Example:Xfollowstheuniformdistributionon[a,b].=,Fromthesetwoequations,compute3.ParameterEstimation16\n2、Thecriterionsofpointestimators(1)UnbiasednessE()=seepage135Example:、themedianareallunbiasedestimatorsofthemeanparameter(μ).S2istheunbiasedestimatorofthevarianceparameter(σ2)ParameterEstimation17\n(2)EffectivenessIfthetwounbiasedestimatorsθ1,θ2ofparameterθsatisfy:E(θ1-θ)2≤E(θ2-θ)2,wesaythatθ1ismoreeffectivethanθ2。Forexample,ismoreeffectivethanthemedian.is“theminimumvarianceunbiasedestimator”inallthemeanestimators.ParameterEstimation18\n(3)Consistencyseep135IflimP(|1-|<)=1,then1istheestimatorofparameterthatsatisfiestheconsistency.ParameterEstimation19\nParameterEstimationUnbiasedBiasedCAUnbiasedness20\nParameterEstimationsamplingdistributionofthemediansamplingdistributionofthemeanABEffectiveness21\nParameterEstimationsamplesizeissmallersamplesizeislargerABConsistency22\n3.IntervalEstimationTheideaaboutintervalestimation:Determineanintervalofaparameter,whichcanensurethattheparameteriswithinitwithalargeprobability.(Generally,thisintervalshouldincludethepointestimatoroftheparameter)。Example:Supposex1,x2……,xnisasamplefromthepopulationN(μ,32),trytodetermineaninterval,whichcanensurethattheparameterμiswithinitwithprobability0.95。(seethenextpage)IntervalEstimation23\nSolution:ItisknownthatfollowsN(0,1)BecauseWecanlookupinthenormaldistributiontableandgetTherefore,wegetiscalledthedegreeofconfidence.Theintervalincludingμiscalledtheconfidenceinterval.ParameterEstimation?1984-1994T/MakerCo.24\nTheintervalestimationofthepopulationmeanSupposeX1…..XniidfollowN(μ,σ2),isthepointestimatorofμ.Computeμ’sconfidenceintervalwiththedegreeofconfidence1-.WhenσisknownBecausez=followsN(0,1),TheconfidenceintervalwegetisNote:Asforthenon-normalpopulation,whenn>30,zstatisticstillcanbeusedforintervalestimation,andσcanbesubstitutedbyS.25\nWhenσisunknown(smallsample)Itisknownthatt=followst(n-1),WegettheconfidenceintervalGgenerally,thesymmetricalintervalhasshortestlength.Theintervalestimationofthepopulationmeanσisunknownt26\nInpointestimation,unbiasednessandeffectivenessareusedasthecriteriaforjudgingthequalityoftheestimator。Inintervalestimation,thedegreeofconfidenceandthewidthoftheintervalareusedforassessingthequalityoftheinterval.ParameterEstimation27\nNoticethefollowingrelationsWhennremainsunchanged,if1-αincreases,thenincreasesaccordingly;thatmeanswhenthewidthincreases,precisiondecreases.Therefore,theincreaseofconfidencelevelisattheexpenseofthedecreaseofprecision.When1-αremainsunchanged,ifnincreases,thenthewidthdecreasesandtheprecisionincreases.However,ifnistoolarge,wastingwillbecausedandsamplingbecomesmeaningless.Therefore,theprecisionshouldbeselectedcarefully.ParameterEstimation28\nDeterminingthesamplesize=Z/2iscalledpermissibleerroriscalledstandarderror标准误wecangettheconfidenceintervalwithExcel(seeaexampleonp154)ParameterEstimation29\nIntervalestimationforpopulationproportion(p141)Ifnp5,nq5,thenpfollowsN(p,p(1-p)/n)normaldistributionconfidenceintervalestimateofapopulationproportionis(6.30)p142ParameterEstimation30\nThisisanotherissueofstatisticalinference,focusingongettingtheconclusionof“Yes”or“No”.Background:Afterimprovingtechnology,doestheaverageproductsizechangesignificantly?Afterimprovingtechnology,whethertheproductionisstableornot?Isthequalifiedrateuptothestandard?Doesthelifeoftheproductfollowthenormaldistribution?Etc.Chapter7HypothesisTests(p156)31\nWhenconsideringtheabovequestions,wecanassumethatthehypothesisistenable,thenaccordingtosample,judgewhetherthehypothesisisrightornot.Ifitisright,thenacceptthehypothesis;ifnot,thenrejectthehypothesis.ThesearethecontentHypothesisTestscovers.Generally,thehypothesistobetestediscalledtheNullHypothesis(H0),theoppositehypothesisiscalledtheAlternativeHypothesis(H1).HypothesisTests32\nHypothesisTestsProcessPopulationSupposeTheaverageageofpopulationis50.(H0)RejecthypothesisSamplemeanis20Sample33\nItstheoreticalbaseistheprincipleofsmallprobability:Inoneexperiment,theeventwithsmallprobabilityhardlyhappens.Example:H0:=0=200mm,H1:0=200mmItisknownthatthepopulationXfollowsN(,σ2),ifH0istenable,thenwegeti.e.itappearswithalargeprobability.Theoppositeeventappearswithasmallprobability.Aftersampling,compute:TheIdeaofHypothesisTests34\nIf,thenthereisnocontradiction.Iftheoppositeappears,thenitisprovedthattheeventwithsmallprobabilityhappensinoneexperiment,whichcontradictswiththeprincipleofsmallprobabilityandprovesthatH0iswrong.HypothesisTests35\nStepsforHypothesisTestsEstablishthenullhypothesisandthealternativehypothesisDeterminethesignificancelevelSelecttheteststatisticComputethevalueofstatisticandmakeacomparisonwiththecriticalvalue36\nIfthestatisticislargerthanthecriticalvalue,rejectH0.Ifthestatisticissmallerthanthecriticalvalue,acceptH0.Ifthestatisticequalstothecriticalvalue,thenenlargethesamplesize,andmakearetesting.HypothesisTests37\nTestinghypothesesconcerningthepopulationmeanWhenσisknown,useZstatistic,whenσisunknown,usetstatistic.Asforalargesample,whateverdistributionitis,Zstatisticcanbeusedasapproximation.TestingahypothesizedvalueofthepopulationproportionTestingthedifferencebetweentwomeansThehypothesistestaboutthepopulationvariance.ContentofTheHypothesisTests38\nIsthereasignificantdifferencebetweenthenetassetincomerateof1993、1994ofcommercialcorporationslistedinShanghaiStockExchange?Solution:SupposetheincomerateofthetwoyearsisX1,X2,andfollowsthenormaldistributionN(1,σ12),N(2,σ22)respectively.Method1:=1-2Method2:Usetheformulaatpage170。AnExampleAboutHypothesisTestsFIRSTPROVISIONSNEWWORLDNortheastHUALIANJINANDEPARTMENTSTORE1993(1)7.95.67.7511.01994(2)12.3513.961.236.1639\nWhenH0istrue,H0mayberejected(causedbystochasticfactors),wecallthiskindoferrorRejectingTruthError.Fromthepriorformulawecanknow,thiskindofprobabilityisα,itisalsocalledTypeⅠErrororSupplierRisk.WhenH0isfalse,H0maybeaccepted(causedbystochasticfactors),wecallthiskindoferrorAcceptingFalsenessError.Itsprobabilityis,itisalsocalledTypeⅡErrororUserRisk.Ingeneral,wecontrolαinmosttime,ourlecturedoesnotcoverthecomputingaspectof.(Ifthesamplesizeisn’tenlarged,thetwotypesofriskscannotbereducedsimultaneously)TwoTypesofErrors40\n&HaveanInverseRelationship0001AcceptH0RejectH0=0=1>041\n&HaveanInverseRelationshipThetwotypesofriskscannotbereducedsimultaneously!42\nOne-tailHypothesisTestsSamplemean=50SamplingDistributionThenthesamplemeanisnotpossibletobethisvalue.IfthepopulationmeanistrueTherefore,rejecttheH0hypothesis=50.20H0BasicIdea43\nLevelofSignificance1.Definition:Itistheintervalthat,ifthenullhypothesisistenable,samplestatisticsareimpossiblewithinit.Itisalsocalled“Rejectionregionofsamplingdistribution”2.DenotedbyTypicalvaluesare0.01,0.05,0.103.Determinedbyresearchstaffwhenateststarts.44\nZ-TestStatistic(Known)Transformsamplestatistic(i.g.)intothestandardnormaldistributionvariableZ.ComparewiththecriticalvalueofZ.Ifthevalueoftestedstatisticiswithinthecriticalregion,thenrejectH0;ifnot,acceptH0ZXXnxx45\npValueTestThep-value,theobservedlevelofsignificance,isameasureofthelikelihoodofthesampleresultswhenthenullhypothesisisassumedtobetrue.Thesmallerthep-value,thelesslikelyitisthatthesampleresultscamefromasituationwherethenullhypothesisistrue.Ifp,donotrejectH0Ifp<,rejectH0.46\nOne-TailZTestaboutMean(Known)Weassumethepopulationfollowsanormaldistribution,whenn30,anon-normaldistributioncanbeapproximatedbyanormaldistribution.2.NullhypothesisonlyusesorTestStatisticZZXXnxx47\nRejectionRegionZ0rejectH0Z0rejectH0H0:0H1:<0H0:0H1:>0Onlywhenstatisticissignificantlylessthanthatitwillberejected.SmallervaluedoesnotcontradictwithH0,therefore,H0willnotberejected.48\nOne-TailZTest:FindingCriticalZValuesZ.05.071.6.4505.4515.45251.7.4599.4608.46161.8.4678.4686.4693.4744.4756Z0Z=11.96.500-.025.475.061.9.4750TableofStandardNormalDistribution(Part)When=0.025,computeZ?=.02549\nTestaboutp-valueP(Z1.50)=0.0668Z01.50p-value=.0668ZvalueofsamplestatisticInZtable,find:1.50.4332.5000-.4332.0668Determinethedirectionoftestbyusingthealternativehypothesis.50\nTestaboutp-value01.50ZrejectH0(p=0.0668)(=0.05).DonotrejectH0=0.05Teststatisticisnotwithintherejectionregion.51\nTwo-TailedZTestforMean(Known)Assumeapopulationfollowsthenormaldistribution,whenn30,thepopulationofanon-normaldistributioncanbeapproximatedbyanormaldistribution.Thynullhypothesisisanequality.TestStatisticZXXZnxx52\nRejectionRegionsH0criticalvaluecriticalvalue1/21/2samplestatisticrejectionregionnon-rejectionregionsamplingdistribution1-confidencelevelrejectionregion53\nTwo-TailedTest:FindingCriticalZvaluesZ.05.071.6.4505.4515.45251.7.4599.4608.46161.8.4678.4686.4693.4744.4756Z0Z=11.96-1.96.500-.025.475.061.9.4750When=0.05,computeZ?/2=.025/2=.02554\nTestaboutp-valueP(Z-1.50orZ1.50)=0.1336Z01.50-1.501/2p=.06681/2p=.0668Zvalueofsamplestatistic.4332FindtheprobabilityofZ=1.50.5000-.4332.0668multiply255\nTestaboutp-value(p=.1336)(=.05)donotrejectthenullhypothesis01.50-1.50ZrejectH0rejectH01/2p=.06681/2p=.06681/2=.0251/2=.025Teststatisticisnotwithintherejectionregion.56\nAmanagerofahotelclaimsthatthemeanofthebillsattheendofeveryweekissmallerorequalto400yuan.However,anaccountantofthishotelfindsthatthetotalincomeisincreasingintherecentmonth.Thisaccountantwilluseasampleconsistedofthebillsofrecentweekendstotestifythemanager’sclaim.a.Whatkindofhypothesisshouldbeused?H0:≥400,H0:≤400,H0:=400b.Inthisexample,what’sthemeaningofrejectingH0?ExercisesforHypothesistests57\nAnewweight-reducingmethodclaimsthattheparticipantwillaveragelyloseatleast8kginthefirstweek.40participantsconsistofarandomsample,thesamplemeanofthereducedweightis7kg,thestandarddeviationis3.2kg.Compute:a.Whena=0.05,whatistherejectioncriterion?b.Whatisyourconclusionaboutthisweight-reducingmethod?ExercisesforHypothesistestsFITTNESSCLUBWelcome58\nRelationshipamongvariablesFunctionCorrelation(statisticalrelationship)YdependsonX,butisn’tmerelydeterminedbyX.Example:price—demandforproducttemperature—demandforair-conditioningRegression—Accordingtoobservantdata,establishregressionmodelandmakestatisticalreferenceonvariableshavingstatisticalrelationship.Chapter10Regression59\nWhatdoesregressiondo?Solvethefollowingproblems:Determinewhethertherehasstatisticalrelationshipamongvariables,ifhas,showtheformula.Forecastthevalueofanothervariableaccordingtoonevariableoragroupofvariables.60\nLinearRegressionAssumptionsNormalityEveryvalueofX,YfollowsthenormaldistributionTheerrorprobabilityfollowsthenormaldistributionHomoscedasticity(ConstantVariance)IndependenceofErrorsLinearity61\nExample:X-price,Y-demandfortheproductWehavedata:1.Scatterplot2.Regressionequation(OrdinaryLeastSquareEstimation)3.CorrelationcoefficientrTestingtheregressionmodel4.Forecasting5.RegressioncanbelinearitiedSimpleLinearRegressionX(Yuan)708090100110Y(thousand)11.2511.2811.6511.7012.1462\nLinearRegressionModelVariablesconsistofalinearfunction.YXiii01SlopeY-InterceptIndependent(Explanatory)VariableDependent(Response)VariableRandomError63\nPopulationLinearRegressionModeli=randomerrorXYXiX01YXiii01ObservedValueObservedValueY64\nSampleLinearRegressionModelei=randomerrorYXYbbXeiii01^YbbXii01UnsampledObservedValueSampledObservedValue65\nOrdinaryLeastSquaresTheleastsquaresmethodprovidesanestimatedregressionequationthatminimizesthesumofsquareddeviationsbetweentheobservedvaluesofthedependentvariableyiandtheestimatedvaluesofthedependentvariable.e2YXe1e3e4YbbXeiii01^YbbXii01OLSMineeeeeii2112223242PredictedValue66\nCoefficient&EquationsYbXbXYnXYXnXbYbXiiiiiniin011122101SampleregressionequationSlopefortheestimatedregressionequationInterceptfortheestimatedregressionequationb67\nEvaluatingtheModelTestCoefficientofDeterminationandStandardDeviationofEstimationResidualAnalysisTestCoefficientsofSignificance^YbbXii0168\nMeasuresofVariationinRegression1.TotalSumofSquares(SST)MeasurethevariationbetweentheobservedvalueYiandthemeanY.2.ExplainedVariation(SSR)VariationcausedbytherelationshipbetweenXandY.3.UnexplainedVariation(SSE)Variationcausedbyotherfactors.69\nVariationMeasuresYXYXiSST(Yi-Y)2SSE(Yi-Yi)2^SSR(Yi-Y)2^Yi^YbbXii0170\nCoefficientofDetermination0r21rbYbXYnYYnYiiiininiin201211212ExplainedvariationTotalvariationSSRSSTAmeasureofthegoodnessoffitoftheestimatedregressionequation.Itcanbeinterpretedastheproportionofthevariationinthedependentvariableythatisexplainedbytheestimatedregressionequation.71\nCorrelationCoefficientAnumericalmeasureoflinearassociationbetweentwovariablesthattakesvaluesbetween–1and+1.Valuesnear+1indicateastrongpositivelinearrelationship,valuesnear–1indicateastrongnegativelinearrelationship,andvaluesnearzeroindicatelackofalinearrelationship.72\nCoefficientsofDetermination(r2)andCorrelation(r)r2=1,r2=0,YYi=b0+b1XiX^YYi=b0+b1XiX^YYi=b0+b1XiX^YYi=b0+b1XiX^r=+1r=-1r=+0.9r=073\nTestofSlopeCoefficientforSignificance1.TestsaLinearRelationshipBetweenX&Y2.HypothesesH0:1=0(NoLinearRelationship)H1:10(LinearRelationship)3.TestStatistic74\nExampleTestofSlopeCoefficientH0:1=0H1:10.05df5-2=3Criticalvalue:Statistic:Determine:Conclusion:tbSb1110700019153655...Rejectat=0.05Thereisevidenceofarelationship.t03.1824-3.1824.025RejectReject.02575\nMultipleRegressionModelThereexistslinearrelationshipamongandependentvariableandtwoormorethantwoindependentvariables.YXXXiiiPPii01122slopeofpopulationinterceptofpopulationYrandomerrorDependentVariableIndependentVariables76\nExample:NewYorkTimesYouworkintheadvertisementdepartmentofNewYorkTimes(NYT).Youwillfindtowhatextentdoadssize(squareinch)andpublishingvolume(thousand)influencetheresponsetoads(hundred).Youhavecollectedthefollowingdata:responsesizevolume112488131357264410677\nExample(NYT)ComputerOutputParameterEstimatesParameterStandardTforH0:VariableDFEstimateErrorParam=0Prob>|T|INTERCEP10.06400.25990.2460.8214ADSIZE10.20490.05883.6560.0399CIRC10.28050.06864.0890.0264b2b0bPb178\nInterpretationofCoefficients1.Slope(b1)Ifthepublishingvolumeremainsunchanged,whenadssizeincreasesonesquareinch,theresponseisexpectedtoincrease0.2049hundredtimes.2.Slope(b2)Ifadssizeremainsunchanged,whenpublishingvolumeincreasesonethousand,theresponseisexpectedtoin-crease0.2805hundredtimes.79\nEvaluatingtheModel1.Howdoesthemodeldescribetherelationshipamongvariables?2.Closenessof‘BestFit’3.Assumptionsmet4.Significanceofestimates5.Correlationamongvariables6.Outliers(unusualobservations)80\nTestingOverallSignificanceTestwhetherthereislinearrelationshipbetweenYandalltheindependentvariables.2.UseFstatistic.HypothesisH0:1=2=...=P=0ThereisnolinearrelationshipbetweenYandindependentvariables.H1:Atleastthereisacoefficientisn’tequalto0.AtleastthereisanindependentvariableinfluencesY81\nTestingOverallSignificanceComputerOutputAnalysisofVarianceSumofMeanSourceDFSquaresSquareFValueProb>FModel29.24974.624955.4400.0043Error30.25030.0834CTotal59.5000Pn-P-1n-1MSR/MSEpValue82\nTransformationsinRegressionModelsNon-linearmodelsthatcanbetransformedintolinearmodels(convenienttocarryoutOLS).DataTransformationMultiplicativeModelExampleYXXYXXiiiiiiii0120112212lnlnlnlnln83\nSquare-RootTransformationYXXiiii011221>01<0YX184\nLogarithmicTransformationYXXiiii01122lnln1>01<0YX185\nExponentialTransformationYiieXXii011221>01<0YX186查看更多