- 2022-08-22 发布 |
- 37.5 KB |
- 786页
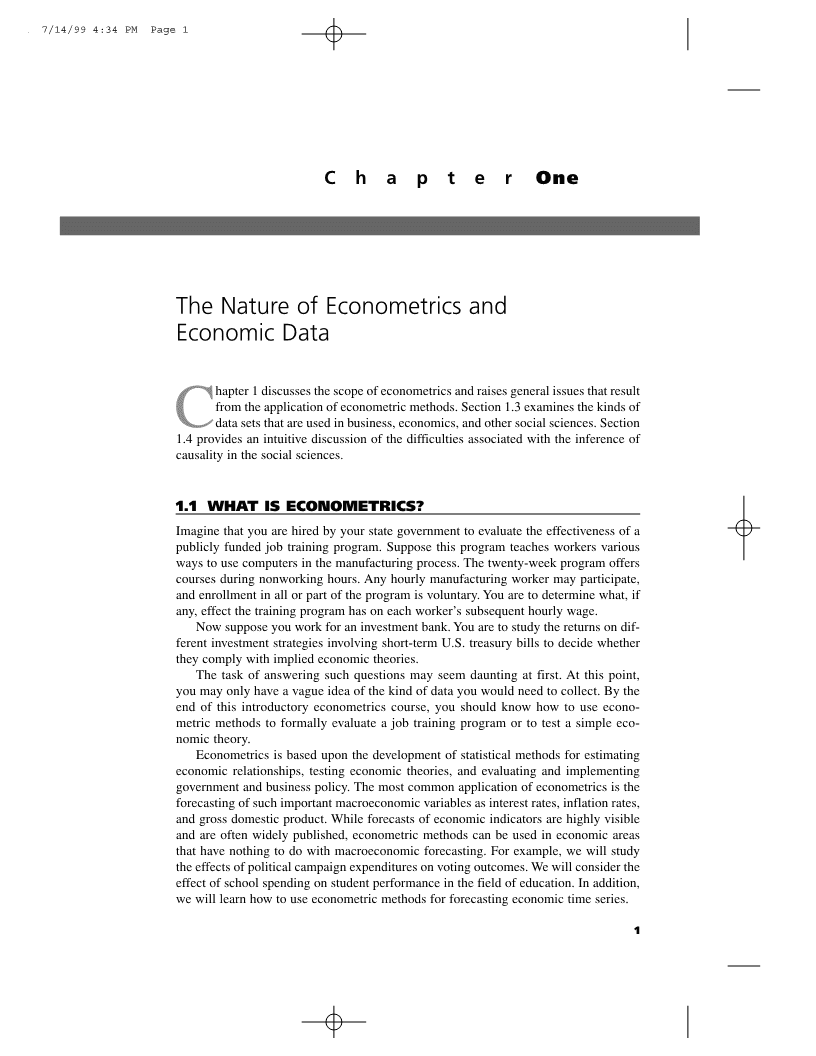
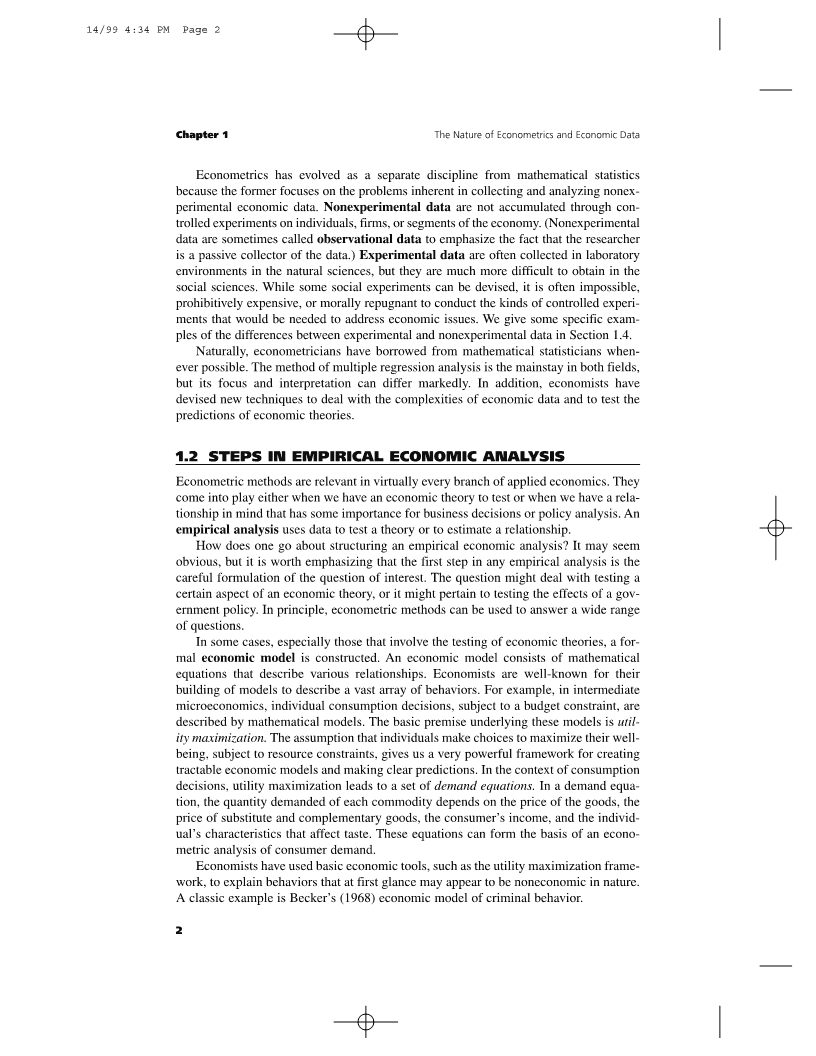
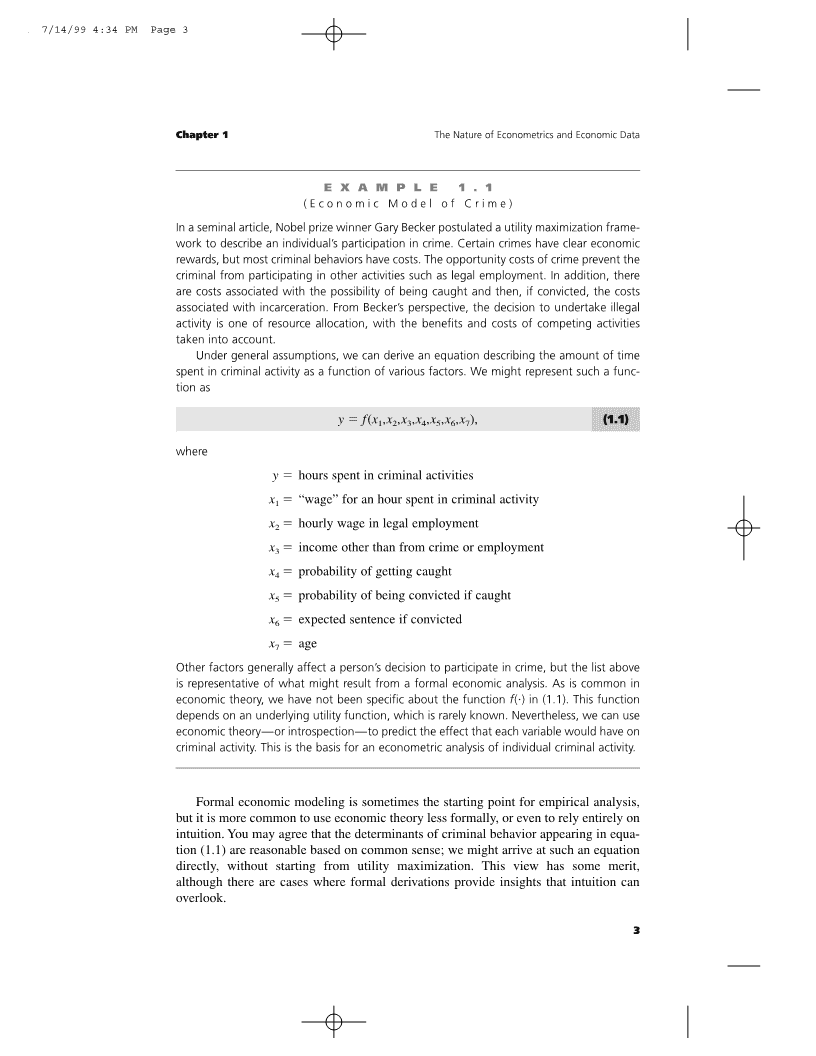
申明敬告: 本站不保证该用户上传的文档完整性,不预览、不比对内容而直接下载产生的反悔问题本站不予受理。
文档介绍
英文原版经济学系列-计量经济学导论:现代视角
d7/14/994:34PMPage1ChapterOneTheNatureofEconometricsandEconomicDatahapter1discussesthescopeofeconometricsandraisesgeneralissuesthatresultCfromtheapplicationofeconometricmethods.Section1.3examinesthekindsofdatasetsthatareusedinbusiness,economics,andothersocialsciences.Section1.4providesanintuitivediscussionofthedifficultiesassociatedwiththeinferenceofcausalityinthesocialsciences.1.1WHATISECONOMETRICS?Imaginethatyouarehiredbyyourstategovernmenttoevaluatetheeffectivenessofapubliclyfundedjobtrainingprogram.Supposethisprogramteachesworkersvariouswaystousecomputersinthemanufacturingprocess.Thetwenty-weekprogramofferscoursesduringnonworkinghours.Anyhourlymanufacturingworkermayparticipate,andenrollmentinallorpartoftheprogramisvoluntary.Youaretodeterminewhat,ifany,effectthetrainingprogramhasoneachworker’ssubsequenthourlywage.Nowsupposeyouworkforaninvestmentbank.Youaretostudythereturnsondif-ferentinvestmentstrategiesinvolvingshort-termU.S.treasurybillstodecidewhethertheycomplywithimpliedeconomictheories.Thetaskofansweringsuchquestionsmayseemdauntingatfirst.Atthispoint,youmayonlyhaveavagueideaofthekindofdatayouwouldneedtocollect.Bytheendofthisintroductoryeconometricscourse,youshouldknowhowtouseecono-metricmethodstoformallyevaluateajobtrainingprogramortotestasimpleeco-nomictheory.Econometricsisbaseduponthedevelopmentofstatisticalmethodsforestimatingeconomicrelationships,testingeconomictheories,andevaluatingandimplementinggovernmentandbusinesspolicy.Themostcommonapplicationofeconometricsistheforecastingofsuchimportantmacroeconomicvariablesasinterestrates,inflationrates,andgrossdomesticproduct.Whileforecastsofeconomicindicatorsarehighlyvisibleandareoftenwidelypublished,econometricmethodscanbeusedineconomicareasthathavenothingtodowithmacroeconomicforecasting.Forexample,wewillstudytheeffectsofpoliticalcampaignexpendituresonvotingoutcomes.Wewillconsidertheeffectofschoolspendingonstudentperformanceinthefieldofeducation.Inaddition,wewilllearnhowtouseeconometricmethodsforforecastingeconomictimeseries.1\n14/994:34PMPage2Chapter1TheNatureofEconometricsandEconomicDataEconometricshasevolvedasaseparatedisciplinefrommathematicalstatisticsbecausetheformerfocusesontheproblemsinherentincollectingandanalyzingnonex-perimentaleconomicdata.Nonexperimentaldataarenotaccumulatedthroughcon-trolledexperimentsonindividuals,firms,orsegmentsoftheeconomy.(Nonexperimentaldataaresometimescalledobservationaldatatoemphasizethefactthattheresearcherisapassivecollectorofthedata.)Experimentaldataareoftencollectedinlaboratoryenvironmentsinthenaturalsciences,buttheyaremuchmoredifficulttoobtaininthesocialsciences.Whilesomesocialexperimentscanbedevised,itisoftenimpossible,prohibitivelyexpensive,ormorallyrepugnanttoconductthekindsofcontrolledexperi-mentsthatwouldbeneededtoaddresseconomicissues.Wegivesomespecificexam-plesofthedifferencesbetweenexperimentalandnonexperimentaldatainSection1.4.Naturally,econometricianshaveborrowedfrommathematicalstatisticianswhen-everpossible.Themethodofmultipleregressionanalysisisthemainstayinbothfields,butitsfocusandinterpretationcandiffermarkedly.Inaddition,economistshavedevisednewtechniquestodealwiththecomplexitiesofeconomicdataandtotestthepredictionsofeconomictheories.1.2STEPSINEMPIRICALECONOMICANALYSISEconometricmethodsarerelevantinvirtuallyeverybranchofappliedeconomics.Theycomeintoplayeitherwhenwehaveaneconomictheorytotestorwhenwehavearela-tionshipinmindthathassomeimportanceforbusinessdecisionsorpolicyanalysis.Anempiricalanalysisusesdatatotestatheoryortoestimatearelationship.Howdoesonegoaboutstructuringanempiricaleconomicanalysis?Itmayseemobvious,butitisworthemphasizingthatthefirststepinanyempiricalanalysisisthecarefulformulationofthequestionofinterest.Thequestionmightdealwithtestingacertainaspectofaneconomictheory,oritmightpertaintotestingtheeffectsofagov-ernmentpolicy.Inprinciple,econometricmethodscanbeusedtoanswerawiderangeofquestions.Insomecases,especiallythosethatinvolvethetestingofeconomictheories,afor-maleconomicmodelisconstructed.Aneconomicmodelconsistsofmathematicalequationsthatdescribevariousrelationships.Economistsarewell-knownfortheirbuildingofmodelstodescribeavastarrayofbehaviors.Forexample,inintermediatemicroeconomics,individualconsumptiondecisions,subjecttoabudgetconstraint,aredescribedbymathematicalmodels.Thebasicpremiseunderlyingthesemodelsisutil-itymaximization.Theassumptionthatindividualsmakechoicestomaximizetheirwell-being,subjecttoresourceconstraints,givesusaverypowerfulframeworkforcreatingtractableeconomicmodelsandmakingclearpredictions.Inthecontextofconsumptiondecisions,utilitymaximizationleadstoasetofdemandequations.Inademandequa-tion,thequantitydemandedofeachcommoditydependsonthepriceofthegoods,thepriceofsubstituteandcomplementarygoods,theconsumer’sincome,andtheindivid-ual’scharacteristicsthataffecttaste.Theseequationscanformthebasisofanecono-metricanalysisofconsumerdemand.Economistshaveusedbasiceconomictools,suchastheutilitymaximizationframe-work,toexplainbehaviorsthatatfirstglancemayappeartobenoneconomicinnature.AclassicexampleisBecker’s(1968)economicmodelofcriminalbehavior.2\nd7/14/994:34PMPage3Chapter1TheNatureofEconometricsandEconomicDataEXAMPLE1.1(EconomicModelofCrime)Inaseminalarticle,NobelprizewinnerGaryBeckerpostulatedautilitymaximizationframe-worktodescribeanindividual’sparticipationincrime.Certaincrimeshavecleareconomicrewards,butmostcriminalbehaviorshavecosts.Theopportunitycostsofcrimepreventthecriminalfromparticipatinginotheractivitiessuchaslegalemployment.Inaddition,therearecostsassociatedwiththepossibilityofbeingcaughtandthen,ifconvicted,thecostsassociatedwithincarceration.FromBecker’sperspective,thedecisiontoundertakeillegalactivityisoneofresourceallocation,withthebenefitsandcostsofcompetingactivitiestakenintoaccount.Undergeneralassumptions,wecanderiveanequationdescribingtheamountoftimespentincriminalactivityasafunctionofvariousfactors.Wemightrepresentsuchafunc-tionasyf(x1,x2,x3,x4,x5,x6,x7),(1.1)whereyhoursspentincriminalactivitiesx1“wage”foranhourspentincriminalactivityx2hourlywageinlegalemploymentx3incomeotherthanfromcrimeoremploymentx4probabilityofgettingcaughtx5probabilityofbeingconvictedifcaughtx6expectedsentenceifconvictedx7ageOtherfactorsgenerallyaffectaperson’sdecisiontoparticipateincrime,butthelistaboveisrepresentativeofwhatmightresultfromaformaleconomicanalysis.Asiscommonineconomictheory,wehavenotbeenspecificaboutthefunctionf()in(1.1).Thisfunctiondependsonanunderlyingutilityfunction,whichisrarelyknown.Nevertheless,wecanuseeconomictheory—orintrospection—topredicttheeffectthateachvariablewouldhaveoncriminalactivity.Thisisthebasisforaneconometricanalysisofindividualcriminalactivity.Formaleconomicmodelingissometimesthestartingpointforempiricalanalysis,butitismorecommontouseeconomictheorylessformally,oreventorelyentirelyonintuition.Youmayagreethatthedeterminantsofcriminalbehaviorappearinginequa-tion(1.1)arereasonablebasedoncommonsense;wemightarriveatsuchanequationdirectly,withoutstartingfromutilitymaximization.Thisviewhassomemerit,althoughtherearecaseswhereformalderivationsprovideinsightsthatintuitioncanoverlook.3\n14/994:34PMPage4Chapter1TheNatureofEconometricsandEconomicDataHereisanexampleofanequationthatwasderivedthroughsomewhatinformalreasoning.EXAMPLE1.2(JobTrainingandWorkerProductivity)ConsidertheproblemposedatthebeginningofSection1.1.Alaboreconomistwouldliketoexaminetheeffectsofjobtrainingonworkerproductivity.Inthiscase,thereislittleneedforformaleconomictheory.Basiceconomicunderstandingissufficientforrealizingthatfactorssuchaseducation,experience,andtrainingaffectworkerproductivity.Also,econ-omistsarewellawarethatworkersarepaidcommensuratewiththeirproductivity.Thissim-plereasoningleadstoamodelsuchaswagef(educ,exper,training)(1.2)wherewageishourlywage,educisyearsofformaleducation,experisyearsofworkforceexperience,andtrainingisweeksspentinjobtraining.Again,otherfactorsgenerallyaffectthewagerate,but(1.2)capturestheessenceoftheproblem.Afterwespecifyaneconomicmodel,weneedtoturnitintowhatwecallanecono-metricmodel.Sincewewilldealwitheconometricmodelsthroughoutthistext,itisimportanttoknowhowaneconometricmodelrelatestoaneconomicmodel.Takeequa-tion(1.1)asanexample.Theformofthefunctionf()mustbespecifiedbeforewecanundertakeaneconometricanalysis.Asecondissueconcerning(1.1)ishowtodealwithvariablesthatcannotreasonablybeobserved.Forexample,considerthewagethatapersoncanearnincriminalactivity.Inprinciple,suchaquantityiswell-defined,butitwouldbedifficultifnotimpossibletoobservethiswageforagivenindividual.Evenvariablessuchastheprobabilityofbeingarrestedcannotrealisticallybeobtainedforagivenindividual,butatleastwecanobserverelevantarreststatisticsandderiveavari-ablethatapproximatestheprobabilityofarrest.Manyotherfactorsaffectcriminalbehaviorthatwecannotevenlist,letaloneobserve,butwemustsomehowaccountforthem.Theambiguitiesinherentintheeconomicmodelofcrimeareresolvedbyspecify-ingaparticulareconometricmodel:crime0+1wagem+2othinc3freqarr4freqconv(1.3)5avgsen6ageu,wherecrimeissomemeasureofthefrequencyofcriminalactivity,wagemisthewagethatcanbeearnedinlegalemployment,othincistheincomefromothersources(assets,inheritance,etc.),freqarristhefrequencyofarrestsforpriorinfractions(toapproxi-matetheprobabilityofarrest),freqconvisthefrequencyofconviction,andavgsenistheaveragesentencelengthafterconviction.Thechoiceofthesevariablesisdeter-minedbytheeconomictheoryaswellasdataconsiderations.Thetermucontainsunob-4\nd7/14/994:34PMPage5Chapter1TheNatureofEconometricsandEconomicDataservedfactors,suchasthewageforcriminalactivity,moralcharacter,familyback-ground,anderrorsinmeasuringthingslikecriminalactivityandtheprobabilityofarrest.Wecouldaddfamilybackgroundvariablestothemodel,suchasnumberofsib-lings,parents’education,andsoon,butwecannevereliminateuentirely.Infact,deal-ingwiththiserrortermordisturbancetermisperhapsthemostimportantcomponentofanyeconometricanalysis.Theconstants0,1,…,6aretheparametersoftheeconometricmodel,andtheydescribethedirectionsandstrengthsoftherelationshipbetweencrimeandthefactorsusedtodeterminecrimeinthemodel.AcompleteeconometricmodelforExample1.2mightbewage01educ2exper3trainingu,(1.4)wherethetermucontainsfactorssuchas“innateability,”qualityofeducation,familybackground,andthemyriadotherfactorsthatcaninfluenceaperson’swage.Ifwearespecificallyconcernedabouttheeffectsofjobtraining,then3istheparameterofinterest.Forthemostpart,econometricanalysisbeginsbyspecifyinganeconometricmodel,withoutconsiderationofthedetailsofthemodel’screation.Wegenerallyfollowthisapproach,largelybecausecarefulderivationofsomethingliketheeconomicmodelofcrimeistimeconsumingandcantakeusintosomespecializedandoftendifficultareasofeconomictheory.Economicreasoningwillplayaroleinourexamples,andwewillmergeanyunderlyingeconomictheoryintotheeconometricmodelspecification.Intheeconomicmodelofcrimeexample,wewouldstartwithaneconometricmodelsuchas(1.3)anduseeconomicreasoningandcommonsenseasguidesforchoosingthevari-ables.Whilethisapproachlosessomeoftherichnessofeconomicanalysis,itiscom-monlyandeffectivelyappliedbycarefulresearchers.Onceaneconometricmodelsuchas(1.3)or(1.4)hasbeenspecified,varioushypothesesofinterestcanbestatedintermsoftheunknownparameters.Forexample,inequation(1.3)wemighthypothesizethatwagem,thewagethatcanbeearnedinlegalemployment,hasnoeffectoncriminalbehavior.Inthecontextofthisparticularecono-metricmodel,thehypothesisisequivalentto10.Anempiricalanalysis,bydefinition,requiresdata.Afterdataontherelevantvari-ableshavebeencollected,econometricmethodsareusedtoestimatetheparametersintheeconometricmodelandtoformallytesthypothesesofinterest.Insomecases,theeconometricmodelisusedtomakepredictionsineitherthetestingofatheoryorthestudyofapolicy’simpact.Becausedatacollectionissoimportantinempiricalwork,Section1.3willdescribethekindsofdatathatwearelikelytoencounter.1.3THESTRUCTUREOFECONOMICDATAEconomicdatasetscomeinavarietyoftypes.Whilesomeeconometricmethodscanbeappliedwithlittleornomodificationtomanydifferentkindsofdatasets,thespe-cialfeaturesofsomedatasetsmustbeaccountedfororshouldbeexploited.Wenextdescribethemostimportantdatastructuresencounteredinappliedwork.5\n14/994:34PMPage6Chapter1TheNatureofEconometricsandEconomicDataCross-SectionalDataAcross-sectionaldatasetconsistsofasampleofindividuals,households,firms,cities,states,countries,oravarietyofotherunits,takenatagivenpointintime.Sometimesthedataonallunitsdonotcorrespondtopreciselythesametimeperiod.Forexample,severalfamiliesmaybesurveyedduringdifferentweekswithinayear.Inapurecrosssectionanalysiswewouldignoreanyminortimingdifferencesincollectingthedata.Ifasetoffamilieswassurveyedduringdifferentweeksofthesameyear,wewouldstillviewthisasacross-sectionaldataset.Animportantfeatureofcross-sectionaldataisthatwecanoftenassumethattheyhavebeenobtainedbyrandomsamplingfromtheunderlyingpopulation.Forexam-ple,ifweobtaininformationonwages,education,experience,andothercharacteristicsbyrandomlydrawing500peoplefromtheworkingpopulation,thenwehavearandomsamplefromthepopulationofallworkingpeople.Randomsamplingisthesamplingschemecoveredinintroductorystatisticscourses,anditsimplifiestheanalysisofcross-sectionaldata.AreviewofrandomsamplingiscontainedinAppendixC.Sometimesrandomsamplingisnotappropriateasanassumptionforanalyzingcross-sectionaldata.Forexample,supposeweareinterestedinstudyingfactorsthatinfluencetheaccumulationoffamilywealth.Wecouldsurveyarandomsampleoffam-ilies,butsomefamiliesmightrefusetoreporttheirwealth.If,forexample,wealthierfamiliesarelesslikelytodisclosetheirwealth,thentheresultingsampleonwealthisnotarandomsamplefromthepopulationofallfamilies.Thisisanillustrationofasam-pleselectionproblem,anadvancedtopicthatwewilldiscussinChapter17.Anotherviolationofrandomsamplingoccurswhenwesamplefromunitsthatarelargerelativetothepopulation,particularlygeographicalunits.Thepotentialprobleminsuchcasesisthatthepopulationisnotlargeenoughtoreasonablyassumetheobser-vationsareindependentdraws.Forexample,ifwewanttoexplainnewbusinessactiv-ityacrossstatesasafunctionofwagerates,energyprices,corporateandpropertytaxrates,servicesprovided,qualityoftheworkforce,andotherstatecharacteristics,itisunlikelythatbusinessactivitiesinstatesnearoneanotherareindependent.Itturnsoutthattheeconometricmethodsthatwediscussdoworkinsuchsituations,buttheysome-timesneedtoberefined.Forthemostpart,wewillignoretheintricaciesthatariseinanalyzingsuchsituationsandtreattheseproblemsinarandomsamplingframework,evenwhenitisnottechnicallycorrecttodoso.Cross-sectionaldataarewidelyusedineconomicsandothersocialsciences.Ineco-nomics,theanalysisofcross-sectionaldataiscloselyalignedwiththeappliedmicro-economicsfields,suchaslaboreconomics,stateandlocalpublicfinance,industrialorganization,urbaneconomics,demography,andhealtheconomics.Dataonindividu-als,households,firms,andcitiesatagivenpointintimeareimportantfortestingmicro-economichypothesesandevaluatingeconomicpolicies.Thecross-sectionaldatausedforeconometricanalysiscanberepresentedandstoredincomputers.Table1.1contains,inabbreviatedform,across-sectionaldataseton526workingindividualsfortheyear1976.(ThisisasubsetofthedatainthefileWAGE1.RAW.)Thevariablesincludewage(indollarsperhour),educ(yearsofeduca-tion),exper(yearsofpotentiallaborforceexperience),female(anindicatorforgender),andmarried(maritalstatus).Theselasttwovariablesarebinary(zero-one)innature6\nd7/14/994:34PMPage7Chapter1TheNatureofEconometricsandEconomicDataTable1.1ACross-SectionalDataSetonWagesandOtherIndividualCharacteristicsobsnowageeducexperfemalemarried13.101121023.2412221133.001120046.008440155.301270152511.56165015263.5014510andservetoindicatequalitativefeaturesoftheindividual.(Thepersonisfemaleornot;thepersonismarriedornot.)WewillhavemuchtosayaboutbinaryvariablesinChapter7andbeyond.ThevariableobsnoinTable1.1istheobservationnumberassignedtoeachpersoninthesample.Unliketheothervariables,itisnotacharacteristicoftheindividual.Alleconometricsandstatisticssoftwarepackagesassignanobservationnumbertoeachdataunit.Intuitionshouldtellyouthat,fordatasuchasthatinTable1.1,itdoesnotmatterwhichpersonislabeledasobservationone,whichpersoniscalledObservationTwo,andsoon.Thefactthattheorderingofthedatadoesnotmatterforeconometricanalysisisakeyfeatureofcross-sectionaldatasetsobtainedfromrandomsampling.Differentvariablessometimescorrespondtodifferenttimeperiodsincross-sectionaldatasets.Forexample,inordertodeterminetheeffectsofgovernmentpoli-ciesonlong-termeconomicgrowth,economistshavestudiedtherelationshipbetweengrowthinrealpercapitagrossdomesticproduct(GDP)overacertainperiod(say1960to1985)andvariablesdeterminedinpartbygovernmentpolicyin1960(governmentconsumptionasapercentageofGDPandadultsecondaryeducationrates).SuchadatasetmightberepresentedasinTable1.2,whichconstitutespartofthedatasetusedinthestudyofcross-countrygrowthratesbyDeLongandSummers(1991).7\n14/994:34PMPage8Chapter1TheNatureofEconometricsandEconomicDataTable1.2ADataSetonEconomicGrowthRatesandCountryCharacteristicsobsnocountrygpcrgdpgovcons60second601Argentina0.899322Austria3.3216503Belgium2.5613694Bolivia1.24181261Zimbabwe2.30176ThevariablegpcrgdprepresentsaveragegrowthinrealpercapitaGDPovertheperiod1960to1985.Thefactthatgovcons60(governmentconsumptionasapercentageofGDP)andsecond60(percentofadultpopulationwithasecondaryeducation)corre-spondtotheyear1960,whilegpcrgdpistheaveragegrowthovertheperiodfrom1960to1985,doesnotleadtoanyspecialproblemsintreatingthisinformationasacross-sectionaldataset.Theorderoftheobservationsislistedalphabeticallybycountry,butthereisnothingaboutthisorderingthataffectsanysubsequentanalysis.TimeSeriesDataAtimeseriesdatasetconsistsofobservationsonavariableorseveralvariablesovertime.Examplesoftimeseriesdataincludestockprices,moneysupply,consumerpriceindex,grossdomesticproduct,annualhomiciderates,andautomobilesalesfigures.Becausepasteventscaninfluencefutureeventsandlagsinbehaviorareprevalentinthesocialsciences,timeisanimportantdimensioninatimeseriesdataset.Unlikethearrangementofcross-sectionaldata,thechronologicalorderingofobservationsinatimeseriesconveyspotentiallyimportantinformation.Akeyfeatureoftimeseriesdatathatmakesitmoredifficulttoanalyzethancross-sectionaldataisthefactthateconomicobservationscanrarely,ifever,beassumedtobeindependentacrosstime.Mosteconomicandothertimeseriesarerelated,oftenstronglyrelated,totheirrecenthistories.Forexample,knowingsomethingaboutthegrossdomesticproductfromlastquartertellsusquiteabitaboutthelikelyrangeoftheGDPduringthisquarter,sinceGDPtendstoremainfairlystablefromonequarterto8\nd7/14/994:34PMPage9Chapter1TheNatureofEconometricsandEconomicDatathenext.Whilemosteconometricprocedurescanbeusedwithbothcross-sectionalandtimeseriesdata,moreneedstobedoneinspecifyingeconometricmodelsfortimeseriesdatabeforestandardeconometricmethodscanbejustified.Inaddition,modifi-cationsandembellishmentstostandardeconometrictechniqueshavebeendevelopedtoaccountforandexploitthedependentnatureofeconomictimeseriesandtoaddressotherissues,suchasthefactthatsomeeconomicvariablestendtodisplaycleartrendsovertime.Anotherfeatureoftimeseriesdatathatcanrequirespecialattentionisthedatafre-quencyatwhichthedataarecollected.Ineconomics,themostcommonfrequenciesaredaily,weekly,monthly,quarterly,andannually.Stockpricesarerecordedatdailyintervals(excludingSaturdayandSunday).ThemoneysupplyintheU.S.economyisreportedweekly.Manymacroeconomicseriesaretabulatedmonthly,includinginfla-tionandemploymentrates.Othermacroseriesarerecordedlessfrequently,suchaseverythreemonths(everyquarter).Grossdomesticproductisanimportantexampleofaquarterlyseries.Othertimeseries,suchasinfantmortalityratesforstatesintheUnitedStates,areavailableonlyonanannualbasis.Manyweekly,monthly,andquarterlyeconomictimeseriesdisplayastrongseasonalpattern,whichcanbeanimportantfactorinatimeseriesanalysis.Forex-ample,monthlydataonhousingstartsdiffersacrossthemonthssimplyduetochangingweatherconditions.WewilllearnhowtodealwithseasonaltimeseriesinChapter10.Table1.3containsatimeseriesdatasetobtainedfromanarticlebyCastillo-FreemanandFreeman(1992)onminimumwageeffectsinPuertoRico.Theearliestyearinthedatasetisthefirstobservation,andthemostrecentyearavailableisthelastTable1.3MinimumWage,Unemployment,andRelatedDataforPuertoRicoobsnoyearavgminavgcovunempgnp119500.2020.115.4878.7219510.2120.716.0925.0319520.2322.614.81015.93719863.3558.118.94281.63819873.3558.216.84496.79\n14/994:34PMPage10Chapter1TheNatureofEconometricsandEconomicDataobservation.Wheneconometricmethodsareusedtoanalyzetimeseriesdata,thedatashouldbestoredinchronologicalorder.Thevariableavgminreferstotheaverageminimumwagefortheyear,avgcovistheaveragecoveragerate(thepercentageofworkerscoveredbytheminimumwagelaw),unempistheunemploymentrate,andgnpisthegrossnationalproduct.Wewillusethesedatalaterinatimeseriesanalysisoftheeffectoftheminimumwageonemployment.PooledCrossSectionsSomedatasetshavebothcross-sectionalandtimeseriesfeatures.Forexample,supposethattwocross-sectionalhouseholdsurveysaretakenintheUnitedStates,onein1985andonein1990.In1985,arandomsampleofhouseholdsissurveyedforvariablessuchasincome,savings,familysize,andsoon.In1990,anewrandomsampleofhouseholdsistakenusingthesamesurveyquestions.Inordertoincreaseoursamplesize,wecanformapooledcrosssectionbycombiningthetwoyears.Becauserandomsamplesaretakenineachyear,itwouldbeaflukeifthesamehouseholdappearedinthesampleduringbothyears.(Thesizeofthesampleisusuallyverysmallcomparedwiththenum-berofhouseholdsintheUnitedStates.)Thisimportantfactordistinguishesapooledcrosssectionfromapaneldataset.Poolingcrosssectionsfromdifferentyearsisoftenaneffectivewayofanalyzingtheeffectsofanewgovernmentpolicy.Theideaistocollectdatafromtheyearsbeforeandafterakeypolicychange.Asanexample,considerthefollowingdatasetonhous-ingpricestakenin1993and1995,whentherewasareductioninpropertytaxesin1994.Supposewehavedataon250housesfor1993andon270housesfor1995.OnewaytostoresuchadatasetisgiveninTable1.4.Observations1through250correspondtothehousessoldin1993,andobservations251through520correspondtothe270housessoldin1995.Whiletheorderinwhichwestorethedataturnsoutnottobecrucial,keepingtrackoftheyearforeachobser-vationisusuallyveryimportant.Thisiswhyweenteryearasaseparatevariable.Apooledcrosssectionisanalyzedmuchlikeastandardcrosssection,exceptthatweoftenneedtoaccountforseculardifferencesinthevariablesacrossthetime.Infact,inadditiontoincreasingthesamplesize,thepointofapooledcross-sectionalanalysisisoftentoseehowakeyrelationshiphaschangedovertime.PanelorLongitudinalDataApaneldata(orlongitudinaldata)setconsistsofatimeseriesforeachcross-sectionalmemberinthedataset.Asanexample,supposewehavewage,education,andemploymenthistoryforasetofindividualsfollowedoveraten-yearperiod.Orwemightcollectinformation,suchasinvestmentandfinancialdata,aboutthesamesetoffirmsoverafive-yeartimeperiod.Paneldatacanalsobecollectedongeographicalunits.Forexample,wecancollectdataforthesamesetofcountiesintheUnitedStatesonimmigrationflows,taxrates,wagerates,governmentexpenditures,etc.,fortheyears1980,1985,and1990.Thekeyfeatureofpaneldatathatdistinguishesitfromapooledcrosssectionisthefactthatthesamecross-sectionalunits(individuals,firms,orcountiesintheabove10\nd7/14/994:34PMPage11Chapter1TheNatureofEconometricsandEconomicDataTable1.4PooledCrossSections:TwoYearsofHousingPricesobsnoyearhpriceproptaxsqrftbdrmsbthrms119938550042160032.0219936730036144032.53199313400038200042.5250199324360041260043.025119956500016125021.0252199518240020220042.025319959750015154032.052019955720016110021.5examples)arefollowedoveragiventimeperiod.ThedatainTable1.4arenotconsid-eredapaneldatasetbecausethehousessoldarelikelytobedifferentin1993and1995;ifthereareanyduplicates,thenumberislikelytobesosmallastobeunimportant.Incontrast,Table1.5containsatwo-yearpaneldatasetoncrimeandrelatedstatisticsfor150citiesintheUnitedStates.ThereareseveralinterestingfeaturesinTable1.5.First,eachcityhasbeengivenanumberfrom1through150.Whichcitywedecidetocallcity1,city2,andsoon,isirrelevant.Aswithapurecrosssection,theorderinginthecrosssectionofapaneldatasetdoesnotmatter.Wecouldusethecitynameinplaceofanumber,butitisoftenuse-fultohaveboth.11\n14/994:34PMPage12Chapter1TheNatureofEconometricsandEconomicDataTable1.5ATwo-YearPanelDataSetonCityCrimeStatisticsobsnocityyearmurderspopulationunempolice11198653500008.744021199083592007.24713219862643005.4754219901651005.5752971491986102607009.6286298149199062450009.83342991501986255430004.35203001501990325462005.2493Asecondusefulpointisthatthetwoyearsofdataforcity1fillthefirsttworowsorobservations.Observations3and4correspondtocity2,andsoon.Sinceeachofthe150citieshastworowsofdata,anyeconometricspackagewillviewthisas300obser-vations.Thisdatasetcanbetreatedastwopooledcrosssections,wherethesamecitieshappentoshowupinthesameyear.But,aswewillseeinChapters13and14,wecanalsousethepanelstructuretorespondtoquestionsthatcannotbeansweredbysimplyviewingthisasapooledcrosssection.InorganizingtheobservationsinTable1.5,weplacethetwoyearsofdataforeachcityadjacenttooneanother,withthefirstyearcomingbeforethesecondinallcases.Forjustabouteverypracticalpurpose,thisisthepreferredwayfororderingpaneldatasets.ContrastthisorganizationwiththewaythepooledcrosssectionsarestoredinTable1.4.Inshort,thereasonfororderingpaneldataasinTable1.5isthatwewillneedtoperformdatatransformationsforeachcityacrossthetwoyears.Becausepaneldatarequirereplicationofthesameunitsovertime,paneldatasets,especiallythoseonindividuals,households,andfirms,aremoredifficulttoobtainthanpooledcrosssections.Notsurprisingly,observingthesameunitsovertimeleadstosev-12\nd7/14/994:34PMPage13Chapter1TheNatureofEconometricsandEconomicDataeraladvantagesovercross-sectionaldataorevenpooledcross-sectionaldata.Theben-efitthatwewillfocusoninthistextisthathavingmultipleobservationsonthesameunitsallowsustocontrolcertainunobservedcharacteristicsofindividuals,firms,andsoon.Aswewillsee,theuseofmorethanoneobservationcanfacilitatecausalinfer-enceinsituationswhereinferringcausalitywouldbeverydifficultifonlyasinglecrosssectionwereavailable.Asecondadvantageofpaneldataisthatitoftenallowsustostudytheimportanceoflagsinbehaviorortheresultofdecisionmaking.Thisinfor-mationcanbesignificantsincemanyeconomicpoliciescanbeexpectedtohaveanimpactonlyaftersometimehaspassed.Mostbooksattheundergraduateleveldonotcontainadiscussionofeconometricmethodsforpaneldata.However,economistsnowrecognizethatsomequestionsaredifficult,ifnotimpossible,toanswersatisfactorilywithoutpaneldata.Asyouwillsee,wecanmakeconsiderableprogresswithsimplepaneldataanalysis,amethodwhichisnotmuchmoredifficultthandealingwithastandardcross-sectionaldataset.ACommentonDataStructuresPart1ofthistextisconcernedwiththeanalysisofcross-sectionaldata,asthisposesthefewestconceptualandtechnicaldifficulties.Atthesametime,itillustratesmostofthekeythemesofeconometricanalysis.Wewillusethemethodsandinsightsfromcross-sectionalanalysisintheremainderofthetext.Whiletheeconometricanalysisoftimeseriesusesmanyofthesametoolsascross-sectionalanalysis,itismorecomplicatedduetothetrending,highlypersistentnatureofmanyeconomictimeseries.Examplesthathavebeentraditionallyusedtoillustratethemannerinwhicheconometricmethodscanbeappliedtotimeseriesdataarenowwidelybelievedtobeflawed.Itmakeslittlesensetousesuchexamplesinitially,sincethispracticewillonlyreinforcepooreconometricpractice.Therefore,wewillpostponethetreatmentoftimeserieseconometricsuntilPart2,whentheimportantissuescon-cerningtrends,persistence,dynamics,andseasonalitywillbeintroduced.InPart3,wetreatpooledcrosssectionsandpaneldataexplicitly.Theanalysisofindependentlypooledcrosssectionsandsimplepaneldataanalysisarefairlystraight-forwardextensionsofpurecross-sectionalanalysis.Nevertheless,wewillwaituntilChapter13todealwiththesetopics.1.4CAUSALITYANDTHENOTIONOFCETERISPARIBUSINECONOMETRICANALYSISInmosttestsofeconomictheory,andcertainlyforevaluatingpublicpolicy,theecono-mist’sgoalistoinferthatonevariablehasacausaleffectonanothervariable(suchascrimerateorworkerproductivity).Simplyfindinganassociationbetweentwoormorevariablesmightbesuggestive,butunlesscausalitycanbeestablished,itisrarelycompelling.Thenotionofceterisparibus—whichmeans“other(relevant)factorsbeingequal”—playsanimportantroleincausalanalysis.Thisideahasbeenimplicitinsomeofourearlierdiscussion,particularlyExamples1.1and1.2,butthusfarwehavenotexplicitlymentionedit.13\n14/994:34PMPage14Chapter1TheNatureofEconometricsandEconomicDataYouprobablyrememberfromintroductoryeconomicsthatmosteconomicques-tionsareceterisparibusbynature.Forexample,inanalyzingconsumerdemand,weareinterestedinknowingtheeffectofchangingthepriceofagoodonitsquantityde-manded,whileholdingallotherfactors—suchasincome,pricesofothergoods,andindividualtastes—fixed.Ifotherfactorsarenotheldfixed,thenwecannotknowthecausaleffectofapricechangeonquantitydemanded.Holdingotherfactorsfixediscriticalforpolicyanalysisaswell.Inthejobtrainingexample(Example1.2),wemightbeinterestedintheeffectofanotherweekofjobtrainingonwages,withallothercomponentsbeingequal(inparticular,educationandexperience).Ifwesucceedinholdingallotherrelevantfactorsfixedandthenfindalinkbetweenjobtrainingandwages,wecanconcludethatjobtraininghasacausaleffectonworkerproductivity.Whilethismayseemprettysimple,evenatthisearlystageitshouldbeclearthat,exceptinveryspecialcases,itwillnotbepossibletoliterallyholdallelseequal.Thekeyquestioninmostempiricalstudiesis:Haveenoughotherfactorsbeenheldfixedtomakeacaseforcausality?Rarelyisaneconometricstudyevaluatedwithoutraisingthisissue.Inmostseriousapplications,thenumberoffactorsthatcanaffectthevariableofinterest—suchascriminalactivityorwages—isimmense,andtheisolationofanyparticularvariablemayseemlikeahopelesseffort.However,wewilleventuallyseethat,whencarefullyapplied,econometricmethodscansimulateaceterisparibusexperiment.Atthispoint,wecannotyetexplainhoweconometricmethodscanbeusedtoesti-mateceterisparibuseffects,sowewillconsidersomeproblemsthatcanariseintryingtoinfercausalityineconomics.Wedonotuseanyequationsinthisdiscussion.Foreachexample,theproblemofinferringcausalitydisappearsifanappropriateexperimentcanbecarriedout.Thus,itisusefultodescribehowsuchanexperimentmightbestruc-tured,andtoobservethat,inmostcases,obtainingexperimentaldataisimpractical.Itisalsohelpfultothinkaboutwhytheavailabledatafailstohavetheimportantfeaturesofanexperimentaldataset.Werelyfornowonyourintuitiveunderstandingoftermssuchasrandom,inde-pendence,andcorrelation,allofwhichshouldbefamiliarfromanintroductoryproba-bilityandstatisticscourse.(TheseconceptsarereviewedinAppendixB.)Webeginwithanexamplethatillustratessomeoftheseimportantissues.EXAMPLE1.3(EffectsofFertilizeronCropYield)Someearlyeconometricstudies[forexample,Griliches(1957)]consideredtheeffectsofnewfertilizersoncropyields.Supposethecropunderconsiderationissoybeans.Sincefer-tilizeramountisonlyonefactoraffectingyields—someothersincluderainfall,qualityofland,andpresenceofparasites—thisissuemustbeposedasaceterisparibusquestion.Onewaytodeterminethecausaleffectoffertilizeramountonsoybeanyieldistoconductanexperiment,whichmightincludethefollowingsteps.Chooseseveralone-acreplotsofland.Applydifferentamountsoffertilizertoeachplotandsubsequentlymeasuretheyields;thisgivesusacross-sectionaldataset.Then,usestatisticalmethods(tobeintroducedinChapter2)tomeasuretheassociationbetweenyieldsandfertilizeramounts.14\nd7/14/994:34PMPage15Chapter1TheNatureofEconometricsandEconomicDataAsdescribedearlier,thismaynotseemlikeaverygoodexperiment,becausewehavesaidnothingaboutchoosingplotsoflandthatareidenticalinallrespectsexceptfortheamountoffertilizer.Infact,choosingplotsoflandwiththisfeatureisnotfeasible:someofthefactors,suchaslandquality,cannotevenbefullyobserved.Howdoweknowtheresultsofthisexperimentcanbeusedtomeasuretheceterisparibuseffectoffertilizer?Theanswerdependsonthespecificsofhowfertilizeramountsarechosen.Ifthelevelsoffer-tilizerareassignedtoplotsindependentlyofotherplotfeaturesthataffectyield—thatis,othercharacteristicsofplotsarecompletelyignoredwhendecidingonfertilizeramounts—thenweareinbusiness.WewilljustifythisstatementinChapter2.Thenextexampleismorerepresentativeofthedifficultiesthatarisewheninferringcausalityinappliedeconomics.EXAMPLE1.4(MeasuringtheReturntoEducation)Laboreconomistsandpolicymakershavelongbeeninterestedinthe“returntoeduca-tion.”Somewhatinformally,thequestionisposedasfollows:Ifapersonischosenfromthepopulationandgivenanotheryearofeducation,byhowmuchwillhisorherwageincrease?Aswiththepreviousexamples,thisisaceterisparibusquestion,whichimpliesthatallotherfactorsareheldfixedwhileanotheryearofeducationisgiventotheperson.Wecanimagineasocialplannerdesigninganexperimenttogetatthisissue,muchastheagriculturalresearchercandesignanexperimenttoestimatefertilizereffects.OneapproachistoemulatethefertilizerexperimentinExample1.3:Chooseagroupofpeople,randomlygiveeachpersonanamountofeducation(somepeoplehaveaneighthgradeeducation,somearegivenahighschooleducation,etc.),andthenmeasuretheirwages(assumingthateachthenworksinajob).Thepeoplehereareliketheplotsintheferti-lizerexample,whereeducationplaystheroleoffertilizerandwagerateplaystheroleofsoybeanyield.AswithExample1.3,iflevelsofeducationareassignedindependentlyofothercharacteristicsthataffectproductivity(suchasexperienceandinnateability),thenananalysisthatignorestheseotherfactorswillyieldusefulresults.Again,itwilltakesomeeffortinChapter2tojustifythisclaim;fornowwestateitwithoutsupport.Unlikethefertilizer-yieldexample,theexperimentdescribedinExample1.4isinfeasible.Themoralissues,nottomentiontheeconomiccosts,associatedwithran-domlydeterminingeducationlevelsforagroupofindividualsareobvious.Asalogis-ticalmatter,wecouldnotgivesomeoneonlyaneighthgradeeducationifheorshealreadyhasacollegedegree.Eventhoughexperimentaldatacannotbeobtainedformeasuringthereturntoedu-cation,wecancertainlycollectnonexperimentaldataoneducationlevelsandwagesforalargegroupbysamplingrandomlyfromthepopulationofworkingpeople.Suchdataareavailablefromavarietyofsurveysusedinlaboreconomics,butthesedatasetshaveafeaturethatmakesitdifficulttoestimatetheceterisparibusreturntoeducation.15\n14/994:34PMPage16Chapter1TheNatureofEconometricsandEconomicDataPeoplechoosetheirownlevelsofeducation,andthereforeeducationlevelsareproba-blynotdeterminedindependentlyofallotherfactorsaffectingwage.Thisproblemisafeaturesharedbymostnonexperimentaldatasets.Onefactorthataffectswageisexperienceintheworkforce.Sincepursuingmoreeducationgenerallyrequirespostponingenteringtheworkforce,thosewithmoreedu-cationusuallyhavelessexperience.Thus,inanonexperimentaldatasetonwagesandeducation,educationislikelytobenegativelyassociatedwithakeyvariablethatalsoaffectswage.Itisalsobelievedthatpeoplewithmoreinnateabilityoftenchoosehigherlevelsofeducation.Sincehigherabilityleadstohigherwages,weagainhaveacorrelationbetweeneducationandacriticalfactorthataffectswage.Theomittedfactorsofexperienceandabilityinthewageexamplehaveanalogsinthethefertilizerexample.Experienceisgenerallyeasytomeasureandthereforeissim-ilartoavariablesuchasrainfall.Ability,ontheotherhand,isnebulousanddifficulttoquantify;itissimilartolandqualityinthefertilizerexample.Aswewillseethrough-outthistext,accountingforotherobservedfactors,suchasexperience,whenestimat-ingtheceterisparibuseffectofanothervariable,suchaseducation,isrelativelystraightforward.Wewillalsofindthataccountingforinherentlyunobservablefactors,suchasability,ismuchmoreproblematical.Itisfairtosaythatmanyoftheadvancesineconometricmethodshavetriedtodealwithunobservedfactorsineconometricmodels.OnefinalparallelcanbedrawnbetweenExamples1.3and1.4.Supposethatinthefertilizerexample,thefertilizeramountswerenotentirelydeterminedatrandom.Instead,theassistantwhochosethefertilizerlevelsthoughtitwouldbebettertoputmorefertilizeronthehigherqualityplotsofland.(Agriculturalresearchersshouldhavearoughideaaboutwhichplotsoflandarebetterquality,eventhoughtheymaynotbeabletofullyquantifythedifferences.)ThissituationiscompletelyanalogoustothelevelofschoolingbeingrelatedtounobservedabilityinExample1.4.Becausebetterlandleadstohigheryields,andmorefertilizerwasusedonthebetterplots,anyobservedrelationshipbetweenyieldandfertilizermightbespurious.EXAMPLE1.5(TheEffectofLawEnforcementonCityCrimeLevels)Theissueofhowbesttopreventcrimehas,andwillprobablycontinuetobe,withusforsometime.Oneespeciallyimportantquestioninthisregardis:Doesthepresenceofmorepoliceofficersonthestreetdetercrime?Theceterisparibusquestioniseasytostate:Ifacityisrandomlychosenandgiven10additionalpoliceofficers,byhowmuchwoulditscrimeratesfall?Anotherwaytostatethequestionis:Iftwocitiesarethesameinallrespects,exceptthatcityAhas10morepoliceofficersthancityB,byhowmuchwouldthetwocities’crimeratesdiffer?Itwouldbevirtuallyimpossibletofindpairsofcommunitiesidenticalinallrespectsexceptforthesizeoftheirpoliceforce.Fortunately,econometricanalysisdoesnotrequirethis.Whatwedoneedtoknowiswhetherthedatawecancollectoncommunitycrimelevelsandthesizeofthepoliceforcecanbeviewedasexperimental.Wecancertainlyimagineatrueexperimentinvolvingalargecollectionofcitieswherewedictatehowmanypoliceofficerseachcitywillusefortheupcomingyear.16\nd7/14/994:34PMPage17Chapter1TheNatureofEconometricsandEconomicDataWhilepoliciescanbeusedtoaffectthesizeofpoliceforces,weclearlycannottelleachcityhowmanypoliceofficersitcanhire.If,asislikely,acity’sdecisiononhowmanypoliceofficerstohireiscorrelatedwithothercityfactorsthataffectcrime,thenthedatamustbeviewedasnonexperimental.Infact,onewaytoviewthisproblemistoseethatacity’schoiceofpoliceforcesizeandtheamountofcrimearesimultaneouslydetermined.WewillexplicitlyaddresssuchproblemsinChapter16.Thefirstthreeexampleswehavediscussedhavedealtwithcross-sectionaldataatvariouslevelsofaggregation(forexample,attheindividualorcitylevels).Thesamehurdlesarisewheninferringcausalityintimeseriesproblems.EXAMPLE1.6(TheEffectoftheMinimumWageonUnemployment)Animportant,andperhapscontentious,policyissueconcernstheeffectoftheminimumwageonunemploymentratesforvariousgroupsofworkers.Whilethisproblemcanbestudiedinavarietyofdatasettings(cross-sectional,timeseries,orpaneldata),timeseriesdataareoftenusedtolookataggregateeffects.AnexampleofatimeseriesdatasetonunemploymentratesandminimumwageswasgiveninTable1.3.Standardsupplyanddemandanalysisimpliesthat,astheminimumwageisincreasedabovethemarketclearingwage,weslideupthedemandcurveforlaborandtotalemploy-mentdecreases.(Laborsupplyexceedslabordemand.)Toquantifythiseffect,wecanstudytherelationshipbetweenemploymentandtheminimumwageovertime.Inadditiontosomespecialdifficultiesthatcanariseindealingwithtimeseriesdata,therearepossibleproblemswithinferringcausality.TheminimumwageintheUnitedStatesisnotdeter-minedinavacuum.Variouseconomicandpoliticalforcesimpingeonthefinalminimumwageforanygivenyear.(Theminimumwage,oncedetermined,isusuallyinplaceforsev-eralyears,unlessitisindexedforinflation.)Thus,itisprobablethattheamountofthemin-imumwageisrelatedtootherfactorsthathaveaneffectonemploymentlevels.WecanimaginetheU.S.governmentconductinganexperimenttodeterminetheemploymenteffectsoftheminimumwage(asopposedtoworryingaboutthewelfareoflowwageworkers).Theminimumwagecouldberandomlysetbythegovernmenteachyear,andthentheemploymentoutcomescouldbetabulated.Theresultingexperimentaltimeseriesdatacouldthenbeanalyzedusingfairlysimpleeconometricmethods.Butthisscenariohardlydescribeshowminimumwagesareset.Ifwecancontrolenoughotherfactorsrelatingtoemployment,thenwecanstillhopetoestimatetheceterisparibuseffectoftheminimumwageonemployment.Inthissense,theproblemisverysimilartothepreviouscross-sectionalexamples.Evenwheneconomictheoriesarenotmostnaturallydescribedintermsofcausali-ty,theyoftenhavepredictionsthatcanbetestedusingeconometricmethods.Thefol-lowingisanexampleofthisapproach.17\n14/994:34PMPage18Chapter1TheNatureofEconometricsandEconomicDataEXAMPLE1.7(TheExpectationsHypothesis)Theexpectationshypothesisfromfinancialeconomicsstatesthat,givenallinformationavailabletoinvestorsatthetimeofinvesting,theexpectedreturnonanytwoinvestmentsisthesame.Forexample,considertwopossibleinvestmentswithathree-monthinvestmenthorizon,purchasedatthesametime:(1)Buyathree-monthT-billwithafacevalueof$10,000,forapricebelow$10,000;inthreemonths,youreceive$10,000.(2)Buyasix-monthT-bill(atapricebelow$10,000)and,inthreemonths,sellitasathree-monthT-bill.Eachinvestmentrequiresroughlythesameamountofinitialcapital,butthereisanimpor-tantdifference.Forthefirstinvestment,youknowexactlywhatthereturnisatthetimeofpurchasebecauseyouknowtheinitialpriceofthethree-monthT-bill,alongwithitsfacevalue.Thisisnottrueforthesecondinvestment:whileyouknowthepriceofasix-monthT-billwhenyoupurchaseit,youdonotknowthepriceyoucansellitforinthreemonths.Therefore,thereisuncertaintyinthisinvestmentforsomeonewhohasathree-monthinvestmenthorizon.Theactualreturnsonthesetwoinvestmentswillusuallybedifferent.Accordingtotheexpectationshypothesis,theexpectedreturnfromthesecondinvestment,givenallinfor-mationatthetimeofinvestment,shouldequalthereturnfrompurchasingathree-monthT-bill.Thistheoryturnsouttobefairlyeasytotest,aswewillseeinChapter11.SUMMARYInthisintroductorychapter,wehavediscussedthepurposeandscopeofeconomet-ricanalysis.Econometricsisusedinallappliedeconomicfieldstotesteconomicthe-ories,informgovernmentandprivatepolicymakers,andtopredicteconomictimeseries.Sometimesaneconometricmodelisderivedfromaformaleconomicmodel,butinothercaseseconometricmodelsarebasedoninformaleconomicreasoningandintuition.Thegoalofanyeconometricanalysisistoestimatetheparametersinthemodelandtotesthypothesesabouttheseparameters;thevaluesandsignsoftheparametersdeterminethevalidityofaneconomictheoryandtheeffectsofcertainpolicies.Cross-sectional,timeseries,pooledcross-sectional,andpaneldataarethemostcommontypesofdatastructuresthatareusedinappliedeconometrics.Datasetsinvolvingatimedimension,suchastimeseriesandpaneldata,requirespecialtreat-mentbecauseofthecorrelationacrosstimeofmosteconomictimeseries.Otherissues,suchastrendsandseasonality,ariseintheanalysisoftimeseriesdatabutnotcross-sectionaldata.InSection1.4,wediscussedthenotionsofceterisparibusandcausalinference.Inmostcases,hypothesesinthesocialsciencesareceterisparibusinnature:allotherrel-evantfactorsmustbefixedwhenstudyingtherelationshipbetweentwovariables.Becauseofthenonexperimentalnatureofmostdatacollectedinthesocialsciences,uncoveringcausalrelationshipsisverychallenging.18\nd7/14/994:34PMPage19Chapter1TheNatureofEconometricsandEconomicDataKEYTERMSCausalEffectExperimentalDataCeterisParibusNonexperimentalDataCross-SectionalDataSetObservationalDataDataFrequencyPanelDataEconometricModelPooledCrossSectionEconomicModelRandomSamplingEmpiricalAnalysisTimeSeriesData19\n\nd7/14/994:30PMPage22ChapterTwoTheSimpleRegressionModelhesimpleregressionmodelcanbeusedtostudytherelationshipbetweentwoTvariables.Forreasonswewillsee,thesimpleregressionmodelhaslimita-tionsasageneraltoolforempiricalanalysis.Nevertheless,itissometimesappropriateasanempiricaltool.Learninghowtointerpretthesimpleregressionmodelisgoodpracticeforstudyingmultipleregression,whichwe’lldoinsubse-quentchapters.2.1DEFINITIONOFTHESIMPLEREGRESSIONMODELMuchofappliedeconometricanalysisbeginswiththefollowingpremise:yandxaretwovariables,representatingsomepopulation,andweareinterestedin“explainingyintermsofx,”orin“studyinghowyvarieswithchangesinx.”Wediscussedsomeexam-plesinChapter1,including:yissoybeancropyieldandxisamountoffertilizer;yishourlywageandxisyearsofeducation;yisacommunitycrimerateandxisnumberofpoliceofficers.Inwritingdownamodelthatwill“explainyintermsofx,”wemustconfrontthreeissues.First,sincethereisneveranexactrelationshipbetweentwovariables,howdoweallowforotherfactorstoaffecty?Second,whatisthefunctionalrelationshipbetweenyandx?Andthird,howcanwebesurewearecapturingaceterisparibusrela-tionshipbetweenyandx(ifthatisadesiredgoal)?Wecanresolvetheseambiguitiesbywritingdownanequationrelatingytox.Asimpleequationisy01xu.(2.1)Equation(2.1),whichisassumedtoholdinthepopulationofinterest,definesthesim-plelinearregressionmodel.Itisalsocalledthetwo-variablelinearregressionmodelorbivariatelinearregressionmodelbecauseitrelatesthetwovariablesxandy.Wenowdiscussthemeaningofeachofthequantitiesin(2.1).(Incidentally,theterm“regres-sion”hasoriginsthatarenotespeciallyimportantformostmoderneconometricappli-cations,sowewillnotexplainithere.SeeStigler[1986]foranengaginghistoryofregressionanalysis.)22\nd7/14/994:30PMPage23Chapter2TheSimpleRegressionModelWhenrelatedby(2.1),thevariablesyandxhaveseveraldifferentnamesusedinterchangeably,asfollows.yiscalledthedependentvariable,theexplainedvari-able,theresponsevariable,thepredictedvariable,ortheregressand.xiscalledtheindependentvariable,theexplanatoryvariable,thecontrolvariable,thepre-dictorvariable,ortheregressor.(Thetermcovariateisalsousedforx.)Theterms“dependentvariable”and“independentvariable”arefrequentlyusedineconomet-rics.Butbeawarethatthelabel“independent”heredoesnotrefertothestatisticalnotionofindependencebetweenrandomvariables(seeAppendixB).Theterms“explained”and“explanatory”variablesareprobablythemostdescrip-tive.“Response”and“control”areusedmostlyintheexperimentalsciences,wherethevariablexisundertheexperimenter’scontrol.Wewillnotusetheterms“predictedvari-able”and“predictor,”althoughyousometimesseethese.OurterminologyforsimpleregressionissummarizedinTable2.1.Table2.1TerminologyforSimpleRegressionyxDependentVariableIndependentVariableExplainedVariableExplanatoryVariableResponseVariableControlVariablePredictedVariablePredictorVariableRegressandRegressorThevariableu,calledtheerrortermordisturbanceintherelationship,representsfactorsotherthanxthataffecty.Asimpleregressionanalysiseffectivelytreatsallfac-torsaffectingyotherthanxasbeingunobserved.Youcanusefullythinkofuasstand-ingfor“unobserved.”Equation(2.1)alsoaddressestheissueofthefunctionalrelationshipbetweenyandx.Iftheotherfactorsinuareheldfixed,sothatthechangeinuiszero,u0,thenxhasalineareffectony:y1xifu0.(2.2)Thus,thechangeinyissimply1multipliedbythechangeinx.Thismeansthat1istheslopeparameterintherelationshipbetweenyandxholdingtheotherfactorsinufixed;itisofprimaryinterestinappliedeconomics.Theinterceptparameter0alsohasitsuses,althoughitisrarelycentraltoananalysis.23\nd7/14/994:30PMPage24Part1RegressionAnalysiswithCross-SectionalDataEXAMPLE2.1(SoybeanYieldandFertilizer)Supposethatsoybeanyieldisdeterminedbythemodelyield01fertilizeru,(2.3)sothatyyieldandxfertilizer.Theagriculturalresearcherisinterestedintheeffectoffertilizeronyield,holdingotherfactorsfixed.Thiseffectisgivenby1.Theerrortermucontainsfactorssuchaslandquality,rainfall,andsoon.Thecoefficient1measurestheeffectoffertilizeronyield,holdingotherfactorsfixed:yield1fertilizer.EXAMPLE2.2(ASimpleWageEquation)Amodelrelatingaperson’swagetoobservededucationandotherunobservedfactorsiswage01educu.(2.4)Ifwageismeasuredindollarsperhourandeducisyearsofeducation,then1measuresthechangeinhourlywagegivenanotheryearofeducation,holdingallotherfactorsfixed.Someofthosefactorsincludelaborforceexperience,innateability,tenurewithcurrentemployer,workethics,andinnumerableotherthings.Thelinearityof(2.1)impliesthataone-unitchangeinxhasthesameeffectony,regardlessoftheinitialvalueofx.Thisisunrealisticformanyeconomicapplications.Forexample,inthewage-educationexample,wemightwanttoallowforincreasingreturns:thenextyearofeducationhasalargereffectonwagesthandidthepreviousyear.WewillseehowtoallowforsuchpossibilitiesinSection2.4.Themostdifficultissuetoaddressiswhethermodel(2.1)reallyallowsustodrawceterisparibusconclusionsabouthowxaffectsy.Wejustsawinequation(2.2)that1doesmeasuretheeffectofxony,holdingallotherfactors(inu)fixed.Isthistheendofthecausalityissue?Unfortunately,no.Howcanwehopetolearningeneralabouttheceterisparibuseffectofxony,holdingotherfactorsfixed,whenweareignoringallthoseotherfactors?AswewillseeinSection2.5,weareonlyabletogetreliableestimatorsof0and1fromarandomsampleofdatawhenwemakeanassumptionrestrictinghowtheunobservableuisrelatedtotheexplanatoryvariablex.Withoutsucharestriction,wewillnotbeabletoestimatetheceterisparibuseffect,1.Becauseuandxarerandomvariables,weneedaconceptgroundedinprobability.Beforewestatethekeyassumptionabouthowxanduarerelated,thereisoneassump-tionaboututhatwecanalwaysmake.Aslongastheintercept0isincludedintheequa-tion,nothingislostbyassumingthattheaveragevalueofuinthepopulationiszero.24\nd7/14/994:30PMPage25Chapter2TheSimpleRegressionModelMathematically,E(u)0.(2.5)Importantly,assume(2.5)saysnothingabouttherelationshipbetweenuandxbutsim-plymakesastatementaboutthedistributionoftheunobservablesinthepopulation.Usingthepreviousexamplesforillustration,wecanseethatassumption(2.5)isnotveryrestrictive.InExample2.1,welosenothingbynormalizingtheunobservedfactorsaffect-ingsoybeanyield,suchaslandquality,tohaveanaverageofzerointhepopulationofallcultivatedplots.ThesameistrueoftheunobservedfactorsinExample2.2.Withoutlossofgenerality,wecanassumethatthingssuchasaverageabilityarezerointhepop-ulationofallworkingpeople.Ifyouarenotconvinced,youcanworkthroughProblem2.2toseethatwecanalwaysredefinetheinterceptinequation(2.1)tomake(2.5)true.Wenowturntothecrucialassumptionregardinghowuandxarerelated.Anaturalmeasureoftheassociationbetweentworandomvariablesisthecorrelationcoefficient.(SeeAppendixBfordefinitionandproperties.)Ifuandxareuncorrelated,then,asran-domvariables,theyarenotlinearlyrelated.Assumingthatuandxareuncorrelatedgoesalongwaytowarddefiningthesenseinwhichuandxshouldbeunrelatedinequation(2.1).Butitdoesnotgofarenough,becausecorrelationmeasuresonlylineardepen-dencebetweenuandx.Correlationhasasomewhatcounterintuitivefeature:itispossi-bleforutobeuncorrelatedwithxwhilebeingcorrelatedwithfunctionsofx,suchas2x.(SeeSectionB.4forfurtherdiscussion.)Thispossibilityisnotacceptableformostregressionpurposes,asitcausesproblemsforinterpretatingthemodelandforderivingstatisticalproperties.Abetterassumptioninvolvestheexpectedvalueofugivenx.Becauseuandxarerandomvariables,wecandefinetheconditionaldistributionofugivenanyvalueofx.Inparticular,foranyx,wecanobtaintheexpected(oraverage)valueofuforthatsliceofthepopulationdescribedbythevalueofx.Thecrucialassumptionisthattheaveragevalueofudoesnotdependonthevalueofx.WecanwritethisasE(ux)E(u)0,(2.6)wherethesecondequalityfollowsfrom(2.5).Thefirstequalityinequation(2.6)isthenewassumption,calledthezeroconditionalmeanassumption.Itsaysthat,foranygivenvalueofx,theaverageoftheunobservablesisthesameandthereforemustequaltheaveragevalueofuintheentirepopulation.Letusseewhat(2.6)entailsinthewageexample.Tosimplifythediscussion,assumethatuisthesameasinnateability.Then(2.6)requiresthattheaveragelevelofabilityisthesameregardlessofyearsofeducation.Forexample,ifE(abil8)denotestheaverageabilityforthegroupofallpeoplewitheightyearsofeducation,andE(abil16)denotestheaverageabilityamongpeopleinthepopulationwith16yearsofeducation,then(2.6)impliesthatthesemustbethesame.Infact,theaverageabilitylevelmustbethesameforalleducationlevels.If,forexample,wethinkthataverageabilityincreaseswithyearsofeducation,then(2.6)isfalse.(Thiswouldhappenif,onaverage,peoplewithmoreabilitychoosetobecomemoreeducated.)Aswecannotobserveinnateability,wehavenowayofknowingwhetherornotaverageabilityisthe25\nd7/14/994:30PMPage26Part1RegressionAnalysiswithCross-SectionalDatasameforalleducationlevels.Butthisisanissuethatwemustaddressbeforeapplyingsimpleregressionanalysis.Inthefertilizerexample,iffertilizeramountsarechosenindependentlyofotherfea-turesoftheplots,then(2.6)willhold:theaveragelandqualitywillnotdependontheQUESTION2.1amountoffertilizer.However,ifmorefer-Supposethatascoreonafinalexam,score,dependsonclassestilizerisputonthehigherqualityplotsofattended(attend)andunobservedfactorsthataffectexamperfor-land,thentheexpectedvalueofuchangesmance(suchasstudentability):withtheleveloffertilizer,and(2.6)fails.Assumption(2.6)gives1anotherscore01attendu(2.7)interpretationthatisoftenuseful.Takingtheexpectedvalueof(2.1)conditionalonWhenwouldyouexpectthismodeltosatisfy(2.6)?xandusingE(ux)0givesE(yx)01x(2.8)Equation(2.8)showsthatthepopulationregressionfunction(PRF),E(yx),isalin-earfunctionofx.Thelinearitymeansthataone-unitincreaseinxchangestheexpect-Figure2.1E(yx)asalinearfunctionofx.yE(yx)01xx1x2x326\nd7/14/994:30PMPage27Chapter2TheSimpleRegressionModeledvalueofybytheamount1.Foranygivenvalueofx,thedistributionofyiscen-teredaboutE(yx),asillustratedinFigure2.1.When(2.6)istrue,itisusefultobreakyintotwocomponents.Thepiece01xissometimescalledthesystematicpartofy—thatis,thepartofyexplainedbyx—anduiscalledtheunsystematicpart,orthepartofynotexplainedbyx.Wewilluseassumption(2.6)inthenextsectionformotivatingestimatesof0and1.Thisassump-tionisalsocrucialforthestatisticalanalysisinSection2.5.2.2DERIVINGTHEORDINARYLEASTSQUARESESTIMATESNowthatwehavediscussedthebasicingredientsofthesimpleregressionmodel,wewilladdresstheimportantissueofhowtoestimatetheparameters0and1inequa-tion(2.1).Todothis,weneedasamplefromthepopulation.Let{(xi,yi):i1,…,n}denotearandomsampleofsizenfromthepopulation.Sincethesedatacomefrom(2.1),wecanwriteyi01xiui(2.9)foreachi.Here,uiistheerrortermforobservationisinceitcontainsallfactorsaffect-ingyiotherthanxi.Asanexample,ximightbetheannualincomeandyitheannualsavingsforfamilyiduringaparticularyear.Ifwehavecollecteddataon15families,thenn15.Ascat-terplotofsuchadatasetisgiveninFigure2.2,alongwiththe(necessarilyfictitious)populationregressionfunction.Wemustdecidehowtousethesedatatoobtainestimatesoftheinterceptandslopeinthepopulationregressionofsavingsonincome.Thereareseveralwaystomotivatethefollowingestimationprocedure.Wewilluse(2.5)andanimportantimplicationofassumption(2.6):inthepopulation,uhasazeromeanandisuncorrelatedwithx.Therefore,weseethatuhaszeroexpectedvalueandthatthecovariancebetweenxanduiszero:E(u)0(2.10)Cov(x,u)E(xu)0,(2.11)wherethefirstequalityin(2.11)followsfrom(2.10).(SeeSectionB.4forthedefini-tionandpropertiesofcovariance.)Intermsoftheobservablevariablesxandyandtheunknownparameters0and1,equations(2.10)and(2.11)canbewrittenasE(y01x)0(2.12)andE[x(y01x)]0,(2.13)respectively.Equations(2.12)and(2.13)implytworestrictionsonthejointprobabilitydistributionof(x,y)inthepopulation.Sincetherearetwounknownparameterstoesti-mate,wemighthopethatequations(2.12)and(2.13)canbeusedtoobtaingoodesti-27\nd7/14/994:30PMPage28Part1RegressionAnalysiswithCross-SectionalDataFigure2.2Scatterplotofsavingsandincomefor15families,andthepopulationregressionE(savingsincome)01income.savingsE(savingsincome)income010income0matorsof0and1.Infact,theycanbe.Givenasampleofdata,wechooseestimatesˆ0andˆ1tosolvethesamplecounterpartsof(2.12)and(2.13):n1n(yiˆ0ˆ1xi)0.(2.14)i1n1nxi(yiˆ0ˆ1xi)0.(2.15)i1Thisisanexampleofthemethodofmomentsapproachtoestimation.(SeeSectionC.4foradiscussionofdifferentestimationapproaches.)Theseequationscanbesolvedforˆ0andˆ1.UsingthebasicpropertiesofthesummationoperatorfromAppendixA,equation(2.14)canberewrittenasy¯ˆ0ˆ1x¯,(2.16)n1wherey¯nyiisthesampleaverageoftheyiandlikewiseforx¯.Thisequationallowsi1ustowriteˆ0intermsofˆ1,y¯,andx¯:28\nd7/14/994:30PMPage29Chapter2TheSimpleRegressionModelˆ0y¯ˆ1x¯.(2.17)Therefore,oncewehavetheslopeestimateˆ1,itisstraightforwardtoobtaintheinter-ceptestimateˆ0,giveny¯andx¯.1Droppingthenin(2.15)(sinceitdoesnotaffectthesolution)andplugging(2.17)into(2.15)yieldsnxi(yi(y¯ˆ1x¯)ˆ1xi)0i1which,uponrearrangement,givesnnxi(yiy¯)ˆ1xi(xix¯).i1i1Frombasicpropertiesofthesummationoperator[see(A.7)and(A.8)],nnnn2xi(xix¯)(xix¯)andxi(yiy¯)(xix¯)(yiy¯).i1i1i1i1Therefore,providedthatn2(xix¯)0,(2.18)i1theestimatedslopeisn(xix¯)(yiy¯)i1ˆ1n.(2.19)2(xix¯)i1Equation(2.19)issimplythesamplecovariancebetweenxandydividedbythesam-plevarianceofx.(SeeAppendixC.Dividingboththenumeratorandthedenominatorbyn1changesnothing.)Thismakessensebecause1equalsthepopulationcovari-ancedividedbythevarianceofxwhenE(u)0andCov(x,u)0.Animmediateimplicationisthatifxandyarepositivelycorrelatedinthesample,thenˆ1ispositive;ifxandyarenegativelycorrelated,thenˆ1isnegative.Althoughthemethodforobtaining(2.17)and(2.19)ismotivatedby(2.6),theonlyassumptionneededtocomputetheestimatesforaparticularsampleis(2.18).Thisishardlyanassumptionatall:(2.18)istrueprovidedthexiinthesamplearenotallequaltothesamevalue.If(2.18)fails,thenwehaveeitherbeenunluckyinobtainingoursamplefromthepopulationorwehavenotspecifiedaninterestingproblem(xdoesnotvaryinthepopulation.).Forexample,ifywageandxeduc,then(2.18)failsonlyifeveryoneinthesamplehasthesameamountofeducation.(Forexample,ifeveryoneisahighschoolgraduate.SeeFigure2.3.)Ifjustonepersonhasadifferentamountofeducation,then(2.18)holds,andtheOLSestimatescanbecomputed.29\nd7/14/994:30PMPage30Part1RegressionAnalysiswithCross-SectionalDataFigure2.3Ascatterplotofwageagainsteducationwheneduci12foralli.wage012educTheestimatesgivenin(2.17)and(2.19)arecalledtheordinaryleastsquares(OLS)estimatesof0and1.Tojustifythisname,foranyˆ0andˆ1,defineafittedvalueforywhenxxisuchasyˆiˆ0ˆ1xi,(2.20)forthegiveninterceptandslope.Thisisthevaluewepredictforywhenxxi.Thereisafittedvalueforeachobservationinthesample.Theresidualforobservationiisthedifferencebetweentheactualyianditsfittedvalue:uˆiyiyˆiyiˆ0ˆ1xi.(2.21)Again,therearensuchresiduals.(Thesearenotthesameastheerrorsin(2.9),apointwereturntoinSection2.5.)ThefittedvaluesandresidualsareindicatedinFigure2.4.Now,supposewechooseˆ0andˆ1tomakethesumofsquaredresiduals,nn22uˆi(yiˆ0ˆ1xi),(2.22)i1i130\nd7/14/994:30PMPage31Chapter2TheSimpleRegressionModelFigure2.4Fittedvaluesandresiduals.yyiyˆˆˆxûresidual01iyˆFittedvalueyi1x1xixassmallaspossible.Theappendixtothischaptershowsthattheconditionsnecessaryfor(ˆ0,ˆ1)tominimize(2.22)aregivenexactlybyequations(2.14)and(2.15),without1n.Equations(2.14)and(2.15)areoftencalledthefirstorderconditionsfortheOLSestimates,atermthatcomesfromoptimizationusingcalculus(seeAppendixA).Fromourpreviouscalculations,weknowthatthesolutionstotheOLSfirstorderconditionsaregivenby(2.17)and(2.19).Thename“ordinaryleastsquares”comesfromthefactthattheseestimatesminimizethesumofsquaredresiduals.OncewehavedeterminedtheOLSinterceptandslopeestimates,weformtheOLSregressionline:yˆˆ0ˆ1x,(2.23)whereitisunderstoodthatˆ0andˆ1havebeenobtainedusingequations(2.17)and(2.19).Thenotationyˆ,readas“yhat,”emphasizesthatthepredictedvaluesfromequa-tion(2.23)areestimates.Theintercept,ˆ0,isthepredictedvalueofywhenx0,althoughinsomecasesitwillnotmakesensetosetx0.Inthosesituations,ˆ0isnot,initself,veryinteresting.Whenusing(2.23)tocomputepredictedvaluesofyforvari-ousvaluesofx,wemustaccountfortheinterceptinthecalculations.Equation(2.23)isalsocalledthesampleregressionfunction(SRF)becauseitistheestimatedversionofthepopulationregressionfunctionE(yx)01x.ItisimportanttorememberthatthePRFissomethingfixed,butunknown,inthepopulation.SincetheSRFis31\nd7/14/994:30PMPage32Part1RegressionAnalysiswithCross-SectionalDataobtainedforagivensampleofdata,anewsamplewillgenerateadifferentslopeandinterceptinequation(2.23).Inmostcasestheslopeestimate,whichwecanwriteasˆ1yˆ/x,(2.24)isofprimaryinterest.Ittellsustheamountbywhichyˆchangeswhenxincreasesbyoneunit.Equivalently,yˆˆ1x,(2.25)sothatgivenanychangeinx(whetherpositiveornegative),wecancomputethepre-dictedchangeiny.Wenowpresentseveralexamplesofsimpleregressionobtainedbyusingrealdata.Inotherwords,wefindtheinterceptandslopeestimateswithequations(2.17)and(2.19).Sincetheseexamplesinvolvemanyobservations,thecalculationsweredoneusinganeconometricsoftwarepackage.Atthispoint,youshouldbecarefulnottoreadtoomuchintotheseregressions;theyarenotnecessarilyuncoveringacausalrelation-ship.WehavesaidnothingsofaraboutthestatisticalpropertiesofOLS.InSection2.5,weconsiderstatisticalpropertiesafterweexplicitlyimposeassumptionsonthepopu-lationmodelequation(2.1).EXAMPLE2.3(CEOSalaryandReturnonEquity)Forthepopulationofchiefexecutiveofficers,letybeannualsalary(salary)inthousandsofdollars.Thus,y856.3indicatesanannualsalaryof$856,300,andy1452.6indicatesasalaryof$1,452,600.Letxbetheaveragereturnequity(roe)fortheCEO’sfirmforthepreviousthreeyears.(Returnonequityisdefinedintermsofnetincomeasapercentageofcommonequity.)Forexample,ifroe10,thenaveragereturnonequityis10percent.TostudytherelationshipbetweenthismeasureoffirmperformanceandCEOcom-pensation,wepostulatethesimplemodelsalary01roeu.Theslopeparameter1measuresthechangeinannualsalary,inthousandsofdollars,whenreturnonequityincreasesbyonepercentagepoint.Becauseahigherroeisgoodforthecompany,wethink10.ThedatasetCEOSAL1.RAWcontainsinformationon209CEOsfortheyear1990;thesedatawereobtainedfromBusinessWeek(5/6/91).Inthissample,theaverageannualsalaryis$1,281,120,withthesmallestandlargestbeing$223,000and$14,822,000,respective-ly.Theaveragereturnonequityfortheyears1988,1989,and1990is17.18percent,withthesmallestandlargestvaluesbeing0.5and56.3percent,respectively.UsingthedatainCEOSAL1.RAW,theOLSregressionlinerelatingsalarytoroeissalˆary963.19118.501roe,(2.26)32\nd7/14/994:30PMPage33Chapter2TheSimpleRegressionModelwheretheinterceptandslopeestimateshavebeenroundedtothreedecimalplaces;weuse“salaryhat”toindicatethatthisisanestimatedequation.Howdoweinterprettheequation?First,ifthereturnonequityiszero,roe0,thenthepredictedsalaryistheinter-cept,963.191,whichequals$963,191sincesalaryismeasuredinthousands.Next,wecanwritethepredictedchangeinsalaryasafunctionofthechangeinroe:salˆary18.501(roe).Thismeansthatifthereturnonequityincreasesbyonepercentagepoint,roe1,thensalaryispredictedtochangebyabout18.5,or$18,500.Because(2.26)isalinearequation,thisistheestimatedchangeregardlessoftheinitialsalary.Wecaneasilyuse(2.26)tocomparepredictedsalariesatdifferentvaluesofroe.Supposeroe30.Thensalˆary963.19118.501(30)1518.221,whichisjustover$1.5million.However,thisdoesnotmeanthataparticularCEOwhosefirmhadanroe30earns$1,518,221.Therearemanyotherfactorsthataffectsalary.ThisisjustourpredictionfromtheOLSregressionline(2.26).TheestimatedlineisgraphedinFig-ure2.5,alongwiththepopulationregressionfunctionE(salaryroe).WewillneverknowthePRF,sowecannottellhowclosetheSRFistothePRF.Anothersampleofdatawillgiveadifferentregressionline,whichmayormaynotbeclosertothepopulationregres-sionline.Figure2.5TheOLSregressionlinesalaryˆ963.19118.50roeandthe(unknown)populationregressionfunction.salarysalaryˆ963.19118.501roeE(salaryroe)01roe963.191roe33\nd7/14/994:30PMPage34Part1RegressionAnalysiswithCross-SectionalDataEXAMPLE2.4(WageandEducation)Forthepopulationofpeopleintheworkforcein1976,letywage,wherewageismea-suredindollarsperhour.Thus,foraparticularperson,ifwage6.75,thehourlywageis$6.75.Letxeducdenoteyearsofschooling;forexample,educ12correspondstoacompletehighschooleducation.Sincetheaveragewageinthesampleis$5.90,thecon-sumerpriceindexindicatesthatthisamountisequivalentto$16.64in1997dollars.UsingthedatainWAGE1.RAWwheren526individuals,weobtainthefollowingOLSregressionline(orsampleregressionfunction):waˆge0.900.54educ.(2.27)Wemustinterpretthisequationwithcaution.Theinterceptof0.90literallymeansthatapersonwithnoeducationhasapredictedhourlywageof90centsanhour.This,ofcourse,issilly.Itturnsoutthatnooneinthesamplehaslessthaneightyearsofeducation,whichhelpstoexplainthecrazypredictionforazeroeducationvalue.Forapersonwitheightyearsofeducation,thepredictedwageiswaˆge0.900.54(8)3.42,orQUESTION2.2$3.42perhour(in1976dollars).Theestimatedwagefrom(2.27),wheneduc8,is$3.42in1976Theslopeestimatein(2.27)impliesthatdollars.Whatisthisvaluein1997dollars?(Hint:YouhaveenoughonemoreyearofeducationincreaseshourlyinformationinExample2.4toanswerthisquestion.)wageby54centsanhour.Therefore,fourmoreyearsofeducationincreasethepre-dictedwageby4(0.54)2.16or$2.16perhour.Thesearefairlylargeeffects.Becauseofthelinearnatureof(2.27),anotheryearofeducationincreasesthewagebythesameamount,regardlessoftheinitiallevelofeducation.InSection2.4,wediscusssomemeth-odsthatallowfornonconstantmarginaleffectsofourexplanatoryvariables.EXAMPLE2.5(VotingOutcomesandCampaignExpenditures)ThefileVOTE1.RAWcontainsdataonelectionoutcomesandcampaignexpendituresfor173two-partyracesfortheU.S.HouseofRepresentativesin1988.Therearetwocandi-datesineachrace,AandB.LetvoteAbethepercentageofthevotereceivedbyCandidateAandshareAbethethepercentageoftotalcampaignexpendituresaccountedforbyCandidateA.ManyfactorsotherthanshareAaffecttheelectionoutcome(includingthequalityofthecandidatesandpossiblythedollaramountsspentbyAandB).Nevertheless,wecanestimateasimpleregressionmodeltofindoutwhetherspendingmorerelativetoone’schallengerimpliesahigherpercentageofthevote.Theestimatedequationusingthe173observationsisvotˆeA40.900.306shareA.(2.28)Thismeansthat,iftheshareofCandidateA’sexpendituresincreasesbyonepercent-agepoint,CandidateAreceivesalmostone-thirdofapercentagepointmoreofthe34\nd7/14/994:30PMPage35Chapter2TheSimpleRegressionModeltotalvote.Whetherornotthisisacausaleffectisunclear,buttheresultiswhatwemightexpect.Insomecases,regressionanalysisisnotusedtodeterminecausalitybuttosimplylookatwhethertwovariablesarepositivelyornegativelyrelated,muchlikeastandardcorrelationanalysis.AnexampleofthisoccursinProblem2.12,whereyouareQUESTION2.3askedtousedatafromBiddleandInExample2.5,whatisthepredictedvoteforCandidateAifshareAHamermesh(1990)ontimespentsleeping60(whichmeans60percent)?Doesthisanswerseemreasonable?andworkingtoinvestigatethetradeoffbetweenthesetwofactors.ANoteonTerminolgyInmostcases,wewillindicatetheestimationofarelationshipthroughOLSbywritinganequationsuchas(2.26),(2.27),or(2.28).Sometimes,forthesakeofbrevity,itisusefultoindicatethatanOLSregressionhasbeenrunwithoutactuallywritingouttheequation.Wewilloftenindicatethatequation(2.23)hasbeenobtainedbyOLSinsay-ingthatweruntheregressionofyonx,(2.29)orsimplythatweregressyonx.Thepositionsofyandxin(2.29)indicatewhichisthedependentvariableandwhichistheindependentvariable:wealwaysregressthedepen-dentvariableontheindependentvariable.Forspecificapplications,wereplaceyandxwiththeirnames.Thus,toobtain(2.26),weregresssalaryonroeortoobtain(2.28),weregressvoteAonshareA.Whenweusesuchterminologyin(2.29),wewillalwaysmeanthatweplantoesti-matetheintercept,ˆ0,alongwiththeslope,ˆ1.Thiscaseisappropriateforthevastmajorityofapplications.Occasionally,wemaywanttoestimatetherelationshipbetweenyandxassumingthattheinterceptiszero(sothatx0impliesthatyˆ0);wecoverthiscasebrieflyinSection2.6.Unlessexplicitlystatedotherwise,wealwaysestimateaninterceptalongwithaslope.2.3MECHANICSOFOLSInthissection,wecoversomealgebraicpropertiesofthefittedOLSregressionline.PerhapsthebestwaytothinkaboutthesepropertiesistorealizethattheyarefeaturesofOLSforaparticularsampleofdata.Theycanbecontrastedwiththestatisticalprop-ertiesofOLS,whichrequiresderivingfeaturesofthesamplingdistributionsoftheesti-mators.WewilldiscussstatisticalpropertiesinSection2.5.Severalofthealgebraicpropertieswearegoingtoderivewillappearmundane.Nevertheless,havingagraspofthesepropertieshelpsustofigureoutwhathappenstotheOLSestimatesandrelatedstatisticswhenthedataaremanipulatedincertainways,suchaswhenthemeasurementunitsofthedependentandindependentvariableschange.35\nd7/14/994:30PMPage36Part1RegressionAnalysiswithCross-SectionalDataFittedValuesandResidualsWeassumethattheinterceptandslopeestimates,ˆ0andˆ1,havebeenobtainedforthegivensampleofdata.Givenˆ0andˆ1,wecanobtainthefittedvalueyˆiforeachobser-vation.[Thisisgivenbyequation(2.20).]Bydefinition,eachfittedvalueofyˆiisontheOLSregressionline.TheOLSresidualassociatedwithobservationi,uˆi,isthediffer-encebetweenyianditsfittedvalue,asgiveninequation(2.21).Ifuˆiispositive,thelineunderpredictsyi;ifuˆiisnegative,thelineoverpredictsyi.Theidealcaseforobservationiiswhenuˆi0,butinmostcaseseveryresidualisnotequaltozero.Inotherwords,noneofthedatapointsmustactuallylieontheOLSline.EXAMPLE2.6(CEOSalaryandReturnonEquity)Table2.2containsalistingofthefirst15observationsintheCEOdataset,alongwiththefittedvalues,calledsalaryhat,andtheresiduals,calleduhat.Table2.2FittedValuesandResidualsfortheFirst15CEOsobsnoroesalarysalaryhatuhat114.110951224.058129.0581210.910011164.854163.8542323.511221397.969275.969245.95781072.348494.3484513.813681218.508149.4923620.011451333.215188.2151716.410781266.611188.6108816.310941264.761170.7606910.512371157.45479.546261026.38331449.773616.77261125.95671442.372875.37211226.89331459.023526.0231continued36\nd7/14/994:30PMPage37Chapter2TheSimpleRegressionModelTable2.2(concluded)obsnoroesalarysalaryhatuhat1314.813391237.009101.99111422.39371375.768438.76781556.320112004.808006.191895ThefirstfourCEOshavelowersalariesthanwhatwepredictedfromtheOLSregressionline(2.26);inotherwords,givenonlythefirm’sroe,theseCEOsmakelessthanwhatwepre-dicted.Ascanbeseenfromthepositiveuhat,thefifthCEOmakesmorethanpredictedfromtheOLSregressionline.AlgebraicPropertiesofOLSStatisticsThereareseveralusefulalgebraicpropertiesofOLSestimatesandtheirassociatedsta-tistics.Wenowcoverthethreemostimportantofthese.(1)Thesum,andthereforethesampleaverageoftheOLSresiduals,iszero.Mathematically,nuˆi0.(2.30)i1Thispropertyneedsnoproof;itfollowsimmediatelyfromtheOLSfirstordercondi-tion(2.14),whenwerememberthattheresidualsaredefinedbyuˆiyiˆ0ˆ1xi.Inotherwords,theOLSestimatesˆ0andˆ1arechosentomaketheresidualsadduptozero(foranydataset).Thissaysnothingabouttheresidualforanyparticularobserva-tioni.(2)ThesamplecovariancebetweentheregressorsandtheOLSresidualsiszero.Thisfollowsfromthefirstordercondition(2.15),whichcanbewrittenintermsoftheresidualsasnxiuˆi0.(2.31)i1ThesampleaverageoftheOLSresidualsiszero,sothelefthandsideof(2.31)ispro-portionaltothesamplecovariancebetweenxianduˆi.(3)Thepoint(x¯,y¯)isalwaysontheOLSregressionline.Inotherwords,ifwetakeequation(2.23)andpluginx¯forx,thenthepredictedvalueisy¯.Thisisexactlywhatequation(2.16)showsus.37\nd7/14/994:30PMPage38Part1RegressionAnalysiswithCross-SectionalDataEXAMPLE2.7(WageandEducation)ForthedatainWAGE1.RAW,theaveragehourlywageinthesampleis5.90,roundedtotwodecimalplaces,andtheaverageeducationis12.56.Ifweplugeduc12.56intotheOLSregressionline(2.27),wegetwaˆge0.900.54(12.56)5.8824,whichequals5.9whenroundedtothefirstdecimalplace.Thereasonthesefiguresdonotexactlyagreeisthatwehaveroundedtheaveragewageandeducation,aswellastheinterceptandslopeestimates.Ifwedidnotinitiallyroundanyofthevalues,wewouldgettheanswerstoagreemoreclosely,butthispracticehaslittleusefuleffect.Writingeachyiasitsfittedvalue,plusitsresidual,providesanotherwaytointepretanOLSregression.Foreachi,writeyiyˆiuˆi.(2.32)Fromproperty(1)above,theaverageoftheresidualsiszero;equivalently,thesampleaverageofthefittedvalues,yˆi,isthesameasthesampleaverageoftheyi,oryˆ¯y¯.Further,properties(1)and(2)canbeusedtoshowthatthesamplecovariancebetweenyˆianduˆiiszero.Thus,wecanviewOLSasdecomposingeachyiintotwoparts,afittedvalueandaresidual.Thefittedvaluesandresidualsareuncorrelatedinthesample.Definethetotalsumofsquares(SST),theexplainedsumofsquares(SSE),andtheresidualsumofsquares(SSR)(alsoknownasthesumofsquaredresiduals),asfollows:n2SST(yiy¯).(2.33)i1n2SSE(yˆiy¯).(2.34)i1n2SSRuˆi.(2.35)i1SSTisameasureofthetotalsamplevariationintheyi;thatis,itmeasureshowspreadouttheyiareinthesample.IfwedivideSSTbyn1,weobtainthesamplevarianceofy,asdiscussedinAppendixC.Similarly,SSEmeasuresthesamplevariationintheyˆi(whereweusethefactthatyˆ¯y¯),andSSRmeasuresthesamplevariationintheuˆi.ThetotalvariationinycanalwaysbeexpressedasthesumoftheexplainedvariationandtheunexplainedvariationSSR.Thus,SSTSSESSR.(2.36)38\nd7/14/994:30PMPage39Chapter2TheSimpleRegressionModelProving(2.36)isnotdifficult,butitrequiresustouseallofthepropertiesofthesum-mationoperatorcoveredinAppendixA.Writenn22(yiy¯)[(yiyˆi)(yˆiy¯)]i1i1n2[uˆi(yˆiy¯)]i1nnn22uˆi2uˆi(yˆiy¯)(yˆiy¯)i1i1i1nSSR2uˆi(yˆiy¯)SSE.i1Now(2.36)holdsifweshowthatnuˆi(yˆiy¯)0.(2.37)i1Butwehavealreadyclaimedthatthesamplecovariancebetweentheresidualsandthefittedvaluesiszero,andthiscovarianceisjust(2.37)dividedbyn1.Thus,wehaveestablished(2.36).SomewordsofcautionaboutSST,SSE,andSSRareinorder.Thereisnouniformagreementonthenamesorabbreviationsforthethreequantitiesdefinedinequations(2.33),(2.34),and(2.35).ThetotalsumofsquaresiscalledeitherSSTorTSS,sothereislittleconfusionhere.Unfortunately,theexplainedsumofsquaresissometimescalledthe“regressionsumofsquares.”Ifthistermisgivenitsnaturalabbreviation,itcaneas-ilybeconfusedwiththetermresidualsumofsquares.Someregressionpackagesrefertotheexplainedsumofsquaresasthe“modelsumofsquares.”Tomakemattersevenworse,theresidualsumofsquaresisoftencalledthe“errorsumofsquares.”Thisisespeciallyunfortunatebecause,aswewillseeinSection2.5,theerrorsandtheresidualsaredifferentquantities.Thus,wewillalwayscall(2.35)theresidualsumofsquaresorthesumofsquaredresiduals.Weprefertousetheabbrevia-tionSSRtodenotethesumofsquaredresiduals,becauseitismorecommoninecono-metricpackages.Goodness-of-FitSofar,wehavenowayofmeasuringhowwelltheexplanatoryorindependentvariable,x,explainsthedependentvariable,y.Itisoftenusefultocomputeanumberthatsum-marizeshowwelltheOLSregressionlinefitsthedata.Inthefollowingdiscussion,besuretorememberthatweassumethataninterceptisestimatedalongwiththeslope.Assumingthatthetotalsumofsquares,SST,isnotequaltozero—whichistrueexceptintheveryunlikelyeventthatalltheyiequalthesamevalue—wecandivide(2.36)bySSTtoget1SSE/SSTSSR/SST.TheR-squaredoftheregression,sometimescalledthecoefficientofdetermination,isdefinedas39\nd7/14/994:30PMPage40Part1RegressionAnalysiswithCross-SectionalData2RSSE/SST1SSR/SST.(2.38)2Ristheratiooftheexplainedvariationcomparedtothetotalvariation,andthusitisinterpretedasthefractionofthesamplevariationinythatisexplainedbyx.Thesec-2ondequalityin(2.38)providesanotherwayforcomputingR.2From(2.36),thevalueofRisalwaysbetweenzeroandone,sinceSSEcanbeno2greaterthanSST.WheninterpretingR,weusuallymultiplyitby100tochangeitinto2apercent:100Risthepercentageofthesamplevariationinythatisexplainedbyx.Ifthedatapointsalllieonthesameline,OLSprovidesaperfectfittothedata.In22thiscase,R1.AvalueofRthatisnearlyequaltozeroindicatesapoorfitoftheOLSline:verylittleofthevariationintheyiiscapturedbythevariationintheyˆi(which2alllieontheOLSregressionline).Infact,itcanbeshownthatRisequaltothesquareofthesamplecorrelationcoefficientbetweenyiandyˆi.Thisiswheretheterm“R-squared”camefrom.(TheletterRwastraditionallyusedtodenoteanestimateofapopulationcorrelationcoefficient,anditsusagehassurvivedinregressionanalysis.)EXAMPLE2.8(CEOSalaryandReturnonEquity)IntheCEOsalaryregression,weobtainthefollowing:salˆary963.19118.501roe(2.39)2n209,R0.0132WehavereproducedtheOLSregressionlineandthenumberofobservationsforclarity.UsingtheR-squared(roundedtofourdecimalplaces)reportedforthisequation,wecanseehowmuchofthevariationinsalaryisactuallyexplainedbythereturnonequity.Theansweris:notmuch.Thefirm’sreturnonequityexplainsonlyabout1.3%ofthevariationinsalariesforthissampleof209CEOs.Thatmeansthat98.7%ofthesalaryvariationsfortheseCEOsisleftunexplained!ThislackofexplanatorypowermaynotbetoosurprisingsincetherearemanyothercharacteristicsofboththefirmandtheindividualCEOthatshouldinfluencesalary;thesefactorsarenecessarilyincludedintheerrorsinasimpleregressionanalysis.Inthesocialsciences,lowR-squaredsinregressionequationsarenotuncommon,especiallyforcross-sectionalanalysis.Wewilldiscussthisissuemoregenerallyundermultipleregressionanalysis,butitisworthemphasizingnowthataseeminglylowR-squareddoesnotnecessarilymeanthatanOLSregressionequationisuseless.Itisstillpossiblethat(2.39)isagoodestimateoftheceterisparibusrelationshipbetweensalaryandroe;whetherornotthisistruedoesnotdependdirectlyonthesizeofR-squared.StudentswhoarefirstlearningeconometricstendtoputtoomuchweightonthesizeoftheR-squaredinevaluatingregressionequations.Fornow,beawarethatusingR-squaredasthemaingaugeofsuccessforaneconometricanalysiscanleadtotrouble.Sometimestheexplanatoryvariableexplainsasubstantialpartofthesamplevaria-tioninthedependentvariable.40\nd7/14/994:30PMPage41Chapter2TheSimpleRegressionModelEXAMPLE2.9(VotingOutcomesandCampaignExpenditures)2Inthevotingoutcomeequationin(2.28),R0.505.Thus,theshareofcampaignexpen-dituresexplainsjustover50percentofthevariationintheelectionoutcomesforthissam-ple.Thisisafairlysizableportion.2.4UNITSOFMEASUREMENTANDFUNCTIONALFORMTwoimportantissuesinappliedeconomicsare(1)understandinghowchangingtheunitsofmeasurementofthedependentand/orindependentvariablesaffectsOLSesti-matesand(2)knowinghowtoincorporatepopularfunctionalformsusedineconomicsintoregressionanalysis.Themathematicsneededforafullunderstandingoffunc-tionalformissuesisreviewedinAppendixA.TheEffectsofChangingUnitsofMeasurementonOLSStatisticsInExample2.3,wechosetomeasureannualsalaryinthousandsofdollars,andthereturnonequitywasmeasuredasapercent(ratherthanasadecimal).Itiscrucialtoknowhowsalaryandroearemeasuredinthisexampleinordertomakesenseoftheestimatesinequation(2.39).WemustalsoknowthatOLSestimateschangeinentirelyexpectedwayswhentheunitsofmeasurementofthedependentandindependentvariableschange.InExample2.3,supposethat,ratherthanmeasuringsalaryinthousandsofdollars,wemeasureitindollars.Letsalardolbesalaryindollars(salardol845,761wouldbeinterpretedas$845,761.).Ofcourse,salardolhasasimplerelationshiptothesalarymeasuredinthousandsofdollars:salardol1,000salary.Wedonotneedtoactuallyruntheregressionofsalardolonroetoknowthattheestimatedequationis:salaˆrdol963,19118,501roe.(2.40)Weobtaintheinterceptandslopein(2.40)simplybymultiplyingtheinterceptandtheslopein(2.39)by1,000.Thisgivesequations(2.39)and(2.40)thesameinterpretation.Lookingat(2.40),ifroe0,thensalaˆrdol963,191,sothepredictedsalaryis$963,191[thesamevalueweobtainedfromequation(2.39)].Furthermore,ifroeincreasesbyone,thenthepredictedsalaryincreasesby$18,501;again,thisiswhatweconcludedfromourearlieranalysisofequation(2.39).Generally,itiseasytofigureoutwhathappenstotheinterceptandslopeestimateswhenthedependentvariablechangesunitsofmeasurement.Ifthedependentvariableismultipliedbytheconstantc—whichmeanseachvalueinthesampleismultipliedbyc—thentheOLSinterceptandslopeestimatesarealsomultipliedbyc.(Thisassumesnothinghaschangedabouttheindependentvariable.)IntheCEOsalaryexample,c1,000inmovingfromsalarytosalardol.41\nd7/14/994:30PMPage42Part1RegressionAnalysiswithCross-SectionalDataWecanalsousetheCEOsalaryexampletoseewhathappenswhenwechangetheunitsofmeasurementoftheindepen-dentvariable.Defineroedecroe/100QUESTION2.4tobethedecimalequivalentofroe;thus,Supposethatsalaryismeasuredinhundredsofdollars,ratherthanroedec0.23meansareturnonequityofinthousandsofdollars,saysalarhun.WhatwillbetheOLSintercept23percent.Tofocusonchangingtheunitsandslopeestimatesintheregressionofsalarhunonroe?ofmeasurementoftheindependentvari-able,wereturntoouroriginaldependentvariable,salary,whichismeasuredinthousandsofdollars.Whenweregresssalaryonroedec,weobtainsalˆary963.1911850.1roedec.(2.41)Thecoefficientonroedecis100timesthecoefficientonroein(2.39).Thisisasitshouldbe.Changingroebyonepercentagepointisequivalenttoroedec0.01.From(2.41),ifroedec0.01,thensalˆary1850.1(0.01)18.501,whichiswhatisobtainedbyusing(2.39).Notethat,inmovingfrom(2.39)to(2.41),theindependentvariablewasdividedby100,andsotheOLSslopeestimatewasmultipliedby100,pre-servingtheinterpretationoftheequation.Generally,iftheindependentvariableisdividedormultipliedbysomenonzeroconstant,c,thentheOLSslopecoefficientisalsomultipliedordividedbycrespectively.Theintercepthasnotchangedin(2.41)becauseroedec0stillcorrespondstoazeroreturnonequity.Ingeneral,changingtheunitsofmeasurementofonlytheinde-pendentvariabledoesnotaffecttheintercept.Intheprevioussection,wedefinedR-squaredasagoodness-of-fitmeasurefor2OLSregression.WecanalsoaskwhathappenstoRwhentheunitofmeasurementofeithertheindependentorthedependentvariablechanges.Withoutdoinganyalge-bra,weshouldknowtheresult:thegoodness-of-fitofthemodelshouldnotdependontheunitsofmeasurementofourvariables.Forexample,theamountofvariationinsalary,explainedbythereturnonequity,shouldnotdependonwhethersalaryismea-suredindollarsorinthousandsofdollarsoronwhetherreturnonequityisapercent2oradecimal.Thisintuitioncanbeverifiedmathematically:usingthedefinitionofR,2itcanbeshownthatRis,infact,invarianttochangesintheunitsofyorx.IncorporatingNonlinearitiesinSimpleRegressionSofarwehavefocusedonlinearrelationshipsbetweenthedependentandindependentvariables.AswementionedinChapter1,linearrelationshipsarenotnearlygeneralenoughforalleconomicapplications.Fortunately,itisrathereasytoincorporatemanynonlinearitiesintosimpleregressionanalysisbyappropriatelydefiningthedependentandindependentvariables.Herewewillcovertwopossibilitiesthatoftenappearinappliedwork.Inreadingappliedworkinthesocialsciences,youwilloftenencounterregressionequationswherethedependentvariableappearsinlogarithmicform.Whyisthisdone?Recallthewage-educationexample,whereweregressedhourlywageonyearsofedu-cation.Weobtainedaslopeestimateof0.54[seeequation(2.27)],whichmeansthateachadditionalyearofeducationispredictedtoincreasehourlywageby54cents.42\nd7/14/994:30PMPage43Chapter2TheSimpleRegressionModelBecauseofthelinearnatureof(2.27),54centsistheincreaseforeitherthefirstyearofeducationorthetwentiethyear;thismaynotbereasonable.Suppose,instead,thatthepercentageincreaseinwageisthesamegivenonemoreyearofeducation.Model(2.27)doesnotimplyaconstantpercentageincrease:theper-centageincreasesdependsontheinitialwage.Amodelthatgives(approximately)aconstantpercentageeffectislog(wage)01educu,(2.42)wherelog()denotesthenaturallogarithm.(SeeAppendixAforareviewofloga-rithms.)Inparticular,ifu0,then%wage(1001)educ.(2.43)Noticehowwemultiply1by100togetthepercentagechangeinwagegivenoneaddi-tionalyearofeducation.Sincethepercentagechangeinwageisthesameforeachaddi-tionalyearofeducation,thechangeinwageforanextrayearofeducationincreasesaseducationincreases;inotherwords,(2.42)impliesanincreasingreturntoeducation.Byexponentiating(2.42),wecanwritewageexp(01educu).ThisequationisgraphedinFigure2.6,withu0.Figure2.6wageexp(01educ),with10.wage0educ43\nd7/14/994:30PMPage44Part1RegressionAnalysiswithCross-SectionalDataEstimatingamodelsuchas(2.42)isstraightforwardwhenusingsimpleregression.Justdefinethedependentvariable,y,tobeylog(wage).Theindependentvariableisrepresentedbyxeduc.ThemechanicsofOLSarethesameasbefore:theinterceptandslopeestimatesaregivenbytheformulas(2.17)and(2.19).Inotherwords,weobtainˆ0andˆ1fromtheOLSregressionoflog(wage)oneduc.EXAMPLE2.10(ALogWageEquation)UsingthesamedataasinExample2.4,butusinglog(wage)asthedependentvariable,weobtainthefollowingrelationship:log(ˆwage)0.5840.083educ(2.44)2n526,R0.186.Thecoefficientoneduchasapercentageinterpretationwhenitismultipliedby100:wageincreasesby8.3percentforeveryadditionalyearofeducation.Thisiswhateconomistsmeanwhentheyrefertothe“returntoanotheryearofeducation.”Itisimportanttorememberthatthemainreasonforusingthelogofwagein(2.42)istoimposeaconstantpercentageeffectofeducationonwage.Onceequation(2.42)isobtained,thenaturallogofwageisrarelymentioned.Inparticular,itisnotcorrecttosaythatanotheryearofeducationincreaseslog(wage)by8.3%.Theinterceptin(2.42)isnotverymeaningful,asitgivesthepredictedlog(wage),wheneduc0.TheR-squaredshowsthateducexplainsabout18.6percentofthevari-ationinlog(wage)(notwage).Finally,equation(2.44)mightnotcaptureallofthenon-linearityintherelationshipbetweenwageandschooling.Ifthereare“diplomaeffects,”thenthetwelfthyearofeducation—graduationfromhighschool—couldbeworthmuchmorethantheeleventhyear.WewilllearnhowtoallowforthiskindofnonlinearityinChapter7.Anotherimportantuseofthenaturallogisinobtainingaconstantelasticitymodel.EXAMPLE2.11(CEOSalaryandFirmSales)WecanestimateaconstantelasticitymodelrelatingCEOsalarytofirmsales.ThedatasetisthesameoneusedinExample2.3,exceptwenowrelatesalarytosales.Letsalesbeannualfirmsales,measuredinmillionsofdollars.Aconstantelasticitymodelislog(salary)01log(sales)u,(2.45)where1istheelasticityofsalarywithrespecttosales.Thismodelfallsunderthesimpleregressionmodelbydefiningthedependentvariabletobeylog(salary)andtheinde-pendentvariabletobexlog(sales).EstimatingthisequationbyOLSgives44\nd7/14/994:30PMPage45Chapter2TheSimpleRegressionModellog(salaryˆ)4.8220.257log(sales)(2.46)2n209,R0.211.Thecoefficientoflog(sales)istheestimatedelasticityofsalarywithrespecttosales.Itimpliesthata1percentincreaseinfirmsalesincreasesCEOsalarybyabout0.257per-cent—theusualinterpretationofanelasticity.Thetwofunctionalformscoveredinthissectionwilloftenariseintheremainderofthistext.Wehavecoveredmodelscontainingnaturallogarithmsherebecausetheyappearsofrequentlyinappliedwork.Theinterpretationofsuchmodelswillnotbemuchdifferentinthemultipleregressioncase.Itisalsousefultonotewhathappenstotheinterceptandslopeestimatesifwechangetheunitsofmeasurementofthedependentvariablewhenitappearsinlogarithmicform.Becausethechangetologarithmicformapproximatesaproportionatechange,itmakessensethatnothinghappenstotheslope.Wecanseethisbywritingtherescaledvari-ableasc1yiforeachobservationi.Theoriginalequationislog(yi)01xiui.Ifweaddlog(c1)tobothsides,wegetlog(c1)log(yi)[log(c1)0]1xiui,orlog(c1yi)[log(c1)0]1xiui.(RememberthatthesumofthelogsisequaltothelogoftheirproductasshowninAppendixA.)Therefore,theslopeisstill1,buttheinterceptisnowlog(c1)0.Similarly,iftheindependentvariableislog(x),andwechangetheunitsofmeasurementofxbeforetakingthelog,thesloperemainsthesamebuttheinterceptdoesnotchange.YouwillbeaskedtoverifytheseclaimsinProblem2.9.Weendthissubsectionbysummarizingfourcombinationsoffunctionalformsavailablefromusingeithertheoriginalvariableoritsnaturallog.InTable2.3,xandystandforthevariablesintheiroriginalform.Themodelwithyasthedependentvari-ableandxastheindependentvariableiscalledthelevel-levelmodel,becauseeachvari-ableappearsinitslevelform.Themodelwithlog(y)asthedependentvariableandxastheindependentvariableiscalledthelog-levelmodel.Wewillnotexplicitlydiscussthelevel-logmodelhere,becauseitariseslessofteninpractice.Inanycase,wewillseeexamplesofthismodelinlaterchapters.Table2.3SummaryofFunctionalFormsInvolvingLogarithmsDependentIndependentInterpretationModelVariableVariableof1level-levelyxy1xlevel-logylog(x)y(1/100)%xlog-levellog(y)x%y(1001)xlog-loglog(y)log(x)%y1%x45\nd7/14/994:30PMPage46Part1RegressionAnalysiswithCross-SectionalDataThelastcolumninTable2.3givestheinterpretationof1.Inthelog-levelmodel,1001issometimescalledthesemi-elasticityofywithrespecttox.AswementionedinExample2.11,inthelog-logmodel,1istheelasticityofywithrespecttox.Table2.3warrantscarefulstudy,aswewillrefertoitoftenintheremainderofthetext.TheMeaningof“Linear”RegressionThesimpleregressionmodelthatwehavestudiedinthischapterisalsocalledthesim-plelinearregressionmodel.Yet,aswehavejustseen,thegeneralmodelalsoallowsforcertainnonlinearrelationships.Sowhatdoes“linear”meanhere?Youcanseebylook-ingatequation(2.1)thaty01xu.Thekeyisthatthisequationislinearintheparameters,0and1.Therearenorestrictionsonhowyandxrelatetotheoriginalexplainedandexplanatoryvariablesofinterest.AswesawinExamples2.7and2.8,yandxcanbenaturallogsofvariables,andthisisquitecommoninapplications.Butweneednotstopthere.Forexample,nothingpreventsusfromusingsimpleregressionto—estimateamodelsuchascons01incu,whereconsisannualconsumptionandincisannualincome.Whilethemechanicsofsimpleregressiondonotdependonhowyandxaredefined,theinterpretationofthecoefficientsdoesdependontheirdefinitions.Forsuc-cessfulempiricalwork,itismuchmoreimportanttobecomeproficientatinterpretingcoefficientsthantobecomeefficientatcomputingformulassuchas(2.19).WewillgetmuchmorepracticewithinterpretingtheestimatesinOLSregressionlineswhenwestudymultipleregression.Thereareplentyofmodelsthatcannotbecastasalinearregressionmodelbecausetheyarenotlinearintheirparameters;anexampleiscons1/(01inc)u.Estimationofsuchmodelstakesusintotherealmofthenonlinearregressionmodel,whichisbeyondthescopeofthistext.Formostapplications,choosingamodelthatcanbeputintothelinearregressionframeworkissufficient.2.5EXPECTEDVALUESANDVARIANCESOFTHEOLSESTIMATORSInSection2.1,wedefinedthepopulationmodely01xu,andweclaimedthatthekeyassumptionforsimpleregressionanalysistobeusefulisthattheexpectedvalueofugivenanyvalueofxiszero.InSections2.2,2.3,and2.4,wediscussedthealge-braicpropertiesofOLSestimation.WenowreturntothepopulationmodelandstudythestatisticalpropertiesofOLS.Inotherwords,wenowviewˆ0andˆ1asestimatorsfortheparameters0and1thatappearinthepopulationmodel.Thismeansthatwewillstudypropertiesofthedistributionsofˆ0andˆ1overdifferentrandomsamplesfromthepopulation.(AppendixCcontainsdefinitionsofestimatorsandreviewssomeoftheirimportantproperties.)UnbiasednessofOLSWebeginbyestablishingtheunbiasednessofOLSunderasimplesetofassumptions.Forfuturereference,itisusefultonumbertheseassumptionsusingtheprefix“SLR”forsimplelinearregression.Thefirstassumptiondefinesthepopulationmodel.46\nd7/14/994:30PMPage47Chapter2TheSimpleRegressionModelASSUMPTIONSLR.1(LINEARINPARAMETERS)Inthepopulationmodel,thedependentvariableyisrelatedtotheindependentvariablexandtheerror(ordisturbance)uasy01xu,(2.47)where0and1arethepopulationinterceptandslopeparameters,respectively.Toberealistic,y,x,anduareallviewedasrandomvariablesinstatingthepopulationmodel.WediscussedtheinterpretationofthismodelatsomelengthinSection2.1andgaveseveralexamples.Intheprevioussection,welearnedthatequation(2.47)isnotasrestrictiveasitinitiallyseems;bychoosingyandxappropriately,wecanobtaininter-estingnonlinearrelationships(suchasconstantelasticitymodels).Weareinterestedinusingdataonyandxtoestimatetheparameters0and,espe-cially,1.Weassumethatourdatawereobtainedasarandomsample.(SeeAppendixCforareviewofrandomsampling.)ASSUMPTIONSLR.2(RANDOMSAMPLING)Wecanusearandomsampleofsizen,{(xi,yi):i1,2,…,n},fromthepopulationmodel.Wewillhavetoaddressfailureoftherandomsamplingassumptioninlaterchaptersthatdealwithtimeseriesanalysisandsampleselectionproblems.Notallcross-sectionalsamplescanbeviewedasoutcomesofrandomsamples,butmanycanbe.Wecanwrite(2.47)intermsoftherandomsampleasyi01xiui,i1,2,…,n,(2.48)whereuiistheerrorordisturbanceforobservationi(forexample,personi,firmi,cityi,etc.).Thus,uicontainstheunobservablesforobservationiwhichaffectyi.Theuishouldnotbeconfusedwiththeresiduals,uˆi,thatwedefinedinSection2.3.Lateron,wewillexploretherelationshipbetweentheerrorsandtheresiduals.Forinterpret-ing0and1inaparticularapplication,(2.47)ismostinformative,but(2.48)isalsoneededforsomeofthestatisticalderivations.Therelationship(2.48)canbeplottedforaparticularoutcomeofdataasshowninFigure2.7.Inordertoobtainunbiasedestimatorsof0and1,weneedtoimposethezerocon-ditionalmeanassumptionthatwediscussedinsomedetailinSection2.1.Wenowexplicitlyaddittoourlistofassumptions.ASSUMPTIONSLR.3(ZEROCONDITIONALMEAN)E(ux)0.47\nd7/14/994:31PMPage48Part1RegressionAnalysiswithCross-SectionalDataFigure2.7Graphofyi01xiui.yyiuPRFiE(yx)x01u1y1x1xixForarandomsample,thisassumptionimpliesthatE(uixi)0,foralli1,2,…,n.Inadditiontorestrictingtherelationshipbetweenuandxinthepopulation,thezeroconditionalmeanassumption—coupledwiththerandomsamplingassumption—allowsforaconvenienttechnicalsimplification.Inparticular,wecanderivethestatis-ticalpropertiesoftheOLSestimatorsasconditionalonthevaluesofthexiinoursam-ple.Technically,instatisticalderivations,conditioningonthesamplevaluesoftheinde-pendentvariableisthesameastreatingthexiasfixedinrepeatedsamples.Thisprocessinvolvesseveralsteps.Wefirstchoosensamplevaluesforx1,x2,…,xn(Thesecanberepeated.).Giventhesevalues,wethenobtainasampleony(effectivelybyobtainingarandomsampleoftheui).Nextanothersampleofyisobtained,usingthesameval-uesforx1,…,xn.Thenanothersampleofyisobtained,againusingthesamexi.Andsoon.Thefixedinrepeatedsamplesscenarioisnotveryrealisticinnonexperimentalcon-texts.Forinstance,insamplingindividualsforthewage-educationexample,itmakeslittlesensetothinkofchoosingthevaluesofeducaheadoftimeandthensamplingindividualswiththoseparticularlevelsofeducation.Randomsampling,whereindivid-ualsarechosenrandomlyandtheirwageandeducationarebothrecorded,isrepresen-tativeofhowmostdatasetsareobtainedforempiricalanalysisinthesocialsciences.OnceweassumethatE(ux)0,andwehaverandomsampling,nothingislostinderivationsbytreatingthexiasnonrandom.Thedangeristhatthefixedinrepeatedsamplesassumptionalwaysimpliesthatuiandxiareindependent.Indecidingwhen48\nd7/14/994:31PMPage49Chapter2TheSimpleRegressionModelsimpleregressionanalysisisgoingtoproduceunbiasedestimators,itiscriticaltothinkintermsofAssumptionSLR.3.Oncewehaveagreedtoconditiononthexi,weneedonefinalassumptionforunbi-asedness.ASSUMPTIONSLR.4(SAMPLEVARIATIONINTHEINDEPENDENTVARIABLE)Inthesample,theindependentvariablesxi,i1,2,…,n,arenotallequaltothesamecon-stant.Thisrequiressomevariationinxinthepopulation.WeencounteredAssumptionSLR.4whenwederivedtheformulasfortheOLSesti-n2mators;itisequivalentto(xix¯)0.Ofthefourassumptionsmade,thisisthei1leastimportantbecauseitessentiallyneverfailsininterestingapplications.IfAssump-tionSLR.4doesfail,wecannotcomputetheOLSestimators,whichmeansstatisticalanalysisisirrelevant.nnUsingthefactthat(xix¯)(yiy¯)(xix¯)yi(seeAppendixA),wecani1i1writetheOLSslopeestimatorinequation(2.19)asn(xix¯)yii1ˆ1n.(2.49)2(xix¯)i1Becausewearenowinterestedinthebehaviorofˆ1acrossallpossiblesamples,ˆ1isproperlyviewedasarandomvariable.Wecanwriteˆ1intermsofthepopulationcoefficientsanderrorsbysubstitutingtherighthandsideof(2.48)into(2.49).Wehavenn(xix¯)yi(xix¯)(01xiui)i1_i1_ˆ122,(2.50)sxsxn22wherewehavedefinedthetotalvariationinxiassx(xix¯)inordertosimplifyi1thenotation.(Thisisnotquitethesamplevarianceofthexibecausewedonotdividebyn1.)Usingthealgebraofthesummationoperator,writethenumeratorofˆ1asnnn(xix¯)0(xix¯)1xi(xix¯)uii1i1i1(2.51)nnn0(xix¯)1(xix¯)xi(xix¯)ui.i1i1i149\nd7/14/994:31PMPage50Part1RegressionAnalysiswithCross-SectionalDatannn22AsshowninAppendixA,(xix¯)0and(xix¯)xi(xix¯)sx.i1i1i1n2Therefore,wecanwritethenumeratorofˆ1as1sx(xix¯)ui.Writingthisoveri1thedenominatorgivesn(xix¯)uini1_2ˆ1121(1/sx)diui,(2.52)sxi1wheredixix¯.Wenowseethattheestimatorˆ1equalsthepopulationslope1,plusatermthatisalinearcombinationintheerrors{u1,u2,…,un}.Conditionalontheval-uesofxi,therandomnessinˆ1isdueentirelytotheerrorsinthesample.Thefactthattheseerrorsaregenerallydifferentfromzeroiswhatcausesˆ1todifferfrom1.Usingtherepresentationin(2.52),wecanprovethefirstimportantstatisticalprop-ertyofOLS.THEOREM2.1(UNBIASEDNESSOFOLS)UsingAssumptionsSLR.1throughSLR.4,E(ˆ0)0,andE(ˆ1)1(2.53)foranyvaluesof0and1.Inotherwords,ˆ0isunbiasedfor0,andˆ1isunbiasedfor1.PROOF:Inthisproof,theexpectedvaluesareconditionalonthesamplevaluesof2theindependentvariable.Sincesxanddiarefunctionsonlyofthexi,theyarenonrandomintheconditioning.Therefore,from(2.53),nn22E(ˆ1)1E[(1/sx)diui]1(1/sx)E(diui)i1i1nn221(1/sx)diE(ui)1(1/sx)di01,i1i1wherewehaveusedthefactthattheexpectedvalueofeachui(conditionalon{x1,x2,...,xn})iszerounderAssumptionsSLR.2andSLR.3.Theproofforˆ0isnowstraightforward.Average(2.48)acrossitogety¯01x¯u¯,andplugthisintotheformulaforˆ0:ˆ0y¯ˆ1x¯01x¯u¯ˆ1x¯0(1ˆ1)x¯u¯.Then,conditionalonthevaluesofthexi,E(ˆ0)0E[(1ˆ1)x¯]E(u¯)0E[(1ˆ1)]x¯,sinceE(u¯)0byAssumptionsSLR.2andSLR.3.But,weshowedthatE(ˆ1)1,whichimpliesthatE[(ˆ11)]0.Thus,E(ˆ0)0.Bothoftheseargumentsarevalidforanyvaluesof0and1,andsowehaveestablishedunbiasedness.50\nd7/14/994:31PMPage51Chapter2TheSimpleRegressionModelRememberthatunbiasednessisafeatureofthesamplingdistributionsofˆ1andˆ0,whichsaysnothingabouttheestimatethatweobtainforagivensample.Wehopethat,ifthesampleweobtainissomehow“typical,”thenourestimateshouldbe“near”thepopulationvalue.Unfortunately,itisalwayspossiblethatwecouldobtainanunluckysamplethatwouldgiveusapointestimatefarfrom1,andwecanneverknowforsurewhetherthisisthecase.YoumaywanttoreviewthematerialonunbiasedestimatorsinAppendixC,especiallythesimulationexerciseinTableC.1thatillustratestheconceptofunbiasedness.Unbiasednessgenerallyfailsifanyofourfourassumptionsfail.Thismeansthatitisimportanttothinkabouttheveracityofeachassumptionforaparticularapplication.Aswehavealreadydiscussed,ifAssumptionSLR.4fails,thenwewillnotbeabletoobtaintheOLSestimates.AssumptionSLR.1requiresthatyandxbelinearlyrelated,withanadditivedisturbance.Thiscancertainlyfail.Butwealsoknowthatyandxcanbechosentoyieldinterestingnonlinearrelationships.Dealingwiththefailureof(2.47)requiresmoreadvancedmethodsthatarebeyondthescopeofthistext.Later,wewillhavetorelaxAssumptionSLR.2,therandomsamplingassumption,fortimeseriesanalysis.Butwhataboutusingitforcross-sectionalanalysis?Randomsamplingcanfailinacrosssectionwhensamplesarenotrepresentativeoftheunder-lyingpopulation;infact,somedatasetsareconstructedbyintentionallyoversamplingdifferentpartsofthepopulation.WewilldiscussproblemsofnonrandomsamplinginChapters9and17.TheassumptionweshouldconcentrateonfornowisSLR.3.IfSLR.3holds,theOLSestimatorsareunbiased.Likewise,ifSLR.3fails,theOLSestimatorsgenerallywillbebiased.Therearewaystodeterminethelikelydirectionandsizeofthebias,whichwewillstudyinChapter3.Thepossibilitythatxiscorrelatedwithuisalmostalwaysaconcerninsimpleregressionanalysiswithnonexperimentaldata,asweindicatedwithseveralexamplesinSection2.1.Usingsimpleregressionwhenucontainsfactorsaffectingythatarealsocorrelatedwithxcanresultinspuriouscorrelation:thatis,wefindarelationshipbetweenyandxthatisreallyduetootherunobservedfactorsthataffectyandalsohap-pentobecorrelatedwithx.EXAMPLE2.12(StudentMathPerformanceandtheSchoolLunchProgram)Letmath10denotethepercentageoftenthgradersatahighschoolreceivingapassingscoreonastandardizedmathematicsexam.Supposewewishtoestimatetheeffectofthefederallyfundedschoollunchprogramonstudentperformance.Ifanything,weexpectthelunchprogramtohaveapositiveceterisparibuseffectonperformance:allotherfactorsbeingequal,ifastudentwhoistoopoortoeatregularmealsbecomeseli-giblefortheschoollunchprogram,hisorherperformanceshouldimprove.Letlnchprgdenotethepercentageofstudentswhoareeligibleforthelunchprogram.Thenasimpleregressionmodelismath1001lnchprgu,(2.54)51\nd7/14/994:31PMPage52Part1RegressionAnalysiswithCross-SectionalDatawhereucontainsschoolandstudentcharacteristicsthataffectoverallschoolperformance.UsingthedatainMEAP93.RAWon408Michiganhighschoolsforthe1992–93schoolyear,weobtainmatˆh1032.140.319lnchprg2n408,R0.171Thisequationpredictsthatifstudenteligibilityinthelunchprogramincreasesby10per-centagepoints,thepercentageofstudentspassingthemathexamfallsbyabout3.2per-centagepoints.Dowereallybelievethathigherparticipationinthelunchprogramactuallycausesworseperformance?Almostcertainlynot.Abetterexplanationisthattheerrortermuinequation(2.54)iscorrelatedwithlnchprg.Infact,ucontainsfactorssuchasthepover-tyrateofchildrenattendingschool,whichaffectsstudentperformanceandishighlycorre-latedwitheligibilityinthelunchprogram.Variablessuchasschoolqualityandresourcesarealsocontainedinu,andthesearelikelycorrelatedwithlnchprg.Itisimportanttoremem-berthattheestimate0.319isonlyforthisparticularsample,butitssignandmagnitudemakeussuspectthatuandxarecorrelated,sothatsimpleregressionisbiased.Inadditiontoomittedvariables,thereareotherreasonsforxtobecorrelatedwithuinthesimpleregressionmodel.Sincethesameissuesariseinmultipleregressionanalysis,wewillpostponeasystematictreatmentoftheproblemuntilthen.VariancesoftheOLSEstimatorsInadditiontoknowingthatthesamplingdistributionofˆ1iscenteredabout1(ˆ1isunbiased),itisimportanttoknowhowfarwecanexpectˆ1tobeawayfrom1onaver-age.Amongotherthings,thisallowsustochoosethebestestimatoramongall,oratleastabroadclassof,theunbiasedestimators.Themeasureofspreadinthedistribu-tionofˆ1(andˆ0)thatiseasiesttoworkwithisthevarianceoritssquareroot,thestan-darddeviation.(SeeAppendixCforamoredetaileddiscussion.)ItturnsoutthatthevarianceoftheOLSestimatorscanbecomputedunderAssumptionsSLR.1throughSLR.4.However,theseexpressionswouldbesomewhatcomplicated.Instead,weaddanassumptionthatistraditionalforcross-sectionalanaly-sis.Thisassumptionstatesthatthevarianceoftheunobservable,u,conditionalonx,isconstant.Thisisknownasthehomoskedasticityor“constantvariance”assumption.ASSUMPTIONSLR.5(HOMOSKEDASTICITY)2Var(ux).Wemustemphasizethatthehomoskedasticityassumptionisquitedistinctfromthezeroconditionalmeanassumption,E(ux)0.AssumptionSLR.3involvestheexpectedvalueofu,whileAssumptionSLR.5concernsthevarianceofu(bothcondi-tionalonx).RecallthatweestablishedtheunbiasednessofOLSwithoutAssumptionSLR.5:thehomoskedasticityassumptionplaysnoroleinshowingthatˆ0andˆ1areunbiased.WeaddAssumptionSLR.5becauseitsimplifiesthevariancecalculationsfor52\nd7/14/994:31PMPage53Chapter2TheSimpleRegressionModelˆ0andˆ1andbecauseitimpliesthatordinaryleastsquareshascertainefficiencyprop-erties,whichwewillseeinChapter3.Ifweweretoassumethatuandxareindepen-dent,thenthedistributionofugivenxdoesnotdependonx,andsoE(ux)E(u)02andVar(ux).Butindependenceissometimestoostrongofanassumption.2222BecauseVar(ux)E(ux)[E(ux)]andE(ux)0,E(ux),whichmeans2222isalsotheunconditionalexpectationofu.Therefore,E(u)Var(u),because22E(u)0.Inotherwords,istheunconditionalvarianceofu,andsoisoftencalled2theerrorvarianceordisturbancevariance.Thesquarerootof,,isthestandarddeviationoftheerror.Alargermeansthatthedistributionoftheunobservablesaffect-ingyismorespreadout.ItisoftenusefultowriteAssumptionsSLR.3andSLR.5intermsofthecondi-tionalmeanandconditionalvarianceofy:E(yx)01x.(2.55)2Var(yx).(2.56)Inotherwords,theconditionalexpectationofygivenxislinearinx,butthevarianceofygivenxisconstant.ThissituationisgraphedinFigure2.8where00and10.Figure2.8Thesimpleregressionmodelunderhomoskedasticity.f(yx)yx1E(yx)01xx2x3x53\nd7/14/994:31PMPage54Part1RegressionAnalysiswithCross-SectionalDataWhenVar(ux)dependsonx,theerrortermissaidtoexhibitheteroskedasticity(ornonconstantvariance).SinceVar(ux)Var(yx),heteroskedasticityispresentwhen-everVar(yx)isafunctionofx.EXAMPLE2.13(HeteroskedasticityinaWageEquation)Inordertogetanunbiasedestimatoroftheceterisparibuseffectofeduconwage,wemustassumethatE(ueduc)0,andthisimpliesE(wageeduc)01educ.Ifwealso2makethehomoskedasticityassumption,thenVar(ueduc)doesnotdependonthe2levelofeducation,whichisthesameasassumingVar(wageeduc).Thus,whileaver-agewageisallowedtoincreasewitheducationlevel—itisthisrateofincreasethatweareinterestedindescribing—thevariabilityinwageaboutitsmeanisassumedtobecon-stantacrossalleducationlevels.Thismaynotberealistic.Itislikelythatpeoplewithmoreeducationhaveawidervarietyofinterestsandjobopportunities,whichcouldleadtomorewagevariabilityathigherlevelsofeducation.Peoplewithverylowlevelsofeduca-tionhaveveryfewopportunitiesandoftenmustworkattheminimumwage;thisservestoreducewagevariabilityatloweducationlevels.ThissituationisshowninFigure2.9.Ultimately,whetherAssumptionSLR.5holdsisanempiricalissue,andinChapter8wewillshowhowtotestAssumptionSLR.5.Figure2.9Var(wageeduc)increasingwitheduc.f(wageeduc)wageE(wageeduc)81201educ16educ54\nd7/14/994:31PMPage55Chapter2TheSimpleRegressionModelWiththehomoskedasticityassumptioninplace,wearereadytoprovethefol-lowing:THEOREM2.2(SAMPLINGVARIANCESOFTHEOLSESTIMATORS)UnderAssumptionsSLR.1throughSLR.5,222Var(ˆ1)n/sx(2.57)2(xix¯)i1n212nxii1Var(ˆ0)n,(2.58)2(xix¯)i1wheretheseareconditionalonthesamplevalues{x1,…,xn}.PROOF:WederivetheformulaforVar(ˆ1),leavingtheotherderivationasann2exercise.Thestartingpointisequation(2.52):ˆ11(1/sx)diui.Since1isjustai12constant,andweareconditioningonthexi,sxanddixix¯arealsononrandom.Furthermore,becausetheuiareindependentrandomvariablesacrossi(byrandomsampling),thevarianceofthesumisthesumofthevariances.Usingthesefacts,wehavenn22222Var(ˆ1)(1/sx)Vardiui(1/sx)diVar(ui)i1i1n22222(1/sx)di[sinceVar(ui)foralli]i1n2222222222(1/sx)di(1/sx)sx/sx,i1whichiswhatwewantedtoshow.Theformulas(2.57)and(2.58)arethe“standard”formulasforsimpleregressionanalysis,whichareinvalidinthepresenceofheteroskedasticity.Thiswillbeimportantwhenweturntoconfidenceintervalsandhypothesistestinginmultipleregressionanalysis.Formostpurposes,weareinterestedinVar(ˆ1).Itiseasytosummarizehowthis22variancedependsontheerrorvariance,,andthetotalvariationin{x1,x2,…,xn},sx.First,thelargertheerrorvariance,thelargerisVar(ˆ1).Thismakessensesincemorevariationintheunobservablesaffectingymakesitmoredifficulttopreciselyestimate1.Ontheotherhand,morevariabilityintheindependentvariableispreferred:asthevariabilityinthexiincreases,thevarianceofˆ1decreases.Thisalsomakesintuitive55\nd7/14/994:31PMPage56Part1RegressionAnalysiswithCross-SectionalDatasensesincethemorespreadoutisthesampleofindependentvariables,theeasieritistotraceouttherelationshipbetweenE(yx)andx.Thatis,theeasieritistoestimate1.Ifthereislittlevariationinthexi,thenitcanbehardtopinpointhowE(yx)varieswithx.Asthesamplesizeincreases,sodoesthetotalvariationinthexi.Therefore,alargersamplesizeresultsinasmallervarianceforˆ1.Thisanalysisshowsthat,ifweareinterestedinˆ1,andwehaveachoice,thenweshouldchoosethexitobeasspreadoutaspossible.Thisissometimespossiblewithexperimentaldata,butrarelydowehavethisluxuryinthesocialsciences:usuallywemusttakethexithatweobtainviarandomsampling.Sometimeswehaveanopportu-QUESTION2.5nitytoobtainlargersamplesizes,althoughShowthat,whenestimating0,itisbesttohavex¯0.WhatisVar(ˆ0)thiscanbecostly.nn22Forthepurposesofconstructingconfi-inthiscase?(Hint:Foranysampleofnumbers,xi(xix¯),i1i1denceintervalsandderivingteststatistics,withequalityonlyifx¯0.)wewillneedtoworkwiththestandarddeviationsofˆ1andˆ0,sd(ˆ1)andsd(ˆ0).Recallthattheseareobtainedbytakingthesquarerootsofthevariancesin(2.57)and2(2.58).Inparticular,sd(ˆ1)/sx,whereisthesquarerootof,andsxisthesquare2rootofsx.EstimatingtheErrorVarianceTheformulasin(2.57)and(2.58)allowustoisolatethefactorsthatcontributetoVar(ˆ1)andVar(ˆ0).Buttheseformulasareunknown,exceptintheextremelyrarecase22thatisknown.Nevertheless,wecanusethedatatoestimate,whichthenallowsustoestimateVar(ˆ1)andVar(ˆ0).Thisisagoodplacetoemphasizethedifferencebetweenthetheerrors(ordistur-bances)andtheresiduals,sincethisdistinctioniscrucialforconstructinganestimator2of.Equation(2.48)showshowtowritethepopulationmodelintermsofarandom-lysampledobservationasyi01xiui,whereuiistheerrorforobservationi.Wecanalsoexpressyiintermsofitsfittedvalueandresidualasinequation(2.32):yiˆ0ˆ1xiuˆi.Comparingthesetwoequations,weseethattheerrorshowsupintheequationcontainingthepopulationparameters,0and1.Ontheotherhand,theresidualsshowupintheestimatedequationwithˆ0andˆ1.Theerrorsareneverobserv-able,whiletheresidualsarecomputedfromthedata.Wecanuseequations(2.32)and(2.48)towritetheresidualsasafunctionoftheerrors:uˆiyiˆ0ˆ1xi(01xiui)ˆ0ˆ1xi,oruˆiui(ˆ00)(ˆ11)xi.(2.59)Althoughtheexpectedvalueofˆ0equals0,andsimilarlyforˆ1,uˆiisnotthesameasui.Thedifferencebetweenthemdoeshaveanexpectedvalueofzero.Nowthatweunderstandthedifferencebetweentheerrorsandtheresiduals,wecan56\nd7/14/994:31PMPage57Chapter2TheSimpleRegressionModeln222212returntoestimating.First,E(u),soanunbiased“estimator”ofisnui.i1Unfortunately,thisisnotatrueestimator,becausewedonotobservetheerrorsui.But,wedohaveestimatesoftheui,namelytheOLSresidualsuˆi.Ifwereplacetheerrorsn12withtheOLSresiduals,havenuˆiSSR/n.Thisisatrueestimator,becauseiti1givesacomputableruleforanysampleofdataonxandy.Oneslightdrawbacktothisestimatoristhatitturnsouttobebiased(althoughforlargenthebiasissmall).Sinceitiseasytocomputeanunbiasedestimator,weusethatinstead.TheestimatorSSR/nisbiasedessentiallybecauseitdoesnotaccountfortworestrictionsthatmustbesatisfiedbytheOLSresiduals.TheserestrictionsaregivenbythetwoOLSfirstorderconditions:nnuˆi0,xiuˆi0.(2.60)i1i1Onewaytoviewtheserestrictionsisthis:ifweknown2oftheresiduals,wecanalwaysgettheothertworesidualsbyusingtherestrictionsimpliedbythefirstorderconditionsin(2.60).Thus,thereareonlyn2degreesoffreedomintheOLSresid-uals[asopposedtondegreesoffreedomintheerrors.Ifwereplaceuˆiwithuiin(2.60),2therestrictionswouldnolongerhold.]Theunbiasedestimatorofthatwewillusemakesadegrees-of-freedomadjustment:n122ˆuˆiSSR/(n2).(2.61)(n2)i12(Thisestimatorissometimesdenoteds,butwecontinuetousetheconventionofputting“hats”overestimators.)2THEOREM2.3(UNBIASEDESTIMATIONOF)UnderAssumptionsSLR.1throughSLR.5,22E(ˆ).PROOF:Ifweaverageequation(2.59)acrossalliandusethefactthattheOLSresidualsaverageouttozero,wehave0u¯(ˆ00)(ˆ11)x¯;subtractingthis22from(2.59)givesuˆi(uiu¯)(ˆ11)(xix¯).Therefore,uˆin(uiu¯)n(ˆ122221)(xix¯)n2(uiu¯)(ˆ11)(xix¯).Summingacrossallnigivesuˆi(uiu¯)i1i122(ˆ11)(xix¯)2(ˆ11)ui(xix¯).Now,theexpectedvalueofthefirsti1i12termis(n1),somethingthatisshowninAppendixC.Theexpectedvalueofthesecond2222termissimplybecauseE[(ˆ11)]Var(ˆ1)/sx.Finally,thethirdtermcanbe222writtenas2(ˆ11)sx;takingexpectationsgives2.Puttingthesethreetermsn222222togethergivesEuˆi(n1)2(n2),sothatE[SSR/(n2)].i157\nd7/14/994:31PMPage58Part1RegressionAnalysiswithCross-SectionalData2Ifˆispluggedintothevarianceformulas(2.57)and(2.58),thenwehaveunbiasedestimatorsofVar(ˆ1)andVar(ˆ0).Lateron,wewillneedestimatorsofthestandarddeviationsofˆ1andˆ0,andthisrequiresestimating.Thenaturalestimatorofis—2ˆˆ,(2.62)andiscalledthestandarderroroftheregression(SER).(Othernamesforˆarethestandarderroroftheestimateandtherootmeansquarederror,butwewillnotusethese.)Althoughˆisnotanunbiasedestimatorof,wecanshowthatitisaconsis-tentestimatorof(seeAppendixC),anditwillserveourpurposeswell.Theestimateˆisinterestingsinceitisanestimateofthestandarddeviationintheunobservablesaffectingy;equivalently,itestimatesthestandarddeviationinyaftertheeffectofxhasbeentakenout.MostregressionpackagesreportthevalueofˆalongwiththeR-squared,intercept,slope,andotherOLSstatistics(underoneoftheseveralnameslistedabove).Fornow,ourprimaryinterestisinusingˆtoestimatethestan-darddeviationsofˆ0andˆ1.Sincesd(ˆ1)/sx,thenaturalestimatorofsd(ˆ1)isn1/22se(ˆ1)ˆ/sxˆ/(xix¯);i1thisiscalledthestandarderrorofˆ1.Notethatse(ˆ1)isviewedasarandomvariablewhenwethinkofrunningOLSoverdifferentsamplesofy;thisisbecauseˆvarieswithdifferentsamples.Foragivensample,se(ˆ1)isanumber,justasˆ1issimplyanumberwhenwecomputeitfromthegivendata.Similarly,se(ˆ0)isobtainedfromsd(ˆ0)byreplacingwithˆ.Thestandarderrorofanyestimategivesusanideaofhowprecisetheestimatoris.Standarderrorsplayacentralrolethroughoutthistext;wewillusethemtoconstructteststatisticsandconfi-denceintervalsforeveryeconometricprocedurewecover,startinginChapter4.2.6REGRESSIONTHROUGHTHEORIGINInrarecases,wewishtoimposetherestrictionthat,whenx0,theexpectedvalueofyiszero.Therearecertainrelationshipsforwhichthisisreasonable.Forexample,ifincome(x)iszero,thenincometaxrevenues(y)mustalsobezero.Inaddition,thereareproblemswhereamodelthatoriginallyhasanonzerointerceptistransformedintoamodelwithoutanintercept.Formally,wenowchooseaslopeestimator,whichwecall˜1,andalineoftheformy˜˜1x,(2.63)wherethetildasover˜1andyareusedtodistinguishthisproblemfromthemuchmorecommonproblemofestimatinganinterceptalongwithaslope.Obtaining(2.63)iscalledregressionthroughtheoriginbecausetheline(2.63)passesthroughthepointx0,y˜0.Toobtaintheslopeestimatein(2.63),westillrelyonthemethodofordi-naryleastsquares,whichinthiscaseminimizesthesumofsquaredresiduals58\nd7/14/994:31PMPage59Chapter2TheSimpleRegressionModeln2(yi˜1xi).(2.64)i1Usingcalculus,itcanbeshownthat˜1mustsolvethefirstorderconditionnxi(yi˜1xi)0.(2.65)i1Fromthiswecansolvefor˜1:nxiyii1˜1n,(2.66)2xii1providedthatnotallthexiarezero,acaseweruleout.Notehow˜1compareswiththeslopeestimatewhenwealsoestimatetheintercept(ratherthansetitequaltozero).Thesetwoestimatesarethesameif,andonlyif,x¯0.(Seeequation(2.49)forˆ1.)Obtaininganestimateof1usingregressionthroughtheoriginisnotdoneveryofteninappliedwork,andforgoodreason:iftheintercept00then˜1isabiasedestimatorof1.YouwillbeaskedtoprovethisinProblem2.8.SUMMARYWehaveintroducedthesimplelinearregressionmodelinthischapter,andwehavecov-ereditsbasicproperties.Givenarandomsample,themethodofordinaryleastsquaresisusedtoestimatetheslopeandinterceptparametersinthepopulationmodel.WehavedemonstratedthealgebraoftheOLSregressionline,includingcomputationoffittedvaluesandresiduals,andtheobtainingofpredictedchangesinthedependentvariableforagivenchangeintheindependentvariable.InSection2.4,wediscussedtwoissuesofpracticalimportance:(1)thebehavioroftheOLSestimateswhenwechangetheunitsofmeasurementofthedependentvariableortheindependentvariable;(2)theuseofthenaturallogtoallowforconstantelasticityandconstantsemi-elasticitymodels.InSection2.5,weshowedthat,underthefourAssumptionsSLR.1throughSLR.4,theOLSestimatorsareunbiased.Thekeyassumptionisthattheerrortermuhaszeromeangivenanyvalueoftheindependentvariablex.Unfortunately,therearereasonstothinkthisisfalseinmanysocialscienceapplicationsofsimpleregression,wheretheomittedfactorsinuareoftencorrelatedwithx.Whenweaddtheassumptionthatthevarianceoftheerrorgivenxisconstant,wegetsimpleformulasforthesamplingvari-ancesoftheOLSestimators.Aswesaw,thevarianceoftheslopeestimatorˆ1increasesastheerrorvarianceincreases,anditdecreaseswhenthereismoresamplevariationin2theindependentvariable.WealsoderivedanunbiasedestimatorforVar(u).InSection2.6,webrieflydiscussedregressionthroughtheorigin,wheretheslopeestimatorisobtainedundertheassumptionthattheinterceptiszero.Sometimesthisisuseful,butitappearsinfrequentlyinappliedwork.59\nd7/14/994:31PMPage60Part1RegressionAnalysiswithCross-SectionalDataMuchworkislefttobedone.Forexample,westilldonotknowhowtotesthypothesesaboutthepopulationparameters,0and1.Thus,althoughweknowthatOLSisunbiasedforthepopulationparametersunderAssumptionsSLR.1throughSLR.4,wehavenowayofdrawinginferenceaboutthepopulation.Othertopics,suchastheefficiencyofOLSrelativetootherpossibleprocedures,havealsobeenomitted.Theissuesofconfidenceintervals,hypothesistesting,andefficiencyarecentraltomultipleregressionanalysisaswell.Sincethewayweconstructconfidenceintervalsandteststatisticsisverysimilarformultipleregression—andbecausesimpleregres-sionisaspecialcaseofmultipleregression—ourtimeisbetterspentmovingontomul-tipleregression,whichismuchmorewidelyapplicablethansimpleregression.OurpurposeinChapter2wastogetyouthinkingabouttheissuesthatariseineconometricanalysisinafairlysimplesetting.KEYTERMSCoefficientofDeterminationPopulationRegressionFunction(PRF)ConstantElasticityModelPredictedVariableControlVariablePredictorVariableCovariateRegressandDegreesofFreedomRegressionThroughtheOriginDependentVariableRegressorElasticityResidualErrorTerm(Disturbance)ResidualSumofSquares(SSR)ErrorVarianceResponseVariableExplainedSumofSquares(SSE)R-squaredExplainedVariableSampleRegressionFunction(SRF)ExplanatoryVariableSemi-elasticityFirstOrderConditionsSimpleLinearRegressionModelFittedValueSlopeParameterHeteroskedasticityStandardErrorofˆ1HomoskedasticityStandardErroroftheRegression(SER)IndependentVariableSumofSquaredResidualsInterceptParameterTotalSumofSquares(SST)OrdinaryLeastSquares(OLS)ZeroConditionalMeanAssumptionOLSRegressionLinePROBLEMS2.1Letkidsdenotethenumberofchildreneverborntoawoman,andleteducdenoteyearsofeducationforthewoman.Asimplemodelrelatingfertilitytoyearsofeduca-tioniskids01educu,whereuistheunobservederror.60\nd7/14/994:31PMPage61Chapter2TheSimpleRegressionModel(i)Whatkindsoffactorsarecontainedinu?Aretheselikelytobecorre-latedwithlevelofeducation?(ii)Willasimpleregressionanalysisuncovertheceterisparibuseffectofeducationonfertility?Explain.2.2Inthesimplelinearregressionmodely01xu,supposethatE(u)0.Letting0E(u),showthatthemodelcanalwaysberewrittenwiththesameslope,butanewinterceptanderror,wherethenewerrorhasazeroexpectedvalue.2.3ThefollowingtablecontainstheACTscoresandtheGPA(gradepointaverage)for8collegestudents.Gradepointaverageisbasedonafour-pointscaleandhasbeenroundedtoonedigitafterthedecimal.StudentGPAACT12.82123.42433.02643.52753.62963.02572.72583.730(i)EstimatetherelationshipbetweenGPAandACTusingOLS;thatis,obtaintheinterceptandslopeestimatesintheequationGPˆAˆ0ˆ1ACT.Commentonthedirectionoftherelationship.Doestheintercepthaveausefulinterpretationhere?Explain.HowmuchhigheristheGPApre-dictedtobe,iftheACTscoreisincreasedby5points?(ii)Computethefittedvaluesandresidualsforeachobservationandverifythattheresiduals(approximately)sumtozero.(iii)WhatisthepredictedvalueofGPAwhenACT20?(iv)HowmuchofthevariationinGPAforthese8studentsisexplainedbyACT?Explain.2.4ThedatasetBWGHT.RAWcontainsdataonbirthstowomenintheUnitedStates.Twovariablesofinterestarethedependentvariable,infantbirthweightinounces(bwght),andanexplanatoryvariable,averagenumberofcigarettesthemothersmoked61\nd7/14/994:31PMPage62Part1RegressionAnalysiswithCross-SectionalDataperdayduringpregnancy(cigs).Thefollowingsimpleregressionwasestimatedusingdataonn1388births:bwgˆht119.770.514cigs(i)Whatisthepredictedbirthweightwhencigs0?Whataboutwhencigs20(onepackperday)?Commentonthedifference.(ii)Doesthissimpleregressionnecessarilycaptureacausalrelationshipbetweenthechild’sbirthweightandthemother’ssmokinghabits?Explain.2.5Inthelinearconsumptionfunctioncoˆnsˆ0ˆ1inc,the(estimated)marginalpropensitytoconsume(MPC)outofincomeissimplytheslope,ˆ1,whiletheaveragepropensitytoconsume(APC)iscoˆns/incˆ0/incˆ1.Usingobservationsfor100familiesonannualincomeandconsumption(bothmeasuredindollars),thefollowingequationisobtained:coˆns124.840.853inc2n100,R0.692(i)Interprettheinterceptinthisequationandcommentonitssignandmagnitude.(ii)Whatispredictedconsumptionwhenfamilyincomeis$30,000?(iii)Withinconthex-axis,drawagraphoftheestimatedMPCandAPC.2.6Usingdatafrom1988forhousessoldinAndover,MA,fromKielandMcClain(1995),thefollowingequationrelateshousingprice(price)tothedistancefromarecentlybuiltgarbageincinerator(dist):log(prˆice)9.400.312log(dist)2n135,R0.162(i)Interpretthecoefficientonlog(dist).Isthesignofthisestimatewhatyouexpectittobe?(ii)Doyouthinksimpleregressionprovidesanunbiasedestimatoroftheceterisparibuselasticityofpricewithrespecttodist?(Thinkaboutthecity’sdecisiononwheretoputtheincinerator.)(iii)Whatotherfactorsaboutahouseaffectitsprice?Mightthesebecorre-latedwithdistancefromtheincinerator?2.7Considerthesavingsfunction—sav01incu,uince,2whereeisarandomvariablewithE(e)0andVar(e)e.Assumethateisinde-pendentofinc.(i)ShowthatE(uinc)0,sothatthekeyzeroconditionalmeanassump-tion(AssumptionSLR.3)issatisfied.[Hint:Ifeisindependentofinc,thenE(einc)E(e).]62\nd7/14/994:31PMPage63Chapter2TheSimpleRegressionModel2(ii)ShowthatVar(uinc)einc,sothatthehomoskedasticityAssumptionSLR.5isviolated.Inparticular,thevarianceofsavincreaseswithinc.[Hint:Var(einc)Var(e),ifeandincareindependent.](iii)Provideadiscussionthatsupportstheassumptionthatthevarianceofsavingsincreaseswithfamilyincome.2.8Considerthestandardsimpleregressionmodely01xuunderAssumptionsSLR.1throughSLR.4.Thus,theusualOLSestimatorsˆ0andˆ1areunbi-asedfortheirrespectivepopulationparameters.Let˜1betheestimatorof1obtainedbyassumingtheinterceptiszero(seeSection2.6).(i)FindE(˜1)intermsofthexi,0,and1.Verifythat˜1isunbiasedfor1whenthepopulationintercept(0)iszero.Arethereothercaseswhere˜1isunbiased?(ii)Findthevarianceof˜1.(Hint:Thevariancedoesnotdependon0.)nn2(iii)ShowthatVar(˜1)Var(ˆ1).[Hint:Foranysampleofdata,xii1i12(xix¯),withstrictinequalityunlessx¯0.](iv)Commentonthetradeoffbetweenbiasandvariancewhenchoosingbetweenˆ1and˜1.2.9(i)Letˆ0andˆ1betheinterceptandslopefromtheregressionofyionxi,usingnobservations.Letc1andc2,withc20,beconstants.Let˜0and˜1betheinterceptandslopefromtheregressionc1yionc2xi.Showthat˜1(c1/c2)ˆ1and˜0c1ˆ0,therebyverifyingtheclaimsonunitsofmeasurementinSection2.4.[Hint:Toobtain˜1,plugthescaledversionsofxandyinto(2.19).Then,use(2.17)for˜0,beingsuretopluginthescaledxandyandthecorrectslope.](ii)Nowlet˜0and˜1befromtheregression(c1yi)on(c2xi)(withnorestrictiononc1orc2).Showthat˜1ˆ1and˜0ˆ0c1c2ˆ1.COMPUTEREXERCISES2.10Thedatain401K.RAWareasubsetofdataanalyzedbyPapke(1995)tostudytherelationshipbetweenparticipationina401(k)pensionplanandthegenerosityoftheplan.Thevariableprateisthepercentageofeligibleworkerswithanactiveaccount;thisisthevariablewewouldliketoexplain.Themeasureofgenerosityistheplanmatchrate,mrate.Thisvariablegivestheaverageamountthefirmcontributestoeachworker’splanforeach$1contributionbytheworker.Forexample,ifmrate0.50,thena$1contributionbytheworkerismatchedbya50¢contributionbythefirm.(i)Findtheaverageparticipationrateandtheaveragematchrateinthesampleofplans.(ii)Nowestimatethesimpleregressionequationpraˆteˆ0ˆ1mrate,andreporttheresultsalongwiththesamplesizeandR-squared.(iii)Interprettheinterceptinyourequation.Interpretthecoefficientonmrate.(iv)Findthepredictedpratewhenmrate3.5.Isthisareasonablepredic-tion?Explainwhatishappeninghere.63\nd7/14/994:31PMPage64Part1RegressionAnalysiswithCross-SectionalData(v)Howmuchofthevariationinprateisexplainedbymrate?Isthisalotinyouropinion?2.11ThedatasetinCEOSAL2.RAWcontainsinformationonchiefexecutiveofficersforU.S.corporations.Thevariablesalaryisannualcompensation,inthousandsofdol-lars,andceotenispriornumberofyearsascompanyCEO.(i)Findtheaveragesalaryandtheaveragetenureinthesample.(ii)HowmanyCEOsareintheirfirstyearasCEO(thatis,ceoten0)?WhatisthelongesttenureasaCEO?(iii)Estimatethesimpleregressionmodellog(salary)01ceotenu,andreportyourresultsintheusualform.Whatisthe(approximate)pre-dictedpercentageincreaseinsalarygivenonemoreyearasaCEO?2.12UsethedatainSLEEP75.RAWfromBiddleandHamermesh(1990)tostudywhetherthereisatradeoffbetweenthetimespentsleepingperweekandthetimespentinpaidwork.Wecoulduseeithervariableasthedependentvariable.Forconcreteness,estimatethemodelsleep01totwrku,wheresleepisminutesspentsleepingatnightperweekandtotwrkistotalminutesworkedduringtheweek.(i)Reportyourresultsinequationformalongwiththenumberofobser-2vationsandR.Whatdoestheinterceptinthisequationmean?(ii)Iftotwrkincreasesby2hours,byhowmuchissleepestimatedtofall?Doyoufindthistobealargeeffect?2.13UsethedatainWAGE2.RAWtoestimateasimpleregressionexplainingmonthlysalary(wage)intermsofIQscore(IQ).(i)FindtheaveragesalaryandaverageIQinthesample.Whatisthestan-darddeviationofIQ?(IQscoresarestandardizedsothattheaverageinthepopulationis100withastandarddeviationequalto15.)(ii)Estimateasimpleregressionmodelwhereaone-pointincreaseinIQchangeswagebyaconstantdollaramount.UsethismodeltofindthepredictedincreaseinwageforanincreaseinIQof15points.DoesIQexplainmostofthevariationinwage?(iii)Nowestimateamodelwhereeachone-pointincreaseinIQhasthesamepercentageeffectonwage.IfIQincreasesby15points,whatistheapproximatepercentageincreaseinpredictedwage?2.14Forthepopulationoffirmsinthechemicalindustry,letrddenoteannualexpen-dituresonresearchanddevelopment,andletsalesdenoteannualsales(bothareinmil-lionsofdollars).(i)Writedownamodel(notanestimatedequation)thatimpliesaconstantelasticitybetweenrdandsales.Whichparameteristheelasticity?(ii)NowestimatethemodelusingthedatainRDCHEM.RAW.Writeouttheestimatedequationintheusualform.Whatistheestimatedelasticityofrdwithrespecttosales?Explaininwordswhatthiselasticitymeans.64\nd7/14/994:31PMPage65Chapter2TheSimpleRegressionModelAPPENDIX2AMinimizingtheSumofSquaredResidualsWeshowthattheOLSestimatesˆ0andˆ1dominimizethesumofsquaredresiduals,asassertedinSection2.2.Formally,theproblemistocharacterizethesolutionsˆ0andˆ1totheminimizationproblemn2min(yib0b1xi),b0,b1i1whereb0andb1arethedummyargumentsfortheoptimizationproblem;forsimplicity,callthisfunctionQ(b0,b1).Byafundamentalresultfrommultivariablecalculus(seeAppendixA),anecessaryconditionforˆ0andˆ1tosolvetheminimizationproblemisthatthepartialderivativesofQ(b0,b1)withrespecttob0andb1mustbezerowheneval-uatedatˆ0,ˆ1:Q(ˆ0,ˆ1)/b00andQ(ˆ0,ˆ1)/b10.Usingthechainrulefromcalculus,thesetwoequationsbecomen2(yiˆ0ˆ1xi)0.i1n2xi(yiˆ0ˆ1xi)0.i1Thesetwoequationsarejust(2.14)and(2.15)multipliedby2nand,therefore,aresolvedbythesameˆ0andˆ1.Howdoweknowthatwehaveactuallyminimizedthesumofsquaredresiduals?Thefirstorderconditionsarenecessarybutnotsufficientconditions.Onewaytoveri-fythatwehaveminimizedthesumofsquaredresidualsistowrite,foranyb0andb1,n2Q(b0,b1)(yiˆ0ˆ1xi(ˆ0b0)(ˆ1b1)xi)i1n2(uˆi(ˆ0b0)(ˆ1b1)xi)i1nnn2222uˆin(ˆ0b0)(ˆ1b1)xi2(ˆ0b0)(ˆ1b1)xi,i1i1i1wherewehaveusedequations(2.30)and(2.31).Thesumofsquaredresidualsdoesnotdependonb0orb1,whilethesumofthelastthreetermscanbewrittenasn2[(ˆ0b0)(ˆ1b1)xi],i1ascanbeverifiedbystraightforwardalgebra.Becausethisisasumofsquaredterms,itcanbeatmostzero.Therefore,itissmallestwhenb0=ˆ0andb1=ˆ1.65\nd7/14/994:55PMPage66ChapterThreeMultipleRegressionAnalysis:EstimationnChapter2,welearnedhowtousesimpleregressionanalysistoexplainadepen-Identvariable,y,asafunctionofasingleindependentvariable,x.Theprimarydraw-backinusingsimpleregressionanalysisforempiricalworkisthatitisverydiffi-culttodrawceterisparibusconclusionsabouthowxaffectsy:thekeyassumption,SLR.3—thatallotherfactorsaffectingyareuncorrelatedwithx—isoftenunrealistic.Multipleregressionanalysisismoreamenabletoceterisparibusanalysisbecauseitallowsustoexplicitlycontrolformanyotherfactorswhichsimultaneouslyaffectthedependentvariable.Thisisimportantbothfortestingeconomictheoriesandforevaluat-ingpolicyeffectswhenwemustrelyonnonexperimentaldata.Becausemultipleregres-sionmodelscanaccommodatemanyexplanatoryvariablesthatmaybecorrelated,wecanhopetoinfercausalityincaseswheresimpleregressionanalysiswouldbemisleading.Naturally,ifweaddmorefactorstoourmodelthatareusefulforexplainingy,thenmoreofthevariationinycanbeexplained.Thus,multipleregressionanalysiscanbeusedtobuildbettermodelsforpredictingthedependentvariable.Anadditionaladvantageofmultipleregressionanalysisisthatitcanincorporatefairlygeneralfunctionalformrelationships.Inthesimpleregressionmodel,onlyonefunctionofasingleexplanatoryvariablecanappearintheequation.Aswewillsee,themultipleregressionmodelallowsformuchmoreflexibility.Section3.1formallyintroducesthemultipleregressionmodelandfurtherdis-cussestheadvantagesofmultipleregressionoversimpleregression.InSection3.2,wedemonstratehowtoestimatetheparametersinthemultipleregressionmodelusingthemethodofordinaryleastsquares.InSections3.3,3.4,and3.5,wedescribevarioussta-tisticalpropertiesoftheOLSestimators,includingunbiasednessandefficiency.Themultipleregressionmodelisstillthemostwidelyusedvehicleforempiricalanalysisineconomicsandothersocialsciences.Likewise,themethodofordinaryleastsquaresispopularlyusedforestimatingtheparametersofthemultipleregressionmodel.3.1MOTIVATIONFORMULTIPLEREGRESSIONTheModelwithTwoIndependentVariablesWebeginwithsomesimpleexamplestoshowhowmultipleregressionanalysiscanbeusedtosolveproblemsthatcannotbesolvedbysimpleregression.66\nd7/14/994:55PMPage67Chapter3MultipleRegressionAnalysis:EstimationThefirstexampleisasimplevariationofthewageequationintroducedinChapter2forobtainingtheeffectofeducationonhourlywage:wage01educ2experu,(3.1)whereexperisyearsoflabormarketexperience.Thus,wageisdeterminedbythetwoexplanatoryorindependentvariables,educationandexperience,andbyotherunob-servedfactors,whicharecontainedinu.Wearestillprimarilyinterestedintheeffectofeduconwage,holdingfixedallotherfactorsaffectingwage;thatis,weareinterest-edintheparameter1.Comparedwithasimpleregressionanalysisrelatingwagetoeduc,equation(3.1)effectivelytakesexperoutoftheerrortermandputsitexplicitlyintheequation.Becauseexperappearsintheequation,itscoefficient,2,measurestheceterisparibuseffectofexperonwage,whichisalsoofsomeinterest.Notsurprisingly,justaswithsimpleregression,wewillhavetomakeassumptionsabouthowuin(3.1)isrelatedtotheindependentvariables,educandexper.However,aswewillseeinSection3.2,thereisonethingofwhichwecanbeconfident:since(3.1)containsexperienceexplicitly,wewillbeabletomeasuretheeffectofeducationonwage,holdingexperiencefixed.Inasimpleregressionanalysis—whichputsexperintheerrorterm—wewouldhavetoassumethatexperienceisuncorrelatedwithedu-cation,atenuousassumption.Asasecondexample,considertheproblemofexplainingtheeffectofperstudentspending(expend)ontheaveragestandardizedtestscore(avgscore)atthehighschoollevel.Supposethattheaveragetestscoredependsonfunding,averagefamilyincome(avginc),andotherunobservables:avgscore01expend2avgincu.(3.2)Thecoefficientofinterestforpolicypurposesis1,theceterisparibuseffectofexpendonavgscore.Byincludingavgincexplicitlyinthemodel,weareabletocontrolforitseffectonavgscore.Thisislikelytobeimportantbecauseaveragefamilyincometendstobecorrelatedwithperstudentspending:spendinglevelsareoftendeterminedbybothpropertyandlocalincometaxes.Insimpleregressionanalysis,avgincwouldbein-cludedintheerrorterm,whichwouldlikelybecorrelatedwithexpend,causingtheOLSestimatorof1inthetwo-variablemodeltobebiased.Inthetwoprevioussimilarexamples,wehaveshownhowobservablefactorsotherthanthevariableofprimaryinterest[educinequation(3.1),expendinequation(3.2)]canbeincludedinaregressionmodel.Generally,wecanwriteamodelwithtwoinde-pendentvariablesasy01x12x2u,(3.3)where0istheintercept,1measuresthechangeinywithrespecttox1,holdingotherfactorsfixed,and2measuresthechangeinywithrespecttox2,holdingotherfactorsfixed.67\nd7/14/994:55PMPage68Part1RegressionAnalysiswithCross-SectionalDataMultipleregressionanalysisisalsousefulforgeneralizingfunctionalrelationshipsbetweenvariables.Asanexample,supposefamilyconsumption(cons)isaquadraticfunctionoffamilyincome(inc):2cons01inc2incu,(3.4)whereucontainsotherfactorsaffectingconsumption.Inthismodel,consumptiondependsononlyoneobservedfactor,income;soitmightseemthatitcanbehandledinasimpleregressionframework.Butthemodelfallsoutsidesimpleregression2becauseitcontainstwofunctionsofincome,incandinc(andthereforethreeparame-ters,0,1,and2).Nevertheless,theconsumptionfunctioniseasilywrittenasa2regressionmodelwithtwoindependentvariablesbylettingx1incandx2inc.Mechanically,therewillbenodifferenceinusingthemethodofordinaryleastsquares(introducedinSection3.2)toestimateequationsasdifferentas(3.1)and(3.4).Eachequationcanbewrittenas(3.3),whichisallthatmattersforcomputation.Thereis,however,animportantdifferenceinhowoneinterpretstheparameters.Inequation(3.1),1istheceterisparibuseffectofeduconwage.Theparameter1hasnosuchinterpretationin(3.4).Inotherwords,itmakesnosensetomeasuretheeffectofincon22conswhileholdingincfixed,becauseifincchanges,thensomustinc!Instead,thechangeinconsumptionwithrespecttothechangeinincome—themarginalpropen-sitytoconsume—isapproximatedbycons122inc.incSeeAppendixAforthecalculusneededtoderivethisequation.Inotherwords,themar-ginaleffectofincomeonconsumptiondependson2aswellason1andthelevelofincome.Thisexampleshowsthat,inanyparticularapplication,thedefinitionoftheindependentvariablesarecrucial.Butforthetheoreticaldevelopmentofmultipleregression,wecanbevagueaboutsuchdetails.WewillstudyexampleslikethismorecompletelyinChapter6.Inthemodelwithtwoindependentvariables,thekeyassumptionabouthowuisrelatedtox1andx2isE(ux1,x2)0.(3.5)Theinterpretationofcondition(3.5)issimilartotheinterpretationofAssumptionSLR.3forsimpleregressionanalysis.Itmeansthat,foranyvaluesofx1andx2inthepopulation,theaverageunobservableisequaltozero.Aswithsimpleregression,theimportantpartoftheassumptionisthattheexpectedvalueofuisthesameforallcom-binationsofx1andx2;thatthiscommonvalueiszeroisnoassumptionatallaslongastheintercept0isincludedinthemodel(seeSection2.1).Howcanweinterpretthezeroconditionalmeanassumptioninthepreviousexam-ples?Inequation(3.1),theassumptionisE(ueduc,exper)0.Thisimpliesthatotherfactorsaffectingwagearenotrelatedonaveragetoeducandexper.Therefore,ifwethinkinnateabilityispartofu,thenwewillneedaverageabilitylevelstobethesameacrossallcombinationsofeducationandexperienceintheworkingpopulation.This68\nd7/14/994:55PMPage69Chapter3MultipleRegressionAnalysis:Estimationmayormaynotbetrue,but,aswewillseeinSection3.3,thisisthequestionweneedtoaskinordertodeterminewhetherthemethodofordinaryleastsquaresproducesunbiasedestimators.Theexamplemeasuringstudentperformance[equation(3.2)]issimilartothewageequation.ThezeroconditionalmeanassumptionisE(uexpend,avginc)0,whichmeansthatotherfactorsaffectingtestscores—schoolorstudentcharacteristics—are,onaverage,unrelatedtoperstudentfund-ingandaveragefamilyincome.QUESTION3.1Whenappliedtothequadraticcon-Asimplemodeltoexplaincitymurderrates(murdrate)intermsofsumptionfunctionin(3.4),thezerocondi-theprobabilityofconviction(prbconv)andaveragesentencelengthtionalmeanassumptionhasaslightlydif-(avgsen)isferentinterpretation.Writtenliterally,murdrate01prbconv2avgsenu.equation(3.5)becomesE(uinc,inc2)0.2Whataresomefactorscontainedinu?Doyouthinkthekeyassum-Sinceincisknownwhenincisknown,2ption(3.5)islikelytohold?includingincintheexpectationisredun-2dant:E(uinc,inc)0isthesameas2E(uinc)0.Nothingiswrongwithputtingincalongwithincintheexpectationwhenstatingtheassumption,butE(uinc)0ismoreconcise.TheModelwithkIndependentVariablesOnceweareinthecontextofmultipleregression,thereisnoneedtostopwithtwoindependentvariables.Multipleregressionanalysisallowsmanyobservedfactorstoaffecty.Inthewageexample,wemightalsoincludeamountofjobtraining,yearsoftenurewiththecurrentemployer,measuresofability,andevendemographicvariableslikenumberofsiblingsormother’seducation.Intheschoolfundingexample,addi-tionalvariablesmightincludemeasuresofteacherqualityandschoolsize.Thegeneralmultiplelinearregressionmodel(alsocalledthemultipleregressionmodel)canbewritteninthepopulationasy01x12x23x3…kxku,(3.6)where0istheintercept,1istheparameterassociatedwithx1,2istheparameterassociatedwithx2,andsoon.Sincetherearekindependentvariablesandanintercept,equation(3.6)containsk1(unknown)populationparameters.Forshorthandpur-poses,wewillsometimesrefertotheparametersotherthantheinterceptasslopepara-meters,eventhoughthisisnotalwaysliterallywhattheyare.[Seeequation(3.4),whereneither1nor2isitselfaslope,buttogethertheydeterminetheslopeoftherelationshipbetweenconsumptionandincome.]TheterminologyformultipleregressionissimilartothatforsimpleregressionandisgiveninTable3.1.Justasinsimpleregression,thevariableuistheerrortermordisturbance.Itcontainsfactorsotherthanx1,x2,…,xkthataffecty.Nomatterhowmanyexplanatoryvariablesweincludeinourmodel,therewillalwaysbefactorswecannotinclude,andthesearecollectivelycontainedinu.Whenapplyingthegeneralmultipleregressionmodel,wemustknowhowtointer-prettheparameters.Wewillgetplentyofpracticenowandinsubsequentchapters,but69\nd7/14/994:55PMPage70Part1RegressionAnalysiswithCross-SectionalDataTable3.1TerminologyforMultipleRegressionyx1,x2,…,xkDependentVariableIndependentVariablesExplainedVariableExplanatoryVariablesResponseVariableControlVariablesPredictedVariablePredictorVariablesRegressandRegressorsitisusefulatthispointtoberemindedofsomethingswealreadyknow.SupposethatCEOsalary(salary)isrelatedtofirmsalesandCEOtenurewiththefirmby2log(salary)01log(sales)2ceoten3ceotenu.(3.7)Thisfitsintothemultipleregressionmodel(withk3)bydefiningylog(salary),2x1log(sales),x2ceoten,andx3ceoten.AsweknowfromChapter2,thepara-meter1isthe(ceterisparibus)elasticityofsalarywithrespecttosales.If30,then1002isapproximatelytheceterisparibuspercentageincreaseinsalarywhenceotenincreasesbyoneyear.When30,theeffectofceotenonsalaryismorecompli-cated.WewillpostponeadetailedtreatmentofgeneralmodelswithquadraticsuntilChapter6.Equation(3.7)providesanimportantreminderaboutmultipleregressionanalysis.Theterm“linear”inmultiplelinearregressionmodelmeansthatequation(3.6)islin-earintheparameters,j.Equation(3.7)isanexampleofamultipleregressionmodelthat,whilelinearinthej,isanonlinearrelationshipbetweensalaryandthevariablessalesandceoten.Manyapplicationsofmultiplelinearregressioninvolvenonlinearrelationshipsamongtheunderlyingvariables.Thekeyassumptionforthegeneralmultipleregressionmodeliseasytostateintermsofaconditionalexpectation:E(ux1,x2,…,xk)0.(3.8)Ataminimum,equation(3.8)requiresthatallfactorsintheunobservederrortermbeuncorrelatedwiththeexplanatoryvariables.Italsomeansthatwehavecorrectlyaccountedforthefunctionalrelationshipsbetweentheexplainedandexplanatoryvari-ables.Anyproblemthatallowsutobecorrelatedwithanyoftheindependentvariablescauses(3.8)tofail.InSection3.3,wewillshowthatassumption(3.8)impliesthatOLSisunbiasedandwillderivethebiasthatariseswhenakeyvariablehasbeenomitted70\nd7/14/994:55PMPage71Chapter3MultipleRegressionAnalysis:Estimationfromtheequation.InChapters15and16,wewillstudyotherreasonsthatmightcause(3.8)tofailandshowwhatcanbedoneincaseswhereitdoesfail.3.2MECHANICSANDINTERPRETATIONOFORDINARYLEASTSQUARESWenowsummarizesomecomputationalandalgebraicfeaturesofthemethodofordi-naryleastsquaresasitappliestoaparticularsetofdata.Wealsodiscusshowtointer-prettheestimatedequation.ObtainingtheOLSEstimatesWefirstconsiderestimatingthemodelwithtwoindependentvariables.TheestimatedOLSequationiswritteninaformsimilartothesimpleregressioncase:yˆˆ0ˆ1x1ˆ2x2,(3.9)whereˆ0istheestimateof0,ˆ1istheestimateof1,andˆ2istheestimateof2.Buthowdoweobtainˆ0,ˆ1,andˆ2?Themethodofordinaryleastsquareschoosestheestimatestominimizethesumofsquaredresiduals.Thatis,givennobservationsony,x1,andx2,{(xi1,xi2,yi):i1,2,…,n},theestimatesˆ0,ˆ1,andˆ2arechosensimulta-neouslytomaken2(yiˆ0ˆ1xi1ˆ2xi2)(3.10)i1assmallaspossible.InordertounderstandwhatOLSisdoing,itisimportanttomasterthemeaningoftheindexingoftheindependentvariablesin(3.10).Theindependentvariableshavetwosubscriptshere,ifollowedbyeither1or2.Theisubscriptreferstotheobservationnumber.Thus,thesumin(3.10)isoveralli1tonobservations.Thesecondindexissimplyamethodofdistinguishingbetweendifferentindependentvariables.Intheexamplerelatingwagetoeducandexper,xi1educiiseducationforpersoniinthesample,andxi2experiisexperienceforpersoni.Thesumofsquaredresidualsinn2equation(3.10)is(wageiˆ0ˆ1educiˆ2experi).Inwhatfollows,theisub-i1scriptisreservedforindexingtheobservationnumber.Ifwewritexij,thenthismeansthththeiobservationonthejindependentvariable.(Someauthorsprefertoswitchtheorderoftheobservationnumberandthevariablenumber,sothatx1iisobservationionvariableone.Butthisisjustamatterofnotationaltaste.)Inthegeneralcasewithkindependentvariables,weseekestimatesˆ0,ˆ1,…,ˆkintheequationyˆˆ0ˆ1x1ˆ2x2…ˆkxk.(3.11)TheOLSestimates,k1ofthem,arechosentominimizethesumofsquaredresiduals:71\nd7/14/994:55PMPage72Part1RegressionAnalysiswithCross-SectionalDatan2(yiˆ0ˆ1xi1…ˆkxik).(3.12)i1Thisminimizationproblemcanbesolvedusingmultivariablecalculus(seeAppendix3A).Thisleadstok1linearequationsink1unknownsˆ0,ˆ1,…,ˆk:n(yiˆ0ˆ1xi1…ˆkxik)0i1nxi1(yiˆ0ˆ1xi1…ˆkxik)0i1nxi2(yiˆ0ˆ1xi1…ˆkxik)0(3.13)i1nxik(yiˆ0ˆ1xi1…ˆkxik)0.i1TheseareoftencalledtheOLSfirstorderconditions.AswiththesimpleregressionmodelinSection2.2,theOLSfirstorderconditionscanbemotivatedbythemethodofmoments:underassumption(3.8),E(u)0andE(xju)0,wherej1,2,…,k.Theequationsin(3.13)arethesamplecounterpartsofthesepopulationmoments.Forevenmoderatelysizednandk,solvingtheequationsin(3.13)byhandcalcula-tionsistedious.Nevertheless,moderncomputersrunningstandardstatisticsandecono-metricssoftwarecansolvetheseequationswithlargenandkveryquickly.Thereisonlyoneslightcaveat:wemustassumethattheequationsin(3.13)canbesolveduniquelyfortheˆj.Fornow,wejustassumethis,asitisusuallythecaseinwell-specifiedmodels.InSection3.3,westatetheassumptionneededforuniqueOLSesti-matestoexist(seeAssumptionMLR.4).Asinsimpleregressionanalysis,equation(3.11)iscalledtheOLSregressionline,orthesampleregressionfunction(SRF).Wewillcallˆ0theOLSinterceptestimateandˆ1,…,ˆktheOLSslopeestimates(correspondingtotheindependentvariablesx1,x2,…,xk).InordertoindicatethatanOLSregressionhasbeenrun,wewilleitherwriteoutequation(3.11)withyandx1,…,xkreplacedbytheirvariablenames(suchaswage,educ,andexper),orwewillsaythat“werananOLSregressionofyonx1,x2,…,xk”orthat“weregressedyonx1,x2,…,xk.”TheseareshorthandforsayingthatthemethodofordinaryleastsquareswasusedtoobtaintheOLSequation(3.11).Unlessexplicitlystatedotherwise,wealwaysestimateaninterceptalongwiththeslopes.InterpretingtheOLSRegressionEquationMoreimportantthanthedetailsunderlyingthecomputationoftheˆjistheinterpretationoftheestimatedequation.Webeginwiththecaseoftwoindependentvariables:72\nd7/14/994:55PMPage73Chapter3MultipleRegressionAnalysis:Estimationyˆˆ0ˆ1x1ˆ2x2.(3.14)Theinterceptˆ0inequation(3.14)isthepredictedvalueofywhenx10andx20.Sometimessettingx1andx2bothequaltozeroisaninterestingscenario,butinothercasesitwillnotmakesense.Nevertheless,theinterceptisalwaysneededtoobtainapredictionofyfromtheOLSregressionline,as(3.14)makesclear.Theestimatesˆ1andˆ2havepartialeffect,orceterisparibus,interpretations.Fromequation(3.14),wehaveyˆˆ1x1ˆ2x2,sowecanobtainthepredictedchangeinygiventhechangesinx1andx2.(Notehowtheintercepthasnothingtodowiththechangesiny.)Inparticular,whenx2isheldfixed,sothatx20,thenyˆˆ1x1,holdingx2fixed.Thekeypointisthat,byincludingx2inourmodel,weobtainacoef-ficientonx1withaceterisparibusinterpretation.Thisiswhymultipleregressionanaly-sisissouseful.Similarly,yˆˆ2x2,holdingx1fixed.EXAMPLE3.1(DeterminantsofCollegeGPA)ThevariablesinGPA1.RAWincludecollegegradepointaverage(colGPA),highschoolGPA(hsGPA),andachievementtestscore(ACT)forasampleof141studentsfromalargeuni-versity;bothcollegeandhighschoolGPAsareonafour-pointscale.Weobtainthefol-lowingOLSregressionlinetopredictcollegeGPAfromhighschoolGPAandachievementtestscore:colˆGPA1.29.453hsGPA.0094ACT.(3.15)Howdoweinterpretthisequation?First,theintercept1.29isthepredictedcollegeGPAifhsGPAandACTarebothsetaszero.SincenoonewhoattendscollegehaseitherazerohighschoolGPAorazeroontheachievementtest,theinterceptinthisequationisnot,byitself,meaningful.MoreinterestingestimatesaretheslopecoefficientsonhsGPAandACT.Asexpected,thereisapositivepartialrelationshipbetweencolGPAandhsGPA:holdingACTfixed,anotherpointonhsGPAisassociatedwith.453ofapointonthecollegeGPA,oralmosthalfapoint.Inotherwords,ifwechoosetwostudents,AandB,andthesestudentshavethesameACTscore,butthehighschoolGPAofStudentAisonepointhigherthanthehighschoolGPAofStudentB,thenwepredictStudentAtohaveacollegeGPA.453higherthanthatofStudentB.[Thissaysnothingaboutanytwoactualpeople,butitisourbestprediction.]73\nd7/14/994:55PMPage74Part1RegressionAnalysiswithCross-SectionalDataThesignonACTimpliesthat,whileholdinghsGPAfixed,achangeintheACTscoreof10points—averylargechange,sincetheaveragescoreinthesampleisabout24withastandarddeviationlessthanthree—affectscolGPAbylessthanone-tenthofapoint.Thisisasmalleffect,anditsuggeststhat,oncehighschoolGPAisaccountedfor,theACTscoreisnotastrongpredictorofcollegeGPA.(Naturally,therearemanyotherfactorsthatcon-tributetoGPA,butherewefocusonstatisticsavailableforhighschoolstudents.)Later,afterwediscussstatisticalinference,wewillshowthatnotonlyisthecoefficientonACTpracticallysmall,itisalsostatisticallyinsignificant.IfwefocusonasimpleregressionanalysisrelatingcolGPAtoACTonly,weobtaincolˆGPA2.40.0271ACT;thus,thecoefficientonACTisalmostthreetimesaslargeastheestimatein(3.15).ButthisequationdoesnotallowustocomparetwopeoplewiththesamehighschoolGPA;itcor-respondstoadifferentexperiment.Wesaymoreaboutthedifferencesbetweenmultipleandsimpleregressionlater.Thecasewithmorethantwoindependentvariablesissimilar.TheOLSregressionlineisyˆˆ0ˆ1x1ˆ2x2…ˆkxk.(3.16)Writtenintermsofchanges,yˆˆ1x1ˆ2x2…ˆkxk.(3.17)Thecoefficientonx1measuresthechangeinyˆduetoaone-unitincreaseinx1,holdingallotherindependentvariablesfixed.Thatis,yˆˆ1x1,(3.18)holdingx2,x3,…,xkfixed.Thus,wehavecontrolledforthevariablesx2,x3,…,xkwhenestimatingtheeffectofx1ony.Theothercoefficientshaveasimilarinterpretation.Thefollowingisanexamplewiththreeindependentvariables.EXAMPLE3.2(HourlyWageEquation)Usingthe526observationsonworkersinWAGE1.RAW,weincludeeduc(yearsofeduca-tion),exper(yearsoflabormarketexperience),andtenure(yearswiththecurrentem-ployer)inanequationexplaininglog(wage).Theestimatedequationislog(ˆwage).284.092educ.0041exper.022tenure.(3.19)Asinthesimpleregressioncase,thecoefficientshaveapercentageinterpretation.Theonlydifferencehereisthattheyalsohaveaceterisparibusinterpretation.Thecoefficient.09274\nd7/14/994:55PMPage75Chapter3MultipleRegressionAnalysis:Estimationmeansthat,holdingexperandtenurefixed,anotheryearofeducationispredictedtoincreaselog(wage)by.092,whichtranslatesintoanapproximate9.2percent[100(.092)]increaseinwage.Alternatively,ifwetaketwopeoplewiththesamelevelsofexperienceandjobtenure,thecoefficientoneducistheproportionatedifferenceinpredictedwagewhentheireducationlevelsdifferbyoneyear.Thismeasureofthereturntoeducationatleastkeepstwoimportantproductivityfactorsfixed;whetheritisagoodestimateoftheceterisparibusreturntoanotheryearofeducationrequiresustostudythestatisticalprop-ertiesofOLS(seeSection3.3).OntheMeaningof“HoldingOtherFactorsFixed”inMultipleRegressionThepartialeffectinterpretationofslopecoefficientsinmultipleregressionanalysiscancausesomeconfusion,soweattempttopreventthatproblemnow.InExample3.1,weobservedthatthecoefficientonACTmeasuresthepredicteddif-ferenceincolGPA,holdinghsGPAfixed.Thepowerofmultipleregressionanalysisisthatitprovidesthisceterisparibusinterpretationeventhoughthedatahavenotbeencollectedinaceterisparibusfashion.IngivingthecoefficientonACTapartialeffectinterpretation,itmayseemthatweactuallywentoutandsampledpeoplewiththesamehighschoolGPAbutpossiblywithdifferentACTscores.Thisisnotthecase.Thedataarearandomsamplefromalargeuniversity:therewerenorestrictionsplacedonthesamplevaluesofhsGPAorACTinobtainingthedata.Rarelydowehavetheluxuryofholdingcertainvariablesfixedinobtainingoursample.IfwecouldcollectasampleofindividualswiththesamehighschoolGPA,thenwecouldperformasimpleregressionanalysisrelatingcolGPAtoACT.Multipleregressioneffectivelyallowsustomimicthissituationwithoutrestrictingthevaluesofanyindependentvariables.Thepowerofmultipleregressionanalysisisthatitallowsustodoinnonexperi-mentalenvironmentswhatnaturalscientistsareabletodoinacontrolledlaboratoryset-ting:keepotherfactorsfixed.ChangingMorethanOneIndependentVariableSimultaneouslySometimeswewanttochangemorethanoneindependentvariableatthesametimetofindtheresultingeffectonthedependentvariable.Thisiseasilydoneusingequation(3.17).Forexample,inequation(3.19),wecanobtaintheestimatedeffectonwagewhenanindividualstaysatthesamefirmforanotheryear:exper(generalworkforceexperi-ence)andtenurebothincreasebyoneyear.Thetotaleffect(holdingeducfixed)islogˆ(wage).0041exper.022tenure.0041.022.0261,orabout2.6percent.Sinceexperandtenureeachincreasebyoneyear,wejustaddthecoefficientsonexperandtenureandmultiplyby100toturntheeffectintoapercent.OLSFittedValuesandResidualsAfterobtainingtheOLSregressionline(3.11),wecanobtainafittedorpredictedvalueforeachobservation.Forobservationi,thefittedvalueissimply75\nd7/14/994:55PMPage76Part1RegressionAnalysiswithCross-SectionalDatayˆiˆ0ˆ1xi1ˆ2xi2…ˆkxik,(3.20)whichisjustthepredictedvalueobtainedbypluggingthevaluesoftheindependentvariablesforobservationiintoequation(3.11).Weshouldnotforgetabouttheinterceptinobtainingthefittedvalues;otherwise,theanswercanbeverymisleading.AsanQUESTION3.2example,ifin(3.15),hsGPAi3.5andInExample3.1,theOLSfittedlineexplainingcollegeGPAintermsACTi24,colˆGPAi1.29.453(3.5)ofhighschoolGPAandACTscoreis.0094(24)3.101(roundedtothreecolˆGPA1.29.453hsGPA.0094ACT.placesafterthedecimal).Normally,theactualvalueyiforanyIftheaveragehighschoolGPAisabout3.4andtheaverageACTscoreisabout24.2,whatistheaveragecollegeGPAinthesample?observationiwillnotequalthepredictedvalue,yˆi:OLSminimizestheaveragesquaredpredictionerror,whichsaysnothingaboutthepredictionerrorforanyparticu-larobservation.Theresidualforobservationiisdefinedjustasinthesimpleregres-sioncase,uˆiyiyˆi.(3.21)Thereisaresidualforeachobservation.Ifuˆi0,thenyˆiisbelowyi,whichmeansthat,forthisobservation,yiisunderpredicted.Ifuˆi0,thenyiyˆi,andyiisover-predicted.TheOLSfittedvaluesandresidualshavesomeimportantpropertiesthatareimme-diateextensionsfromthesinglevariablecase:1.Thesampleaverageoftheresidualsiszero.2.ThesamplecovariancebetweeneachindependentvariableandtheOLSresidu-alsiszero.Consequently,thesamplecovariancebetweentheOLSfittedvaluesandtheOLSresidualsiszero.3.Thepoint(x¯1,x¯2,…,x¯k,y¯)isalwaysontheOLSregressionline:y¯ˆ0ˆ1x¯1ˆ2x¯2…ˆkx¯k.ThefirsttwopropertiesareimmediateconsequencesofthesetofequationsusedtoobtaintheOLSestimates.Thefirstequationin(3.13)saysthatthesumoftheresidualsniszero.Theremainingequationsareoftheformxijuˆi0,whichimplythattheeachi1independentvariablehaszerosamplecovariancewithuˆi.Property3followsimmedi-atelyfromProperty1.A“PartiallingOut”InterpretationofMultipleRegressionWhenapplyingOLS,wedonotneedtoknowexplicitformulasfortheˆjthatsolvethesystemofequations(3.13).Nevertheless,forcertainderivations,wedoneedexplicitformulasfortheˆj.TheseformulasalsoshedfurtherlightontheworkingsofOLS.Consideragainthecasewithk2independentvariables,yˆˆ0ˆ1x1ˆ2x2.Forconcreteness,wefocusonˆ1.Onewaytoexpressˆ1is76\nd7/14/994:55PMPage77Chapter3MultipleRegressionAnalysis:Estimationnn2ˆ1rˆi1yirˆi1,(3.22)i1i1wheretherˆi1aretheOLSresidualsfromasimpleregressionofx1onx2,usingthesam-pleathand.Weregressourfirstindependentvariable,x1,onoursecondindependentvariable,x2,andthenobtaintheresiduals(yplaysnorolehere).Equation(3.22)showsthatwecanthendoasimpleregressionofyonrˆ1toobtainˆ1.(Notethattheresidu-alsrˆi1haveazerosampleaverage,andsoˆ1istheusualslopeestimatefromsimpleregression.)Therepresentationinequation(3.22)givesanotherdemonstrationofˆ1’spartialeffectinterpretation.Theresidualsrˆi1arethepartofxi1thatisuncorrelatedwithxi2.Anotherwayofsayingthisisthatrˆi1isxi1aftertheeffectsofxi2havebeenpartialledout,ornettedout.Thus,ˆ1measuresthesamplerelationshipbetweenyandx1afterx2hasbeenpartialledout.Insimpleregressionanalysis,thereisnopartiallingoutofothervariablesbecausenoothervariablesareincludedintheregression.Problem3.17stepsyouthroughthepartiallingoutprocessusingthewagedatafromExample3.2.Forpracticalpurposes,theimportantthingisthatˆ1intheequationyˆˆ0ˆ1x1ˆ2x2measuresthechangeinygivenaone-unitincreaseinx1,holdingx2fixed.Inthegeneralmodelwithkexplanatoryvariables,ˆ1canstillbewrittenasinequa-tion(3.22),buttheresidualsrˆi1comefromtheregressionofx1onx2,…,xk.Thus,ˆ1measurestheeffectofx1onyafterx2,…,xkhavebeenpartialledornettedout.ComparisonofSimpleandMultipleRegressionEstimatesTwospecialcasesexistinwhichthesimpleregressionofyonx1willproducethesameOLSestimateonx1astheregressionofyonx1andx2.Tobemoreprecise,writethesimpleregressionofyonx1asy˜˜0˜1x1andwritethemultipleregressionasyˆˆ0ˆ1x1ˆ2x2.Weknowthatthesimpleregressioncoefficient˜1doesnotusu-allyequalthemultipleregressioncoefficientˆ1.Therearetwodistinctcaseswhere˜1andˆ1areidentical:1.Thepartialeffectofx2onyiszerointhesample.Thatis,ˆ20.2.x1andx2areuncorrelatedinthesample.Thefirstassertioncanbeprovenbylookingattwooftheequationsusedtodeterminenˆ0,ˆ1,andˆ2:xi1(yiˆ0ˆ1xi1ˆ2xi2)0andˆ0y¯ˆ1x¯1ˆ2x¯2.Settingi1ˆ20givesthesameinterceptandslopeasdoestheregressionofyonx1.Thesecondassertionfollowsfromequation(3.22).Ifx1andx2areuncorrelatedinthesample,thenregressingx1onx2resultsinnopartiallingout,andsothesimpleregressionofyonx1andthemultipleregressionofyonx1andx2produceidenticalesti-matesonx1.Eventhoughsimpleandmultipleregressionestimatesarealmostneveridentical,wecanusethepreviouscharacterizationstoexplainwhytheymightbeeitherverydif-ferentorquitesimilar.Forexample,ifˆ2issmall,wemightexpectthesimpleandmul-77\nd7/14/994:55PMPage78Part1RegressionAnalysiswithCross-SectionalDatatipleregressionestimatesof1tobesimilar.InExample3.1,thesamplecorrelationbetweenhsGPAandACTisabout0.346,whichisanontrivialcorrelation.Butthecoef-ficientonACTisfairlylittle.ItisnotsuprisingtofindthatthesimpleregressionofcolGPAonhsGPAproducesaslopeestimateof.482,whichisnotmuchdifferentfromtheestimate.453in(3.15).EXAMPLE3.3(Participationin401(k)PensionPlans)Weusethedatain401K.RAWtoestimatetheeffectofaplan’smatchrate(mrate)ontheparticipationrate(prate)inits401(k)pensionplan.Thematchrateistheamountthefirmcontributestoaworker’sfundforeachdollartheworkercontributes(uptosomelimit);thus,mrate.75meansthatthefirmcontributes75centsforeachdollarcontributedbytheworker.Theparticipationrateisthepercentageofeligibleworkershavinga401(k)account.Thevariableageistheageofthe401(k)plan.Thereare1,534plansinthedataset,theaverageprateis87.36,theaveragemrateis.732,andtheaverageageis13.2.Regressingprateonmrate,agegivespraˆte80.125.52mrate.243age.(3.23)Thus,bothmrateandagehavetheexpectedeffects.Whathappensifwedonotcontrolforage?Theestimatedeffectofageisnottrivial,andsowemightexpectalargechangeintheestimatedeffectofmrateifageisdroppedfromtheregression.However,thesimpleregressionofprateonmrateyieldspraˆte83.085.86mrate.Thesimpleregressionesti-mateoftheeffectofmrateonprateisclearlydifferentfromthemultipleregressionesti-mate,butthedifferenceisnotverybig.(Thesimpleregressionestimateisonlyabout6.2percentlargerthanthemultipleregressionestimate.)Thiscanbeexplainedbythefactthatthesamplecorrelationbetweenmrateandageisonly.12.Inthecasewithkindependentvariables,thesimpleregressionofyonx1andthemultipleregressionofyonx1,x2,…,xkproduceanidenticalestimateofx1onlyif(1)theOLScoefficientsonx2throughxkareallzeroor(2)x1isuncorrelatedwitheachofx2,…,xk.Neitheroftheseisverylikelyinpractice.Butifthecoefficientsonx2throughxkaresmall,orthesamplecorrelationsbetweenx1andtheotherindependentvariablesareinsubstantial,thenthesimpleandmultipleregressionestimatesoftheeffectofx1onycanbesimilar.Goodness-of-FitAswithsimpleregression,wecandefinethetotalsumofsquares(SST),theexplainedsumofsquares(SSE),andtheresidualsumofsquaresorsumofsquaredresiduals(SSR),asn2SST(yiy¯)(3.24)i178\nd7/14/994:55PMPage79Chapter3MultipleRegressionAnalysis:Estimationn2SSE(yˆiy¯)(3.25)i1n2SSRuˆi.(3.26)i1Usingthesameargumentasinthesimpleregressioncase,wecanshowthatSSTSSESSR.(3.27)Inotherwords,thetotalvariationin{yi}isthesumofthetotalvariationsin{yˆi}andin{uˆi}.Assumingthatthetotalvariationinyisnonzero,asisthecaseunlessyiisconstantinthesample,wecandivide(3.27)bySSTtogetSSR/SSTSSE/SST1.Justasinthesimpleregressioncase,theR-squaredisdefinedtobe2RSSE/SST1SSR/SST,(3.28)anditisinterpretedastheproportionofthesamplevariationinyithatisexplainedby2theOLSregressionline.Bydefinition,Risanumberbetweenzeroandone.2Rcanalsobeshowntoequalthesquaredcorrelationcoefficientbetweentheactualyiandthefittedvaluesyˆi.Thatis,n2(yiy¯)(yˆiyˆ¯)2i1R(3.29)nn22(yiy¯)(yˆiyˆ¯)i1i1(Wehaveputtheaverageoftheyˆiin(3.29)tobetruetotheformulaforacorrelationcoefficient;weknowthatthisaverageequalsy¯becausethesampleaverageoftheresid-ualsiszeroandyiyˆiuˆi.)2AnimportantfactaboutRisthatitneverdecreases,anditusuallyincreaseswhenanotherindependentvariableisaddedtoaregression.Thisalgebraicfactfollowsbecause,bydefinition,thesumofsquaredresidualsneverincreaseswhenadditionalregressorsareaddedtothemodel.2ThefactthatRneverdecreaseswhenanyvariableisaddedtoaregressionmakesitapoortoolfordecidingwhetheronevariableorseveralvariablesshouldbeaddedtoamodel.Thefactorthatshoulddeterminewhetheranexplanatoryvariablebelongsinamodeliswhethertheexplanatoryvariablehasanonzeropartialeffectonyinthepop-ulation.WewillshowhowtotestthishypothesisinChapter4whenwecoverstatisti-2calinference.Wewillalsoseethat,whenusedproperly,Rallowsustotestagroupofvariablestoseeifitisimportantforexplainingy.Fornow,weuseitasagoodness-of-fitmeasureforagivenmodel.79\nd7/14/994:55PMPage80Part1RegressionAnalysiswithCross-SectionalDataEXAMPLE3.4(DeterminantsofCollegeGPA)2Fromthegradepointaverageregressionthatwedidearlier,theequationwithRiscolˆGPA1.29.453hsGPA.0094ACT2n141,R.176.ThismeansthathsGPAandACTtogetherexplainabout17.6percentofthevariationincol-legeGPAforthissampleofstudents.Thismaynotseemlikeahighpercentage,butwemustrememberthattherearemanyotherfactors—includingfamilybackground,person-ality,qualityofhighschooleducation,affinityforcollege—thatcontributetoastudent’scollegeperformance.IfhsGPAandACTexplainedalmostallofthevariationincolGPA,thenperformanceincollegewouldbepreordainedbyhighschoolperformance!EXAMPLE3.5(ExplainingArrestRecords)CRIME1.RAWcontainsdataonarrestsduringtheyear1986andotherinformationon2,725menbornineither1960or1961inCalifornia.Eachmaninthesamplewasarrest-edatleastoncepriorto1986.Thevariablenarr86isthenumberoftimesthemanwasarrestedduring1986,itiszeroformostmeninthesample(72.29percent),anditvariesfrom0to12.(Thepercentageofthemenarrestedonceduring1986was20.51.)Thevari-ablepcnvistheproportion(notpercentage)ofarrestspriorto1986thatledtoconviction,avgsenisaveragesentencelengthservedforpriorconvictions(zeroformostpeople),ptime86ismonthsspentinprisonin1986,andqemp86isthenumberofquartersduringwhichthemanwasemployedin1986(fromzerotofour).Alinearmodelexplainingarrestsisnarr8601pcnv2avgsen3ptime864qemp86u,wherepcnvisaproxyforthelikelihoodforbeingconvictedofacrimeandavgsenisamea-sureofexpectedseverityofpunishment,ifconvicted.Thevariableptime86capturestheincarcerativeeffectsofcrime:ifanindividualisinprison,hecannotbearrestedforacrimeoutsideofprison.Labormarketopportunitiesarecrudelycapturedbyqemp86.First,weestimatethemodelwithoutthevariableavgsen.Weobtainnarˆr86.712.150pcnv.034ptime86.104qemp862n2,725,R.0413Thisequationsaysthat,asagroup,thethreevariablespcnv,ptime86,andqemp86explainabout4.1percentofthevariationinnarr86.EachoftheOLSslopecoefficientshastheanticipatedsign.Anincreaseinthepropor-tionofconvictionslowersthepredictednumberofarrests.Ifweincreasepcnvby.50(alargeincreaseintheprobabilityofconviction),then,holdingtheotherfactorsfixed,narˆr86.150(.5).075.Thismayseemunusualbecauseanarrestcannotchangebyafraction.Butwecanusethisvaluetoobtainthepredictedchangeinexpectedarrestsforalargegroupofmen.Forexample,among100men,thepredictedfallinarrestswhenpcnvincreasesby.5is7.5.80\nd7/14/994:55PMPage81Chapter3MultipleRegressionAnalysis:EstimationSimilarly,alongerprisontermleadstoalowerpredictednumberofarrests.Infact,ifptime86increasesfrom0to12,predictedarrestsforaparticularmanfallsby.034(12).408.Anotherquarterinwhichlegalemploymentisreportedlowerspredictedarrestsby.104,whichwouldbe10.4arrestsamong100men.2Ifavgsenisaddedtothemodel,weknowthatRwillincrease.Theestimatedequationisnarˆr86.707.151pcnv.0074avgsen.037ptime86.103qemp862n2,725,R.0422.2Thus,addingtheaveragesentencevariableincreasesRfrom.0413to.0422,apracticallysmalleffect.Thesignofthecoefficientonavgsenisalsounexpected:itsaysthatalongeraveragesentencelengthincreasescriminalactivity.Example3.5deservesafinalwordofcaution.Thefactthatthefourexplanatoryvariablesincludedinthesecondregressionexplainonlyabout4.2percentofthevaria-tioninnarr86doesnotnecessarilymeanthattheequationisuseless.Eventhoughthesevariablescollectivelydonotexplainmuchofthevariationinarrests,itisstillpossiblethattheOLSestimatesarereliableestimatesoftheceterisparibuseffectsofeachinde-pendentvariableonnarr86.Aswewillsee,whetherthisisthecasedoesnotdirectly22dependonthesizeofR.Generally,alowRindicatesthatitishardtopredictindivid-ualoutcomesonywithmuchaccuracy,somethingwestudyinmoredetailinChapter26.Inthearrestexample,thesmallRreflectswhatwealreadysuspectinthesocialsci-ences:itisgenerallyverydifficulttopredictindividualbehavior.RegressionThroughtheOriginSometimes,aneconomictheoryorcommonsensesuggeststhat0shouldbezero,andsoweshouldbrieflymentionOLSestimationwhentheinterceptiszero.Specifically,wenowseekanequationoftheformy˜˜1x1˜2x2…˜kxk,(3.30)wherethesymbol“~”overtheestimatesisusedtodistinguishthemfromtheOLSesti-matesobtainedalongwiththeintercept[asin(3.11)].In(3.30),whenx10,x20,…,xk0,thepredictedvalueiszero.Inthiscase,˜1,…,˜karesaidtobetheOLSesti-matesfromtheregressionofyonx1,x2,…,xkthroughtheorigin.TheOLSestimatesin(3.30),asalways,minimizethesumofsquaredresiduals,butwiththeinterceptsetatzero.YoushouldbewarnedthatthepropertiesofOLSthatwederivedearliernolongerholdforregressionthroughtheorigin.Inparticular,the2OLSresidualsnolongerhaveazerosampleaverage.Further,ifRisdefinedasn1SSR/SST,whereSSTisgivenin(3.24)andSSRisnow(yi˜1xi1…i122˜kxik),thenRcanactuallybenegative.Thismeansthatthesampleaverage,y¯,“explains”moreofthevariationintheyithantheexplanatoryvariables.Eitherweshouldincludeaninterceptintheregressionorconcludethattheexplanatoryvariablespoorlyexplainy.InordertoalwayshaveanonnegativeR-squared,someeconomists2prefertocalculateRasthesquaredcorrelationcoefficientbetweentheactualandfit-81\nd7/14/994:55PMPage82Part1RegressionAnalysiswithCross-SectionalDatatedvaluesofy,asin(3.29).(Inthiscase,theaveragefittedvaluemustbecomputeddirectlysinceitnolongerequalsy¯.)However,thereisnosetruleoncomputingR-squaredforregressionthroughtheorigin.Oneseriousdrawbackwithregressionthroughtheoriginisthat,iftheintercept0inthepopulationmodelisdifferentfromzero,thentheOLSestimatorsoftheslopeparameterswillbebiased.Thebiascanbesevereinsomecases.Thecostofestimatinganinterceptwhen0istrulyzeroisthatthevariancesoftheOLSslopeestimatorsarelarger.3.3THEEXPECTEDVALUEOFTHEOLSESTIMATORSWenowturntothestatisticalpropertiesofOLSforestimatingtheparametersinanunderlyingpopulationmodel.Inthissection,wederivetheexpectedvalueoftheOLSestimators.Inparticular,westateanddiscussfourassumptions,whicharedirectexten-sionsofthesimpleregressionmodelassumptions,underwhichtheOLSestimatorsareunbiasedforthepopulationparameters.WealsoexplicitlyobtainthebiasinOLSwhenanimportantvariablehasbeenomittedfromtheregression.Youshouldrememberthatstatisticalpropertieshavenothingtodowithaparticularsample,butratherwiththepropertyofestimatorswhenrandomsamplingisdonerepeatedly.Thus,Sections3.3,3.4,and3.5aresomewhatabstract.Whilewegiveexam-plesofderivingbiasforparticularmodels,itisnotmeaningfultotalkaboutthestatis-ticalpropertiesofasetofestimatesobtainedfromasinglesample.Thefirstassumptionwemakesimplydefinesthemultiplelinearregression(MLR)model.ASSUMPTIONMLR.1(LINEARINPARAMETERS)Themodelinthepopulationcanbewrittenasy01x12x2…kxku,(3.31)where0,1,…,karetheunknownparameters(constants)ofinterest,anduisanunob-servablerandomerrororrandomdisturbanceterm.Equation(3.31)formallystatesthepopulationmodel,sometimescalledthetruemodel,toallowforthepossibilitythatwemightestimateamodelthatdiffersfrom(3.31).Thekeyfeatureisthatthemodelislinearintheparameters0,1,…,k.Asweknow,(3.31)isquiteflexiblebecauseyandtheindependentvariablescanbearbi-traryfunctionsoftheunderlyingvariablesofinterest,suchasnaturallogarithmsandsquares[see,forexample,equation(3.7)].ASSUMPTIONMLR.2(RANDOMSAMPLING)Wehavearandomsampleofnobservations,{(xi1,xi2,…,xik,yi):i1,2,…,n},fromthepop-ulationmodeldescribedby(3.31).82\nd7/14/994:55PMPage83Chapter3MultipleRegressionAnalysis:EstimationSometimesweneedtowritetheequationforaparticularobservationi:foraran-domlydrawnobservationfromthepopulation,wehaveyi01xi12xi2…kxikui.(3.32)Rememberthatireferstotheobservation,andthesecondsubscriptonxisthevariablenumber.Forexample,wecanwriteaCEOsalaryequationforaparticularCEOias2log(salaryi)01log(salesi)2ceoteni3ceoteniui.(3.33)ThetermuicontainstheunobservedfactorsforCEOithataffecthisorhersalary.Forapplications,itisusuallyeasiesttowritethemodelinpopulationform,asin(3.31).Itcontainslessclutterandemphasizesthefactthatweareinterestedinestimatingapop-ulationrelationship.Inlightofmodel(3.31),theOLSestimatorsˆ0,ˆ1,ˆ2,…,ˆkfromtheregressionofyonx1,…,xkarenowconsideredtobeestimatorsof0,1,…,k.Wesaw,inSection3.2,thatOLSchoosestheestimatesforaparticularsamplesothattheresidu-alsaverageouttozeroandthesamplecorrelationbetweeneachindependentvariableandtheresidualsiszero.ForOLStobeunbiased,weneedthepopulationversionofthisconditiontobetrue.ASSUMPTIONMLR.3(ZEROCONDITIONALMEAN)Theerroruhasanexpectedvalueofzero,givenanyvaluesoftheindependentvariables.Inotherwords,E(ux1,x2,…,xk)0.(3.34)OnewaythatAssumptionMLR.3canfailisifthefunctionalrelationshipbetweentheexplainedandexplanatoryvariablesismisspecifiedinequation(3.31):forexample,2ifweforgettoincludethequadratictermincintheconsumptionfunctioncons201inc2incuwhenweestimatethemodel.Anotherfunctionalformmis-specificationoccurswhenweusethelevelofavariablewhenthelogofthevariableiswhatactuallyshowsupinthepopulationmodel,orviceversa.Forexample,ifthetruemodelhaslog(wage)asthedependentvariablebutweusewageasthedependentvariableinourregressionanalysis,thentheestimatorswillbebiased.Intuitively,thisshouldbeprettyclear.WewilldiscusswaysofdetectingfunctionalformmisspecificationinChapter9.Omittinganimportantfactorthatiscorrelatedwithanyofx1,x2,…,xkcausesAssumptionMLR.3tofailalso.Withmultipleregressionanalysis,weareabletoincludemanyfactorsamongtheexplanatoryvariables,andomittedvariablesarelesslikelytobeaprobleminmultipleregressionanalysisthaninsimpleregressionanaly-sis.Nevertheless,inanyapplicationtherearealwaysfactorsthat,duetodatalimitationsorignorance,wewillnotbeabletoinclude.Ifwethinkthesefactorsshouldbecon-trolledforandtheyarecorrelatedwithoneormoreoftheindependentvariables,thenAssumptionMLR.3willbeviolated.Wewillderivethisbiasinsomesimplemodelslater.83\nd7/14/994:55PMPage84Part1RegressionAnalysiswithCross-SectionalDataThereareotherwaysthatucanbecorrelatedwithanexplanatoryvariable.InChapter15,wewilldiscusstheproblemofmeasurementerrorinanexplanatoryvari-able.InChapter16,wecovertheconceptuallymoredifficultprobleminwhichoneormoreoftheexplanatoryvariablesisdeterminedjointlywithy.Wemustpostponeourstudyoftheseproblemsuntilwehaveafirmgraspofmultipleregressionanalysisunderanidealsetofassumptions.WhenAssumptionMLR.3holds,weoftensaywehaveexogenousexplanatoryvariables.Ifxjiscorrelatedwithuforanyreason,thenxjissaidtobeanendogenousexplanatoryvariable.Theterms“exogenous”and“endogenous”originatedinsimul-taneousequationsanalysis(seeChapter16),buttheterm“endogenousexplanatoryvariable”hasevolvedtocoveranycasewhereanexplanatoryvariablemaybecor-relatedwiththeerrorterm.ThefinalassumptionweneedtoshowthatOLSisunbiasedensuresthattheOLSestimatorsareactuallywell-defined.Forsimpleregression,weneededtoassumethatthesingleindependentvariablewasnotconstantinthesample.Thecorrespondingassumptionformultipleregressionanalysisismorecomplicated.ASSUMPTIONMLR.4(NOPERFECTCOLLINEARITY)Inthesample(andthereforeinthepopulation),noneoftheindependentvariablesiscon-stant,andtherearenoexactlinearrelationshipsamongtheindependentvariables.Thenoperfectcollinearityassumptionconcernsonlytheindependentvariables.BeginningstudentsofeconometricstendtoconfuseAssumptionsMLR.4andMLR.3,soweemphasizeherethatMLR.4saysnothingabouttherelationshipbetweenuandtheexplanatoryvariables.AssumptionMLR.4ismorecomplicatedthanitscounterpartforsimpleregressionbecausewemustnowlookatrelationshipsbetweenallindependentvariables.Ifanindependentvariablein(3.31)isanexactlinearcombinationoftheotherindependentvariables,thenwesaythemodelsuffersfromperfectcollinearity,anditcannotbeesti-matedbyOLS.ItisimportanttonotethatAssumptionMLR.4doesallowtheindependentvariablestobecorrelated;theyjustcannotbeperfectlycorrelated.Ifwedidnotallowforanycor-relationamongtheindependentvariables,thenmultipleregressionwouldnotbeveryusefulforeconometricanalysis.Forexample,inthemodelrelatingtestscorestoedu-cationalexpendituresandaveragefamilyincome,avgscore01expend2avgincu,wefullyexpectexpendandavginctobecorrelated:schooldistrictswithhighaveragefamilyincomestendtospendmoreperstudentoneducation.Infact,theprimarymoti-vationforincludingavgincintheequationisthatwesuspectitiscorrelatedwithexpend,andsowewouldliketoholditfixedintheanalysis.AssumptionMLR.4onlyrulesoutperfectcorrelationbetweenexpendandavgincinoursample.Wewouldbeveryunluckytoobtainasamplewhereperstudentexpendituresareperfectlycorre-latedwithaveragefamilyincome.Butsomecorrelation,perhapsasubstantialamount,isexpectedandcertainlyallowed.84\nd7/14/994:55PMPage85Chapter3MultipleRegressionAnalysis:EstimationThesimplestwaythattwoindependentvariablescanbeperfectlycorrelatediswhenonevariableisaconstantmultipleofanother.Thiscanhappenwhenaresearcherinad-vertentlyputsthesamevariablemeasuredindifferentunitsintoaregressionequation.Forexample,inestimatingarelationshipbetweenconsumptionandincome,itmakesnosensetoincludeasindependentvariablesincomemeasuredindollarsaswellasincomemeasuredinthousandsofdollars.Oneoftheseisredundant.Whatsensewoulditmaketoholdincomemeasuredindollarsfixedwhilechangingincomemeasuredinthousandsofdollars?Wealreadyknowthatdifferentnonlinearfunctionsofthesamevariablecanappear2amongtheregressors.Forexample,themodelcons01inc2incudoes2notviolateAssumptionMLR.4:eventhoughx2incisanexactfunctionofx1inc,22incisnotanexactlinearfunctionofinc.Includingincinthemodelisausefulwaytogeneralizefunctionalform,unlikeincludingincomemeasuredindollarsandinthou-sandsofdollars.Commonsensetellsusnottoincludethesameexplanatoryvariablemeasuredindifferentunitsinthesameregressionequation.Therearealsomoresubtlewaysthatoneindependentvariablecanbeamultipleofanother.Supposewewouldliketoestimateanextensionofaconstantelasticityconsumptionfunction.Itmightseemnaturaltospecifyamodelsuchas2log(cons)01log(inc)2log(inc)u,(3.35)2wherex1log(inc)andx2log(inc).Usingthebasicpropertiesofthenaturallog(see2AppendixA),log(inc)2log(inc).Thatis,x22x1,andnaturallythisholdsforallobservationsinthesample.ThisviolatesAssumptionMLR.4.Whatweshoulddo22insteadisinclude[log(inc)],notlog(inc),alongwithlog(inc).Thisisasensibleexten-sionoftheconstantelasticitymodel,andwewillseehowtointerpretsuchmodelsinChapter6.Anotherwaythatindependentvariablescanbeperfectlycollineariswhenoneinde-pendentvariablecanbeexpressedasanexactlinearfunctionoftwoormoreoftheotherindependentvariables.Forexample,supposewewanttoestimatetheeffectofcampaignspendingoncampaignoutcomes.Forsimplicity,assumethateachelectionhastwocandidates.LetvoteAbethepercentofthevoteforCandidateA,letexpendAbecampaignexpendituresbyCandidateA,letexpendBbecampaignexpendituresbyCandidateB,andlettotexpendbetotalcampaignexpenditures;thelatterthreevariablesareallmeasuredindollars.ItmayseemnaturaltospecifythemodelasvoteA01expendA2expendB3totexpendu,(3.36)inordertoisolatetheeffectsofspendingbyeachcandidateandthetotalamountofspending.ButthismodelviolatesAssumptionMLR.4becausex3x1x2bydefini-tion.Tryingtointerpretthisequationinaceterisparibusfashionrevealstheproblem.Theparameterof1inequation(3.36)issupposedtomeasuretheeffectofincreasingexpendituresbyCandidateAbyonedollaronCandidateA’svote,holdingCandidateB’sspendingandtotalspendingfixed.Thisisnonsense,becauseifexpendBandtotex-pendareheldfixed,thenwecannotincreaseexpendA.85\nd7/14/994:55PMPage86Part1RegressionAnalysiswithCross-SectionalDataThesolutiontotheperfectcollinearityin(3.36)issimple:dropanyoneofthethreevariablesfromthemodel.Wewouldprobablydroptotexpend,andthenthecoefficientonexpendAwouldmeasuretheeffectofincreasingexpendituresbyAonthepercent-ageofthevotereceivedbyA,holdingthespendingbyBfixed.ThepriorexamplesshowthatAssumptionMLR.4canfailifwearenotcarefulinspecifyingourmodel.AssumptionMLR.4alsofailsifthesamplesize,n,istoosmallinrelationtothenumberofparametersbeingestimated.InthegeneralregressionQUESTION3.3modelinequation(3.31),therearek1Inthepreviousexample,ifweuseasexplanatoryvariablesexpendA,parameters,andMLR.4failsifnk1.expendB,andshareA,whereshareA100(expendA/totexpend)isIntuitively,thismakessense:toestimatethepercentageshareoftotalcampaignexpendituresmadebyCandidateA,doesthisviolateAssumptionMLR.4?k1parameters,weneedatleastk1observations.Notsurprisingly,itisbettertohaveasmanyobservationsaspossible,somethingwewillseewithourvariancecal-culationsinSection3.4.Ifthemodeliscarefullyspecifiedandnk1,AssumptionMLR.4canfailinrarecasesduetobadluckincollectingthesample.Forexample,inawageequationwitheducationandexperienceasvariables,itispossiblethatwecouldobtainarandomsamplewhereeachindividualhasexactlytwiceasmucheducationasyearsofexperi-ence.ThisscenariowouldcauseAssumptionMLR.4tofail,butitcanbeconsideredveryunlikelyunlesswehaveanextremelysmallsamplesize.Wearenowreadytoshowthat,underthesefourmultipleregressionassumptions,theOLSestimatorsareunbiased.Asinthesimpleregressioncase,theexpectationsareconditionalonthevaluesoftheindependentvariablesinthesample,butwedonotshowthisconditioningexplicitly.THEOREM3.1(UNBIASEDNESSOFOLS)UnderAssumptionsMLR.1throughMLR.4,E(ˆj)j,j0,1,…,k,(3.37)foranyvaluesofthepopulationparameterj.Inotherwords,theOLSestimatorsareunbi-asedestimatorsofthepopulationparameters.Inourpreviousempiricalexamples,AssumptionMLR.4hasbeensatisfied(sincewehavebeenabletocomputetheOLSestimates).Furthermore,forthemostpart,thesamplesarerandomlychosenfromawell-definedpopulation.IfwebelievethatthespecifiedmodelsarecorrectunderthekeyAssumptionMLR.3,thenwecanconcludethatOLSisunbiasedintheseexamples.Sinceweareapproachingthepointwherewecanusemultipleregressioninseriousempiricalwork,itisusefultorememberthemeaningofunbiasedness.Itistempting,inexamplessuchasthewageequationinequation(3.19),tosaysomethinglike“9.2per-centisanunbiasedestimateofthereturntoeducation.”Asweknow,anestimatecan-notbeunbiased:anestimateisafixednumber,obtainedfromaparticularsample,whichusuallyisnotequaltothepopulationparameter.WhenwesaythatOLSisunbi-86\nd7/14/994:55PMPage87Chapter3MultipleRegressionAnalysis:EstimationasedunderAssumptionsMLR.1throughMLR.4,wemeanthattheprocedurebywhichtheOLSestimatesareobtainedisunbiasedwhenweviewtheprocedureasbeingappliedacrossallpossiblerandomsamples.Wehopethatwehaveobtainedasamplethatgivesusanestimateclosetothepopulationvalue,but,unfortunately,thiscannotbeassured.IncludingIrrelevantVariablesinaRegressionModelOneissuethatwecandispensewithfairlyquickyisthatofinclusionofanirrelevantvariableoroverspecifyingthemodelinmultipleregressionanalysis.Thismeansthatone(ormore)oftheindependentvariablesisincludedinthemodeleventhoughithasnopartialeffectonyinthepopulation.(Thatis,itspopulationcoefficientiszero.)Toillustratetheissue,supposewespecifythemodelasy01x12x23x3u,(3.38)andthismodelsatisfiesAssumptionsMLR.1throughMLR.4.However,x3hasnoeffectonyafterx1andx2havebeencontrolledfor,whichmeansthat30.Thevariablex3mayormaynotbecorrelatedwithx1orx2;allthatmattersisthat,oncex1andx2arecontrolledfor,x3hasnoeffectony.Intermsofconditionalexpectations,E(yx1,x2,x3)E(yx1,x2)01x12x2.Becausewedonotknowthat30,weareinclinedtoestimatetheequationincludingx3:yˆˆ0ˆ1x1ˆ2x2ˆ3x3.(3.39)Wehaveincludedtheirrelevantvariable,x3,inourregression.Whatistheeffectofincludingx3in(3.39)whenitscoefficientinthepopulationmodel(3.38)iszero?Intermsoftheunbiasednessofˆ1andˆ2,thereisnoeffect.Thisconclusionrequiresnospecialderivation,asitfollowsimmediatelyfromTheorem3.1.Remember,unbiased-nessmeansE(ˆj)jforanyvalueofj,includingj0.Thus,wecanconcludethatE(ˆ0)0,E(ˆ1)1,E(ˆ2)2,andE(ˆ3)0(foranyvaluesof0,1,and2).Eventhoughˆ3itselfwillneverbeexactlyzero,itsaveragevalueacrossmanyrandomsampleswillbezero.Theconclusionoftheprecedingexampleismuchmoregeneral:includingoneormoreirrelevantvariablesinamultipleregressionmodel,oroverspecifyingthemodel,doesnotaffecttheunbiasednessoftheOLSestimators.Doesthismeanitisharmlesstoincludeirrelevantvariables?No.AswewillseeinSection3.4,includingirrelevantvariablescanhaveundesirableeffectsonthevariancesoftheOLSestimators.OmittedVariableBias:TheSimpleCaseNowsupposethat,ratherthanincludinganirrelevantvariable,weomitavariablethatactuallybelongsinthetrue(orpopulation)model.Thisisoftencalledtheproblemofexcludingarelevantvariableorunderspecifyingthemodel.WeclaimedinChapter2andearlierinthischapterthatthisproblemgenerallycausestheOLSestimatorstobebiased.Itistimetoshowthisexplicitlyand,justasimportantly,toderivethedirectionandsizeofthebias.87\nd7/14/994:55PMPage88Part1RegressionAnalysiswithCross-SectionalDataDerivingthebiascausedbyomittinganimportantvariableisanexampleofmis-specificationanalysis.Webeginwiththecasewherethetruepopulationmodelhastwoexplanatoryvariablesandanerrorterm:y01x12x2u,(3.40)andweassumethatthismodelsatisfiesAssumptionsMLR.1throughMLR.4.Supposethatourprimaryinterestisin1,thepartialeffectofx1ony.Forexample,yishourlywage(orlogofhourlywage),x1iseducation,andx2isameasureofinnateability.Inordertogetanunbiasedestimatorof1,weshouldrunaregressionofyonx1andx2(whichgivesunbiasedestimatorsof0,1,and2).However,duetoourigno-ranceordatainavailability,weestimatethemodelbyexcludingx2.Inotherwords,weperformasimpleregressionofyonx1only,obtainingtheequationy˜˜0˜1x1.(3.41)Weusethesymbol“~”ratherthan“^”toemphasizethat˜1comesfromanunderspec-ifiedmodel.Whenfirstlearningabouttheomittedvariablesproblem,itcanbedifficultforthestudenttodistinguishbetweentheunderlyingtruemodel,(3.40)inthiscase,andthemodelthatweactuallyestimate,whichiscapturedbytheregressionin(3.41).Itmayseemsillytoomitthevariablex2ifitbelongsinthemodel,butoftenwehavenochoice.Forexample,supposethatwageisdeterminedbywage01educ2abilu.(3.42)Sinceabilityisnotobserved,weinsteadestimatethemodelwage01educv,wherev2abilu.Theestimatorof1fromthesimpleregressionofwageoneduciswhatwearecalling˜1.Wederivetheexpectedvalueof˜1conditionalonthesamplevaluesofx1andx2.Derivingthisexpectationisnotdifficultbecause˜1isjusttheOLSslopeestimatorfromasimpleregression,andwehavealreadystudiedthisestimatorextensivelyinChapter2.Thedifferencehereisthatwemustanalyzeitspropertieswhenthesimpleregressionmodelismisspecifiedduetoanomittedvariable.Fromequation(2.49),wecanexpress˜1asn(xi1x¯1)yii1˜1n.(3.43)2(xi1x¯1)i1Thenextstepisthemostimportantone.Since(3.40)isthetruemodel,wewriteyforeachobservationias88\nd7/14/994:55PMPage89Chapter3MultipleRegressionAnalysis:Estimationyi01xi12xi2ui(3.44)(notyi01xi1ui,becausethetruemodelcontainsx2).LetSST1bethedenom-inatorin(3.43).Ifweplug(3.44)inforyiin(3.43),thenumeratorin(3.43)becomesn(xi1x¯1)(01xi12xi2ui)i1nnn21(xi1x¯1)2(xi1x¯1)xi2(xi1x¯1)uii1i1i1nn1SST12(xi1x¯1)xi2(xi1x¯1)ui.(3.45)i1i1Ifwedivide(3.45)bySST1,taketheexpectationconditionalonthevaluesoftheinde-pendentvariables,anduseE(ui)0,weobtainn(xi1x¯1)xi2i1E(˜1)12n.(3.46)2(xi1x¯1)i1Thus,E(˜1)doesnotgenerallyequal1:˜1isbiasedfor1.Theratiomultiplying2in(3.46)hasasimpleinterpretation:itisjusttheslopecoefficientfromtheregressionofx2onx1,usingoursampleontheindependentvari-ables,whichwecanwriteasx˜2˜0˜1x1.(3.47)Becauseweareconditioningonthesamplevaluesofbothindependentvariables,˜1isnotrandomhere.Therefore,wecanwrite(3.46)asE(˜1)12˜1,(3.48)whichimpliesthatthebiasin˜1isE(˜1)12˜1.Thisisoftencalledtheomittedvariablebias.Fromequation(3.48),weseethattherearetwocaseswhere˜1isunbiased.Thefirstisprettyobvious:if20—sothatx2doesnotappearinthetruemodel(3.40)—then˜1isunbiased.WealreadyknowthisfromthesimpleregressionanalysisinChapter2.Thesecondcaseismoreinteresting.If˜10,then˜1isunbiasedfor1,evenif20.Since˜1isthesamplecovariancebetweenx1andx2overthesamplevarianceofx1,˜10if,andonlyif,x1andx2areuncorrelatedinthesample.Thus,wehavetheimpor-tantconclusionthat,ifx1andx2areuncorrelatedinthesample,then˜1isunbiased.Thisisnotsurprising:inSection3.2,weshowedthatthesimpleregressionestimator˜1andthemultipleregressionestimatorˆ1arethesamewhenx1andx2areuncorrelatedinthesample.[Wecanalsoshowthat˜1isunbiasedwithoutconditioningonthexi2if89\nd7/14/994:55PMPage90Part1RegressionAnalysiswithCross-SectionalDataTable3.2SummaryofBiasin˜1Whenx2isOmittedinEstimatingEquation(3.40)Corr(x1,x2)>0Corr(x1,x2)<020positivebiasnegativebias20negativebiaspositivebiasE(x2x1)E(x2);then,forestimating1,leavingx2intheerrortermdoesnotviolatethezeroconditionalmeanassumptionfortheerror,onceweadjusttheintercept.]Whenx1andx2arecorrelated,˜1hasthesamesignasthecorrelationbetweenx1andx2:˜10ifx1andx2arepositivelycorrelatedand˜10ifx1andx2arenegativelycor-related.Thesignofthebiasin˜1dependsonthesignsofboth2and˜1andissum-marizedinTable3.2forthefourpossiblecaseswhenthereisbias.Table3.2warrantscarefulstudy.Forexample,thebiasin˜1ispositiveif20(x2hasapositiveeffectony)andx1andx2arepositivelycorrelated.Thebiasisnegativeif20andx1andx2arenegativelycorrelated.Andsoon.Table3.2summarizesthedirectionofthebias,butthesizeofthebiasisalsoveryimportant.Asmallbiasofeithersignneednotbeacauseforconcern.Forexample,ifthereturntoeducationinthepopulationis8.6percentandthebiasintheOLSestima-toris0.1percent(atenthofonepercentagepoint),thenwewouldnotbeverycon-cerned.Ontheotherhand,abiasontheorderofthreepercentagepointswouldbemuchmoreserious.Thesizeofthebiasisdeterminedbythesizesof2and˜1.Inpractice,since2isanunknownpopulationparameter,wecannotbecertainwhether2ispositiveornegative.Nevertheless,weusuallyhaveaprettygoodideaaboutthedirectionofthepartialeffectofx2ony.Further,eventhoughthesignofthecorrelationbetweenx1andx2cannotbeknownifx2isnotobserved,inmanycaseswecanmakeaneducatedguessaboutwhetherx1andx2arepositivelyornegativelycorrelated.Inthewageequation(3.42),bydefinitionmoreabilityleadstohigherproductivityandthereforehigherwages:20.Also,therearereasonstobelievethateducandabilarepositivelycorrelated:onaverage,individualswithmoreinnateabilitychoosehigherlevelsofeducation.Thus,theOLSestimatesfromthesimpleregressionequa-tionwage01educvareonaveragetoolarge.Thisdoesnotmeanthattheestimateobtainedfromoursampleistoobig.Wecanonlysaythatifwecollectmanyrandomsamplesandobtainthesimpleregressionestimateseachtime,thentheaverageoftheseestimateswillbegreaterthan1.EXAMPLE3.6(HourlyWageEquation)Supposethemodellog(wage)01educ2abilusatisfiesAssumptionsMLR.1throughMLR.4.ThedatasetinWAGE1.RAWdoesnotcontaindataonability,soweesti-mate1fromthesimpleregression90\nd7/14/994:55PMPage91Chapter3MultipleRegressionAnalysis:Estimationlog(ˆwage).584.083educ2n526,R.186.Thisisonlytheresultfromasinglesample,sowecannotsaythat.083isgreaterthan1;thetruereturntoeducationcouldbelowerorhigherthan8.3percent(andwewillneverknowforsure).Nevertheless,weknowthattheaverageoftheestimatesacrossallrandomsampleswouldbetoolarge.Asasecondexample,supposethat,attheelementaryschoollevel,theaveragescoreforstudentsonastandardizedexamisdeterminedbyavgscore01expend2povrateu,whereexpendisexpenditureperstudentandpovrateisthepovertyrateofthechildrenintheschool.Usingschooldistrictdata,weonlyhaveobservationsonthepercentofstudentswithapassinggradeandperstudentexpenditures;wedonothaveinformationonpovertyrates.Thus,weestimate1fromthesimpleregressionofavgscoreonexpend.Wecanagainobtainthelikelybiasin˜1.First,2isprobablynegative:thereisampleevidencethatchildrenlivinginpovertyscorelower,onaverage,onstandardizedtests.Second,theaverageexpenditureperstudentisprobablynegativelycorrelatedwiththepovertyrate:thehigherthepovertyrate,thelowertheaverageper-studentspending,sothatCorr(x1,x2)0.FromTable3.2,˜1willhaveapositivebias.Thisobservationhasimportantimplications.Itcouldbethatthetrueeffectofspendingiszero;thatis,10.However,thesimpleregressionestimateof1willusuallybegreaterthanzero,andthiscouldleadustoconcludethatexpendituresareimportantwhentheyarenot.Whenreadingandperformingempiricalworkineconomics,itisimportanttomas-tertheterminologyassociatedwithbiasedestimators.Inthecontextofomittingavari-ablefrommodel(3.40),ifE(˜1)1,thenwesaythat˜1hasanupwardbias.WhenE(˜1)1,˜1hasadownwardbias.Thesedefinitionsarethesamewhether1ispos-itiveornegative.ThephrasebiasedtowardszeroreferstocaseswhereE(˜1)isclosertozerothan1.Therefore,if1ispositive,then˜1isbiasedtowardszeroifithasadownwardbias.Ontheotherhand,if10,then˜1isbiasedtowardszeroifithasanupwardbias.OmittedVariableBias:MoreGeneralCasesDerivingthesignofomittedvariablebiaswhentherearemultipleregressorsintheesti-matedmodelismoredifficult.WemustrememberthatcorrelationbetweenasingleexplanatoryvariableandtheerrorgenerallyresultsinallOLSestimatorsbeingbiased.Forexample,supposethepopulationmodely01x12x23x3u,(3.49)satisfiesAssumptionsMLR.1throughMLR.4.Butweomitx3andestimatethemodelas91\nd7/14/994:55PMPage92Part1RegressionAnalysiswithCross-SectionalDatay˜˜0˜1x1˜2x2.(3.50)Now,supposethatx2andx3areuncorrelated,butthatx1iscorrelatedwithx3.Inotherwords,x1iscorrelatedwiththeomittedvariable,butx2isnot.Itistemptingtothinkthat,while˜1isprobablybiasedbasedonthederivationintheprevioussubsection,˜2isunbiasedbecausex2isuncorrelatedwithx3.Unfortunately,thisisnotgenerallythecase:both˜1and˜2willnormallybebiased.Theonlyexceptiontothisiswhenx1andx2arealsouncorrelated.Eveninthefairlysimplemodelabove,itisdifficulttoobtainthedirectionofthebiasin˜1and˜2.Thisisbecausex1,x2,andx3canallbepairwisecorrelated.Nevertheless,anapproximationisoftenpracticallyuseful.Ifweassumethatx1andx2areuncorrelated,thenwecanstudythebiasin˜1asifx2wereabsentfromboththepop-ulationandtheestimatedmodels.Infact,whenx1andx2areuncorrelated,itcanbeshownthatn(xi1x¯1)xi3i1E(˜1)13n.2(xi1x¯1)i1Thisisjustlikeequation(3.46),but3replaces2andx3replacesx2.Therefore,thebiasin˜1isobtainedbyreplacing2with3andx2withx3inTable3.2.If30andCorr(x1,x3)0,thebiasin˜1ispositive.Andsoon.Asanexample,supposeweaddexpertothewagemodel:wage01educ2exper3abilu.Ifabilisomittedfromthemodel,theestimatorsofboth1and2arebiased,evenifweassumeexperisuncorrelatedwithabil.Wearemostlyinterestedinthereturntoedu-cation,soitwouldbeniceifwecouldconcludethat˜1hasanupwardordownwardbiasduetoomittedability.Thisconclusionisnotpossiblewithoutfurtherassumptions.Asanapproximation,letussupposethat,inadditiontoexperandabilbeinguncorrelated,educandexperarealsouncorrelated.(Inreality,theyaresomewhatnegativelycorre-lated.)Since30andeducandabilarepositivelycorrelated,˜1wouldhaveanupwardbias,justasifexperwerenotinthemodel.Thereasoningusedinthepreviousexampleisoftenfollowedasaroughguideforobtainingthelikelybiasinestimatorsinmorecomplicatedmodels.Usually,thefocusisontherelationshipbetweenaparticularexplanatoryvariable,sayx1,andthekeyomittedfactor.Strictlyspeaking,ignoringallotherexplanatoryvariablesisavalidprac-ticeonlywheneachoneisuncorrelatedwithx1,butitisstillausefulguide.3.4THEVARIANCEOFTHEOLSESTIMATORSWenowobtainthevarianceoftheOLSestimatorssothat,inadditiontoknowingthecentraltendenciesofˆj,wealsohaveameasureofthespreadinitssamplingdistribu-tion.Beforefindingthevariances,weaddahomoskedasticityassumption,asinChapter2.Wedothisfortworeasons.First,theformulasaresimplifiedbyimposingthecon-92\nd7/14/994:55PMPage93Chapter3MultipleRegressionAnalysis:Estimationstanterrorvarianceassumption.Second,inSection3.5,wewillseethatOLShasanimportantefficiencypropertyifweaddthehomoskedasticityassumption.Inthemultipleregressionframework,homoskedasticityisstatedasfollows:ASSUMPTIONMLR.5(HOMOSKEDASTICITY)2Var(ux1,…,xk).AssumptionMLR.5meansthatthevarianceintheerrorterm,u,conditionalontheexplanatoryvariables,isthesameforallcombinationsofoutcomesoftheexplanatoryvariables.Ifthisassumptionfails,thenthemodelexhibitsheteroskedasticity,justasinthetwo-variablecase.Intheequationwage01educ2exper3tenureu,homoskedasticityrequiresthatthevarianceoftheunobservederrorudoesnotdependonthelevelsofeducation,experience,ortenure.Thatis,2Var(ueduc,exper,tenure).Ifthisvariancechangeswithanyofthethreeexplanatoryvariables,thenheteroskedas-ticityispresent.AssumptionsMLR.1throughMLR.5arecollectivelyknownastheGauss-Markovassumptions(forcross-sectionalregression).Sofar,ourstatementsoftheassumptionsaresuitableonlywhenappliedtocross-sectionalanalysiswithrandomsampling.Aswewillsee,theGauss-Markovassumptionsfortimeseriesanalysis,andforothersitua-tionssuchaspaneldataanalysis,aremoredifficulttostate,althoughtherearemanysimilarities.Inthediscussionthatfollows,wewillusethesymbolxtodenotethesetofallinde-pendentvariables,(x1,…,xk).Thus,inthewageregressionwitheduc,exper,andtenureasindependentvariables,x(educ,exper,tenure).NowwecanwriteAssumptionMLR.3asE(yx)01x12x2…kxk,2andAssumptionMLR.5isthesameasVar(yx).StatingthetwoassumptionsinthiswayclearlyillustrateshowAssumptionMLR.5differsgreatlyfromAssumptionMLR.3.AssumptionMLR.3saysthattheexpectedvalueofy,givenx,islinearintheparameters,butitcertainlydependsonx1,x2,…,xk.AssumptionMLR.5saysthatthevarianceofy,givenx,doesnotdependonthevaluesoftheindependentvariables.Wecannowobtainthevariancesoftheˆj,whereweagainconditiononthesamplevaluesoftheindependentvariables.Theproofisintheappendixtothischapter.THEOREM3.2(SAMPLINGVARIANCESOFTHEOLSSLOPEESTIMATORS)UnderAssumptionsMLR.1throughMLR.5,conditionalonthesamplevaluesoftheinde-pendentvariables,93\nd7/14/994:55PMPage94Part1RegressionAnalysiswithCross-SectionalData2Var(ˆj)2,(3.51)SSTj(1Rj)n22forj1,2,…,k,whereSSTj(xijx¯j)isthetotalsamplevariationinxj,andRjisi1theR-squaredfromregressingxjonallotherindependentvariables(andincludinganintercept).Beforewestudyequation(3.51)inmoredetail,itisimportanttoknowthatalloftheGauss-Markovassumptionsareusedinobtainingthisformula.WhilewedidnotneedthehomoskedasticityassumptiontoconcludethatOLSisunbiased,wedoneedittovalidateequation(3.51).ThesizeofVar(ˆj)ispracticallyimportant.Alargervariancemeansalesspreciseestimator,andthistranslatesintolargerconfidenceintervalsandlessaccuratehypothe-sestests(aswewillseeinChapter4).Inthenextsubsection,wediscusstheelementscomprising(3.51).TheComponentsoftheOLSVariances:Multicollinearity2Equation(3.51)showsthatthevarianceofˆjdependsonthreefactors:,SSTj,and2Rj.Rememberthattheindexjsimplydenotesanyoneoftheindependentvariables(suchaseducationorpovertyrate).WenowconsidereachofthefactorsaffectingVar(ˆj)inturn.22THEERRORVARIANCE,.Fromequation(3.51),alargermeanslargervariancesfortheOLSestimators.Thisisnotatallsurprising:more“noise”intheequation(a2larger)makesitmoredifficulttoestimatethepartialeffectofanyoftheindependentvariablesony,andthisisreflectedinhighervariancesfortheOLSslopeestimators.2Sinceisafeatureofthepopulation,ithasnothingtodowiththesamplesize.Itistheonecomponentof(3.51)thatisunknown.Wewillseelaterhowtoobtainanunbi-2asedestimatorof.Foragivendependentvariabley,thereisreallyonlyonewaytoreducetheerrorvariance,andthatistoaddmoreexplanatoryvariablestotheequation(takesomefac-torsoutoftheerrorterm).Thisisnotalwayspossible,norisitalwaysdesirableforrea-sonsdiscussedlaterinthechapter.THETOTALSAMPLEVARIATIONINxj,SSTj.Fromequation(3.51),thelargerthetotalvariationinxj,thesmallerisVar(ˆj).Thus,everythingelsebeingequal,foresti-matingjweprefertohaveasmuchsamplevariationinxjaspossible.Wealreadydis-coveredthisinthesimpleregressioncaseinChapter2.Whileitisrarelypossibleforustochoosethesamplevaluesoftheindependentvariables,thereisawaytoincreasethesamplevariationineachoftheindependentvariables:increasethesamplesize.Infact,whensamplingrandomlyfromapopulation,SSTjincreaseswithoutboundasthesamplesizegetslargerandlarger.Thisisthecomponentofthevariancethatsystemat-icallydependsonthesamplesize.94\nd7/14/994:55PMPage95Chapter3MultipleRegressionAnalysis:EstimationWhenSSTjissmall,Var(ˆj)cangetverylarge,butasmallSSTjisnotaviolationofAssumptionMLR.4.Technically,asSSTjgoestozero,Var(ˆj)approachesinfinity.Theextremecaseofnosamplevariationinxj,SSTj0,isnotallowedbyAssumptionMLR.4.2THELINEARRELATIONSHIPSAMONGTHEINDEPENDENTVARIABLES,Rj.The2termRjinequation(3.51)isthemostdifficultofthethreecomponentstounderstand.Thistermdoesnotappearinsimpleregressionanalysisbecausethereisonlyoneinde-pendentvariableinsuchcases.ItisimportanttoseethatthisR-squaredisdistinctfrom2theR-squaredintheregressionofyonx1,x2,…,xk:Rjisobtainedfromaregressioninvolvingonlytheindependentvariablesintheoriginalmodel,wherexjplaystheroleofadependentvariable.Considerfirstthek2case:y01x12x2u.ThenVar(ˆ1)222/[SST1(1R1)],whereR1istheR-squaredfromthesimpleregressionofx1onx2(andanintercept,asalways).SincetheR-squaredmeasuresgoodness-of-fit,avalueof2R1closetooneindicatesthatx2explainsmuchofthevariationinx1inthesample.Thismeansthatx1andx2arehighlycorrelated.2AsR1increasestoone,Var(ˆ1)getslargerandlarger.Thus,ahighdegreeoflinearrelationshipbetweenx1andx2canleadtolargevariancesfortheOLSslopeestimators.(Asimilarargumentappliestoˆ2.)SeeFigure3.1fortherelationshipbetweenVar(ˆ1)andtheR-squaredfromtheregressionofx1onx2.2Inthegeneralcase,Rjistheproportionofthetotalvariationinxjthatcanbe2explainedbytheotherindependentvariablesappearingintheequation.Foragiven2andSSTj,thesmallestVar(ˆj)isobtainedwhenRj0,whichhappensif,andonlyif,xjhaszerosamplecorrelationwitheveryotherindependentvariable.Thisisthebestcaseforestimatingj,butitisrarelyencountered.2Theotherextremecase,Rj1,isruledoutbyAssumptionMLR.4,because2Rj1meansthat,inthesample,xjisaperfectlinearcombinationofsomeoftheother2independentvariablesintheregression.AmorerelevantcaseiswhenRjis“close”toone.Fromequation(3.51)andFigure3.1,weseethatthiscancauseVar(ˆj)tobelarge:2Var(ˆj)*asRj*1.High(butnotperfect)correlationbetweentwoormoreoftheindependentvariablesiscalledmulticollinearity.Beforewediscussthemulticollinearityissuefurther,itisimportanttobeveryclear2ononething:acasewhereRjisclosetooneisnotaviolationofAssumptionMLR.4.Sincemulticollinearityviolatesnoneofourassumptions,the“problem”ofmulti-collinearityisnotreallywell-defined.Whenwesaythatmulticollinearityarisesforesti-2matingjwhenRjis“close”toone,weput“close”inquotationmarksbecausethereisnoabsolutenumberthatwecancitetoconcludethatmulticollinearityisaproblem.2Forexample,Rj.9meansthat90percentofthesamplevariationinxjcanbeexplainedbytheotherindependentvariablesintheregressionmodel.Unquestionably,thismeansthatxjhasastronglinearrelationshiptotheotherindependentvariables.ButwhetherthistranslatesintoaVar(ˆj)thatistoolargetobeusefuldependsonthesizes2ofandSSTj.AswewillseeinChapter4,forstatisticalinference,whatultimatelymattersishowbigˆjisinrelationtoitsstandarddeviation.2JustasalargevalueofRjcancauselargeVar(ˆj),socanasmallvalueofSSTj.Therefore,asmallsamplesizecanleadtolargesamplingvariances,too.Worrying95\nd7/14/994:55PMPage96Part1RegressionAnalysiswithCross-SectionalDataFigure3.1Var(ˆ)asafunctionofR2.11Var(ˆ)102R11abouthighdegreesofcorrelationamongtheindependentvariablesinthesampleisreallynodifferentfromworryingaboutasmallsamplesize:bothworktoincreaseVar(ˆj).ThefamousUniversityofWisconsineconometricianArthurGoldberger,react-ingtoeconometricians’obsessionwithmulticollinearity,has[tongue-in-cheek]coinedthetermmicronumerosity,whichhedefinesasthe“problemofsmallsamplesize.”[Foranengagingdiscussionofmulticollinearityandmicronumerosity,seeGoldberger(1991).]Althoughtheproblemofmulticollinearitycannotbeclearlydefined,onethingisclear:everythingelsebeingequal,forestimatingjitisbettertohavelesscorrelationbetweenxjandtheotherindependentvariables.Thisobservationoftenleadstoadis-cussionofhowto“solve”themulticollinearityproblem.Inthesocialsciences,whereweareusuallypassivecollectorsofdata,thereisnogoodwaytoreducevariancesofunbiasedestimatorsotherthantocollectmoredata.Foragivendataset,wecantrydroppingotherindependentvariablesfromthemodelinanefforttoreducemulti-collinearity.Unfortunately,droppingavariablethatbelongsinthepopulationmodelcanleadtobias,aswesawinSection3.3.Perhapsanexampleatthispointwillhelpclarifysomeoftheissuesraisedcon-cerningmulticollinearity.Supposeweareinterestedinestimatingtheeffectofvarious96\nd7/14/994:55PMPage97Chapter3MultipleRegressionAnalysis:Estimationschoolexpenditurecategoriesonstudentperformance.Itislikelythatexpendituresonteachersalaries,instructionalmaterials,athletics,andsoon,arehighlycorrelated:wealthierschoolstendtospendmoreoneverything,andpoorerschoolsspendlessoneverything.Notsurprisingly,itcanbedifficulttoestimatetheeffectofanyparticularexpenditurecategoryonstudentperformancewhenthereislittlevariationinonecate-gorythatcannotlargelybeexplainedbyvariationsintheotherexpenditurecategories2(thisleadstohighRjforeachoftheexpenditurevariables).Suchmulticollinearityproblemscanbemitigatedbycollectingmoredata,butinasensewehaveimposedtheproblemonourselves:weareaskingquestionsthatmaybetoosubtlefortheavailabledatatoanswerwithanyprecision.Wecanprobablydomuchbetterbychangingthescopeoftheanalysisandlumpingallexpenditurecategoriestogether,sincewewouldnolongerbetryingtoestimatethepartialeffectofeachseparatecategory.Anotherimportantpointisthatahighdegreeofcorrelationbetweencertaininde-pendentvariablescanbeirrelevantastohowwellwecanestimateotherparametersinthemodel.Forexample,consideramodelwiththreeindependentvariables:y01x12x23x3u,wherex2andx3arehighlycorrelated.ThenVar(ˆ2)andVar(ˆ3)maybelarge.Buttheamountofcorrelationbetweenx2andx3hasnodirecteffectonVar(ˆ1).Infact,ifx1is22uncorrelatedwithx2andx3,thenR10andVar(ˆ1)/SST1,regardlessofhowmuchcorrelationthereisbetweenx2andx3.If1istheparameterofinterest,wedonotreallycareabouttheamountofcorrelationbetweenx2andx3.QUESTION3.4ThepreviousobservationisimportantSupposeyoupostulateamodelexplainingfinalexamscoreintermsbecauseeconomistsoftenincludemanyofclassattendance.Thus,thedependentvariableisfinalexamcontrolsinordertoisolatethecausaleffectscore,andthekeyexplanatoryvariableisnumberofclassesattend-ed.Tocontrolforstudentabilitiesandeffortsoutsidetheclassroom,ofaparticularvariable.Forexample,inyouincludeamongtheexplanatoryvariablescumulativeGPA,SATlookingattherelationshipbetweenloanscore,andmeasuresofhighschoolperformance.Someonesays,approvalratesandpercentofminoritiesin“Youcannothopetolearnanythingfromthisexercisebecauseaneighborhood,wemightincludevari-cumulativeGPA,SATscore,andhighschoolperformancearelikelyableslikeaverageincome,averagehous-tobehighlycollinear.”Whatshouldbeyourresponse?ingvalue,measuresofcreditworthiness,andsoon,becausethesefactorsneedtobeaccountedforinordertodrawcausalcon-clusionsaboutdiscrimination.Income,housingprices,andcreditworthinessaregener-allyhighlycorrelatedwitheachother.Buthighcorrelationsamongthesevariablesdonotmakeitmoredifficulttodeterminetheeffectsofdiscrimination.VariancesinMisspecifiedModelsThechoiceofwhetherornottoincludeaparticularvariableinaregressionmodelcanbemadebyanalyzingthetradeoffbetweenbiasandvariance.InSection3.3,wederivedthebiasinducedbyleavingoutarelevantvariablewhenthetruemodelcontainstwoexplanatoryvariables.Wecontinuetheanalysisofthismodelbycomparingthevari-ancesoftheOLSestimators.Writethetruepopulationmodel,whichsatisfiestheGauss-Markovassumptions,asy01x12x2u.97\nd7/14/994:55PMPage98Part1RegressionAnalysiswithCross-SectionalDataWeconsidertwoestimatorsof1.Theestimatorˆ1comesfromthemultipleregressionyˆˆ0ˆ1x1ˆ2x2.(3.52)Inotherwords,weincludex2,alongwithx1,intheregressionmodel.Theestimator˜1isobtainedbyomittingx2fromthemodelandrunningasimpleregressionofyonx1:y˜˜0˜1x1.(3.53)When20,equation(3.53)excludesarelevantvariablefromthemodeland,aswesawinSection3.3,thisinducesabiasin˜1unlessx1andx2areuncorrelated.Ontheotherhand,ˆ1isunbiasedfor1foranyvalueof2,including20.Itfollowsthat,ifbiasisusedastheonlycriterion,ˆ1ispreferredto˜1.Theconclusionthatˆ1isalwayspreferredto˜1doesnotcarryoverwhenwebringvarianceintothepicture.Conditioningonthevaluesofx1andx2inthesample,wehave,from(3.51),22Var(ˆ1)/[SST1(1R1)],(3.54)2whereSST1isthetotalvariationinx1,andR1istheR-squaredfromtheregressionofx1onx2.Further,asimplemodificationoftheproofinChapter2fortwo-variableregressionshowsthat2Var(˜1)/SST1.(3.55)Comparing(3.55)to(3.54)showsthatVar(˜1)isalwayssmallerthanVar(ˆ1),unlessx1andx2areuncorrelatedinthesample,inwhichcasethetwoestimators˜1andˆ1arethesame.Assumingthatx1andx2arenotuncorrelated,wecandrawthefollowingconclusions:1.When20,˜1isbiased,ˆ1isunbiased,andVar(˜1)Var(ˆ1).2.When20,˜1andˆ1arebothunbiased,andVar(˜1)Var(ˆ1).Fromthesecondconclusion,itisclearthat˜1ispreferredif20.Intuitively,ifx2doesnothaveapartialeffectony,thenincludingitinthemodelcanonlyexacerbatethemulticollinearityproblem,whichleadstoalessefficientestimatorof1.Ahighervariancefortheestimatorof1isthecostofincludinganirrelevantvariableinamodel.Thecasewhere20ismoredifficult.Leavingx2outofthemodelresultsinabiasedestimatorof1.Traditionally,econometricianshavesuggestedcomparingthelikelysizeofthebiasduetoomittingx2withthereductioninthevariance—summa-2rizedinthesizeofR1—todecidewhetherx2shouldbeincluded.However,when20,therearetwofavorablereasonsforincludingx2inthemodel.Themostimpor-tantoftheseisthatanybiasin˜1doesnotshrinkasthesamplesizegrows;infact,thebiasdoesnotnecessarilyfollowanypattern.Therefore,wecanusefullythinkofthebiasasbeingroughlythesameforanysamplesize.Ontheotherhand,Var(˜1)andVar(ˆ1)bothshrinktozeroasngetslarge,whichmeansthatthemulticollinearityinducedbyaddingx2becomeslessimportantasthesamplesizegrows.Inlargesam-ples,wewouldpreferˆ1.98\nd7/14/994:55PMPage99Chapter3MultipleRegressionAnalysis:EstimationTheotherreasonforfavoringˆ1ismoresubtle.Thevarianceformulain(3.55)isconditionalonthevaluesofxi1andxi2inthesample,whichprovidesthebestscenariofor˜1.When20,thevarianceof˜1conditionalonlyonx1islargerthanthatpre-sentedin(3.55).Intuitively,when20andx2isexcludedfromthemodel,theerrorvarianceincreasesbecausetheerroreffectivelycontainspartofx2.Butformula(3.55)ignorestheerrorvarianceincreasebecauseittreatsbothregressorsasnonrandom.Afulldiscussionofwhichindependentvariablestoconditiononwouldleadustoofarastray.Itissufficienttosaythat(3.55)istoogenerouswhenitcomestomeasuringtheprecisionin˜1.2Estimating:StandardErrorsoftheOLSEstimators2Wenowshowhowtochooseanunbiasedestimatorof,whichthenallowsustoobtainunbiasedestimatorsofVar(ˆj).222SinceE(u),anunbiased“estimator”ofisthesampleaverageofthen-12squarederrors:nui.Unfortunately,thisisnotatrueestimatorbecausewedonoti1observetheui.Nevertheless,recallthattheerrorscanbewrittenasuiyi01xi12xi2…kxik,andsothereasonwedonotobservetheuiisthatwedonotknowthej.WhenwereplaceeachjwithitsOLSestimator,wegettheOLSresiduals:uˆiyiˆ0ˆ1xi1ˆ2xi2…ˆkxik.2Itseemsnaturaltoestimatebyreplacinguiwiththeuˆi.Inthesimpleregressioncase,2wesawthatthisleadstoabiasedestimator.Theunbiasedestimatorofinthegen-eralmultipleregressioncaseisn22ˆuˆi(nk1)SSR(nk1).(3.56)i1Wealreadyencounteredthisestimatorinthek1caseinsimpleregression.Thetermnk1in(3.56)isthedegreesoffreedom(df)forthegeneralOLSproblemwithnobservationsandkindependentvariables.Sincetherearek1para-metersinaregressionmodelwithkindependentvariablesandanintercept,wecanwritedfn(k1)(numberofobservations)(numberofestimatedparameters).(3.57)Thisistheeasiestwaytocomputethedegreesoffreedominaparticularapplication:countthenumberofparameters,includingtheintercept,andsubtractthisamountfromthenumberofobservations.(Intherarecasethataninterceptisnotestimated,thenum-berofparametersdecreasesbyone.)Technically,thedivisionbynk1in(3.56)comesfromthefactthattheex-2pectedvalueofthesumofsquaredresidualsisE(SSR)(nk1).Intuitively,wecanfigureoutwhythedegreesoffreedomadjustmentisnecessarybyreturningtonthefirstorderconditionsfortheOLSestimators.Thesecanbewrittenasuˆi0andi199\nd7/14/994:55PMPage100Part1RegressionAnalysiswithCross-SectionalDatanxijuˆi0,wherej1,2,…,k.Thus,inobtainingtheOLSestimates,k1restric-i1tionsareimposedontheOLSresiduals.Thismeansthat,givenn(k1)oftheresiduals,theremainingk1residualsareknown:thereareonlyn(k1)degreesoffreedomintheresiduals.(Thiscanbecontrastedwiththeerrorsui,whichhavendegreesoffreedominthesample.)Forreference,wesummarizethisdiscussionwithTheorem3.3.Weprovedthisthe-oremforthecaseofsimpleregressionanalysisinChapter2(seeTheorem2.3).(Agen-eralproofthatrequiresmatrixalgebraisprovidedinAppendixE.)THEOREM3.3(UNBIASEDESTIMATIONOF2)22UndertheGauss-MarkovAssumptionsMLR.1throughMLR.5,E(ˆ).2Thepositivesquarerootofˆ,denotedˆ,iscalledthestandarderroroftheregressionorSER.TheSERisanestimatorofthestandarddeviationoftheerrorterm.Thisestimateisusuallyreportedbyregressionpackages,althoughitiscalleddifferentthingsbydifferentpackages.(Inadditiontoser,ˆisalsocalledthestandarderroroftheestimateandtherootmeansquarederror.)Notethatˆcaneitherdecreaseorincreasewhenanotherindependentvariableisaddedtoaregression(foragivensample).Thisisbecause,whileSSRmustfallwhenanotherexplanatoryvariableisadded,thedegreesoffreedomalsofallsbyone.BecauseSSRisinthenumeratoranddfisinthedenominator,wecannottellbeforehandwhicheffectwilldominate.ForconstructingconfidenceintervalsandconductingtestsinChapter4,weneedtoestimatethestandarddeviationofˆj,whichisjustthesquarerootofthevariance:21/2sd(ˆj)/[SSTj(1Rj)].Sinceisunknown,wereplaceitwithitsestimator,ˆ.Thisgivesusthestandarderrorofˆj:21/2se(ˆj)ˆ/[SSTj(1Rj)].(3.58)JustastheOLSestimatescanbeobtainedforanygivensample,socanthestandarderrors.Sincese(ˆj)dependsonˆ,thestandarderrorhasasamplingdistribution,whichwillplayaroleinChapter4.Weshouldemphasizeonethingaboutstandarderrors.Because(3.58)isobtaineddirectlyfromthevarianceformulain(3.51),andbecause(3.51)reliesonthehomoskedasticityAssumptionMLR.5,itfollowsthatthestandarderrorformulain(3.58)isnotavalidestimatorofsd(ˆj)iftheerrorsexhibitheteroskedasticity.Thus,whilethepresenceofheteroskedasticitydoesnotcausebiasintheˆj,itdoesleadtobiasintheusualformulaforVar(ˆj),whichtheninvalidatesthestandarderrors.Thisisimportantbecauseanyregressionpackagecomputes(3.58)asthedefaultstandarderrorforeachcoefficient(withasomewhatdifferentrepresentationfortheintercept).Ifwesuspectheteroskedasticity,thenthe“usual”OLSstandarderrorsareinvalidandsomecorrectiveactionshouldbetaken.WewillseeinChapter8whatmethodsareavailablefordealingwithheteroskedasticity.100\nd7/14/994:55PMPage101Chapter3MultipleRegressionAnalysis:Estimation3.5EFFICIENCYOFOLS:THEGAUSS-MARKOVTHEOREMInthissection,westateanddiscusstheimportantGauss-MarkovTheorem,whichjus-tifiestheuseoftheOLSmethodratherthanusingavarietyofcompetingestimators.WeknowonejustificationforOLSalready:underAssumptionsMLR.1throughMLR.4,OLSisunbiased.However,therearemanyunbiasedestimatorsofthejundertheseassumptions(forexample,seeProblem3.12).Mighttherebeotherunbiasedesti-matorswithvariancessmallerthantheOLSestimators?Ifwelimittheclassofcompetingestimatorsappropriately,thenwecanshowthatOLSisbestwithinthisclass.Specifically,wewillarguethat,underAssumptionsMLR.1throughMLR.5,theOLSestimatorˆjforjisthebestlinearunbiasedesti-mator(BLUE).Inordertostatethetheorem,weneedtounderstandeachcomponentoftheacronym“BLUE.”First,weknowwhatanestimatoris:itisarulethatcanbeappliedtoanysampleofdatatoproduceanestimate.Wealsoknowwhatanunbiasedestimatoris:inthecurrentcontext,anestimator,say˜j,ofjisanunbiasedestimatorofjifE(˜j)jforany0,1,…,k.Whataboutthemeaningoftheterm“linear”?Inthecurrentcontext,anestimator˜jofjislinearif,andonlyif,itcanbeexpressedasalinearfunctionofthedataonthedependentvariable:n˜jwijyi,(3.59)i1whereeachwijcanbeafunctionofthesamplevaluesofalltheindependentvariables.TheOLSestimatorsarelinear,ascanbeseenfromequation(3.22).Finally,howdowedefine“best”?Forthecurrenttheorem,bestisdefinedassmall-estvariance.Giventwounbiasedestimators,itislogicaltoprefertheonewiththesmallestvariance(seeAppendixC).Now,letˆ0,ˆ1,…,ˆkdenotetheOLSestimatorsinthemodel(3.31)underAssumptionsMLR.1throughMLR.5.TheGauss-Markovtheoremsaysthat,foranyestimator˜jwhichislinearandunbiased,Var(ˆj)Var(˜j),andtheinequalityisusu-allystrict.Inotherwords,intheclassoflinearunbiasedestimators,OLShasthesmall-estvariance(underthefiveGauss-Markovassumptions).Actually,thetheoremsaysmorethanthis.Ifwewanttoestimateanylinearfunctionofthej,thenthecorre-spondinglinearcombinationoftheOLSestimatorsachievesthesmallestvarianceamongalllinearunbiasedestimators.Weconcludewithatheorem,whichisproveninAppendix3A.THEOREM3.4(GAUSS-MARKOVTHEOREM)UnderAssumptionsMLR.1throughMLR.5,ˆ0,ˆ1,…,ˆkarethebestlinearunbiasedesti-mators(BLUEs)of0,1,…,k,respectively.ItisbecauseofthistheoremthatAssumptionsMLR.1throughMLR.5areknownastheGauss-Markovassumptions(forcross-sectionalanalysis).101\nd7/14/994:55PMPage102Part1RegressionAnalysiswithCross-SectionalDataTheimportanceoftheGauss-Markovtheoremisthat,whenthestandardsetofassumptionsholds,weneednotlookforalternativeunbiasedestimatorsoftheform(3.59):nonewillbebetterthanOLS.Equivalently,ifwearepresentedwithanesti-matorthatisbothlinearandunbiased,thenweknowthatthevarianceofthisestima-torisatleastaslargeastheOLSvariance;noadditionalcalculationisneededtoshowthis.Forourpurposes,Theorem3.4justifiestheuseofOLStoestimatemultipleregres-sionmodels.IfanyoftheGauss-Markovassumptionsfail,thenthistheoremnolongerholds.Wealreadyknowthatfailureofthezeroconditionalmeanassumption(AssumptionMLR.3)causesOLStobebiased,soTheorem3.4alsofails.Wealsoknowthatheteroskedasticity(failureofAssumptionMLR.5)doesnotcauseOLStobebiased.However,OLSnolongerhasthesmallestvarianceamonglinearunbiasedesti-matorsinthepresenceofheteroskedasticity.InChapter8,weanalyzeanestimatorthatimprovesuponOLSwhenweknowthebrandofheteroskedasticity.SUMMARY1.Themultipleregressionmodelallowsustoeffectivelyholdotherfactorsfixedwhileexaminingtheeffectsofaparticularindependentvariableonthedependentvari-able.Itexplicitlyallowstheindependentvariablestobecorrelated.2.Althoughthemodelislinearinitsparameters,itcanbeusedtomodelnonlinearrelationshipsbyappropriatelychoosingthedependentandindependentvariables.3.Themethodofordinaryleastsquaresiseasilyappliedtothemultipleregressionmodel.Eachslopeestimatemeasuresthepartialeffectofthecorrespondingindepen-dentvariableonthedependentvariable,holdingallotherindependentvariablesfixed.24.Ristheproportionofthesamplevariationinthedependentvariableexplainedbytheindependentvariables,anditservesasagoodness-of-fitmeasure.Itisimportantnot2toputtoomuchweightonthevalueofRwhenevaluatingeconometricmodels.5.UnderthefirstfourGauss-Markovassumptions(MLR.1throughMLR.4),theOLSestimatorsareunbiased.Thisimpliesthatincludinganirrelevantvariableinamodelhasnoeffectontheunbiasednessoftheinterceptandotherslopeestimators.Ontheotherhand,omittingarelevantvariablecausesOLStobebiased.Inmanycircum-stances,thedirectionofthebiascanbedetermined.6.UnderthefiveGauss-Markovassumptions,thevarianceofanOLSslopeestima-222torisgivenbyVar(ˆj)/[SSTj(1Rj)].Astheerrorvarianceincreases,sodoesVar(ˆj),whileVar(ˆj)decreasesasthesamplevariationinxj,SSTj,increases.Theterm2Rjmeasurestheamountofcollinearitybetweenxjandtheotherexplanatoryvariables.2AsRjapproachesone,Var(ˆj)isunbounded.7.AddinganirrelevantvariabletoanequationgenerallyincreasesthevariancesoftheremainingOLSestimatorsbecauseofmulticollinearity.8.UndertheGauss-Markovassumptions(MLR.1throughMLR.5),theOLSestima-torsarebestlinearunbiasedestimators(BLUE).102\nd7/14/994:55PMPage103Chapter3MultipleRegressionAnalysis:EstimationKEYTERMSBestLinearUnbiasedEstimator(BLUE)OmittedVariableBiasBiasedTowardsZeroOLSInterceptEstimateCeterisParibusOLSRegressionLineDegreesofFreedom(df)OLSSlopeEstimateDisturbanceOrdinaryLeastSquaresDownwardBiasOverspecifyingtheModelEndogenousExplanatoryVariablePartialEffectErrorTermPerfectCollinearityExcludingaRelevantVariablePopulationModelExogenousExplanatoryVariablesResidualExplainedSumofSquares(SSE)ResidualSumofSquaresFirstOrderConditionsSampleRegressionFunction(SRF)Gauss-MarkovAssumptionsSlopeParametersGauss-MarkovTheoremStandardDeviationofˆjInclusionofanIrrelevantVariableStandardErrorofˆjInterceptStandardErroroftheRegression(SER)MicronumerositySumofSquaredResiduals(SSR)MisspecificationAnalysisTotalSumofSquares(SST)MulticollinearityTrueModelMultipleLinearRegressionModelUnderspecifyingtheModelMultipleRegressionAnalysisUpwardBiasPROBLEMS3.1UsingthedatainGPA2.RAWon4,137collegestudents,thefollowingequationwasestimatedbyOLS:colˆgpa1.392.0135hsperc.00148sat2n4,137,R.273,wherecolgpaismeasuredonafour-pointscale,hspercisthepercentileinthehighschoolgraduatingclass(definedsothat,forexample,hsperc5meansthetopfivepercentoftheclass),andsatisthecombinedmathandverbalscoresonthestudentachievementtest.(i)Whydoesitmakesenseforthecoefficientonhsperctobenegative?(ii)WhatisthepredictedcollegeGPAwhenhsperc20andsat1050?(iii)Supposethattwohighschoolgraduates,AandB,graduatedinthesamepercentilefromhighschool,butStudentA’sSATscorewas140pointshigher(aboutonestandarddeviationinthesample).Whatisthepre-dicteddifferenceincollegeGPAforthesetwostudents?Isthediffer-encelarge?(iv)Holdinghspercfixed,whatdifferenceinSATscoresleadstoapredict-edcolgpadifferenceof.50,orone-halfofagradepoint?Commentonyouranswer.103\nd7/14/994:55PMPage104Part1RegressionAnalysiswithCross-SectionalData3.2ThedatainWAGE2.RAWonworkingmenwasusedtoestimatethefollowingequation:educˆ10.36.094sibs.131meduc.210feduc2n722,R.214,whereeducisyearsofschooling,sibsisnumberofsiblings,meducismother’syearsofschooling,andfeducisfather’syearsofschooling.(i)Doessibshavetheexpectedeffect?Explain.Holdingmeducandfeducfixed,byhowmuchdoessibshavetoincreasetoreducepredictedyearsofeducationbyoneyear?(Anonintegeranswerisacceptablehere.)(ii)Discusstheinterpretationofthecoefficientonmeduc.(iii)SupposethatManAhasnosiblings,andhismotherandfathereachhave12yearsofeducation.ManBhasnosiblings,andhismotherandfathereachhave16yearsofeducation.WhatisthepredicteddifferenceinyearsofeducationbetweenBandA?3.3ThefollowingmodelisasimplifiedversionofthemultipleregressionmodelusedbyBiddleandHamermesh(1990)tostudythetradeoffbetweentimespentsleepingandworkingandtolookatotherfactorsaffectingsleep:sleep01totwrk2educ3ageu,wheresleepandtotwrk(totalwork)aremeasuredinminutesperweekandeducandagearemeasuredinyears.(SeealsoProblem2.12.)(i)Ifadultstradeoffsleepforwork,whatisthesignof1?(ii)Whatsignsdoyouthink2and3willhave?(iii)UsingthedatainSLEEP75.RAW,theestimatedequationissleˆep3638.25.148totwrk11.13educ2.20age2n706,R.113.Ifsomeoneworksfivemorehoursperweek,byhowmanyminutesissleeppredictedtofall?Isthisalargetradeoff?(iv)Discussthesignandmagnitudeoftheestimatedcoefficientoneduc.(v)Wouldyousaytotwrk,educ,andageexplainmuchofthevariationinsleep?Whatotherfactorsmightaffectthetimespentsleeping?Aretheselikelytobecorrelatedwithtotwrk?3.4Themedianstartingsalaryfornewlawschoolgraduatesisdeterminedbylog(salary)01LSAT2GPA3log(libvol)4log(cost)5ranku,whereLSATismedianLSATscoreforthegraduatingclass,GPAisthemediancollegeGPAfortheclass,libvolisthenumberofvolumesinthelawschoollibrary,costistheannualcostofattendinglawschool,andrankisalawschoolranking(withrank1beingthebest).(i)Explainwhyweexpect50.104\nd7/14/994:55PMPage105Chapter3MultipleRegressionAnalysis:Estimation(ii)Whatsignstoyouexpectfortheotherslopeparameters?Justifyyouranswers.(iii)UsingthedatainLAWSCH85.RAW,theestimatedequationislog(saˆlary)8.34.0047LSAT.248GPA.095log(libvol).038log(cost).0033rank2n136,R.842.WhatisthepredictedceterisparibusdifferenceinsalaryforschoolswithamedianGPAdifferentbyonepoint?(Reportyouranswerasapercent.)(iv)Interpretthecoefficientonthevariablelog(libvol).(v)Wouldyousayitisbettertoattendahigherrankedlawschool?Howmuchisadifferenceinrankingof20worthintermsofpredictedstart-ingsalary?3.5Inastudyrelatingcollegegradepointaveragetotimespentinvariousactivities,youdistributeasurveytoseveralstudents.Thestudentsareaskedhowmanyhourstheyspendeachweekinfouractivities:studying,sleeping,working,andleisure.Anyactiv-ityisputintooneofthefourcategories,sothatforeachstudentthesumofhoursinthefouractivitiesmustbe168.(i)InthemodelGPA01study2sleep3work4leisureu,doesitmakesensetoholdsleep,work,andleisurefixed,whilechang-ingstudy?(ii)ExplainwhythismodelviolatesAssumptionMLR.4.(iii)Howcouldyoureformulatethemodelsothatitsparametershaveause-fulinterpretationanditsatisfiesAssumptionMLR.4?3.6Considerthemultipleregressionmodelcontainingthreeindependentvariables,underAssumptionsMLR.1throughMLR.4:y01x12x23x3u.Youareinterestedinestimatingthesumoftheparametersonx1andx2;callthis112.Showthatˆ1ˆ1ˆ2isanunbiasedestimatorof1.3.7WhichofthefollowingcancauseOLSestimatorstobebiased?(i)Heteroskedasticity.(ii)Omittinganimportantvariable.(iii)Asamplecorrelationcoefficientof.95betweentwoindependentvari-ablesbothincludedinthemodel.3.8Supposethataverageworkerproductivityatmanufacturingfirms(avgprod)dependsontwofactors,averagehoursoftraining(avgtrain)andaverageworkerability(avgabil):avgprod01avgtrain2avgabilu.105\nd7/14/994:55PMPage106Part1RegressionAnalysiswithCross-SectionalDataAssumethatthisequationsatisfiestheGauss-Markovassumptions.Ifgrantshavebeengiventofirmswhoseworkershavelessthanaverageability,sothatavgtrainandavga-bilarenegativelycorrelated,whatisthelikelybiasin˜1obtainedfromthesimpleregressionofavgprodonavgtrain?3.9Thefollowingequationdescribesthemedianhousingpriceinacommunityintermsofamountofpollution(noxfornitrousoxide)andtheaveragenumberofroomsinhousesinthecommunity(rooms):log(price)01log(nox)2roomsu.(i)Whataretheprobablesignsof1and2?Whatistheinterpretationof1?Explain.(ii)Whymightnox[moreprecisely,log(nox)]androomsbenegativelycor-related?Ifthisisthecase,doesthesimpleregressionoflog(price)onlog(nox)produceanupwardordownwardbiasedestimatorof1?(iii)UsingthedatainHPRICE2.RAW,thefollowingequationswereesti-mated:2log(prˆice)11.711.043log(nox),n506,R.264.2log(prˆice)9.23.718log(nox).306rooms,n506,R.514.Istherelationshipbetweenthesimpleandmultipleregressionestimatesoftheelastic-ityofpricewithrespecttonoxwhatyouwouldhavepredicted,givenyouranswerinpart(ii)?Doesthismeanthat.718isdefinitelyclosertothetrueelasticitythan1.043?3.10Supposethatthepopulationmodeldeterminingyisy01x12x23x3u,andthismodelsatisifiestheGauss-Markovassumptions.However,weestimatethemodelthatomitsx3.Let˜0,˜1,and˜2betheOLSestimatorsfromtheregressionofyonx1andx2.Showthattheexpectedvalueof˜1(giventhevaluesoftheindependentvariablesinthesample)isnrˆi1xi3i1E(˜1)13n,2rˆi1i1wheretherˆi1aretheOLSresidualsfromtheregressionofx1onx2.[Hint:Theformulafor˜1comesfromequation(3.22).Plugyi01xi12xi23xi3uiintothisequation.Aftersomealgebra,taketheexpectationtreatingxi3andrˆi1asnonrandom.]3.11ThefollowingequationrepresentstheeffectsoftaxrevenuemixonsubsequentemploymentgrowthforthepopulationofcountiesintheUnitedStates:growth01shareP2shareI3shareSotherfactors,wheregrowthisthepercentagechangeinemploymentfrom1980to1990,sharePistheshareofpropertytaxesintotaltaxrevenue,shareIistheshareofincometaxrevenues,106\nd7/14/994:55PMPage107Chapter3MultipleRegressionAnalysis:EstimationandshareSistheshareofsalestaxrevenues.Allofthesevariablesaremeasuredin1980.Theomittedshare,shareF,includesfeesandmiscellaneoustaxes.Bydefinition,thefoursharesadduptoone.Otherfactorswouldincludeexpendituresoneducation,infrastructure,andsoon(allmeasuredin1980).(i)Whymustweomitoneofthetaxsharevariablesfromtheequation?(ii)Giveacarefulinterpretationof1.3.12(i)Considerthesimpleregressionmodely01xuunderthefirstfour2Gauss-Markovassumptions.Forsomefunctiong(x),forexampleg(x)xorg(x)2log(1x),definezig(xi).Defineaslopeestimatorasnn˜1(ziz¯)yi(ziz¯)xi.i1i1Showthat˜1islinearandunbiased.Remember,becauseE(ux)0,youcantreatbothxiandziasnonrandominyourderivation.(ii)Addthehomoskedasticityassumption,MLR.5.Showthatnn222Var(˜1)(ziz¯)(ziz¯)xi.i1i1(iii)Showdirectlythat,undertheGauss-Markovassumptions,Var(ˆ1)Var(˜1),whereˆ1istheOLSestimator.[Hint:TheCauchy-SchwartzinequalityinAppendixBimpliesthatn2nn-1-12-12n(ziz¯)(xix¯)n(ziz¯)n(xix¯);i1i1i1noticethatwecandropx¯fromthesamplecovariance.]COMPUTEREXERCISES3.13Aproblemofinteresttohealthofficials(andothers)istodeterminetheeffectsofsmokingduringpregnancyoninfanthealth.Onemeasureofinfanthealthisbirthweight;abirthratethatistoolowcanputaninfantatriskforcontractingvariousill-nesses.Sincefactorsotherthancigarettesmokingthataffectbirthweightarelikelytobecorrelatedwithsmoking,weshouldtakethosefactorsintoaccount.Forexample,higherincomegenerallyresultsinaccesstobetterprenatalcare,aswellasbetternutri-tionforthemother.Anequationthatrecognizesthisisbwght01cigs2famincu.(i)Whatisthemostlikelysignfor2?(ii)Doyouthinkcigsandfamincarelikelytobecorrelated?Explainwhythecorrelationmightbepositiveornegative.(iii)Nowestimatetheequationwithandwithoutfaminc,usingthedatainBWGHT.RAW.Reporttheresultsinequationform,includingthesam-plesizeandR-squared.Discussyourresults,focusingonwhether107\nd7/14/994:55PMPage108Part1RegressionAnalysiswithCross-SectionalDataaddingfamincsubstantiallychangestheestimatedeffectofcigsonbwght.3.14UsethedatainHPRICE1.RAWtoestimatethemodelprice01sqrft2bdrmsu,wherepriceisthehousepricemeasuredinthousandsofdollars.(i)Writeouttheresultsinequationform.(ii)Whatistheestimatedincreaseinpriceforahousewithonemorebed-room,holdingsquarefootageconstant?(iii)Whatistheestimatedincreaseinpriceforahousewithanadditionalbedroomthatis140squarefeetinsize?Comparethistoyouranswerinpart(ii).(iv)Whatpercentageofthevariationinpriceisexplainedbysquarefootageandnumberofbedrooms?(v)Thefirsthouseinthesamplehassqrft2,438andbdrms4.FindthepredictedsellingpriceforthishousefromtheOLSregressionline.(vi)Theactualsellingpriceofthefirsthouseinthesamplewas$300,000(soprice300).Findtheresidualforthishouse.Doesitsuggestthatthebuyerunderpaidoroverpaidforthehouse?3.15ThefileCEOSAL2.RAWcontainsdataon177chiefexecutiveofficers,whichcanbeusedtoexaminetheeffectsoffirmperformanceonCEOsalary.(i)Estimateamodelrelatingannualsalarytofirmsalesandmarketvalue.Makethemodeloftheconstantelasticityvarietyforbothindependentvariables.Writetheresultsoutinequationform.(ii)Addprofitstothemodelfrompart(i).Whycanthisvariablenotbeincludedinlogarithmicform?Wouldyousaythatthesefirmperfor-mancevariablesexplainmostofthevariationinCEOsalaries?(iii)Addthevariableceotentothemodelinpart(ii).WhatistheestimatedpercentagereturnforanotheryearofCEOtenure,holdingotherfactorsfixed?(iv)Findthesamplecorrelationcoefficientbetweenthevariableslog(mktval)andprofits.Arethesevariableshighlycorrelated?WhatdoesthissayabouttheOLSestimators?3.16UsethedatainATTEND.RAWforthisexercise.(i)Obtaintheminimum,maximum,andaveragevaluesforthevariablesatndrte,priGPA,andACT.(ii)Estimatethemodelatndrte01priGPA2ACTuandwritetheresultsinequationform.Interprettheintercept.Doesithaveausefulmeaning?(iii)Discusstheestimatedslopecoefficients.Arethereanysurprises?(iv)Whatisthepredictedatndrte,ifpriGPA3.65andACT20?Whatdoyoumakeofthisresult?Arethereanystudentsinthesamplewiththesevaluesoftheexplanatoryvariables?108\nd7/14/994:55PMPage109Chapter3MultipleRegressionAnalysis:Estimation(v)IfStudentAhaspriGPA3.1andACT21andStudentBhaspriGPA2.1andACT26,whatisthepredicteddifferenceintheirattendancerates?3.17ConfirmthepartiallingoutinterpretationoftheOLSestimatesbyexplicitlydoingthepartiallingoutforExample3.2.Thisfirstrequiresregressingeduconexperandtenure,andsavingtheresiduals,rˆ1.Then,regresslog(wage)onrˆ1.Comparethecoeffi-cientonrˆ1withthecoefficientoneducintheregressionoflog(wage)oneduc,exper,andtenure.APPENDIX3A3A.1DerivationoftheFirstOrderConditions,Equations(3.13)Theanalysisisverysimilartothesimpleregressioncase.Wemustcharacterizethesolutionstotheproblemn2min(yib0b1xi1…bkxik).b0,b1,…,bki1Takingthepartialderivativeswithrespecttoeachofthebj(seeAppendixA),evaluat-ingthematthesolutions,andsettingthemequaltozerogivesn2(yiˆ0ˆ1xi1v…ˆkxik)0i1n2xij(yiˆ0ˆ1xi1…ˆkxik)0,j1,…,k.i1Cancellingthe2givesthefirstorderconditionsin(3.13).3A.2DerivationofEquation(3.22)Toderive(3.22),writexi1intermsofitsfittedvalueanditsresidualfromtheregressionofx1ontox2,…,xk:xi1xˆi1rˆi1,i1,…,n.Now,plugthisintothesecondequa-tionin(3.13):n(xˆi1rˆi1)(yiˆ0ˆ1xi1…ˆkxik)0.(3.60)i1BythedefinitionoftheOLSresidualuˆi,sincexˆi1isjustalinearfunctionoftheexplana-ntoryvariablesxi2,…,xik,itfollowsthatxˆi1uˆi0.Therefore,(3.60)canbeexpressedi1asnrˆi1(yiˆ0ˆ1xi1…ˆkxik)0.(3.61)i1109\nd7/14/994:55PMPage110Part1RegressionAnalysiswithCross-SectionalDatanSincetherˆi1aretheresidualsfromregressingx1ontox2,…,xk,xijrˆi10forj2,ni1…,k.Therefore,(3.61)isequivalenttorˆi1(yiˆ1xi1)0.Finally,weusethefactni1thatxˆi1rˆi10,whichmeansthatˆ1solvesi1nrˆi1(yiˆ1rˆi1)0.i1n2Nowstraightforwardalgebragives(3.22),provided,ofcourse,thatrˆi10;thisisi1ensuredbyAssumptionMLR.4.3A.3ProofofTheorem3.1WeproveTheorem3.1forˆ1;theprooffortheotherslopeparametersisvirtuallyiden-tical.(SeeAppendixEforamoresuccinctproofusingmatrices.)UnderAssumptionMLR.4,theOLSestimatorsexist,andwecanwriteˆ1asin(3.22).UnderAssumptionMLR.1,wecanwriteyiasin(3.32);substitutethisforyiin(3.22).Then,usingnnnn2rˆi10,xijrˆi10forallj2,…,k,andxi1rˆi1rˆi1,wehavei1i1i1i1nn2ˆ11rˆi1uirˆi1.(3.62)i1i1Now,underAssumptionsMLR.2andMLR.4,theexpectedvalueofeachui,givenallindependentvariablesinthesample,iszero.Sincetherˆi1arejustfunctionsofthesam-pleindependentvariables,itfollowsthatnn2E(ˆ1X)1rˆi1E(uiX)rˆi1i1i1nn21rˆi10rˆi11,i1i1whereXdenotesthedataonallindependentvariablesandE(ˆ1X)istheexpectedvalueofˆ1,givenxi1,…,xikforalli1,…,n.Thiscompletestheproof.3A.4ProofofTheorem3.2Again,weprovethisforj1.Writeˆ1asinequation(3.62).Now,underMLR.5,2Var(uiX)foralli1,…,n.Underrandomsampling,theuiareindependent,evenconditionalonX,andtherˆi1arenonrandomconditionalonX.Therefore,nn222Var(ˆ1X)rˆi1Var(uiX)rˆi1i1i1nn2n22222rˆi1rˆi1rˆi1.i1i1i1110\nd7/14/994:55PMPage111Chapter3MultipleRegressionAnalysis:Estimationn2Now,sincerˆi1isthesumofsquaredresidualsfromregressingx1ontox2,…,xk,ni122rˆi1SST1(1R1).Thiscompletestheproof.i13A.5ProofofTheorem3.4Weshowthat,foranyotherlinearunbiasedestimator˜1of1,Var(˜1)Var(ˆ1),whereˆ1istheOLSestimator.Thefocusonj1iswithoutlossofgenerality.For˜1asinequation(3.59),wecanpluginforyitoobtainnnnnn˜10wi11wi1xi12wi1xi2…kwi1xikwi1ui.i1i1i1i1i1Now,sincethewi1arefunctionsofthexij,nnnnnE(˜1X)0wi11wi1xi12wi1xi2…kwi1xikwi1E(uiX)i1i1i1i1i1nnnn0wi11wi1xi12wi1xi2…kwi1xiki1i1i1i1becauseE(uiX)0,foralli1,…,nunderMLR.3.Therefore,forE(˜1X)toequal1foranyvaluesoftheparameters,wemusthavennnwi10,wi1xi11,wi1xij0,j2,…,k.(3.63)i1i1i1Now,letrˆi1betheresidualsfromtheregressionofxi1ontoxi2,…,xik.Then,from(3.63),itfollowsthatnwi1rˆi11.(3.64)i1Now,considerthedifferencebetweenVar(˜1X)andVar(ˆ1X)underMLR.1throughMLR.5:nn2222wi1rˆi1.(3.65)i1i12Becauseof(3.64),wecanwritethedifferencein(3.65),without,asnn2n22wi1wi1rˆi1rˆi1.(3.66)i1i1i1But(3.66)issimplyn2(wi1ˆ1rˆi1),(3.67)i1111\nd7/14/994:55PMPage112Part1RegressionAnalysiswithCross-SectionalDatann2whereˆ1wi1rˆi1rˆi1,ascanbeseenbysquaringeachtermin(3.67),i1i1summing,andthencancellingterms.Because(3.67)isjustthesumofsquaredresidu-alsfromthesimpleregressionofwi1ontorˆi1—rememberthatthesampleaverageofrˆi1iszero—(3.67)mustbenonnegative.Thiscompletestheproof.112\n\nd7/14/995:15PMPage113ChapterFourMultipleRegressionAnalysis:Inferencehischaptercontinuesourtreatmentofmultipleregressionanalysis.WenowturnTtotheproblemoftestinghypothesesabouttheparametersinthepopulationregressionmodel.WebeginbyfindingthedistributionsoftheOLSestimatorsundertheaddedassumptionthatthepopulationerrorisnormallydistributed.Sections4.2and4.3coverhypothesistestingaboutindividualparameters,whileSection4.4dis-cusseshowtotestasinglehypothesisinvolvingmorethanoneparameter.WefocusontestingmultiplerestrictionsinSection4.5andpayparticularattentiontodeterminingwhetheragroupofindependentvariablescanbeomittedfromamodel.4.1SAMPLINGDISTRIBUTIONSOFTHEOLSESTIMATORSUptothispoint,wehaveformedasetofassumptionsunderwhichOLSisunbiased,andwehavealsoderivedanddiscussedthebiascausedbyomittedvariables.InSection3.4,weobtainedthevariancesoftheOLSestimatorsundertheGauss-Markovassump-tions.InSection3.5,weshowedthatthisvarianceissmallestamonglinearunbiasedestimators.KnowingtheexpectedvalueandvarianceoftheOLSestimatorsisusefulfordescribingtheprecisionoftheOLSestimators.However,inordertoperformstatisticalinference,weneedtoknowmorethanjustthefirsttwomomentsofˆj;weneedtoknowthefullsamplingdistributionoftheˆj.EvenundertheGauss-Markovassumptions,thedistributionofˆjcanhavevirtuallyanyshape.Whenweconditiononthevaluesoftheindependentvariablesinoursample,itisclearthatthesamplingdistributionsoftheOLSestimatorsdependontheunderlyingdistributionoftheerrors.Tomakethesamplingdistributionsoftheˆjtractable,wenowassumethattheunobservederrorisnormallydistributedinthepopulation.Wecallthisthenormalityassumption.ASSUMPTIONMLR.6(NORMALITY)Thepopulationerroruisindependentoftheexplanatoryvariablesx1,x2,…,xkandisnor-22mallydistributedwithzeromeanandvariance:u~Normal(0,).113\nd7/14/995:15PMPage114Part1RegressionAnalysiswithCross-SectionalDataAssumptionMLR.6ismuchstrongerthananyofourpreviousassumptions.Infact,sinceuisindependentofthexjunderMLR.6,E(ux1,…,xk)E(u)0,andVar(ux1,2…,xk)Var(u).Thus,ifwemakeAssumptionMLR.6,thenwearenecessarilyassumingMLR.3andMLR.5.Toemphasizethatweareassumingmorethanbefore,wewillrefertothethefullsetofassumptionsMLR.1throughMLR.6.Forcross-sectionalregressionapplications,thesixassumptionsMLR.1throughMLR.6arecalledtheclassicallinearmodel(CLM)assumptions.Thus,wewillrefertothemodelunderthesesixassumptionsastheclassicallinearmodel.ItisbesttothinkoftheCLMassumptionsascontainingalloftheGauss-Markovassumptionsplustheassumptionofanormallydistributederrorterm.UndertheCLMassumptions,theOLSestimatorsˆ0,ˆ1,…,ˆkhaveastrongereffi-ciencypropertythantheywouldundertheGauss-Markovassumptions.ItcanbeshownthattheOLSestimatorsaretheminimumvarianceunbiasedestimators,whichmeansthatOLShasthesmallestvarianceamongunbiasedestimators;wenolongerhavetorestrictourcomparisontoestimatorsthatarelinearintheyi.ThispropertyofOLSundertheCLMassumptionsisdiscussedfurtherinAppendixE.AsuccinctwaytosummarizethepopulationassumptionsoftheCLMis2yx~Normal(01x12x2…kxk,),wherexisagainshorthandfor(x1,…,xk).Thus,conditionalonx,yhasanormaldis-tributionwithmeanlinearinx1,…,xkandaconstantvariance.Forasingleindependentvariablex,thissituationisshowninFigure4.1.Theargumentjustifyingthenormaldistributionfortheerrorsusuallyrunssome-thinglikethis:Becauseuisthesumofmanydifferentunobservedfactorsaffectingy,wecaninvokethecentrallimittheorem(seeAppendixC)toconcludethatuhasanapproximatenormaldistribution.Thisargumenthassomemerit,butitisnotwithoutweaknesses.First,thefactorsinucanhaveverydifferentdistributionsinthepopula-tion(forexample,abilityandqualityofschoolingintheerrorinawageequation).Whilethecentrallimittheorem(CLT)canstillholdinsuchcases,thenormalapprox-imationcanbepoordependingonhowmanyfactorsappearinuandhowdifferentaretheirdistributions.AmoreseriousproblemwiththeCLTargumentisthatitassumesthatallunob-servedfactorsaffectyinaseparate,additivefashion.Nothingguaranteesthatthisisso.Ifuisacomplicatedfunctionoftheunobservedfactors,thentheCLTargumentdoesnotreallyapply.Inanyapplication,whethernormalityofucanbeassumedisreallyanempiricalmatter.Forexample,thereisnotheoremthatsayswageconditionaloneduc,exper,andtenureisnormallydistributed.Ifanything,simplereasoningsuggeststhattheoppositeistrue:sincewagecanneverbelessthanzero,itcannot,strictlyspeaking,haveanor-maldistribution.Further,sincethereareminimumwagelaws,somefractionofthepop-ulationearnsexactlytheminimumwage,whichalsoviolatesthenormalityassumption.Nevertheless,asapracticalmatterwecanaskwhethertheconditionalwagedistribu-tionis“close”tobeingnormal.Pastempiricalevidencesuggeststhatnormalityisnotagoodassumptionforwages.Often,usingatransformation,especiallytakingthelog,yieldsadistributionthatisclosertonormal.Forexample,somethinglikelog(price)tendstohaveadistribution114\nd7/14/995:15PMPage115Chapter4MultipleRegressionAnalysis:InferenceFigure4.1Thehomoskedasticnormaldistributionwithasingleexplanatoryvariable.f(ylx)ynormaldistributionsE(yx)x01x1x2x3xthatlooksmorenormalthanthedistributionofprice.Again,thisisanempiricalissue,whichwewilldiscussfurtherinChapter5.TherearesomeexampleswhereMLR.6isclearlyfalse.Wheneverytakesonjustafewvalues,itcannothaveanythingclosetoanormaldistribution.Thedependentvari-ableinExample3.5providesagoodexample.Thevariablenarr86,thenumberoftimesayoungmanwasarrestedin1986,takesonasmallrangeofintegervaluesandiszeroformostmen.Thus,narr86isfarfrombeingnormallydistributed.Whatcanbedoneinthesecases?AswewillseeinChapter5—andthisisimportant—nonnormalityoftheerrorsisnotaseriousproblemwithlargesamplesizes.Fornow,wejustmakethenormalityassumption.NormalityoftheerrortermtranslatesintonormalsamplingdistributionsoftheOLSestimators:THEOREM4.1(NORMALSAMPLINGDISTRIBUTIONS)UndertheCLMassumptionsMLR.1throughMLR.6,conditionalonthesamplevaluesoftheindependentvariables,ˆj~Normal[j,Var(ˆj)],(4.1)115\nd7/14/995:15PMPage116Part1RegressionAnalysiswithCross-SectionalDatawhereVar(ˆj)wasgiveninChapter3[equation(3.51)].Therefore,(ˆjj)/sd(ˆj)~Normal(0,1).Theproofof(4.1)isnotthatdifficult,giventhepropertiesofnormallydistributedran-ndomvariablesinAppendixB.Eachˆjcanbewrittenasˆjjwijui,wherewiji1thrˆij/SSRj,rˆijistheiresidualfromtheregressionofthexjonalltheotherindependentvariables,andSSRjisthesumofsquaredresidualsfromthisregression[seeequation(3.62)].Sincethewijdependonlyontheindependentvariables,theycanbetreatedasnonrandom.Thus,ˆjisjustalinearcombi-nationoftheerrorsinthesample,{ui:iQUESTION4.11,2,…,n}.UnderAssumptionMLR.6Supposethatuisindependentoftheexplanatoryvariables,andit(andtherandomsamplingAssumptiontakesonthevalues2,1,0,1,and2withequalprobabilityofMLR.2),theerrorsareindependent,iden-1/5.DoesthisviolatetheGauss-Markovassumptions?Doesthisvio-2ticallydistributedNormal(0,)randomlatetheCLMassumptions?variables.Animportantfactaboutinde-pendentnormalrandomvariablesisthatalinearcombinationofsuchrandomvariablesisnormallydistributed(seeAppendixB).Thisbasicallycompletestheproof.InSection3.3,weshowedthatE(ˆj)j,andwederivedVar(ˆj)inSection3.4;thereisnoneedtore-derivethesefacts.Thesecondpartofthistheoremfollowsimmediatelyfromthefactthatwhenwestandardizeanormalrandomvariablebydividingitbyitsstandarddeviation,weendupwithastandardnormalrandomvariable.TheconclusionsofTheorem4.1canbestrengthened.Inadditionto(4.1),anylin-earcombinationoftheˆ0,ˆ1,…,ˆkisalsonormallydistributed,andanysubsetoftheˆjhasajointnormaldistribution.Thesefactsunderliethetestingresultsintheremain-derofthischapter.InChapter5,wewillshowthatthenormalityoftheOLSestimatorsisstillapproximatelytrueinlargesamplesevenwithoutnormalityoftheerrors.4.2TESTINGHYPOTHESESABOUTASINGLEPOPULATIONPARAMETER:THEtTESTThissectioncoverstheveryimportanttopicoftestinghypothesesaboutanysinglepara-meterinthepopulationregressionfunction.Thepopulationmodelcanbewrittenasy01x1…kxku,(4.2)andweassumethatitsatisfiestheCLMassumptions.WeknowthatOLSproducesunbiasedestimatorsofthej.Inthissection,westudyhowtotesthypothesesaboutaparticularj.Forafullunderstandingofhypothesistesting,onemustrememberthatthejareunknownfeaturesofthepopulation,andwewillneverknowthemwithcertainty.Nevertheless,wecanhypothesizeaboutthevalueofjandthenusestatisticalinferencetotestourhypothesis.Inordertoconstructhypothesestests,weneedthefollowingresult:116\nd7/14/995:15PMPage117Chapter4MultipleRegressionAnalysis:InferenceTHEOREM4.2(tDISTRIBUTIONFORTHESTANDARDIZEDESTIMATORS)UndertheCLMassumptionsMLR.1throughMLR.6,(ˆjj)/se(ˆj)~tnk1,(4.3)wherek1isthenumberofunknownparametersinthepopulationmodely01x1…kxku(kslopeparametersandtheintercept0).ThisresultdiffersfromTheorem4.1insomenotablerespects.Theorem4.1showedthat,undertheCLMassumptions,(ˆjj)/sd(ˆj)~Normal(0,1).Thetdistributionin(4.3)comesfromthefactthattheconstantinsd(ˆj)hasbeenreplacedwiththeran-domvariableˆ.Theproofthatthisleadstoatdistributionwithnk1degreesoffreedomisnotespeciallyinsightful.Essentially,theproofshowsthat(4.3)canbewrit-tenastheratioofthestandardnormalrandomvariable(ˆjj)/sd(ˆj)overthesquare22rootofˆ/.Theserandomvariablescanbeshowntobeindependent,and(nk2221)ˆ/nk1.Theresultthenfollowsfromthedefinitionofatrandomvariable(seeSectionB.5).Theorem4.2isimportantinthatitallowsustotesthypothesesinvolvingthej.Inmostapplications,ourprimaryinterestliesintestingthenullhypothesisH0:j0,(4.4)wherejcorrespondstoanyofthekindependentvariables.Itisimportanttounderstandwhat(4.4)meansandtobeabletodescribethishypothesisinsimplelanguageforapar-ticularapplication.Sincejmeasuresthepartialeffectofxjon(theexpectedvalueof)y,aftercontrollingforallotherindependentvariables,(4.4)meansthat,oncex1,x2,…,xj1,xj1,…,xkhavebeenaccountedfor,xjhasnoeffectontheexpectedvalueofy.Wecannotstatethenullhypothesisas“xjdoeshaveapartialeffectony”becausethisistrueforanyvalueofjotherthanzero.Classicaltestingissuitedfortestingsimplehypothe-seslike(4.4).Asanexample,considerthewageequationlog(wage)01educ2exper3tenureu.ThenullhypothesisH0:20meansthat,onceeducationandtenurehavebeenaccountedfor,thenumberofyearsintheworkforce(exper)hasnoeffectonhourlywage.Thisisaneconomicallyinterestinghypothesis.Ifitistrue,itimpliesthataper-son’sworkhistorypriortothecurrentemploymentdoesnotaffectwage.If20,thenpriorworkexperiencecontributestoproductivity,andhencetowage.Youprobablyrememberfromyourstatisticscoursetherudimentsofhypothesistestingforthemeanfromanormalpopulation.(ThisisreviewedinAppendixC.)Themechanicsoftesting(4.4)inthemultipleregressioncontextareverysimilar.Thehardpartisobtainingthecoefficientestimates,thestandarderrors,andthecriticalvalues,butmostofthisworkisdoneautomaticallybyeconometricssoftware.Ourjobistolearnhowregressionoutputcanbeusedtotesthypothesesofinterest.Thestatisticweusetotest(4.4)(againstanyalternative)iscalled“the”tstatisticor“the”tratioofˆjandisdefinedas117\nd7/14/995:15PMPage118Part1RegressionAnalysiswithCross-SectionalDatatˆjˆj/se(ˆj).(4.5)Wehaveput“the”inquotationmarksbecause,aswewillseeshortly,amoregeneralformofthetstatisticisneededfortestingotherhypothesesaboutj.Fornow,itisimportanttoknowthat(4.5)issuitableonlyfortesting(4.4).Whenitcausesnoconfu-sion,wewillsometimeswritetinplaceoftˆ.jThetstatisticforˆjissimpletocomputegivenˆjanditsstandarderror.Infact,mostregressionpackagesdothedivisionforyouandreportthetstatisticalongwitheachcoefficientanditsstandarderror.Beforediscussinghowtouse(4.5)formallytotestH0:j0,itisusefultoseewhytˆhasfeaturesthatmakeitreasonableasateststatistictodetectj0.First,sincejse(ˆj)isalwayspositive,tˆjhasthesamesignasˆj:ifˆjispositive,thensoistˆj,andifˆjisnegative,soistˆj.Second,foragivenvalueofse(ˆj),alargervalueofˆjleadstolargervaluesoftˆj.Ifˆjbecomesmorenegative,sodoestˆj.SincewearetestingH0:j0,itisonlynaturaltolookatourunbiasedestimatorofj,ˆj,forguidance.Inanyinterestingapplication,thepointestimateˆjwillneverexactlybezero,whetherornotH0istrue.Thequestionis:Howfarisˆjfromzero?AsamplevalueofˆjveryfarfromzeroprovidesevidenceagainstH0:j0.However,wemustrecognizethatthereisasamplingerrorinourestimateˆj,sothesizeofˆjmustbeweighedagainstitssamplingerror.Sincethethestandarderrorofˆjisanestimateofthestandarddeviationofˆj,tˆjmeasureshowmanyestimatedstandarddeviationsˆjisawayfromzero.Thisispreciselywhatwedointestingwhetherthemeanofapop-ulationiszero,usingthestandardtstatisticfromintroductorystatistics.ValuesoftˆjsufficientlyfarfromzerowillresultinarejectionofH0.Thepreciserejectionruledependsonthealternativehypothesisandthechosensignificancelevelofthetest.Determiningaruleforrejecting(4.4)atagivensignificancelevel—thatis,theprob-abilityofrejectingH0whenitistrue—requiresknowingthesamplingdistributionoftˆjwhenH0istrue.FromTheorem4.2,weknowthistobetnk1.Thisisthekeytheoret-icalresultneededfortesting(4.4).Beforeproceeding,itisimportanttorememberthatwearetestinghypothesesaboutthepopulationparameters.Wearenottestinghypothesesabouttheestimatesfromaparticularsample.Thus,itnevermakessensetostateanullhypothesisas“H0:ˆ10”or,evenworse,as“H0:.2370”whentheestimateofaparameteris.237inthesam-ple.Wearetestingwhethertheunknownpopulationvalue,1,iszero.Sometreatmentsofregressionanalysisdefinethetstatisticastheabsolutevalueof(4.5),sothatthetstatisticisalwayspositive.Thispracticehasthedrawbackofmakingtestingagainstone-sidedalternativesclumsy.Throughoutthistext,thetstatisticalwayshasthesamesignasthecorrespondingOLScoefficientestimate.TestingAgainstOne-SidedAlternativesInordertodeterminearuleforrejectingH0,weneedtodecideontherelevantalter-nativehypothesis.Firstconsideraone-sidedalternativeoftheformH1:j0.(4.6)118\nd7/14/995:15PMPage119Chapter4MultipleRegressionAnalysis:InferenceThismeansthatwedonotcareaboutalternativestoH0oftheformH1:j0;forsomereason,perhapsonthebasisofintrospectionoreconomictheory,wearerulingoutpop-ulationvaluesofjlessthanzero.(Anotherwaytothinkaboutthisisthatthenullhypoth-esisisactuallyH0:j0;ineithercase,thestatistictˆjisusedastheteststatistic.)Howshouldwechoosearejectionrule?WemustfirstdecideonasignificancelevelortheprobabilityofrejectingH0whenitisinfacttrue.Forconcreteness,supposewehavedecidedona5%significancelevel,asthisisthemostpopularchoice.Thus,wearewillingtomistakenlyrejectH0whenitistrue5%ofthetime.Now,whiletˆjhasatdistributionunderH0—sothatithaszeromean—underthealternativej0,theexpectedvalueoftˆispositive.Thus,wearelookingfora“sufficientlylarge”positivejvalueoftˆjinordertorejectH0:j0infavorofH1:j0.NegativevaluesoftˆjprovidenoevidenceinfavorofH1.thThedefinitionof“sufficientlylarge,”witha5%significancelevel,isthe95per-centileinatdistributionwithnk1degreesoffreedom;denotethisbyc.Inotherwords,therejectionruleisthatH0isrejectedinfavorofH1atthe5%signifi-canceleveliftˆc.(4.7)jFigure4.25%rejectionruleforthealternativeH1:j0with28df.Area=.0501.701rejectionregion119\nd7/14/995:15PMPage120Part1RegressionAnalysiswithCross-SectionalDataByourchoiceofthecriticalvaluec,rejectionofH0willoccurfor5%ofallrandomsampleswhenH0istrue.Therejectionrulein(4.7)isanexampleofaone-tailedtest.Inordertoobtainc,weonlyneedthesignificancelevelandthedegreesoffreedom.Forexample,fora5%leveltestandwithnk128degreesoffreedom,thecriticalvalueisc1.701.Iftˆ1.701,thenwefailtorejectH0infavorof(4.6)atthe5%level.Notethataneg-jativevaluefortˆ,nomatterhowlargeinabsolutevalue,leadstoafailureinrejectingjH0infavorof(4.6).(SeeFigure4.2.)Thesameprocedurecanbeusedwithothersignificancelevels.Fora10%leveltestandifdf21,thecriticalvalueisc1.323.Fora1%significancelevelandifdf21,c2.518.AllofthesecriticalvaluesareobtaineddirectlyfromTableG.2.Youshouldnoteapatterninthecriticalvalues:asthesignificancelevelfalls,thecriticalvalueincreases,sothatwerequirealargerandlargervalueoftˆjinordertorejectH0.Thus,ifH0isrejectedat,say,the5%level,thenitisautomaticallyrejectedatthe10%levelaswell.Itmakesnosensetorejectthenullhypothesisat,say,the5%levelandthentoredothetesttodeterminetheoutcomeatthe10%level.Asthedegreesoffreedominthetdistributiongetlarge,thetdistributionapproachesthestandardnormaldistribution.Forexample,whennk1120,the5%criticalvaluefortheone-sidedalternative(4.7)is1.658,comparedwiththestan-dardnormalvalueof1.645.Thesearecloseenoughforpracticalpurposes;fordegreesoffreedomgreaterthan120,onecanusethestandardnormalcriticalvalues.EXAMPLE4.1(HourlyWageEquation)UsingthedatainWAGE1.RAWgivestheestimatedequationlog(wˆage)(.284)(.092)educ(.0041)exper(.022)tenurelog(wˆage)(.104)(.007)educ(.0017)exper(.003)tenure2n526,R.316,wherestandarderrorsappearinparenthesesbelowtheestimatedcoefficients.Wewillfol-lowthisconventionthroughoutthetext.Thisequationcanbeusedtotestwhetherthereturntoexper,controllingforeducandtenure,iszerointhepopulation,againstthealter-nativethatitispositive.WritethisasH0:exper0versusH1:exper0.(Inapplications,indexingaparameterbyitsassociatedvariablenameisanicewaytolabelparameters,sincethenumericalindicesthatweuseinthegeneralmodelarearbitraryandcancauseconfu-sion.)Rememberthatexperdenotestheunknownpopulationparameter.Itisnonsensetowrite“H0:.00410”or“H0:ˆexper0.”Sincewehave522degreesoffreedom,wecanusethestandardnormalcriticalvalues.The5%criticalvalueis1.645,andthe1%criticalvalueis2.326.Thetstatisticforˆexperistˆ.0041/.00172.41,experandsoˆexper,orexper,isstatisticallysignificantevenatthe1%level.Wealsosaythat“ˆexperisstatisticallygreaterthanzeroatthe1%significancelevel.”Theestimatedreturnforanotheryearofexperience,holdingtenureandeducationfixed,isnotlarge.Forexample,addingthreemoreyearsincreaseslog(wage)by3(.0041)120\nd7/14/995:15PMPage121Chapter4MultipleRegressionAnalysis:Inference.0123,sowageisonlyabout1.2%higher.Nevertheless,wehavepersuasivelyshownthatthepartialeffectofexperienceispositiveinthepopulation.Theone-sidedalternativethattheparameterislessthanzero,H1:j0,(4.8)alsoarisesinapplications.Therejectionruleforalternative(4.8)isjustthemirrorimageofthepreviouscase.Now,thecriticalvaluecomesfromthelefttailofthetdistribution.Inpractice,itiseas-iesttothinkoftherejectionruleasQUESTION4.2tˆc,(4.9)jLetcommunityloanapprovalratesbedeterminedbywherecisthecriticalvalueforthealterna-apprate01percmin2avginctiveH1:j0.Forsimplicity,wealways3avgwlth4avgdebtu,assumecispositive,sincethisishowcrit-wherepercministhepercentminorityinthecommunity,avgincisicalvaluesarereportedinttables,andsoaverageincome,avgwlthisaveragewealth,andavgdebtissomethecriticalvaluecisanegativenumber.measureofaveragedebtobligations.HowdoyoustatethenullForexample,ifthesignificancelevelishypothesisthatthereisnodifferenceinloanratesacrossneighbor-5%andthedegreesoffreedomis18,thenhoodsduetoracialandethniccomposition,whenaverageincome,averagewealth,andaveragedebthavebeencontrolledfor?Howc1.734,andsoH0:j0isrejectedindoyoustatethealternativethatthereisdiscriminationagainstfavorofH1:j0atthe5%leveliftˆjminoritiesinloanapprovalrates?1.734.Itisimportanttorememberthat,torejectH0againstthenegativealternative(4.8),wemustgetanegativetstatistic.Apositivetratio,nomatterhowlarge,providesnoevidenceinfavorof(4.8).TherejectionruleisillustratedinFigure4.3.EXAMPLE4.2(StudentPerformanceandSchoolSize)Thereismuchinterestintheeffectofschoolsizeonstudentperformance.(See,forexam-ple,TheNewYorkTimesMagazine,5/28/95.)Oneclaimisthat,everythingelsebeingequal,studentsatsmallerschoolsfarebetterthanthoseatlargerschools.Thishypothesisisassumedtobetrueevenafteraccountingfordifferencesinclasssizesacrossschools.ThefileMEAP93.RAWcontainsdataon408highschoolsinMichiganfortheyear1993.Wecanusethesedatatotestthenullhypothesisthatschoolsizehasnoeffectonstandardizedtestscores,againstthealternativethatsizehasanegativeeffect.PerformanceismeasuredbythepercentageofstudentsreceivingapassingscoreontheMichiganEducationalAssessmentProgram(MEAP)standardizedtenthgrademathtest(math10).Schoolsizeismeasuredbystudentenrollment(enroll).ThenullhypothesisisH0:enroll0,andthealternativeisH1:enroll0.Fornow,wewillcontrolfortwootherfactors,aver-ageannualteachercompensation(totcomp)andthenumberofstaffperonethousandstudents(staff).Teachercompensationisameasureofteacherquality,andstaffsizeisaroughmeasureofhowmuchattentionstudentsreceive.121\nd7/14/995:15PMPage122Part1RegressionAnalysiswithCross-SectionalDataFigure4.35%rejectionruleforthealternativeH1:j0with18df.Area=.050rejection–1.734regionTheestimatedequation,withstandarderrorsinparentheses,ismatˆh10(2.274)(.00046)totcomp(.048)staff(.00020)enrollmatˆh10(6.113)(.00010)totcomp(.040)staff(.00022)enroll2n408,R.0541.Thecoefficientonenroll,.0002,isinaccordancewiththeconjecturethatlargerschoolshamperperformance:higherenrollmentleadstoalowerpercentageofstudentswithapassingtenthgrademathscore.(Thecoefficientsontotcompandstaffalsohavethesignsweexpect.)Thefactthatenrollhasanestimatedcoefficientdifferentfromzerocouldjustbeduetosamplingerror;tobeconvincedofaneffect,weneedtoconductattest.Sincenk14084404,weusethestandardnormalcriticalvalue.Atthe5%level,thecriticalvalueis1.65;thetstatisticonenrollmustbelessthan1.65torejectH0atthe5%level.Thetstatisticonenrollis.0002/.00022.91,whichislargerthan1.65:wefailtorejectH0infavorofH1atthe5%level.Infact,the15%criticalvalueis1.04,andsince.911.04,wefailtorejectH0evenatthe15%level.Weconcludethatenrollisnotstatisticallysignificantatthe15%level.122\nd7/14/995:15PMPage123Chapter4MultipleRegressionAnalysis:InferenceThevariabletotcompisstatisticallysignificantevenatthe1%significancelevelbecauseitststatisticis4.6.Ontheotherhand,thetstatisticforstaffis1.2,andsowecannotrejectH0:staff0againstH1:staff0evenatthe10%significancelevel.(Thecriticalvalueisc1.28fromthestandardnormaldistribution.)Toillustratehowchangingfunctionalformcanaffectourconclusions,wealsoestimatethemodelwithallindependentvariablesinlogarithmicform.Thisallows,forexample,theschoolsizeeffecttodiminishasschoolsizeincreases.Theestimatedequationismatˆh10(207.66)(21.16)log(totcomp)(3.98)log(staff)(1.29)log(enroll)matˆh10(48.70)(4.06)log(totcomp)(4.19)log(staff)(0.69)log(enroll)2n408,R.0654.Thetstatisticonlog(enroll)isabout1.87;sincethisisbelowthe5%criticalvalue1.65,werejectH0:log(enroll)0infavorofH1:log(enroll)0atthe5%level.InChapter2,weencounteredamodelwherethedependentvariableappearedinitsoriginalform(calledlevelform),whiletheindependentvariableappearedinlogform(calledlevel-logmodel).Theinterpretationoftheparametersisthesameinthemultipleregressioncontext,except,ofcourse,thatwecangivetheparametersaceterisparibusinterpretation.Holdingtotcompandstafffixed,wehavematˆh101.29[log(enroll)],sothatmatˆh10(1.29/100)(%enroll).013(%enroll).Onceagain,wehaveusedthefactthatthechangeinlog(enroll),whenmultipliedby100,isapproximatelythepercentagechangeinenroll.Thus,ifenrollmentis10%higherataschool,matˆh10ispredictedtobe1.3percentagepointslower(math10ismeasuredasapercent).Whichmodeldoweprefer:theoneusingthelevelofenrollortheoneusinglog(enroll)?Inthelevel-levelmodel,enrollmentdoesnothaveastatisticallysignificanteffect,butinthelevel-logmodelitdoes.ThistranslatesintoahigherR-squaredforthelevel-logmodel,whichmeansweexplainmoreofthevariationinmath10byusingenrollinlogarithmicform(6.5%to5.4%).Thelevel-logmodelispreferred,asitmorecloselycap-turestherelationshipbetweenmath10andenroll.WewillsaymoreaboutusingR-squaredtochoosefunctionalforminChapter6.Two-SidedAlternativesInapplications,itiscommontotestthenullhypothesisH0:j0againstatwo-sidedalternative,thatis,H1:j0.(4.10)Underthisalternative,xjhasaceterisparibuseffectonywithoutspecifyingwhethertheeffectispositiveornegative.Thisistherelevantalternativewhenthesignofjisnotwell-determinedbytheory(orcommonsense).Evenwhenweknowwhetherjispos-itiveornegativeunderthealternative,atwo-sidedtestisoftenprudent.Ataminimum,123\nd7/14/995:15PMPage124Part1RegressionAnalysiswithCross-SectionalDatausingatwo-sidedalternativepreventsusfromlookingattheestimatedequationandthenbasingthealternativeonwhetherˆjispositiveornegative.Usingtheregressionestimatestohelpusformulatethenulloralternativehypothesesisnotallowedbecauseclassicalstatisticalinferencepresumesthatwestatethenullandalternativeaboutthepopulationbeforelookingatthedata.Forexample,weshouldnotfirstestimatetheequationrelatingmathperformancetoenrollment,notethattheestimatedeffectisneg-ative,andthendecidetherelevantalternativeisH1:enroll0.Whenthealternativeistwo-sided,weareinterestedintheabsolutevalueofthetstatistic.TherejectionruleforH0:j0against(4.10)istˆc,(4.11)jwheredenotesabsolutevalueandcisanappropriatelychosencriticalvalue.Tofindc,weagainspecifyasignificancelevel,say5%.Foratwo-tailedtest,cischosentothmaketheareaineachtailofthetdistributionequal2.5%.Inotherwords,cisthe97.5percentileinthetdistributionwithnk1degreesoffreedom.Whennk125,the5%criticalvalueforatwo-sidedtestisc2.060.Figure4.4providesanillus-trationofthisdistribution.Figure4.45%rejectionruleforthealternativeH1:j0with25df.Area=.025Area=.0250rejection–2.062.06rejectionregionregion124\nd7/14/995:15PMPage125Chapter4MultipleRegressionAnalysis:InferenceWhenaspecificalternativeisnotstated,itisusuallyconsideredtobetwo-sided.Intheremainderofthistext,thedefaultwillbeatwo-sidedalternative,and5%willbethedefaultsignificancelevel.Whencarryingoutempiricaleconometricanalysis,itisalwaysagoodideatobeexplicitaboutthealternativeandthesignificancelevel.IfH0isrejectedinfavorof(4.10)atthe5%level,weusuallysaythat“xjisstatisticallysig-nificant,orstatisticallydifferentfromzero,atthe5%level.”IfH0isnotrejected,wesaythat“xjisstatisticallyinsignificantatthe5%level.”EXAMPLE4.3(DeterminantsofCollegeGPA)WeuseGPA1.RAWtoestimateamodelexplainingcollegeGPA(colGPA),withtheaveragenumberoflecturesmissedperweek(skipped)asanadditionalexplanatoryvariable.TheestimatedmodeliscolˆGPA(1.39)(.412)hsGPA(.015)ACT(.083)skippedcolˆGPA(0.33)(.094)hsGPA(.011)ACT(.026)skipped2n141,R.234.Wecaneasilycomputetstatisticstoseewhichvariablesarestatisticallysignificant,usingatwo-sidedalternativeineachcase.The5%criticalvalueisabout1.96,sincethedegreesoffreedom(1414137)islargeenoughtousethestandardnormalapproximation.The1%criticalvalueisabout2.58.ThetstatisticonhsGPAis4.38,whichissignificantatverysmallsignificancelevels.Thus,wesaythat“hsGPAisstatisticallysignificantatanyconventionalsignificancelevel.”ThetstatisticonACTis1.36,whichisnotstatisticallysignificantatthe10%levelagainstatwo-sidedalternative.ThecoefficientonACTisalsopracticallysmall:a10-pointincreaseinACT,whichislarge,ispredictedtoincreasecolGPAbyonly.15point.Thus,thevariableACTispractically,aswellasstatistically,insignificant.Thecoefficientonskippedhasatstatisticof.083/.0263.19,soskippedisstatisti-callysignificantatthe1%significancelevel(3.192.58).ThiscoefficientmeansthatanotherlecturemissedperweeklowerspredictedcolGPAbyabout.083.Thus,holdinghsGPAandACTfixed,thepredicteddifferenceincolGPAbetweenastudentwhomissesnolecturesperweekandastudentwhomissesfivelecturesperweekisabout.42.Rememberthatthissaysnothingaboutspecificstudents,butpertainstoaveragestudentsacrossthepopulation.Inthisexample,foreachvariableinthemodel,wecouldarguethataone-sidedalter-nativeisappropriate.ThevariableshsGPAandskippedareverysignificantusingatwo-tailedtestandhavethesignsthatweexpect,sothereisnoreasontodoaone-tailedtest.Ontheotherhand,againstaone-sidedalternative(30),ACTissignificantatthe10%levelbutnotatthe5%level.ThisdoesnotchangethefactthatthecoefficientonACTisprettysmall.TestingOtherHypothesesAboutjAlthoughH0:j0isthemostcommonhypothesis,wesometimeswanttotestwhetherjisequaltosomeothergivenconstant.Twocommonexamplesarej1andj1.Generally,ifthenullisstatedas125\nd7/14/995:15PMPage126Part1RegressionAnalysiswithCross-SectionalDataH0:jaj,(4.12)whereajisourhypothesizedvalueofj,thentheappropriatetstatisticist(ˆjaj)/se(ˆj).Asbefore,tmeasureshowmanyestimatedstandarddeviationsˆjisfromthehypothe-sizedvalueofj.Thegeneraltstatisticisusefullywrittenas(estimatehypothesizedvalue)t.(4.13)standarderrorUnder(4.12),thiststatisticisdistributedastnk1fromTheorem4.2.Theusualtsta-tisticisobtainedwhenaj0.Wecanusethegeneraltstatistictotestagainstone-sidedortwo-sidedalternatives.Forexample,ifthenullandalternativehypothesesareH0:j1andH1:j1,thenwefindthecriticalvalueforaone-sidedalternativeexactlyasbefore:thedifferenceisinhowwecomputethetstatistic,notinhowweobtaintheappropriatec.WerejectH0infavorofH1iftc.Inthiscase,wewouldsaythat“ˆjisstatisticallygreaterthanone”attheappropriatesignificancelevel.EXAMPLE4.4(CampusCrimeandEnrollment)Considerasimplemodelrelatingtheannualnumberofcrimesoncollegecampuses(crime)tostudentenrollment(enroll):log(crime)01log(enroll)u.Thisisaconstantelasticitymodel,where1istheelasticityofcrimewithrespecttoenroll-ment.ItisnotmuchusetotestH0:10,asweexpectthetotalnumberofcrimestoincreaseasthesizeofthecampusincreases.Amoreinterestinghypothesistotestwouldbethattheelasticityofcrimewithrespecttoenrollmentisone:H0:11.Thismeansthata1%increaseinenrollmentleadsto,onaverage,a1%increaseincrime.AnoteworthyalternativeisH1:11,whichimpliesthata1%increaseinenrollmentincreasescampuscrimebymorethan1%.If11,then,inarelativesense—notjustanabsolutesense—crimeismoreofaproblemonlargercampuses.Onewaytoseethisistotaketheexpo-nentialoftheequation:crimeexp(0)enroll1exp(u).(SeeAppendixAforpropertiesofthenaturallogarithmandexponentialfunctions.)For00andu0,thisequationisgraphedinFigure4.5for11,11,and11.Wetest11against11usingdataon97collegesanduniversitiesintheUnitedStatesfortheyear1992.ThedatacomefromtheFBI’sUniformCrimeReports,andtheaveragenumberofcampuscrimesinthesampleisabout394,whiletheaverageenroll-mentisabout16,076.Theestimatedequation(withestimatesandstandarderrorsroundedtotwodecimalplaces)is126\nd7/14/995:15PMPage127Chapter4MultipleRegressionAnalysis:InferenceFigure4.5Graphofcrimeenroll1for11,11,and11.crime1>1=111<100enrolllog(ˆcrime)6.631.27log(enroll)(1.03)(0.11)(4.14)2n97,R.585.Theestimatedelasticityofcrimewithrespecttoenroll,1.27,isinthedirectionofthealter-native11.Butisthereenoughevidencetoconcludethat11?Weneedtobecare-fulintestingthishypothesis,especiallybecausethestatisticaloutputofstandardregressionpackagesismuchmorecomplexthanthesimplifiedoutputreportedinequation(4.14).Ourfirstinstinctmightbetoconstruct“the”tstatisticbytakingthecoefficientonlog(enroll)anddividingitbyitsstandarderror,whichisthetstatisticreportedbyaregressionpack-age.ButthisisthewrongstatisticfortestingH0:11.Thecorrecttstatisticisobtainedfrom(4.13):wesubtractthehypothesizedvalue,unity,fromtheestimateanddividetheresultbythestandarderrorofˆ1:t(1.271)/.11.27/.112.45.Theone-sided5%criticalvalueforatdistributionwith97295dfisabout1.66(usingdf120),soweclearlyreject11infavorof11atthe5%level.Infact,the1%criticalvalueisabout2.37,andsowerejectthenullinfavorofthealternativeateventhe1%level.Weshouldkeepinmindthatthisanalysisholdsnootherfactorsconstant,sotheelas-ticityof1.27isnotnecessarilyagoodestimateofceterisparibuseffect.Itcouldbethat127\nd7/14/995:15PMPage128Part1RegressionAnalysiswithCross-SectionalDatalargerenrollmentsarecorrelatedwithotherfactorsthatcausehighercrime:largerschoolsmightbelocatedinhighercrimeareas.Wecouldcontrolforthisbycollectingdataoncrimeratesinthelocalcity.Foratwo-sidedalternative,forexampleH0:j1,H1:j1,westillcom-putethetstatisticasin(4.13):t(ˆj1)/se(ˆj)(noticehowsubtracting1meansadding1).Therejectionruleistheusualoneforatwo-sidedtest:rejectH0iftc,wherecisatwo-tailedcriticalvalue.IfH0isrejected,wesaythat“ˆjisstatisticallydif-ferentfromnegativeone”attheappropriatesignificancelevel.EXAMPLE4.5(HousingPricesandAirPollution)Forasampleof506communitiesintheBostonarea,weestimateamodelrelatingmedianhousingprice(price)inthecommunitytovariouscommunitycharacteristics:noxistheamountofnitrousoxideintheair,inpartspermillion;distisaweighteddistanceofthecommunityfromfiveemploymentcenters,inmiles;roomsistheaveragenumberofroomsinhousesinthecommunity;andstratioistheaveragestudent-teacherratioofschoolsinthecommunity.Thepopulationmodelislog(price)01log(nox)2log(dist)3rooms4stratiou.Thus,1istheelasticityofpricewithrespecttonox.WewishtotestH0:11againstthealternativeH1:11.Thetstatisticfordoingthistestist(ˆ11)/se(ˆ1).UsingthedatainHPRICE2.RAW,theestimatedmodelislog(prˆice)(11.08)(.954)log(nox)(.134)log(dist)(.255)rooms(.052)stratiolog(prˆice)(0.32)(.117)log(nox)(.043)log(dist)(.019)rooms(.006)stratio2n506,R.581.Theslopeestimatesallhavetheanticipatedsigns.Eachcoefficientisstatisticallydifferentfromzeroatverysmallsignificancelevels,includingthecoefficientonlog(nox).Butwedonotwanttotestthat10.ThenullhypothesisofinterestisH0:11,withcorre-spondingtstatistic(.9541)/.117.393.Thereislittleneedtolookinthettableforacriticalvaluewhenthetstatisticisthissmall:theestimatedelasticityisnotstatisticallydif-ferentfrom1evenatverylargesignificancelevels.Controllingforthefactorswehaveincluded,thereislittleevidencethattheelasticityisdifferentfrom1.Computingp-valuesforttestsSofar,wehavetalkedabouthowtotesthypothesesusingaclassicalapproach:afterstatingthealternativehypothesis,wechooseasignificancelevel,whichthendeter-minesacriticalvalue.Oncethecriticalvaluehasbeenidentified,thevalueofthetsta-tisticiscomparedwiththecriticalvalue,andthenulliseitherrejectedornotrejectedatthegivensignificancelevel.128\nd7/14/995:15PMPage129Chapter4MultipleRegressionAnalysis:InferenceEvenafterdecidingontheappropriatealternative,thereisacomponentofarbi-trarinesstotheclassicalapproach,whichresultsfromhavingtochooseasignificancelevelaheadoftime.Differentresearcherspreferdifferentsignificancelevels,depend-ingontheparticularapplication.Thereisno“correct”significancelevel.Committingtoasignificancelevelaheadoftimecanhideusefulinformationabouttheoutcomeofahypothesistest.Forexample,supposethatwewishtotestthenullhypothesisthataparameteriszeroagainstatwo-sidedalternative,andwith40degreesoffreedomweobtainatstatisticequalto1.85.Thenullhypothesisisnotrejectedatthe5%level,sincethetstatisticislessthanthetwo-tailedcriticalvalueofc2.021.Aresearcherwhoseagendaisnottorejectthenullcouldsimplyreportthisoutcomealongwiththeestimate:thenullhypothesisisnotrejectedatthe5%level.Ofcourse,ifthetstatistic,orthecoefficientanditsstandarderror,arereported,thenwecanalsodeterminethatthenullhypothesiswouldberejectedatthe10%level,sincethe10%criticalvalueisc1.684.Ratherthantestingatdifferentsignificancelevels,itismoreinformativetoanswerthefollowingquestion:Giventheobservedvalueofthetstatistic,whatisthesmallestsignificancelevelatwhichthenullhypothesiswouldberejected?Thislevelisknownasthep-valueforthetest(seeAppendixC).Inthepreviousexample,weknowthep-valueisgreaterthan.05,sincethenullisnotrejectedatthe5%level,andweknowthatthep-valueislessthan.10,sincethenullisrejectedatthe10%level.Weobtaintheactualp-valuebycomputingtheprobabilitythatatrandomvariable,with40df,islargerthan1.85inabsolutevalue.Thatis,thep-valueisthesignificancelevelofthetestwhenweusethevalueoftheteststatistic,1.85intheaboveexample,asthecriticalvalueforthetest.Thisp-valueisshowninFigure4.6.Sinceap-valueisaprobability,itsvalueisalwaysbetweenzeroandone.Inordertocomputep-values,weeitherneedextremelydetailedprintedtablesofthetdistri-bution—whichisnotverypractical—oracomputerprogramthatcomputesareasundertheprobabilitydensityfunctionofthetdistribution.Mostmodernregressionpackageshavethiscapability.Somepackagescomputep-valuesroutinelywitheachOLSregression,butonlyforcertainhypotheses.Ifaregressionpackagereportsap-valuealongwiththestandardOLSoutput,itisalmostcertainlythep-valuefortest-ingthenullhypothesisH0:j0againstthetwo-sidedalternative.Thep-valueinthiscaseisP(Tt),(4.15)where,forclarity,weletTdenoteatdistributedrandomvariablewithnk1degreesoffreedomandlettdenotethenumericalvalueoftheteststatistic.Thep-valuenicelysummarizesthestrengthorweaknessoftheempiricalevidenceagainstthenullhypothesis.Perhapsitsmostusefulinterpretationisthefollowing:thep-valueistheprobabilityofobservingatstatisticasextremeaswedidifthenullhypothesisistrue.Thismeansthatsmallp-valuesareevidenceagainstthenull;largep-valuesprovidelittleevidenceagainstH0.Forexample,ifthep-value.50(reportedalwaysasadecimal,notapercent),thenwewouldobserveavalueofthetstatisticasextremeaswedidin50%ofallrandomsampleswhenthenullhypothesisistrue;thisisprettyweakevidenceagainstH0.129\nd7/14/995:15PMPage130Part1RegressionAnalysiswithCross-SectionalDataFigure4.6Obtainingthep-valueagainstatwo-sidedalternative,whent1.85anddf40.area=.9282area=.0359area=.0359–1.8501.85Intheexamplewithdf40andt1.85,thep-valueiscomputedasp-valueP(T1.85)2P(T1.85)2(.0359).0718,whereP(T1.85)istheareatotherightof1.85inatdistributionwith40df.(ThisvaluewascomputedusingtheeconometricspackageStata;itisnotavailableinTableG.2.)Thismeansthat,ifthenullhypothesisistrue,wewouldobserveanabsolutevalueofthetstatisticaslargeas1.85about7.2%ofthetime.Thisprovidessomeevidenceagainstthenullhypothesis,butwewouldnotrejectthenullatthe5%significancelevel.Thepreviousexampleillustratesthatoncethep-valuehasbeencomputed,aclassi-caltestcanbecarriedoutatanydesiredlevel.Ifdenotesthesignificancelevelofthetest(indecimalform),thenH0isrejectedifp-value;otherwiseH0isnotrejectedatthe100%level.Computingp-valuesforone-sidedalternativesisalsoquitesimple.Suppose,forexample,thatwetestH0:j0againstH1:j0.Ifˆj0,thencomputingap-valueisnotimportant:weknowthatthep-valueisgreaterthan.50,whichwillnevercauseustorejectH0infavorofH1.Ifˆj0,thent0andthep-valueisjusttheprobabil-itythatatrandomvariablewiththeappropriatedfexceedsthevaluet.Someregressionpackagesonlycomputep-valuesfortwo-sidedalternatives.Butitissimpletoobtaintheone-sidedp-value:justdividethetwo-sidedp-valueby2.130\nd7/14/995:15PMPage131Chapter4MultipleRegressionAnalysis:InferenceIfthealternativeisH1:j0,itmakessensetocomputeap-valueifˆj0(andhencet0):p-valueP(Tt)P(Tt)becausethetdistributionissymmetricaboutzero.Again,thiscanbeobtainedasone-halfofthep-valueforthetwo-tailedtest.BecauseyouwillquicklybecomefamiliarwiththemagnitudesoftstatisticsQUESTION4.3thatleadtostatisticalsignificance,espe-Supposeyouestimatearegressionmodelandobtainˆ1.56andciallyforlargesamplesizes,itisnotp-value.086fortestingH0:10againstH1:10.Whatisthealwayscrucialtoreportp-valuesfortsta-p-valuefortestingH0:10againstH1:10?tistics.Butitdoesnothurttoreportthem.Further,whenwediscussFtestinginSection4.5,wewillseethatitisimportanttocomputep-values,becausecriticalvaluesforFtestsarenotsoeasilymemorized.AReminderontheLanguageofClassicalHypothesisTestingWhenH0isnotrejected,weprefertousethelanguage“wefailtorejectH0atthex%level,”ratherthan“H0isacceptedatthex%level.”WecanuseExample4.5toillustratewhytheformerstatementispreferred.Inthisexample,theestimatedelasticityofpricewithrespecttonoxis.954,andthetstatisticfortestingH0:nox1ist.393;therefore,wecannotrejectH0.Buttherearemanyothervaluesfornox(morethanwecancount)thatcannotberejected.Forexample,thetstatisticforH0:nox.9is(.954.9)/.117.462,andsothisnullisnotrejectedeither.Clearlynox1andnox.9cannotbothbetrue,soitmakesnosensetosaythatwe“accept”eitherofthesehypotheses.Allwecansayisthatthedatadonotallowustorejecteitherofthesehypothesesatthe5%significancelevel.Economic,orPractical,versusStatisticalSignificanceSincewehaveemphasizedstatisticalsignificancethroughoutthissection,nowisagoodtimetorememberthatweshouldpayattentiontothemagnitudeofthecoefficientestimatesinadditiontothesizeofthetstatistics.Thestatisticalsignificanceofavari-ablexjisdeterminedentirelybythesizeoftˆ,whereastheeconomicsignificanceorjpracticalsignificanceofavariableisrelatedtothesize(andsign)ofˆj.RecallthatthetstatisticfortestingH0:j0isdefinedbydividingtheestimatebyitsstandarderror:tˆjˆj/se(ˆj).Thus,tˆjcanindicatestatisticalsignificanceeitherbecauseˆjis“large”orbecausese(ˆj)is“small.”Itisimportantinpracticetodistin-guishbetweenthesereasonsforstatisticallysignificanttstatistics.Toomuchfocusonstatisticalsignificancecanleadtothefalseconclusionthatavariableis“important”forexplainingyeventhoughitsestimatedeffectismodest.EXAMPLE4.6[ParticipationRatesin401(k)Plans]InExample3.3,weusedthedataon401(k)planstoestimateamodeldescribingparticipa-tionratesintermsofthefirm’smatchrateandtheageoftheplan.Wenowincludeamea-sureoffirmsize,thetotalnumberoffirmemployees(totemp).Theestimatedequationis131\nd7/14/995:15PMPage132Part1RegressionAnalysiswithCross-SectionalDatapraˆte(80.29)(5.44)mrate(.269)age(.00013)totemppraˆte(0.78)(0.52)mrate(.045)age(.00004)totemp2n1,534,R.100.Thesmallesttstatisticinabsolutevalueisthatonthevariabletotemp:t.00013/.000043.25,andthisisstatisticallysignificantatverysmallsignificancelevels.(Thetwo-tailedp-valueforthiststatisticisabout.001.)Thus,allofthevariablesarestatisticallysignificantatrathersmallsignificancelevels.Howbig,inapracticalsense,isthecoefficientontotemp?Holdingmrateandagefixed,ifafirmgrowsby10,000employees,theparticipationratefallsby10,000(.00013)1.3percentagepoints.Thisisahugeincreaseinnumberofemployeeswithonlyamod-esteffectontheparticipationrate.Thus,whilefirmsizedoesaffecttheparticipationrate,theeffectisnotpracticallyverylarge.Thepreviousexampleshowsthatitisespeciallyimportanttointerpretthemagni-tudeofthecoefficient,inadditiontolookingattstatistics,whenworkingwithlargesamples.Withlargesamplesizes,parameterscanbeestimatedveryprecisely:standarderrorsareoftenquitesmallrelativetothecoefficientestimates,whichusuallyresultsinstatisticalsignificance.Someresearchersinsistonusingsmallersignificancelevelsasthesamplesizeincreases,partlyasawaytooffsetthefactthatstandarderrorsaregettingsmaller.Forexample,ifwefeelcomfortablewitha5%levelwhennisafewhundred,wemightusethe1%levelwhennisafewthousand.Usingasmallersignificancelevelmeansthateconomicandstatisticalsignificancearemorelikelytocoincide,buttherearenoguar-antees:inthethepreviousexample,evenifweuseasignificancelevelassmallas.1%(one-tenthofonepercent),wewouldstillconcludethattotempisstatisticallysignifi-cant.Mostresearchersarealsowillingtoentertainlargersignificancelevelsinapplica-tionswithsmallsamplesizes,reflectingthefactthatitishardertofindsignificancewithsmallersamplesizes(thecriticalvaluesarelargerinmagnitudeandtheestimatorsarelessprecise).Unfortunately,whetherornotthisisthecasecandependontheresearcher’sunderlyingagenda.EXAMPLE4.7(EffectofJobTrainingGrantsonFirmScrapRates)Thescraprateforamanufacturingfirmisthenumberofdefectiveitemsoutofevery100itemsproducedthatmustbediscarded.Thus,adecreaseinthescrapratereflectshigherproductivity.Wecanusethescrapratetomeasuretheeffectofworkertrainingonproductivity.ForasampleofMichiganmanufacturingfirmsin1987,thefollowingequationisestimated:log(sˆcrap)(13.72)(.028)hrsemp(1.21)log(sales)(1.48)log(employ)log(sˆcrap)(4.91)(.019)hrsemp(0.41)log(sales)(0.43)log(employ)2n30,R.431.132\nd7/14/995:15PMPage133Chapter4MultipleRegressionAnalysis:Inference(ThisregressionusesasubsetofthedatainJTRAIN.RAW.)Thevariablehrsempisannualhoursoftrainingperemployee,salesisannualfirmsales(indollars),andemployisnumberoffirmemployees.Theaveragescraprateinthesampleisabout3.5,andtheaveragehrsempisabout7.3.Themainvariableofinterestishrsemp.Onemorehouroftrainingperemployeelow-erslog(scrap)by.028,whichmeansthescraprateisabout2.8%lower.Thus,ifhrsempincreasesby5—eachemployeeistrained5morehoursperyear—thescraprateisesti-matedtofallby5(2.8)14%.Thisseemslikeareasonablylargeeffect,butwhethertheadditionaltrainingisworthwhiletothefirmdependsonthecostoftrainingandtheben-efitsfromalowerscraprate.Wedonothavethenumbersneededtodoacostbenefitanalysis,buttheestimatedeffectseemsnontrivial.Whataboutthestatisticalsignificanceofthetrainingvariable?Thetstatisticonhrsempis.028/.0191.47,andnowyouprobablyrecognizethisasnotbeinglargeenoughinmagnitudetoconcludethathrsempisstatisticallysignificantatthe5%level.Infact,with30426degreesoffreedomfortheone-sidedalternativeH1:hrsemp0,the5%crit-icalvalueisabout1.71.Thus,usingastrict5%leveltest,wemustconcludethathrsempisnotstatisticallysignificant,evenusingaone-sidedalternative.Becausethesamplesizeisprettysmall,wemightbemoreliberalwiththesignificancelevel.The10%criticalvalueis1.32,andsohrsempissignificantagainsttheone-sidedalternativeatthe10%level.Thep-valueiseasilycomputedasP(T261.47).077.Thismaybealowenoughp-valuetoconcludethattheestimatedeffectoftrainingisnotjustduetosamplingerror,butsomeeconomistswouldhavedifferentopinionsonthis.Rememberthatlargestandarderrorscanalsobearesultofmulticollinearity(highcorrelationamongsomeoftheindependentvariables),evenifthesamplesizeseemsfairlylarge.AswediscussedinSection3.4,thereisnotmuchwecandoaboutthisproblemotherthantocollectmoredataorchangethescopeoftheanalysisbydroppingcertainindependentvariablesfromthemodel.Asinthecaseofasmallsamplesize,itcanbehardtopreciselyestimatepartialeffectswhensomeoftheexplanatoryvariablesarehighlycorrelated.(Section4.5containsanexample.)Weendthissectionwithsomeguidelinesfordiscussingtheeconomicandstatisti-calsignificanceofavariableinamultipleregressionmodel:1.Checkforstatisticalsignificance.Ifthevariableisstatisticallysignificant,dis-cussthemagnitudeofthecoefficienttogetanideaofitspracticaloreconomicimportance.Thislatterstepcanrequiresomecare,dependingonhowtheinde-pendentanddependentvariablesappearintheequation.(Inparticular,whataretheunitsofmeasurement?Dothevariablesappearinlogarithmicform?)2.Ifavariableisnotstatisticallysignificantattheusuallevels(10%,5%or1%),youmightstillaskifthevariablehastheexpectedeffectonyandwhetherthateffectispracticallylarge.Ifitislarge,youshouldcomputeap-valueforthetstatistic.Forsmallsamplesizes,youcansometimesmakeacaseforp-valuesaslargeas.20(buttherearenohardrules).Withlargep-values,thatis,smalltsta-tistics,wearetreadingonthinicebecausethepracticallylargeestimatesmaybeduetosamplingerror:adifferentrandomsamplecouldresultinaverydifferentestimate.133\nd7/14/995:15PMPage134Part1RegressionAnalysiswithCross-SectionalData3.Itiscommontofindvariableswithsmalltstatisticsthathavethe“wrong”sign.Forpracticalpurposes,thesecanbeignored:weconcludethatthevariablesarestatisticallyinsignificant.Asignificantvariablethathastheunexpectedsignandapracticallylargeeffectismuchmoretroublinganddifficulttoresolve.Onemustusuallythinkmoreaboutthemodelandthenatureofthedatainordertosolvesuchproblems.Oftenacounterintuitive,significantestimateresultsfromtheomissionofakeyvariableorfromoneoftheimportantproblemswewilldis-cussinChapters9and15.4.3CONFIDENCEINTERVALSUndertheclassicallinearmodelassumptions,wecaneasilyconstructaconfidenceinterval(CI)forthepopulationparameterj.Confidenceintervalsarealsocalledintervalestimatesbecausetheyprovidearangeoflikelyvaluesforthepopulationpara-meter,andnotjustapointestimate.Usingthefactthat(ˆjj)/se(ˆj)hasatdistributionwithnk1degreesoffreedom[see(4.3)],simplemanipulationleadstoaCIfortheunknownj.A95%con-fidenceinterval,givenbyˆjcse(ˆj),(4.16)thwheretheconstantcisthe97.5percentileinatnk1distribution.Moreprecisely,thelowerandupperboundsoftheconfidenceintervalaregivenbyjˆjcse(ˆj)¯and¯jˆjcse(ˆj),respectively.Atthispoint,itisusefultoreviewthemeaningofaconfidenceinterval.Ifrandomsampleswereobtainedoverandoveragain,withj,and¯jcomputedeachtime,then¯the(unknown)populationvaluejwouldlieintheinterval(j,¯j)for95%ofthesam-¯ples.Unfortunately,forthesinglesamplethatweusetocontructtheCI,wedonotknowwhetherjisactuallycontainedintheinterval.Wehopewehaveobtainedasam-plethatisoneofthe95%ofallsampleswheretheintervalestimatecontainsj,butwehavenoguarantee.Constructingaconfidenceintervalisverysimplewhenusingcurrentcomputingtechnology.Threequantitiesareneeded:ˆj,se(ˆj),andc.Thecoefficientestimateanditsstandarderrorarereportedbyanyregressionpackage.Toobtainthevaluec,wemustknowthedegreesoffreedom,nk1,andthelevelofconfidence—95%inthiscase.Then,thevalueforcisobtainedfromthetn-k-1distribution.Asanexample,fordfnk125,a95%confidenceintervalforanyjisgivenby[ˆj2.06se(ˆj),ˆj2.06se(ˆj)].Whennk1120,thetnk1distributioniscloseenoughtonormaltousetheth97.5percentileinastandardnormaldistributionforconstructinga95%CI:ˆj1.96se(ˆj).Infact,whennk150,thevalueofcissocloseto2thatwecan134\nd7/14/995:15PMPage135Chapter4MultipleRegressionAnalysis:Inferenceuseasimpleruleofthumbfora95%confidenceinterval:ˆjplusorminustwoofitsstandarderrors.Forsmalldegreesoffreedom,theexactpercentilesshouldbeobtainedfromthettables.Itiseasytoconstructconfidenceintervalsforanyotherlevelofconfidence.Forthexample,a90%CIisobtainedbychoosingctobethe95percentileinthetnk1dis-tribution.Whendfnk125,c1.71,andsothe90%CIisˆj1.71se(ˆj),thwhichisnecessarilynarrowerthanthe95%CI.Fora99%CI,cisthe99.5percentileinthet25distribution.Whendf25,the99%CIisroughlyˆj2.79se(ˆj),whichisinevitablywiderthanthe95%CI.Manymodernregressionpackagessaveusfromdoinganycalculationsbyreport-inga95%CIalongwitheachcoefficientanditsstandarderror.Onceaconfidenceinter-valisconstructed,itiseasytocarryouttwo-tailedhypothesestests.IfthenullhypothesisisH0:jaj,thenH0isrejectedagainstH1:jajat(say)the5%signif-icancelevelif,andonlyif,ajisnotinthe95%confidenceinterval.EXAMPLE4.8(HedonicPriceModelforHouses)Amodelthatexplainsthepriceofagoodintermsofthegood’scharacteristicsiscalledanhedonicpricemodel.Thefollowingequationisanhedonicpricemodelforhousingprices;thecharacteristicsaresquarefootage(sqrft),numberofbedrooms(bdrms),andnumberofbathrooms(bthrms).Oftenpriceappearsinlogarithmicform,asdosomeoftheexplana-toryvariables.Usingn19observationsonhousesthatweresoldinWaltham,Massachusetts,in1990,theestimatedequation(withstandarderrorsinparenthesesbelowthecoefficientestimates)islog(pˆrice)(7.46)(.634)log(sqrft)(.066)bdrms(.158)bthrmslog(pˆrice)(1.15)(.184)log(sqrft)(.059)bdrms(.075)bthrms2n19,R.806.Sincepriceandsqrftbothappearinlogarithmicform,thepriceelasticitywithrespecttosquarefootageis.634,sothat,holdingnumberofbedroomsandbathroomsfixed,a1%increaseinsquarefootageincreasesthepredictedhousingpricebyabout.634%.Wecanconstructa95%confidenceintervalforthepopulationelasticityusingthefactthattheesti-matedmodelhasnk1193115degreesoffreedom.FromTableG.2,wethfindthe97.5percentileinthet15distribution:c2.131.Thus,the95%confidenceinter-valforlog(sqrft)is.6342.131(.184),or(.242,1.026).Sincezeroisexcludedfromthiscon-fidenceinterval,werejectH0:log(sqrft)0againstthetwo-sidedalternativeatthe5%level.Thecoefficientonbdrmsisnegative,whichseemscounterintuitive.However,itisimportanttoremembertheceterisparibusnatureofthiscoefficient:itmeasurestheeffectofanotherbedroom,holdingsizeofthehouseandnumberofbathroomsfixed.Iftwohousesarethesamesizebutonehasmorebedrooms,thenthehousewithmorebedroomshassmallerbedrooms;morebedroomsthataresmallerisnotnecessarilyagoodthing.Inanycase,wecanseethatthe95%confidenceintervalforbdrmsisfairlywide,anditcon-tainsthevaluezero:.0662.131(.059)or(.192,.060).Thus,bdrmsdoesnothaveastatisticallysignificantceterisparibuseffectonhousingprice.135\nd7/14/995:15PMPage136Part1RegressionAnalysiswithCross-SectionalDataGivensizeandnumberofbedrooms,onemorebathroomispredictedtoincreasehous-ingpricebyabout15.8%.(Rememberthatwemustmultiplythecoefficientonbthrmsby100toturntheeffectintoapercent.)The95%confidenceintervalforbthrmsis(.002,.318).Inthiscase,zeroisbarelyintheconfidenceinterval,sotechnicallyspeakingˆbthrmsisnotstatisticallysignificantatthe5%levelagainstatwo-sidedalternative.Sinceitisveryclosetobeingsignificant,wewouldprobablyconcludethatnumberofbathroomshasaneffectonlog(price).Youshouldrememberthataconfidenceintervalisonlyasgoodastheunderlyingassumptionsusedtoconstructit.Ifwehaveomittedimportantfactorsthatarecorre-latedwiththeexplanatoryvariables,thenthecoefficientestimatesarenotreliable:OLSisbiased.Ifheteroskedasticityispresent—forinstance,inthepreviousexample,ifthevarianceoflog(price)dependsonanyoftheexplanatoryvariables—thenthestandarderrorisnotvalidasanestimateofsd(ˆj)(aswediscussedinSection3.4),andthecon-fidenceintervalcomputedusingthesestandarderrorswillnottrulybea95%CI.WehavealsousedthenormalityassumptionontheerrorsinobtainingtheseCIs,but,aswewillseeinChapter5,thisisnotasimportantforapplicationsinvolvinghundredsofobservations.4.4TESTINGHYPOTHESESABOUTASINGLELINEARCOMBINATIONOFTHEPARAMETERSTheprevioustwosectionshaveshownhowtouseclassicalhypothesistestingorconfi-denceintervalstotesthypothesesaboutasinglejatatime.Inapplications,wemustoftentesthypothesesinvolvingmorethanoneofthepopulationparameters.Inthissec-tion,weshowhowtotestasinglehypothesisinvolvingmorethanoneofthej.Section4.5showshowtotestmultiplehypotheses.Toillustratethegeneralapproach,wewillconsiderasimplemodeltocomparethereturnstoeducationatjuniorcollegesandfour-yearcolleges;forsimplicity,werefertothelatteras“universities.”[ThisexampleismotivatedbyKaneandRouse(1995),whoprovideadetailedanalysisofthisquestion.]Thepopulationincludesworkingpeoplewithahighschooldegree,andthemodelislog(wage)01jc2univ3experu,(4.17)wherejcisnumberofyearsattendingatwo-yearcollegeandunivisnumberofyearsatafour-yearcollege.Notethatanycombinationofjuniorcollegeandcollegeisallowed,includingjc0anduniv0.Thehypothesisofinterestiswhetherayearatajuniorcollegeisworthayearatauniversity:thisisstatedasH0:12.(4.18)UnderH0,anotheryearatajuniorcollegeandanotheryearatauniversityleadtothesameceterisparibuspercentageincreaseinwage.Forthemostpart,thealternativeof136\nd7/14/995:15PMPage137Chapter4MultipleRegressionAnalysis:Inferenceinterestisone-sided:ayearatajuniorcollegeisworthlessthanayearatauniversity.ThisisstatedasH1:12.(4.19)Thehypothesesin(4.18)and(4.19)concerntwoparameters,1and2,asituationwehavenotfacedyet.Wecannotsimplyusetheindividualtstatisticsforˆ1andˆ2totestH0.However,conceptually,thereisnodifficultyinconstructingatstatisticfortest-ing(4.18).Inordertodoso,werewritethenullandalternativeasH0:120andH1:120,respectively.Thetstatisticisbasedonwhethertheestimateddiffer-enceˆ1ˆ2issufficientlylessthanzerotowarrantrejecting(4.18)infavorof(4.19).Toaccountforthesamplingerrorinourestimators,westandardizethisdifferencebydividingbythestandarderror:ˆ1ˆ2t.(4.20)se(ˆ1ˆ2)Oncewehavethetstatisticin(4.20),testingproceedsasbefore.Wechooseasignifi-cancelevelforthetestand,basedonthedf,obtainacriticalvalue.Becausethealter-nativeisoftheformin(4.19),therejectionruleisoftheformtc,wherecisapositivevaluechosenfromtheappropriatetdistribution.Or,wecomputethetstatisticandthencomputethep-value(seeSection4.2).Theonlythingthatmakestestingtheequalityoftwodifferentparametersmoredif-ficultthantestingaboutasinglejisobtainingthestandarderrorinthedenominatorof(4.20).ObtainingthenumeratoristrivialoncewehavepeformedtheOLSregression.Forconcreteness,supposethefollowingequationhasbeenobtainedusingn285indi-viduals:log(wˆage)1.43.098jc.124univ.019experlog(wˆage)(0.27)(.031)jc(.035)univ(.008)exper(4.21)2n285,R.243.Itisclearfrom(4.21)thatjcandunivhavebotheconomicallyandstatisticallysignifi-canteffectsonwage.Thisiscertainlyofinterest,butwearemoreconcernedabouttest-ingwhethertheestimateddifferenceinthecoefficientsisstatisticallysignificant.Thedifferenceisestimatedasˆ1ˆ2.026,sothereturntoayearatajuniorcollegeisabout2.6percentagepointslessthanayearatauniversity.Economically,thisisnotatrivialdifference.Thedifferenceof.026isthenumeratorofthetstatisticin(4.20).Unfortunately,theregressionresultsinequation(4.21)donotcontainenoughin-formationtoobtainthestandarderrorofˆ1ˆ2.Itmightbetemptingtoclaimthatse(ˆ1ˆ2)se(ˆ1)se(ˆ2),butthisdoesnotmakesenseinthecurrentexamplebecausese(ˆ1)se(ˆ2).038.Standarderrorsmustalwaysbepositivebecausetheyareestimatesofstandarddeviations.Whilethestandarderrorofthedifferenceˆ1ˆ2certainlydependsonse(ˆ1)andse(ˆ2),itdoessoinasomewhatcomplicatedway.Tofindse(ˆ1ˆ2),wefirstobtainthevarianceofthedifference.UsingtheresultsonvariancesinAppendixB,wehave137\nd7/14/995:15PMPage138Part1RegressionAnalysiswithCross-SectionalDataVar(ˆ1ˆ2)Var(ˆ1)Var(ˆ2)2Cov(ˆ1,ˆ2).(4.22)Observecarefullyhowthetwovariancesareaddedtogether,andtwicethecovarianceisthensubtracted.Thestandarddeviationofˆ1ˆ2isjustthesquarerootof(4.22)22and,since[se(ˆ1)]isanunbiasedestimatorofVar(ˆ1),andsimilarlyfor[se(ˆ2)],wehave221/2se(ˆ1ˆ2)[se(ˆ1)][se(ˆ2)]2s12,(4.23)wheres12denotesanestimateofCov(ˆ1,ˆ2).WehavenotdisplayedaformulaforCov(ˆ1,ˆ2).Someregressionpackageshavefeaturesthatallowonetoobtains12,inwhichcaseonecancomputethestandarderrorin(4.23)andthenthetstatisticin(4.20).AppendixEshowshowtousematrixalgebratoobtains12.Wesuggestanotherroutethatismuchsimplertocompute,lesslikelytoleadtoanerror,andreadilyappliedtoavarietyofproblems.Ratherthantryingtocomputese(ˆ1ˆ2)from(4.23),itismucheasiertoestimateadifferentmodelthatdirectlydeliversthestandarderrorofinterest.Defineanewparameterasthedifferencebetween1and2:112.ThenwewanttotestH0:10againstH1:10.(4.24)Thetstatistic(4.20)intermsofˆ1isjusttˆ1/se(ˆ1).Thechallengeisfindingse(ˆ1).Wecandothisbyrewritingthemodelsothat1appearsdirectlyononeoftheinde-pendentvariables.Since112,wecanalsowrite112.Pluggingthisinto(4.17)andrearranginggivestheequationlog(wage)0(12)jc2univ3experu(4.25)01jc2(jcuniv)3experu.Thekeyinsightisthattheparameterweareinterestedintestinghypothesesabout,1,nowmultipliesthevariablejc.Theinterceptisstill0,andexperstillshowsupasbeingmultipliedby3.Moreimportantly,thereisanewvariablemultiplying2,namelyjcuniv.Thus,ifwewanttodirectlyestimate1andobtainthestandarderrorˆ1,thenwemustconstructthenewvariablejcunivandincludeitintheregressionmodelinplaceofuniv.Inthisexample,thenewvariablehasanaturalinterpretation:itistotalyearsofcollege,sodefinetotcolljcunivandwrite(4.25)aslog(wage)01jc2totcoll3experu.(4.26)Theparameter1hasdisappearedfromthemodel,while1appearsexplicitly.Thismodelisreallyjustadifferentwayofwritingtheoriginalmodel.Theonlyreasonwehavedefinedthisnewmodelisthat,whenweestimateit,thecoefficientonjcisˆ1and,moreimportantly,se(ˆ1)isreportedalongwiththeestimate.Thetstatisticthatwewantistheonereportedbyanyregressionpackageonthevariablejc(notthevariabletotcoll).138\nd7/14/995:15PMPage139Chapter4MultipleRegressionAnalysis:InferenceWhenwedothiswiththe285observationsusedearlier,theresultislog(wˆage)1.43.026jc.124totcoll.019experlog(wˆage)(0.27)(.018)jc(.035)totcoll(.008)exper(4.27)2n285,R.243.Theonlynumberinthisequationthatwecouldnotgetfrom(4.21)isthestandarderrorfortheestimate.026,whichis.018.Thetstatisticfortesting(4.18)is.026/.0181.44.Againsttheone-sidedalternative(4.19),thep-valueisabout.075,sothereissome,butnotstrong,evidenceagainst(4.18).Theinterceptandslopeestimateonexper,alongwiththeirstandarderrors,arethesameasin(4.21).Thisfactmustbetrue,anditprovidesonewayofcheckingwhetherthetransformedequationhasbeenproperlyestimated.Thecoefficientonthenewvari-able,totcoll,isthesameasthecoefficientonunivin(4.21),andthestandarderrorisalsothesame.Weknowthatthismusthappenbycomparing(4.17)and(4.25).Itisquitesimpletocomputea95%confidenceintervalfor112.Usingthestandardnormalapproximation,theCIisobtainedasusual:ˆ11.96se(ˆ1),whichinthiscaseleadsto.026.035.Thestrategyofrewritingthemodelsothatitcontainstheparameterofinterestworksinallcasesandiseasytoimplement.(SeeProblems4.12and4.14forotherexamples.)4.5TESTINGMULTIPLELINEARRESTRICTIONS:THEFTESTThetstatisticassociatedwithanyOLScoefficientcanbeusedtotestwhetherthecor-respondingunknownparameterinthepopulationisequaltoanygivenconstant(whichisusually,butnotalways,zero).Wehavejustshownhowtotesthypothesesaboutasin-glelinearcombinationofthejbyrearrangingtheequationandrunningaregressionusingtransformedvariables.Butsofar,wehaveonlycoveredhypothesesinvolvingasinglerestriction.Frequently,wewishtotestmultiplehypothesesabouttheunderlyingparameters0,1,…,k.Webeginwiththeleadingcaseoftestingwhetherasetofindependentvariableshasnopartialeffectonadependentvariable.TestingExclusionRestrictionsWealreadyknowhowtotestwhetheraparticularvariablehasnopartialeffectonthedependentvariable:usethetstatistic.Nowwewanttotestwhetheragroupofvariableshasnoeffectonthedependentvariable.Moreprecisely,thenullhypothesisisthatasetofvariableshasnoeffectony,onceanothersetofvariableshasbeencontrolled.Asanillustrationofwhytestingsignificanceofagroupofvariablesisuseful,weconsiderthefollowingmodelthatexplainsmajorleaguebaseballplayers’salaries:log(salary)01years2gamesyr3bavg(4.28)4hrunsyr5rbisyru,139\nd7/14/995:15PMPage140Part1RegressionAnalysiswithCross-SectionalDatawheresalaryisthe1993totalsalary,yearsisyearsintheleague,gamesyrisaver-agegamesplayedperyear,bavgiscareerbattingaverage(forexample,bavg250),hrunsyrishomerunsperyear,andrbisyrisrunsbattedinperyear.Supposewewanttotestthenullhypothesisthat,onceyearsintheleagueandgamesperyearhavebeencontrolledfor,thestatisticsmeasuringperformance—bavg,hrunsyr,andrbisyr—havenoeffectonsalary.Essentially,thenullhypothesisstatesthatproductivityasmeasuredbybaseballstatisticshasnoeffectonsalary.Intermsoftheparametersofthemodel,thenullhypothesisisstatedasH0:30,40,50.(4.29)Thenull(4.29)constitutesthreeexclusionrestrictions:if(4.29)istrue,thenbavg,hrunsyr,andrbisyrhavenoeffectonlog(salary)afteryearsandgamesyrhavebeencon-trolledforandthereforeshouldbeexcludedfromthemodel.Thisisanexampleofasetofmultiplerestrictionsbecauseweareputtingmorethanonerestrictiononthepara-metersin(4.28);wewillseemoregeneralexamplesofmultiplerestrictionslater.Atestofmultiplerestrictionsiscalledamultiplehypothesestestorajointhypothesestest.Whatshouldbethealternativeto(4.29)?Ifwhatwehaveinmindisthat“perfor-mancestatisticsmatter,evenaftercontrollingforyearsintheleagueandgamesperyear,”thentheappropriatealternativeissimplyH1:H0isnottrue.(4.30)Thealternative(4.30)holdsifatleastoneof3,4,or5isdifferentfromzero.(Anyorallcouldbedifferentfromzero.)ThetestwestudyhereisconstructedtodetectanyviolationofH0.ItisalsovalidwhenthealternativeissomethinglikeH1:30,or40,or50,butitwillnotbethebestpossibletestundersuchalternatives.Wedonothavethespaceorstatisticalbackgroundnecessarytocoverteststhathavemorepowerundermultipleone-sidedalternatives.Howshouldweproceedintesting(4.29)against(4.30)?Itistemptingtotest(4.29)byusingthetstatisticsonthevariablesbavg,hrunsyr,andrbisyrtodeterminewhethereachvariableisindividuallysignificant.Thisoptionisnotappropriate.Aparticulartstatistictestsahypothesisthatputsnorestrictionsontheotherparameters.Besides,wewouldhavethreeoutcomestocontendwith—oneforeachtstatistic.Whatwouldcon-stituterejectionof(4.29)at,say,the5%level?Shouldallthreeoronlyoneofthethreetstatisticsberequiredtobesignificantatthe5%level?Thesearehardquestions,andfortunatelywedonothavetoanswerthem.Furthermore,usingseparatetstatisticstotestamultiplehypothesislike(4.29)canbeverymisleading.Weneedawaytotesttheexclusionrestrictionsjointly.Toillustratetheseissues,weestimateequation(4.28)usingthedatainMLB1.RAW.Thisgiveslog(saˆlary)(11.10)(.0689)years(.0126)gamesyrlog(saˆlary)(0.29)(.0121)years(.0026)gamesyr(.00098)bavg(.0144)hrunsyr(.0108)rbisyr(4.31)(.00110)bavg(.0161)hrunsyr(.0072)rbisyr2n353,SSR183.186,R.6278,140\nd7/14/995:15PMPage141Chapter4MultipleRegressionAnalysis:InferencewhereSSRisthesumofsquaredresiduals.(Wewillusethislater.)Wehaveleftsev-eraltermsafterthedecimalinSSRandR-squaredtofacilitatefuturecomparisons.Equation(4.31)revealsthat,whileyearsandgamesyrarestatisticallysignificant,noneofthevariablesbavg,hrunsyr,andrbisyrhasastatisticallysignificanttstatisticagainstatwo-sidedalternative,atthe5%significancelevel.(Thetstatisticonrbisyristheclos-esttobeingsignificant;itstwo-sidedp-valueis.134.)Thus,basedonthethreetstatis-tics,itappearsthatwecannotrejectH0.Thisconclusionturnsouttobewrong.Inordertoseethis,wemustderiveatestofmultiplerestrictionswhosedistributionisknownandtabulated.Thesumofsquaredresidualsnowturnsouttoprovideaveryconvenientbasisfortestingmultiplehypothe-ses.WewillalsoshowhowtheR-squaredcanbeusedinthespecialcaseoftestingforexclusionrestrictions.Knowingthesumofsquaredresidualsin(4.31)tellsusnothingaboutthetruthofthehypothesisin(4.29).However,thefactorthatwilltellussomethingishowmuchtheSSRincreaseswhenwedropthevariablesbavg,hrunsyr,andrbisyrfromthemodel.Rememberthat,becausetheOLSestimatesarechosentominimizethesumofsquaredresiduals,theSSRalwaysincreaseswhenvariablesaredroppedfromthemodel;thisisanalgebraicfact.Thequestioniswhetherthisincreaseislargeenough,relativetotheSSRinthemodelwithallofthevariables,towarrantrejectingthenullhypothesis.Themodelwithoutthethreevariablesinquestionissimplylog(salary)01years2gamesyru.(4.32)Inthecontextofhypothesistesting,equation(4.32)istherestrictedmodelfortesting(4.29);model(4.28)iscalledtheunrestrictedmodel.Therestrictedmodelalwayshasfewerparametersthantheunrestrictedmodel.WhenweestimatetherestrictedmodelusingthedatainMLB1.RAW,weobtainlog(saˆlary)(11.22)(.0713)years(.0202)gamesyrlog(saˆlary)(0.11)(.0125)years(.0013)gamesyr(4.33)2n353,SSR198.311,R.5971.Aswesurmised,theSSRfrom(4.33)isgreaterthantheSSRfrom(4.31),andtheR-squaredfromtherestrictedmodelislessthantheR-squaredfromtheunrestrictedmodel.WhatweneedtodecideiswhethertheincreaseintheSSRingoingfromtheunrestrictedmodeltotherestrictedmodel(183.186to198.311)islargeenoughtowar-rantrejectionof(4.29).Aswithalltesting,theanswerdependsonthesignificancelevelofthetest.Butwecannotcarryoutthetestatachosensignificanceleveluntilwehaveastatisticwhosedistributionisknown,andcanbetabulated,underH0.Thus,weneedawaytocombinetheinformationinthetwoSSRstoobtainateststatisticwithaknowndistributionunderH0.Sinceitisnomoredifficult,wemightaswellderivethetestforthegeneralcase.Writetheunrestrictedmodelwithkindependentvariablesasy01x1…kxku;(4.34)141\nd7/14/995:15PMPage142Part1RegressionAnalysiswithCross-SectionalDatathenumberofparametersintheunrestrictedmodelisk1.(Remembertoaddonefortheintercept.)Supposethatwehaveqexclusionrestrictionstotest:thatis,thenullhypothesisstatesthatqofthevariablesin(4.34)havezerocoefficients.Fornotationalsimplicity,assumethatitisthelastqvariablesinthelistofindependentvariables:xkq+1,…,xk.(Theorderofthevariables,ofcourse,isarbitraryandunimportant.)ThenullhypothesisisstatedasH0:kq10,…,k0,(4.35)whichputsqexclusionrestrictionsonthemodel(4.34).Thealternativeto(4.35)issim-plythatitisfalse;thismeansthatatleastoneoftheparameterslistedin(4.35)isdif-ferentfromzero.WhenweimposetherestrictionsunderH0,weareleftwiththerestrictedmodel:y01x1…kqxkqu.(4.36)Inthissubsection,weassumethatboththeunrestrictedandrestrictedmodelscontainanintercept,sincethatisthecasemostwidelyencounteredinpractice.Nowfortheteststatisticitself.Earlier,wesuggestedthatlookingattherelativeincreaseintheSSRwhenmovingfromtheunrestrictedtotherestrictedmodelshouldbeinformativefortestingthehypothesis(4.35).TheFstatistic(orFratio)isdefinedby(SSRrSSRur)/qF,(4.37)SSRur/(nk1)whereSSRristhesumofsquaredresidualsfromtherestrictedmodelandSSRuristhesumofsquaredresidualsfromtheunrestrictedmodel.Youshouldimmediatelynoticethat,sinceSSRrcanbenosmallerthanSSRur,QUESTION4.4theFstatisticisalwaysnonnegative(andalmostalwaysstrictlypositive).Thus,ifConsiderrelatingindividualperformanceonastandardizedtest,score,toavarietyofothervariables.SchoolfactorsincludeaverageyoucomputeanegativeFstatistic,thenclasssize,perstudentexpenditures,averageteachercompensation,somethingiswrong;theorderoftheSSRsandtotalschoolenrollment.OthervariablesspecifictothestudentinthenumeratorofFhasusuallybeenarefamilyincome,mother’seducation,father’seducation,andnum-reversed.Also,theSSRinthedenominatorberofsiblings.ThemodelisofFistheSSRfromtheunrestrictedscore01classize2expend3tchcompmodel.Theeasiestwaytoremember4enroll5faminc6motheducwheretheSSRsappearistothinkofFas7fatheduc8siblingsu.measuringtherelativeincreaseinSSRwhenmovingfromtheunrestrictedtotheStatethenullhypothesisthatstudent-specificvariableshavenoeffectonstandardizedtestperformance,onceschool-relatedfactorsrestrictedmodel.havebeencontrolledfor.Whatarekandqforthisexample?WriteThedifferenceinSSRsinthenumera-downtherestrictedversionofthemodel.torofFisdividedbyq,whichisthenum-berofrestrictionsimposedinmovingfromtheunrestrictedtotherestrictedmodel(qindependentvariablesaredropped).Therefore,wecanwrite142\nd7/14/995:15PMPage143Chapter4MultipleRegressionAnalysis:Inferenceqnumeratordegreesoffreedomdfrdfur,(4.38)whichalsoshowsthatqisthedifferenceindegreesoffreedombetweentherestrictedandunrestrictedmodels.(Recallthatdfnumberofobservationsnumberofesti-matedparameters.)Sincetherestrictedmodelhasfewerparameters—andeachmodelisestimatedusingthesamenobservations—dfrisalwaysgreaterthandfur.TheSSRinthedenominatorofFisdividedbythedegreesoffreedomintheunre-strictedmodel:nk1denominatordegreesoffreedomdfur.(4.39)2Infact,thedenominatorofFisjusttheunbiasedestimatorofVar(u)intheunre-strictedmodel.Inaparticularapplication,computingtheFstatisticiseasierthanwadingthroughthesomewhatcumbersomenotationusedtodescribethegeneralcase.Wefirstobtainthedegreesoffreedomintheunrestrictedmodel,dfur.Then,wecounthowmanyvari-ablesareexcludedintherestrictedmodel;thisisq.TheSSRsarereportedwitheveryOLSregression,andsoformingtheFstatisticissimple.Inthemajorleaguebaseballsalaryregression,n353,andthefullmodel(4.28)containssixparameters.Thus,nk1dfur3536347.Therestrictedmodel(4.32)containsthreefewerindependentvariablesthan(4.28),andsoq3.Thus,wehavealloftheingredientstocomputetheFstatistic;weholdoffdoingsountilweknowwhattodowithit.InordertousetheFstatistic,wemustknowitssamplingdistributionunderthenullinordertochoosecriticalvaluesandrejectionrules.Itcanbeshownthat,underH0(andassumingtheCLMassumptionshold),FisdistributedasanFrandomvariablewith(q,nk1)degreesoffreedom.WewritethisasF~Fq,nk1.ThedistributionofFq,nk1isreadilytabulatedandavailableinstatisticaltables(seeTableG.3)and,evenmoreimportantly,instatisticalsoftware.WewillnotderivetheFdistributionbecausethemathematicsisveryinvolved.Basically,itcanbeshownthatequation(4.37)isactuallytheratiooftwoindependentchi-squarerandomvariables,dividedbytheirrespectivedegreesoffreedom.Thenumeratorchi-squarerandomvariablehasqdegreesoffreedom,andthechi-squareinthedenominatorhasnk1degreesoffreedom.ThisisthedefinitionofanFdis-tributedrandomvariable(seeAppendixB).ItisprettyclearfromthedefinitionofFthatwewillrejectH0infavorofH1whenFissufficiently“large.”Howlargedependsonourchosensignificancelevel.Supposeththatwehavedecidedona5%leveltest.Letcbethe95percentileintheFq,nk1dis-tribution.Thiscriticalvaluedependsonq(thenumeratordf)andnk1(thedenominatordf).Itisimportanttokeepthenumeratoranddenominatordegreesoffree-domstraight.The10%,5%,and1%criticalvaluesfortheFdistributionaregiveninTableG.3.Therejectionruleissimple.Oncechasbeenobtained,werejectH0infavorofH1atthechosensignificancelevelif143\nd7/14/995:15PMPage144Part1RegressionAnalysiswithCross-SectionalDataFc.(4.40)Witha5%significancelevel,q3,nk160,andthecriticalvalueisc2.76.WewouldrejectH0atthe5%levelifthecomputedvalueoftheFstatisticexceeds2.76.The5%criticalvalueandrejectionregionareshowninFigure4.7.Forthesamedegreesoffreedom,the1%criticalvalueis4.13.Inmostapplications,thenumeratordegreesoffreedom(q)willbenotablysmallerthanthedenominatordegreesoffreedom(nk1).Applicationswherenk1issmallareunlikelytobesuccessfulbecausetheparametersinthenullmodelwillprobablynotbepreciselyestimated.Whenthedenominatordfreachesabout120,theFdistributionisnolongersensitivetoit.(Thisisentirelyanalogoustothetdistributionbeingwell-approximatedbythestandardnormaldistributionasthedfgetslarge.)Thus,thereisanentryinthetableforthedenominatordf,andthisiswhatweusewithlargesamples(sincenk1isthenlarge).Asimilarstatementholdsforaverylargenumeratordf,butthisrarelyoccursinapplications.IfH0isrejected,thenwesaythatxkq1,…,xkarejointlystatisticallysignificant(orjustjointlysignificant)attheappropriatesignificancelevel.ThistestalonedoesnotFigure4.7The5%criticalvalueandrejectionregioninanF3,60distribution.area=.95area=.050rejection2.76region144\nd7/14/995:15PMPage145Chapter4MultipleRegressionAnalysis:Inferenceallowustosaywhichofthevariableshasapartialeffectony;theymayallaffectyormaybeonlyoneaffectsy.Ifthenullisnotrejected,thenthevariablesarejointlyinsignificant,whichoftenjustifiesdroppingthemfromthemodel.Forthemajorleaguebaseballexamplewiththreenumeratordegreesoffreedomand347denominatordegreesoffreedom,the5%criticalvalueis2.60,andthe1%criticalvalueis3.78.WerejectH0atthe1%levelifFisabove3.78;werejectatthe5%levelifFisabove2.60.Wearenowinapositiontotestthehypothesisthatwebeganthissectionwith:aftercontrollingforyearsandgamesyr,thevariablesbavg,hrunsyr,andrbisyrhavenoeffectonplayers’salaries.Inpractice,itiseasiesttofirstcompute(SSRrSSRur)/SSRurandtomultiplytheresultby(nk1)/q;thereasontheformulaisstatedasin(4.37)isthatitmakesiteasiertokeepthenumeratoranddenominatordegreesoffreedomstraight.UsingtheSSRsin(4.31)and(4.33),wehave(198.311183.186)347F9.55.183.1863Thisnumberiswellabovethe1%criticalvalueintheFdistributionwith3and347degreesoffreedom,andsowesoundlyrejectthehypothesisthatbavg,hrunsyr,andrbisyrhavenoeffectonsalary.Theoutcomeofthejointtestmayseemsurprisinginlightoftheinsignificanttsta-tisticsforthethreevariables.Whatishappeningisthatthetwovariableshrunsyrandrbisyrarehighlycorrelated,andthismulticollinearitymakesitdifficulttouncoverthepartialeffectofeachvariable;thisisreflectedintheindividualtstatistics.TheFsta-tistictestswhetherthesevariables(includingbavg)arejointlysignificant,andmulti-collinearitybetweenhrunsyrandrbisyrismuchlessrelevantfortestingthishypothesis.InProblem4.16,youareaskedtoreestimatethemodelwhiledroppingrbisyr,inwhichcasehrunsyrbecomesverysignificant.Thesameistrueforrbisyrwhenhrunsyrisdroppedfromthemodel.TheFstatisticisoftenusefulfortestingexclusionofagroupofvariableswhenthevariablesinthegrouparehighlycorrelated.Forexample,supposewewanttotestwhetherfirmperformanceaffectsthesalariesofchiefexecutiveofficers.Therearemanywaystomeasurefirmperformance,anditprobablywouldnotbeclearaheadoftimewhichmeasureswouldbemostimportant.Sincemeasuresoffirmperformancearelikelytobehighlycorrelated,hopingtofindindividuallysignificantmeasuresmightbeaskingtoomuchduetomulticollinearity.ButanFtestcanbeusedtodeterminewhether,asagroup,thefirmperformancevariablesaffectsalary.RelationshipBetweenFandtStatisticsWehaveseeninthissectionhowtheFstatisticcanbeusedtotestwhetheragroupofvariablesshouldbeincludedinamodel.WhathappensifweapplytheFstatistictothecaseoftestingsignificanceofasingleindependentvariable?Thiscaseiscertainlynotruledoutbythepreviousdevelopment.Forexample,wecantakethenulltobeH0:k0andq1(totestthesingleexclusionrestrictionthatxkcanbeexcludedfromthemodel).FromSection4.2,weknowthatthetstatisticonkcanbeusedtotestthishypothesis.Thequestion,then,isdowehavetwoseparatewaysoftestinghypotheses145\nd7/14/995:15PMPage146Part1RegressionAnalysiswithCross-SectionalDataaboutasinglecoefficient?Theanswerisno.ItcanbeshownthattheFstatisticfortest-ingexclusionofasinglevariableisequaltothesquareofthecorrespondingtstatistic.2Sincetnk1hasanF1,nk1distribution,thetwoapproachesleadtoexactlythesameoutcome,providedthatthealternativeistwo-sided.Thetstatisticismoreflexiblefortestingasinglehypothesisbecauseitcanbeusedtotestagainstone-sidedalternatives.SincetstatisticsarealsoeasiertoobtainthanFstatistics,thereisreallynoreasontouseanFstatistictotesthypothesesaboutasingleparameter.TheR-SquaredFormoftheFStatisticInmostapplications,itturnsouttobemoreconvenienttouseaformoftheFstatisticthatcanbecomputedusingtheR-squaredsfromtherestrictedandunrestrictedmodels.OnereasonforthisisthattheR-squaredisalwaysbetweenzeroandone,whereastheSSRscanbeverylargedependingontheunitsofmeasurementofy,makingthecal-culationbasedontheSSRstedious.UsingthefactthatSSRrSST(1R2)andr2SSRurSST(1Rur),wecansubstituteinto(4.37)toobtain22(RurRr)/qF(4.41)2(1Rur)/(nk1)(notethattheSSTtermscanceleverywhere).ThisiscalledtheR-squaredformoftheFstatistic.SincetheR-squaredisreportedwithalmostallregressions(whereastheSSRisnot),itiseasytousetheR-squaredsfromtheunrestrictedandrestrictedmodelstotestforexclusionofsomevariables.ParticularattentionshouldbepaidtotheorderoftheR-squaredsinthenumerator:theunrestrictedR-squaredcomesfirst[contrastthiswiththeSSRsin(4.37)].SinceR2R2,thisshowsagainthatFwillalwaysbepositive.urrInusingtheR-squaredformofthetestforexcludingasetofvariables,itisimpor-tanttonotsquaretheR-squaredbeforepluggingitintoformula(4.41);thesquaringhas2alreadybeendone.AllregressionsreportR,andthesenumbersarepluggeddirectlyinto(4.41).Forthebaseballsalaryexample,wecanuse(4.41)toobtaintheFstatistic:(.6278.5971)347F9.54,1.62783whichisveryclosetowhatweobtainedbefore.(Thedifferenceisduetoaroundingerror.)EXAMPLE4.9(Parents’EducationinaBirthWeightEquation)AsanotherexampleofcomputinganFstatistic,considerthefollowingmodeltoexplainchildbirthweightintermsofvariousfactors:bwght01cigs2parity3faminc(4.42)4motheduc5fatheducu,146\nd7/14/995:15PMPage147Chapter4MultipleRegressionAnalysis:Inferencewherebwghtisbirthweight,inpounds,cigsisaveragenumberofcigarettesthemothersmokedperdayduringpregnancy,parityisthebirthorderofthischild,famincisannualfamilyincome,motheducisyearsofschoolingforthemother,andfatheducisyearsofschoolingforthefather.Letustestthenullhypothesisthat,aftercontrollingforcigs,par-ity,andfaminc,parents’educationhasnoeffectonbirthweight.ThisisstatedasH0:40,50,andsothereareq2exclusionrestrictionstobetested.Therearek16parametersintheunrestrictedmodel(4.42),sothedfintheunrestrictedmodelisn6,wherenisthesamplesize.WewilltestthishypothesisusingthedatainBWGHT.RAW.Thisdatasetcontainsinfor-mationon1,388births,butwemustbecarefulincountingtheobservationsusedintest-ingthenullhypothesis.Itturnsoutthatinformationonatleastoneofthevariablesmotheducandfatheducismissingfor197birthsinthesample;theseobservationscannotbeincludedwhenestimatingtheunrestrictedmodel.Thus,wereallyhaven1,191obser-vations,andsothereare1,19161,185dfintheunrestrictedmodel.Wemustbesuretousethesesame1,191observationswhenestimatingtherestrictedmodel(notthefull1,388observationsthatareavailable).Generally,whenestimatingtherestrictedmodeltocomputeanFtest,wemustusethesameobservationstoestimatetheunrestrictedmodel;otherwisethetestisnotvalid.Whentherearenomissingdata,thiswillnotbeanissue.Thenumeratordfis2,andthedenominatordfis1,185;fromTableG.3,the5%criti-calvalueisc3.0.Ratherthanreportthecompleteresults,forbrevitywepresentonlythe2R-squareds.TheR-squaredforthefullmodelturnsouttobeRur.0387.Whenmotheducandfatheducaredroppedfromtheregression,theR-squaredfallstoR2.0364.Thus,therFstatisticisF[(.0387.0364)/(1.0387)](1,185/2)1.42;sincethisiswellbelowthe5%criticalvalue,wefailtorejectH0.Inotherwords,motheducandfatheducarejointlyinsignificantinthebirthweightequation.Computingp-valuesforFTestsForreportingtheoutcomesofFtests,p-valuesareespeciallyuseful.SincetheFdistri-butiondependsonthenumeratoranddenominatordf,itisdifficulttogetafeelforhowstrongorweaktheevidenceisagainstthenullhypothesissimplybylookingattheQUESTION4.5valueoftheFstatisticandoneortwocrit-ThedatainATTEND.RAWwereusedtoestimatethetwoequationsicalvalues.atnˆdrte(47.13)(13.37)priGPAIntheFtestingcontext,thep-valueisatnˆdrte(2.87)(1.09)priGPAdefinedas2n680,R.183,andp-valueP(F),(4.43)atnˆdrte(75.70)(17.26)priGPA1.72ACT,atnˆdrte(3.88)(1.08)priGPA1(?)ACT,where,foremphasis,weletdenoteanFn680,R2.291,randomvariablewith(q,nk1)degreesoffreedom,andFistheactualwhere,asalways,standarderrorsareinparentheses;thestandardvalueoftheteststatistic.Thep-valuestillerrorforACTismissinginthesecondequation.Whatisthetstatis-ticforthecoefficientonACT?(Hint:FirstcomputetheFstatisticforhasthesameinterpretationasitdidfortsignificanceofACT.)statistics:itistheprobabilityofobserving147\nd7/14/995:15PMPage148Part1RegressionAnalysiswithCross-SectionalDataavalueoftheFatleastaslargeaswedid,giventhatthenullhypothesisistrue.Asmallp-valueisevidenceagainstH0.Forexample,p-value.016meansthatthechanceofobservingavalueofFaslargeaswedidwhenthenullhypothesiswastrueisonly1.6%;weusuallyrejectH0insuchcases.Ifthep-value.314,thenthechanceofobservingavalueoftheFstatisticaslargeaswedidunderthenullhypothesisis31.4%.MostwouldfindthistobeprettyweakevidenceagainstH0.Aswithttesting,oncethep-valuehasbeencomputed,theFtestcanbecarriedoutatanysignificancelevel.Forexample,ifthep-value.024,werejectH0atthe5%sig-nificancelevelbutnotatthe1%level.Thep-valuefortheFtestinExample4.9is.238,andsothenullhypothesisthatmotheducandfatheducarebothzeroisnotrejectedateventhe20%significancelevel.Manyeconometricspackageshaveabuilt-infeaturefortestingmultipleexclusionrestrictions.Thesepackageshaveseveraladvantagesovercalculatingthestatisticsbyhand:wewilllesslikelymakeamistake,p-valuesarecomputedautomatically,andtheproblemofmissingdata,asinExample4.9,ishandledwithoutanyadditionalworkonourpart.TheFStatisticforOverallSignificanceofaRegressionAspecialsetofexclusionrestrictionsisroutinelytestedbymostregressionpackages.Theserestrictionshavethesameinterpretation,regardlessofthemodel.Inthemodelwithkindependentvariables,wecanwritethenullhypothesisasH0:x1,x2,…,xkdonothelptoexplainy.Thisnullhypothesisis,inaway,verypessimistic.Itstatesthatnoneoftheexplanatoryvariableshasaneffectony.Statedintermsoftheparameters,thenullisthatallslopeparametersarezero:H0:12…k0,(4.44)andthealternativeisthatatleastoneofthejisdifferentfromzero.AnotherusefulwayofstatingthenullisthatH0:E(yx1,x2,…,xk)E(y),sothatknowingthevaluesofx1,x2,…,xkdoesnotaffecttheexpectedvalueofy.Therearekrestrictionsin(4.44),andwhenweimposethem,wegettherestrictedmodely0u;(4.45)allindependentvariableshavebeendroppedfromtheequation.Now,theR-squaredfromestimating(4.45)iszero;noneofthevariationinyisbeingexplainedbecausetherearenoexplanatoryvariables.Therefore,theFstatisticfortesting(4.44)canbewrittenas2R/k,(4.46)2(1R)/(nk1)2whereRisjusttheusualR-squaredfromtheregressionofyonx1,x2,…,xk.148\nd7/14/995:15PMPage149Chapter4MultipleRegressionAnalysis:InferenceMostregressionpackagesreporttheFstatisticin(4.46)automatically,whichmakesittemptingtousethisstatistictotestgeneralexclusionrestrictions.Youmustavoidthistemptation.TheFstatisticin(4.41)isusedforgeneralexclusionrestrictions;itdependsontheR-squaredsfromtherestrictedandunrestrictedmodels.Thespecialformof(4.46)isvalidonlyfortestingjointexclusionofallindependentvariables.Thisissome-timescalledtestingtheoverallsignificanceoftheregression.Ifwefailtoreject(4.44),thenthereisnoevidencethatanyoftheindependentvari-ableshelptoexplainy.Thisusuallymeansthatwemustlookforothervariablestoexplainy.ForExample4.9,theFstatisticfortesting(4.44)isabout9.55withk5andnk11,185df.Thep-valueiszerotofourplacesafterthedecimalpoint,sothat(4.44)isrejectedverystrongly.Thus,weconcludethatthevariablesinthebwghtequationdoexplainsomevariationinbwght.Theamountexplainedisnotlarge:only3.87%.ButtheseeminglysmallR-squaredresultsinahighlysignificantFstatis-tic.ThatiswhywemustcomputetheFstatistictotestforjointsignificanceandnotjustlookatthesizeoftheR-squared.Occasionally,theFstatisticforthehypothesisthatallindependentvariablesarejointlyinsignificantisthefocusofastudy.Problem4.10asksyoutousestockreturndatatotestwhetherstockreturnsoverafour-yearhorizonarepredictablebasedoninformationknownonlyatthebeginningoftheperiod.Undertheefficientmarketshypothesis,thereturnsshouldnotbepredictable;thenullhypothesisisprecisely(4.44).TestingGeneralLinearRestrictionsTestingexclusionrestrictionsisbyfarthemostimportantapplicationofFstatistics.Sometimes,however,therestrictionsimpliedbyatheoryaremorecomplicatedthanjustexcludingsomeindependentvariables.ItisstillstraightforwardtousetheFstatis-ticfortesting.Asanexample,considerthefollowingequation:log(price)01log(assess)2log(lotsize)(4.47)3log(sqrft)4bdrmsu,wherepriceishouseprice,assessistheassessedhousingvalue(beforethehousewassold),lotsizeissizeofthelot,infeet,sqrftissquarefootage,andbdrmsisnumberofbedrooms.Now,supposewewouldliketotestwhethertheassessedhousingpriceisarationalvaluation.Ifthisisthecase,thena1%changeinassessshouldbeassociatedwitha1%changeinprice;thatis,11.Inaddition,lotsize,sqrft,andbdrmsshouldnothelptoexplainlog(price),oncetheassessedvaluehasbeencontrolledfor.Together,thesehypothesescanbestatedasH0:11,20,30,40.(4.48)Therearefourrestrictionsheretobetested;threeareexclusionrestrictions,but11isnot.HowcanwetestthishypothesisusingtheFstatistic?Asintheexclusionrestrictioncase,weestimatetheunrestrictedmodel,(4.47)inthiscase,andthenimposetherestrictionsin(4.48)toobtaintherestrictedmodel.Itis149\nd7/14/995:15PMPage150Part1RegressionAnalysiswithCross-SectionalDatathesecondstepthatcanbealittletricky.Butallwedoisplugintherestrictions.Ifwewrite(4.47)asy01x12x23x34x4u,(4.49)thentherestrictedmodelisy0x1u.Now,inordertoimposetherestrictionthatthecoefficientonx1isunity,wemustestimatethefollowingmodel:yx10u.(4.50)Thisisjustamodelwithanintercept(0)butwithadifferentdependentvariablethanin(4.49).TheprocedureforcomputingtheFstatisticisthesame:estimate(4.50),obtaintheSSR(SSRr),andusethiswiththeunrestrictedSSRfrom(4.49)intheFsta-tistic(4.37).Wearetestingq4restrictions,andtherearen5dfintheunrestrictedmodel.TheFstatisticissimply[(SSRrSSRur)/SSRur][(n5)/4].Beforeillustratingthistestusingadataset,wemustemphasizeonepoint:wecan-notusetheR-squaredformoftheFstatisticforthisexamplebecausethedependentvariablein(4.50)isdifferentfromtheonein(4.49).Thismeansthetotalsumofsquaresfromthetworegressionswillbedifferent,and(4.41)isnolongerequivalentto(4.37).Asageneralrule,theSSRformoftheFstatisticshouldbeusedifadifferentdepen-dentvariableisneededinrunningtherestrictedregression.TheestimatedunrestrictedmodelusingthedatainHPRICE1.RAWislog(prˆice)(.034)(1.043)log(assess)(.0074)log(lotsize)log(prˆice)(.972)(.151)log(assess)(.0386)log(lotsize)log(prˆice)()(.1032)log(sqrft)(.0338)bdrmslog(prˆice)()(.1384)log(sqrft)(.0221)bdrms2n88,SSR1.822,R.773.Ifweuseseparatetstatisticstotesteachhypothesisin(4.48),wefailtorejecteachone.Butrationalityoftheassessmentisajointhypothesis,soweshouldtesttherestrictionsjointly.TheSSRfromtherestrictedmodelturnsouttobeSSRr1.880,andsotheFstatisticis[(1.8801.822)/1.822](83/4).661.The5%criticalvalueinanFdistri-butionwith(4,83)dfisabout2.50,andsowefailtorejectH0.Thereisessentiallynoevidenceagainstthehypothesisthattheassessedvaluesarerational.4.6REPORTINGREGRESSIONRESULTSWeendthischapterbyprovidingafewguidelinesonhowtoreportmultipleregressionresultsforrelativelycomplicatedempiricalprojects.Thisshouldteachyoutoreadpub-lishedworksintheappliedsocialsciences,whilealsopreparingyoutowriteyourownempiricalpapers.Wewillexpandonthistopicintheremainderofthetextbyreportingresultsfromvariousexamples,butmanyofthekeypointscanbemadenow.Naturally,theestimatedOLScoefficientsshouldalwaysbereported.Forthekeyvariablesinananalysis,youshouldinterprettheestimatedcoefficients(whichoftenrequiresknowingtheunitsofmeasurementofthevariables).Forexample,isanesti-150\nd7/14/995:15PMPage151Chapter4MultipleRegressionAnalysis:Inferencemateanelasticity,ordoesithavesomeotherinterpretationthatneedsexplanation?Theeconomicorpracticalimportanceoftheestimatesofthekeyvariablesshouldbedis-cussed.Thestandarderrorsshouldalwaysbeincludedalongwiththeestimatedcoeffi-cients.Someauthorsprefertoreportthetstatisticsratherthanthestandarderrors(andoftenjusttheabsolutevalueofthetstatistics).Whilenothingisreallywrongwiththis,thereissomepreferenceforreportingstandarderrors.First,itforcesustothinkcare-fullyaboutthenullhypothesisbeingtested;thenullisnotalwaysthatthepopulationparameteriszero.Second,havingstandarderrorsmakesiteasiertocomputeconfi-denceintervals.TheR-squaredfromtheregressionshouldalwaysbeincluded.Wehaveseenthat,inadditiontoprovidingagoodness-of-fitmeasure,itmakescalculationofFstatisticsforexclusionrestrictionssimple.Reportingthesumofsquaredresidualsandthestan-darderroroftheregressionissometimesagoodidea,butitisnotcrucial.Thenumberofobservationsusedinestimatinganyequationshouldappearneartheestimatedequa-tion.Ifonlyacoupleofmodelsarebeingestimated,theresultscanbesummarizedinequationform,aswehavedoneuptothispoint.However,inmanypapers,severalequationsareestimatedwithmanydifferentsetsofindependentvariables.Wemayesti-matethesameequationfordifferentgroupsofpeople,orevenhaveequationsexplain-ingdifferentdependentvariables.Insuchcases,itisbettertosummarizetheresultsinoneormoretables.Thedependentvariableshouldbeindicatedclearlyinthetable,andtheindependentvariablesshouldbelistedinthefirstcolumn.Standarderrors(ortsta-tistics)canbeputinparenthesesbelowtheestimates.EXAMPLE4.10(Salary-PensionTradeoffforTeachers)Lettotcompdenoteaveragetotalannualcompensationforateacher,includingsalaryandallfringebenefits(pension,healthinsurance,andsoon).Extendingthestandardwageequation,totalcompensationshouldbeafunctionofproductivityandperhapsotherchar-acteristics.Asisstandard,weuselogarithmicform:log(totcomp)f(productivitycharacteristics,otherfactors),wheref()issomefunction(unspecifiedfornow).Writebenefitstotcompsalarybenefitssalary1.salaryThisequationshowsthattotalcompensationistheproductoftwoterms:salaryand1b/s,whereb/sisshorthandforthe“benefitstosalaryratio.”Takingthelogofthisequa-tiongiveslog(totcomp)log(salary)log(1b/s).Now,for“small”b/s,log(1b/s)b/s;wewillusethisapproximation.Thisleadstotheeconometricmodellog(salary)01(b/s)otherfactors.Testingthewage-benefitstradeoffthenisthesameasatestofH0:11againstH1:11.151\nd7/14/995:15PMPage152Part1RegressionAnalysiswithCross-SectionalDataWeusethedatainMEAP93.RAWtotestthishypothesis.Thesedataareaveragedattheschoollevel,andwedonotobserveverymanyotherfactorsthatcouldaffecttotalcompensation.Wewillincludecontrolsforsizeoftheschool(enroll),staffperthousandstudents(staff),andmeasuressuchastheschooldropoutandgraduationrates.Theaver-ageb/sinthesampleisabout.205,andthelargestvalueis.450.TheestimatedequationsaregiveninTable4.1,wherestandarderrorsaregiveninparenthesesbelowthecoefficientestimates.Thekeyvariableisb/s,thebenefits-salaryratio.FromthefirstcolumninTable4.1,weseethat,withoutcontrollingforanyotherfactors,theOLScoefficientforb/sis.825.Thetstatisticfortestingthenullhypoth-esisH0:11ist(.8251)/.200.875,andsothesimpleregressionfailstorejectH0.Afteraddingcontrolsforschoolsizeandstaffsize(whichroughlycap-turesthenumberofstudentstaughtbyeachteacher),theestimateoftheb/scoef-Table4.1TestingtheSalary-BenefitsTradeoffDependentVariable:log(salary)IndependentVariables(1)(2)(3)b/s.825.605.589(.200)(.165)(.165)log(enroll)—.0874.0881(.0073)(.0073)log(staff)—.222.218(.050)(.050)droprate——.00028(.00161)gradrate——.00097(.00066)intercept10.52310.88410.738(0.042)(0.252)(0.258)Observations408408408R-Squared.040.353.361152\nd7/14/995:15PMPage153Chapter4MultipleRegressionAnalysis:Inferenceficientbecomes.605.NowthetestofQUESTION4.611givesatstatisticofabout2.39;Howdoesaddingdroprateandgradrateaffecttheestimateofthethus,H0isrejectedatthe5%levelagainstsalary-benefitstradeoff?Arethesevariablesjointlysignificantattheatwo-sidedalternative.Thevariables5%level?Whataboutthe10%level?log(enroll)andlog(staff)areverystatisti-callysignificant.SUMMARYInthischapter,wehavecoveredtheveryimportanttopicofstatisticalinference,whichallowsustoinfersomethingaboutthepopulationmodelfromarandomsample.Wesummarizethemainpoints:1.UndertheclassicallinearmodelassumptionsMLR.1throughMLR.6,theOLSestimatorsarenormallydistributed.2.UndertheCLMassumptions,thetstatisticshavetdistributionsunderthenullhypothesis.3.Weusetstatisticstotesthypothesesaboutasingleparameteragainstone-ortwo-sidedalternatives,usingone-ortwo-tailedtests,respectively.ThemostcommonnullhypothesisisH0:j0,butwesometimeswanttotestothervaluesofjunderH0.4.Inclassicalhypothesistesting,wefirstchooseasignificancelevel,which,alongwiththedfandalternativehypothesis,determinesthecriticalvalueagainstwhichwecomparethetstatistic.Itismoreinformativetocomputethep-valueforattest—thesmallestsignificancelevelforwhichthenullhypothesisisrejected—sothatthehypothesiscanbetestedatanysignificancelevel.5.UndertheCLMassumptions,confidenceintervalscanbeconstructedforeachj.TheseCIscanbeusedtotestanynullhypothesisconcerningjagainstatwo-sidedalternative.6.Singlehypothesistestsconcerningmorethanonejcanalwaysbetestedbyrewritingthemodeltocontaintheparameterofinterest.Then,astandardtstatis-ticcanbeused.7.TheFstatisticisusedtotestmultipleexclusionrestrictions,andtherearetwoequivalentformsofthetest.OneisbasedontheSSRsfromtherestrictedandunrestrictedmodels.AmoreconvenientformisbasedontheR-squaredsfromthetwomodels.8.WhencomputinganFstatistic,thenumeratordfisthenumberofrestrictionsbeingtested,whilethedenominatordfisthedegreesoffreedomintheunrestrictedmodel.9.ThealternativeforFtestingistwo-sided.Intheclassicalapproach,wespecifyasignificancelevelwhich,alongwiththenumeratordfandthedenominatordf,determinesthecriticalvalue.Thenullhypothesisisrejectedwhenthestatistic,F,exceedsthecriticalvalue,c.Alternatively,wecancomputeap-valuetosumma-rizetheevidenceagainstH0.10.Generalmultiplelinearrestrictionscanbetestedusingthesumofsquaredresid-ualsformoftheFstatistic.153\nd7/14/995:15PMPage154Part1RegressionAnalysiswithCross-SectionalData11.TheFstatisticfortheoverallsignificanceofaregressionteststhenullhypothesisthatallslopeparametersarezero,withtheinterceptunrestricted.UnderH0,theexplanatoryvariableshavenoeffectontheexpectedvalueofy.KEYTERMSAlternativeHypothesisNumeratorDegreesofFreedomClassicalLinearModelOne-SidedAlternativeClassicalLinearModel(CLM)One-TailedTestAssumptionsOverallSignificanceoftheRegressionConfidenceInterval(CI)p-ValueCriticalValuePracticalSignificanceDenominatorDegreesofFreedomR-squaredFormoftheFStatisticEconomicSignificanceRejectionRuleExclusionRestrictionsRestrictedModelFStatisticSignificanceLevelJointHypothesesTestStatisticallyInsignificantJointlyInsignificantStatisticallySignificantJointlyStatisticallySignificanttRatioMinimumVarianceUnbiasedEstimatorstStatisticMultipleHypothesesTestTwo-SidedAlternativeMultipleRestrictionsTwo-TailedTestNormalityAssumptionUnrestrictedModelNullHypothesisPROBLEMS4.1WhichofthefollowingcancausetheusualOLStstatisticstobeinvalid(thatis,nottohavetdistributionsunderH0)?(i)Heteroskedasticity.(ii)Asamplecorrelationcoefficientof.95betweentwoindependentvari-ablesthatareinthemodel.(iii)Omittinganimportantexplanatoryvariable.4.2ConsideranequationtoexplainsalariesofCEOsintermsofannualfirmsales,returnonequity(roe,inpercentform),andreturnonthefirm’sstock(ros,inpercentform):log(salary)01log(sales)2roe3rosu.(i)Intermsofthemodelparameters,statethenullhypothesisthat,aftercon-trollingforsalesandroe,roshasnoeffectonCEOsalary.Statethealter-nativethatbetterstockmarketperformanceincreasesaCEO’ssalary.(ii)UsingthedatainCEOSAL1.RAW,thefollowingequationwasobtainedbyOLS:154\nd7/14/995:15PMPage155Chapter4MultipleRegressionAnalysis:Inferencelog(saˆlary)(4.32)(.280)log(sales)(.0174)roe(.00024)roslog(saˆlary)(0.32)(.035)log(sales)(.0041)roe(.00054)ros2n209,R.283Bywhatpercentissalarypredictedtoincrease,ifrosincreasesby50points?Doesroshaveapracticallylargeeffectonsalary?(iii)Testthenullhypothesisthatroshasnoeffectonsalary,againstthealternativethatroshasapositiveeffect.Carryoutthetestatthe10%significancelevel.(iv)WouldyouincluderosinafinalmodelexplainingCEOcompensationintermsoffirmperformance?Explain.4.3Thevariablerdintensisexpendituresonresearchanddevelopment(R&D)asapercentageofsales.Salesaremeasuredinmillionsofdollars.Thevariableprofmargisprofitsasapercentageofsales.UsingthedatainRDCHEM.RAWfor32firmsinthechemicalindustry,thefol-lowingequationisestimated:rdinˆtens(.472)(.321)log(sales)(.050)profmargrdinˆtens(1.369)(.216)log(sales)(.046)profmarg2n32,R.099(i)Interpretthecoefficientonlog(sales).Inparticular,ifsalesincreasesby10%,whatistheestimatedpercentagepointchangeinrdintens?Isthisaneconomicallylargeeffect?(ii)TestthehypothesisthatR&Dintensitydoesnotchangewithsales,againstthealternativethatitdoesincreasewithsales.Dothetestatthe5%and10%levels.(iii)Doesprofmarghaveastatisticallysignificanteffectonrdintens?4.4Arerentratesinfluencedbythestudentpopulationinacollegetown?LetrentbetheaveragemonthlyrentpaidonrentalunitsinacollegetownintheUnitedStates.Letpopdenotethetotalcitypopulation,avginctheaveragecityincome,andpctstuthestudentpopulationasapercentofthetotalpopulation.Onemodeltotestforarela-tionshipislog(rent)01log(pop)2log(avginc)3pctstuu.(i)Statethenullhypothesisthatsizeofthestudentbodyrelativetothepopulationhasnoceterisparibuseffectonmonthlyrents.Statethealternativethatthereisaneffect.(ii)Whatsignsdoyouexpectfor1and2?(iii)Theequationestimatedusing1990datafromRENTAL.RAWfor64collegetownsislog(ˆrent)(.043)(.066)log(pop)(.507)log(avginc)(.0056)pctstulog(ˆrent)(.844)(.039)log(pop)(.081)log(avginc)(.0017)pctstu2n64,R.458.155\nd7/14/995:15PMPage156Part1RegressionAnalysiswithCross-SectionalDataWhatiswrongwiththestatement:“A10%increaseinpopulationisassociatedwithabouta6.6%increaseinrent”?(iv)Testthehypothesisstatedinpart(i)atthe1%level.4.5ConsidertheestimatedequationfromExample4.3,whichcanbeusedtostudytheeffectsofskippingclassoncollegeGPA:colˆGPA(1.39)(.412)hsGPA(.015)ACT(.083)skippedcolˆGPA(0.33)(.094)hsGPA(.011)ACT(.026)skipped2n141,R.234.(i)Usingthestandardnormalapproximation,findthe95%confidenceintervalforhsGPA.(ii)CanyourejectthehypothesisH0:hsGPA.4againstthetwo-sidedalternativeatthe5%level?(iii)CanyourejectthehypothesisH0:hsGPA1againstthetwo-sidedalternativeatthe5%level?4.6InSection4.5,weusedasanexampletestingtherationalityofassessmentsofhousingprices.There,weusedalog-logmodelinpriceandassess[seeequation(4.47)].Here,weusealevel-levelformulation.(i)Inthesimpleregressionmodelprice01assessu,theassessmentisrationalif11and00.Theestimatedequa-tionis(priˆce14.47)(.976)assesspriˆce(16.27)(.049)assess2n88,SSR165,644.51,R.820.First,testthehypothesisthatH0:00againstthetwo-sidedalterna-tive.Then,testH0:11againstthetwo-sidedalternative.Whatdoyouconclude?(ii)Totestthejointhypothesisthat00and11,weneedtheSSRinn2therestrictedmodel.Thisamountstocomputing(priceiassessi),i1wheren88,sincetheresidualsintherestrictedmodelarejustpriceiassessi.(NoestimationisneededfortherestrictedmodelbecausebothparametersarespecifiedunderH0.)ThisturnsouttoyieldSSR209,448.99.CarryouttheFtestforthejointhypothesis.(iii)NowtestH0:20,30,and40inthemodelprice01assess2sqrft3lotsize4bdrmsu.TheR-squaredfromestimatingthismodelusingthesame88housesis.829.(iv)Ifthevarianceofpricechangeswithassess,sqrft,lotsize,orbdrms,whatcanyousayabouttheFtestfrompart(iii)?156\nd7/14/995:15PMPage157Chapter4MultipleRegressionAnalysis:Inference4.7InExample4.7,weuseddataonMichiganmanufacturingfirmstoestimatetherelationshipbetweenthescraprateandotherfirmcharacteristics.Wenowlookatthisexamplemorecloselyandusealargersampleoffirms.(i)ThepopulationmodelestimatedinExample4.7canbewrittenaslog(scrap)01hrsemp2log(sales)3log(employ)u.Usingthe43observationsavailablefor1987,theestimatedequationislog(sˆcrap)(11.74)(.042)hrsemp(.951)log(sales)(.992)log(employ)log(sˆcrap)(4.57)(.019)hrsemp(.370)log(sales)(.360)log(employ)2n43,R.310.Comparethisequationtothatestimatedusingonly30firmsinthesample.(ii)Showthatthepopulationmodelcanalsobewrittenaslog(scrap)01hrsemp2log(sales/employ)3log(employ)u,where323.[Hint:Recallthatlog(x2/x3)log(x2)log(x3).]InterpretthehypothesisH0:30.(iii)Whentheequationfrompart(ii)isestimated,weobtainlog(sˆcrap)(11.74)(.042)hrsemp(.951)log(sales/employ)(.041)log(employ)log(sˆcrap)(4.57)(.019)hrsemp(.370)log(sales/employ)(.205)log(employ)2n43,R.310.Controllingforworkertrainingandforthesales-to-employeeratio,dobiggerfirmshavelargerstatisticallysignificantscraprates?(iv)Testthehypothesisthata1%increaseinsales/employisassociatedwitha1%dropinthescraprate.4.8Considerthemultipleregressionmodelwiththreeindependentvariables,undertheclassicallinearmodelassumptionsMLR.1throughMLR.6:y01x12x23x3u.YouwouldliketotestthenullhypothesisH0:1321.(i)Letˆ1andˆ2denotetheOLSestimatorsof1and2.FindVar(ˆ13ˆ2)intermsofthevariancesofˆ1andˆ2andthecovariancebetweenthem.Whatisthestandarderrorofˆ13ˆ2?(ii)WritethetstatisticfortestingH0:1321.(iii)Define1132andˆ1ˆ13ˆ2.Writearegressionequationinvolving0,1,2,and3thatallowsyoutodirectlyobtainˆ1anditsstandarderror.4.9InProblem3.3,weestimatedtheequationsleˆep(3,638.25)(.148)totwrk(11.13)educ(2.20)agesleˆep3,(112.28)(.017)totwrk(5.88)educ(1.45)age2n706,R.113,157\nd7/14/995:15PMPage158Part1RegressionAnalysiswithCross-SectionalDatawherewenowreportstandarderrorsalongwiththeestimates.(i)Iseithereducorageindividuallysignificantatthe5%levelagainstatwo-sidedalternative?Showyourwork.(ii)Droppingeducandagefromtheequationgivessleˆep(3,586.38)(.151)totwrksleˆep3,(38.91)(.017)totwrk2n706,R.103.Areeducandagejointlysignificantintheoriginalequationatthe5%level?Justifyyouranswer.(iii)Doesincludingeducandageinthemodelgreatlyaffecttheestimatedtradeoffbetweensleepingandworking?(iv)Supposethatthesleepequationcontainsheteroskedasticity.Whatdoesthismeanaboutthetestscomputedinparts(i)and(ii)?4.10Regressionanalysiscanbeusedtotestwhetherthemarketefficientlyusesinfor-mationinvaluingstocks.Forconcreteness,letreturnbethetotalreturnfromholdingafirm’sstockoverthefour-yearperiodfromtheendof1990totheendof1994.Theefficientmarketshypothesissaysthatthesereturnsshouldnotbesystematicallyrelatedtoinformationknownin1990.Iffirmcharacteristicsknownatthebeginningoftheperiodhelptopredictstockreturns,thenwecouldusethisinformationinchoosingstocks.For1990,letdkrbeafirm’sdebttocapitalratio,letepsdenotetheearningspershare,let(log)netincdenotenetincome,andlet(log)salarydenotetotalcompensationfortheCEO.(i)UsingthedatainRETURN.RAW,thefollowingequationwasesti-mated:retˆurn(40.44)(.952)dkr(.472)eps(.025)netinc(.003)salaryretˆurn(29.30)(.854)dkr(.332)eps(.020)netinc(.009)salary2n142,R.0285.Testwhethertheexplanatoryvariablesarejointlysignificantatthe5%level.Isanyexplanatoryvariableindividuallysignificant?(ii)Nowreestimatethemodelusingthelogformfornetincandsalary:(retˆurn69.12)(1.056)dkr(.586)eps(31.18)netinc(39.26)salaryretˆurn(164.66)(.847)dkr(.336)eps(14.16)netinc(26.40)salary2n142,R.0531.Doanyofyourconclusionsfrompart(i)change?(iii)Overall,istheevidenceforpredictabilityofstockreturnsstrongorweak?4.11ThefollowingtablewascreatedusingthedatainCEOSAL2.RAW:158\nd7/14/995:15PMPage159Chapter4MultipleRegressionAnalysis:InferenceDependentVariable:log(salary)IndependentVariables(1)(2)(3)log(sales).224.158.188(.027)(.040)(.040)log(mktval)—.112.100(.050)(.049)profmarg—.0023.0022(.0022)(.0021)ceoten——.0171(.0055)comten——.0092(.0033)intercept4.944.624.57(0.20)(0.25)(0.25)Observations177177177R-Squared.281.304.353Thevariablemktvalismarketvalueofthefirm,profmargisprofitasapercentageofsales,ceotenisyearsasCEOwiththecurrentcompany,andcomtenistotalyearswiththecompany.(i)CommentontheeffectofprofmargonCEOsalary.(ii)Doesmarketvaluehaveasignificanteffect?Explain.(iii)Interpretthecoefficientsonceotenandcomten.Arethevariablessta-tisticallysignificant?Whatdoyoumakeofthefactthatlongertenurewiththecompany,holdingtheotherfactorsfixed,isassociatedwithalowersalary?COMPUTEREXERCISES4.12Thefollowingmodelcanbeusedtostudywhethercampaignexpendituresaffectelectionoutcomes:voteA01log(expendA)2log(expendB)3prtystrAu,wherevoteAisthepercentofthevotereceivedbyCandidateA,expendAandexpendBarecampaignexpendituresbyCandidatesAandB,andprtystrAisameasureofparty159\nd7/14/995:15PMPage160Part1RegressionAnalysiswithCross-SectionalDatastrengthforCandidateA(thepercentofthemostrecentpresidentialvotethatwenttoA’sparty).(i)Whatistheinterpretationof1?(ii)Intermsoftheparameters,statethenullhypothesisthata1%increaseinA’sexpendituresisoffsetbya1%increaseinB’sexpenditures.(iii)EstimatethemodelaboveusingthedatainVOTE1.RAWandreporttheresultsinusualform.DoA’sexpendituresaffecttheoutcome?WhataboutB’sexpenditures?Canyouusetheseresultstotestthehypothe-sisinpart(ii)?(iv)Estimateamodelthatdirectlygivesthetstatisticfortestingthehypoth-esisinpart(ii).Whatdoyouconclude?(Useatwo-sidedalternative.)4.13UsethedatainLAWSCH85.RAWforthisexercise.(i)UsingthesamemodelasProblem3.4,stateandtestthenullhypothe-sisthattherankoflawschoolshasnoceterisparibuseffectonmedianstartingsalary.(ii)Arefeaturesoftheincomingclassofstudents—namely,LSATandGPA—individuallyorjointlysignificantforexplainingsalary?(iii)Testwhetherthesizeoftheenteringclass(clsize)orthesizeofthefac-ulty(faculty)needtobeaddedtothisequation;carryoutasingletest.(Becarefultoaccountformissingdataonclsizeandfaculty.)(iv)Whatfactorsmightinfluencetherankofthelawschoolthatarenotincludedinthesalaryregression?4.14RefertoProblem3.14.Now,usethelogofthehousingpriceasthedependentvariable:log(price)01sqrft2bdrmsu.(i)Youareinterestedinestimatingandobtainingaconfidenceintervalforthepercentagechangeinpricewhena150-square-footbedroomisaddedtoahouse.Indecimalform,thisis115012.UsethedatainHPRICE1.RAWtoestimate1.(ii)Write2intermsof1and1andplugthisintothelog(price)equation.(iii)Usepart(ii)toobtainastandarderrorforˆ1andusethisstandarderrortoconstructa95%confidenceinterval.4.15InExample4.9,therestrictedversionofthemodelcanbeestimatedusingall1,388observationsinthesample.ComputetheR-squaredfromtheregressionofbwghtoncigs,parity,andfamincusingallobservations.ComparethistotheR-squaredreportedfortherestrictedmodelinExample4.9.4.16UsethedatainMLB1.RAWforthisexercise.(i)Usethemodelestimatedinequation(4.31)anddropthevariablerbisyr.Whathappenstothestatisticalsignificanceofhrunsyr?Whataboutthesizeofthecoefficientonhrunsyr?(ii)Addthevariablesrunsyr,fldperc,andsbasesyrtothemodelfrompart(i).Whichofthesefactorsareindividuallysignificant?(iii)Inthemodelfrompart(ii),testthejointsignificanceofbavg,fldperc,andsbasesyr.160\nd7/14/995:15PMPage161Chapter4MultipleRegressionAnalysis:Inference4.17UsethedatainWAGE2.RAWforthisexercise.(i)Considerthestandardwageequationlog(wage)01educ2exper3tenureu.Statethenullhypothesisthatanotheryearofgeneralworkforceexperi-encehasthesameeffectonlog(wage)asanotheryearoftenurewiththecurrentemployer.(ii)Testthenullhypothesisinpart(i)againstatwo-sidedalternative,atthe5%significancelevel,byconstructinga95%confidenceinterval.Whatdoyouconclude?161\n\nd7/14/995:21PMPage162ChapterFiveMultipleRegressionAnalysis:OLSAsymptoticsnChapters3and4,wecoveredwhatarecalledfinitesample,smallsample,orexactIpropertiesoftheOLSestimatorsinthepopulationmodely01x12x2…kxku.(5.1)Forexample,theunbiasednessofOLS(derivedinChapter3)underthefirstfourGauss-Markovassumptionsisafinitesamplepropertybecauseitholdsforanysamplesizen(subjecttothemildrestrictionthatnmustbeatleastaslargeasthetotalnumberofparametersintheregressionmodel,k1).Similarly,thefactthatOLSisthebestlin-earunbiasedestimatorunderthefullsetofGauss-Markovassumptions(MLR.1throughMLR.5)isafinitesampleproperty.InChapter4,weaddedtheclassicallinearmodelAssumptionMLR.6,whichstatesthattheerrortermuisnormallydistributedandindependentoftheexplanatoryvari-ables.ThisallowedustoderivetheexactsamplingdistributionsoftheOLSestimators(conditionalontheexplanatoryvariablesinthesample).Inparticular,Theorem4.1showedthattheOLSestimatorshavenormalsamplingdistributions,whichleddirectlytothetandFdistributionsfortandFstatistics.Iftheerrorisnotnormallydistributed,thedistributionofatstatisticisnotexactlyt,andanFstatisticdoesnothaveanexactFdistributionforanysamplesize.Inadditiontofinitesampleproperties,itisimportanttoknowtheasymptoticprop-ertiesorlargesamplepropertiesofestimatorsandteststatistics.Thesepropertiesarenotdefinedforaparticularsamplesize;rather,theyaredefinedasthesamplesizegrowswithoutbound.Fortunately,undertheassumptionswehavemade,OLShassatisfactorylargesampleproperties.Onepracticallyimportantfindingisthatevenwithoutthenormalityassumption(AssumptionMLR.6),tandFstatisticshaveapproximatelytandFdistributions,atleastinlargesamplesizes.WediscussthisinmoredetailinSection5.2,afterwecoverconsistencyofOLSinSection5.1.5.1CONSISTENCYUnbiasednessofestimators,whileimportant,cannotalwaysbeachieved.Forexample,aswediscussedinChapter3,thestandarderroroftheregression,ˆ,isnotanunbiased162\nd7/14/995:21PMPage163Chapter5MultipleRegressionAnalysis:OLSAsymptoticsestimatorfor,thestandarddeviationoftheerroruinamultipleregressionmodel.WhiletheOLSestimatorsareunbiasedunderMLR.1throughMLR.4,inChapter11wewillfindthattherearetimeseriesregressionswheretheOLSestimatorsarenotunbi-ased.Further,inPart3ofthetext,weencounterseveralotherestimatorsthatarebiased.Whilenotallusefulestimatorsareunbiased,virtuallyalleconomistsagreethatconsistencyisaminimalrequirementforanestimator.ThefamouseconometricianCliveW.J.Grangeronceremarked:“Ifyoucan’tgetitrightasngoestoinfinity,youshouldn’tbeinthisbusiness.”Theimplicationisthat,ifyourestimatorofaparticularpopulationparameterisnotconsistent,thenyouarewastingyourtime.Thereareafewdifferentwaystodescribeconsistency.FormaldefinitionsandresultsaregiveninAppendixC;herewefocusonanintuitiveunderstanding.Forcon-creteness,letˆjbetheOLSestimatorofjforsomej.Foreachn,ˆjhasaprobabilitydistribution(representingitspossiblevaluesindifferentrandomsamplesofsizen).BecauseˆjisunbiasedunderassumptionsMLR.1throughMLR.4,thisdistributionhasmeanvaluej.Ifthisestimatorisconsistent,thenthedistributionofˆjbecomesmoreandmoretightlydistributedaroundjasthesamplesizegrows.Asntendstoinfinity,thedistributionofˆjcollapsestothesinglepointj.Ineffect,thismeansthatwecanmakeourestimatorarbitrarilyclosetojifwecancollectasmuchdataaswewant.ThisconvergenceisillustratedinFigure5.1.Figure5.1Samplingdistributionsofˆ1forsamplesizesn1n2n3.n3fˆ1n2n11ˆ1163\nd7/14/995:21PMPage164Part1RegressionAnalysiswithCross-SectionalDataNaturally,foranyapplicationwehaveafixedsamplesize,whichisthereasonanasymptoticpropertysuchasconsistencycanbedifficulttograsp.Consistencyinvolvesathoughtexperimentaboutwhatwouldhappenasthesamplesizegetslarge(whileatthesametimeweobtainnumerousrandomsamplesforeachsamplesize).Ifobtainingmoreandmoredatadoesnotgenerallygetusclosertotheparametervalueofinterest,thenweareusingapoorestimationprocedure.Conveniently,thesamesetofassumptionsimplybothunbiasednessandconsistencyofOLS.Wesummarizewithatheorem.THEOREM5.1(CONSISTENCYOFOLS)UnderassumptionsMLR.1throughMLR.4,theOLSestimatorˆjisconsistentforj,forallj0,1,…,k.Ageneralproofofthisresultismosteasilydevelopedusingthematrixalgebrameth-odsdescribedinAppendicesDandE.ButwecanproveTheorem5.1withoutdifficultyinthecaseofthesimpleregressionmodel.Wefocusontheslopeestimator,ˆ1.Theproofstartsoutthesameastheproofofunbiasedness:wewritedownthefor-mulaforˆ1,andthenpluginyi01xi1ui:nn2ˆ1(xi1x¯1)yi(xi1x¯1)i1i1(5.2)nn1121n(xi1x¯1)uin(xi1x¯1).i1i1Wecanapplythelawoflargenumberstothenumeratoranddenominator,whichcon-vergeinprobabilitytothepopulationquantities,Cov(x1,u)andVar(x1),respectively.ProvidedthatVar(x1)0—whichisassumedinMLR.4—wecanusethepropertiesofprobabilitylimits(seeAppendixC)togetplimˆ11Cov(x1,u)/Var(x1)(5.3)1,becauseCov(x1,u)0.Wehaveusedthefact,discussedinChapters2and3,thatE(ux1)0impliesthatx1anduareuncorrelated(havezerocovariance).Asatechnicalmatter,toensurethattheprobabilitylimitsexist,weshouldassumethatVar(x1)andVar(u)(whichmeansthattheirprobabilitydistributionsarenottoospreadout),butwewillnotworryaboutcaseswheretheseassumptionsmightfail.Thepreviousarguments,andequation(5.3)inparticular,showthatOLSisconsis-tentinthesimpleregressioncaseifweassumeonlyzerocorrelation.Thisisalsotrueinthegeneralcase.Wenowstatethisasanassumption.ASSUMPTIONMLR.3(ZEROMEANANDZEROCORRELATION)E(u)0andCov(xj,u)0,forj1,2,…,k.164\nd7/14/995:21PMPage165Chapter5MultipleRegressionAnalysis:OLSAsymptoticsInChapter3,wediscussedwhyassumptionMLR.3impliesMLR.3,butnotviceversa.ThefactthatOLSisconsistentundertheweakerassumptionMLR.3turnsouttobeusefulinChapter15andinothersituations.Interestingly,whileOLSisunbiasedunderMLR.3,thisisnotthecaseunderAssumptionMLR.3.(ThiswastheleadingreasonwehaveassumedMLR.3.)DerivingtheInconsistencyinOLSJustasfailureofE(ux1,…,xk)0causesbiasintheOLSestimators,correlationbetweenuandanyofx1,x2,…,xkgenerallycausesalloftheOLSestimatorstobeinconsistent.Thissimplebutimportantobservationisoftensummarizedas:iftheerroriscorrelatedwithanyoftheindependentvariables,thenOLSisbiasedandinconsis-tent.Thisisveryunfortunatebecauseitmeansthatanybiaspersistsasthesamplesizegrows.Inthesimpleregressioncase,wecanobtaintheinconsistencyfromequation(5.3),whichholdswhetherornotuandx1areuncorrelated.Theinconsistencyinˆ1(some-timeslooselycalledtheasymptoticbias)isplimˆ11Cov(x1,u)/Var(x1).(5.4)BecauseVar(x1)0,theinconsistencyinˆ1ispositiveifx1anduarepositivelycorre-lated,andtheinconsistencyisnegativeifx1anduarenegativelycorrelated.Ifthecovariancebetweenx1anduissmallrelativetothevarianceinx1,theinconsistencycanbenegligible;unfortunately,wecannotevenestimatehowbigthecovarianceisbecauseuisunobserved.Wecanuse(5.4)toderivetheasymptoticanalogoftheomittedvariablebias(seeTable3.2inChapter3).Supposethetruemodel,y01x12x2v,satisfiesthefirstfourGauss-Markovassumptions.Thenvhasazeromeanandisuncor-relatedwithx1andx2.Ifˆ0,ˆ1,andˆ2denotetheOLSestimatorsfromtheregressionofyonx1andx2,thenTheorem5.1impliesthattheseestimatorsareconsistent.Ifweomitx2fromtheregressionanddothesimpleregressionofyonx1,thenu2x2v.Let˜1denotethesimpleregressionslopeestimator.Thenplim˜1121(5.5)where1Cov(x1,x2)/Var(x1).(5.6)Thus,forpracticalpurposes,wecanviewtheinconsistencyasbeingthesameasthebias.Thedifferenceisthattheinconsistencyisexpressedintermsofthepopulationvarianceofx1andthepopulationcovariancebetweenx1andx2,whilethebiasisbasedontheirsamplecounterparts(becauseweconditiononthevaluesofx1andx2inthesample).165\nd7/14/995:21PMPage166Part1RegressionAnalysiswithCross-SectionalDataIfx1andx2areuncorrelated(inthepopulation),then10,and˜1isaconsistentestimatorof1(althoughnotnecessarilyunbiased).Ifx2hasapositivepartialeffectony,sothat20,andx1andx2arepositivelycorrelated,sothat10,thentheincon-sistencyin˜1ispositive.Andsoon.WecanobtainthedirectionoftheinconsistencyorasymptoticbiasfromTable3.2.Ifthecovariancebetweenx1andx2issmallrelativetothevarianceofx1,theinconsistencycanbesmall.EXAMPLE5.1(HousingPricesandDistancefromanIncinerator)Letydenotethepriceofahouse(price),letx1denotethedistancefromthehousetoanewtrashincinerator(distance),andletx2denotethe“quality”ofthehouse(quality).Thevariablequalityisleftvaguesothatitcanincludethingslikesizeofthehouseandlot,num-berofbedroomsandbathrooms,andintangiblessuchasattractivenessoftheneighbor-hood.Iftheincineratordepresseshouseprices,then1shouldbepositive:everythingelsebeingequal,ahousethatisfartherawayfromtheincineratorisworthmore.Bydefinition,2ispositivesincehigherqualityhousessellformore,otherfactorsbeingequal.Iftheincin-eratorwasbuiltfartheraway,onaverage,frombetterhomes,thendistanceandqualityarepositivelycorrelated,andso10.Asimpleregressionofpriceondistance[orlog(price)onlog(distance)]willtendtooverestimatetheeffectoftheincinerator:1211.AnimportantpointaboutinconsistencyinOLSestimatorsisthat,bydefinition,theproblemdoesnotgoawaybyaddingmoreobservationstothesample.Ifanything,theproblemgetsworsewithmoredata:theOLSestimatorgetscloserandclosertoQUESTION5.1121asthesamplesizegrows.SupposethatthemodelDerivingthesignandmagnitudeoftheinconsistencyinthegeneralkregressorscore01skipped2priGPAucaseismuchharder,justasderivingthesatisfiesthefirstfourGauss-Markovassumptions,wherescoreisbiasisverydifficult.Weneedtorememberscoreonafinalexam,skippedisnumberofclassesskipped,andthatifwehavethemodelinequation(5.1)priGPAisGPApriortothecurrentsemester.If˜1isfromthesimpleregressionofscoreonskipped,whatisthedirectionoftheasymp-where,say,x1iscorrelatedwithubutthetoticbiasin˜1?otherindependentvariablesareuncorre-latedwithu,alloftheOLSestimatorsaregenerallyinconsistent.Forexample,inthek2case,y01x12x2u,supposethatx2anduareuncorrelatedbutx1anduarecorrelated.ThentheOLSesti-matorsˆ1andˆ2willgenerallybothbeinconsistent.(Theinterceptwillalsobeincon-sistent.)Theinconsistencyinˆ2ariseswhenx1andx2arecorrelated,asisusuallythecase.Ifx1andx2areuncorrelated,thenanycorrelationbetweenx1andudoesnotresultintheinconsistencyofˆ2:plimˆ22.Further,theinconsistencyinˆ1isthesameasin(5.4).Thesamestatementholdsinthegeneralcase:ifx1iscorrelatedwithu,butx1anduareuncorrelatedwiththeotherindependentvariables,thenonlyˆ1isinconsis-tent,andtheinconsistencyisgivenby(5.4).166\nd7/14/995:21PMPage167Chapter5MultipleRegressionAnalysis:OLSAsymptotics5.2ASYMPTOTICNORMALITYANDLARGESAMPLEINFERENCEConsistencyofanestimatorisanimportantproperty,butitalonedoesnotallowustoperformstatisticalinference.Simplyknowingthattheestimatorisgettingclosertothepopulationvalueasthesamplesizegrowsdoesnotallowustotesthypothe-sesabouttheparameters.Fortesting,weneedthesamplingdistributionoftheOLSestimators.UndertheclassicallinearmodelassumptionsMLR.1throughMLR.6,Theorem4.1showsthatthesamplingdistributionsarenormal.ThisresultisthebasisforderivingthetandFdistributionsthatweusesoofteninappliedeconometrics.TheexactnormalityoftheOLSestimatorshingescruciallyonthenormalityofthedistributionoftheerror,u,inthepopulation.Iftheerrorsu1,u2,…,unareran-domdrawsfromsomedistributionotherthanthenormal,theˆjwillnotbenormallydistributed,whichmeansthatthetstatisticswillnothavetdistributionsandtheFsta-tisticswillnothaveFdistributions.Thisisapotentiallyseriousproblembecauseourinferencehingesonbeingabletoobtaincriticalvaluesorp-valuesfromthetorFdis-tributions.RecallthatAssumptionMLR.6isequivalenttosayingthatthedistributionofygivenx1,x2,…,xkisnormal.Sinceyisobservedanduisnot,inaparticularapplica-tion,itismucheasiertothinkaboutwhetherthedistributionofyislikelytobenor-mal.Infact,wehavealreadyseenafewexampleswhereydefinitelycannothaveanormaldistribution.Anormallydistributedrandomvariableissymmetricallydistrib-utedaboutitsmean,itcantakeonanypositiveornegativevalue(butwithzeroprob-ability),andmorethan95%oftheareaunderthedistributioniswithintwostandarddeviations.InExample3.4,weestimatedamodelexplainingthenumberofarrestsofyoungmenduringaparticularyear(narr86).Inthepopulation,mostmenarenotarrestedduringtheyear,andthevastmajorityarearrestedonetimeatthemost.(Inthesam-pleof2,725meninthedatasetCRIME1.RAW,fewerthan8%werearrestedmorethanonceduring1986.)Becausenarr86takesononlytwovaluesfor92%ofthesam-ple,itcannotbeclosetobeingnormallydistributedinthepopulation.InExample4.6,weestimatedamodelexplainingparticipationpercentages(prate)in401(k)pensionplans.Thefrequencydistribution(alsocalledahistogram)inFigure5.2showsthatthedistributionofprateisheavilyskewedtotheright,ratherthanbeingnormallydistributed.Infact,over40%oftheobservationsonprateareatthevalue100,indicating100%participation.Thisviolatesthenormalityassumptionevenconditionalontheexplanatoryvariables.WeknowthatnormalityplaysnoroleintheunbiasednessofOLS,nordoesitaffecttheconclusionthatOLSisthebestlinearunbiasedestimatorundertheGauss-Markovassumptions.ButexactinferencebasedontandFstatisticsrequiresMLR.6.Doesthismeanthat,inouranalysisofprateinExample4.6,wemustaban-donthetstatisticsfordeterminingwhichvariablesarestatisticallysignificant?Fortunately,theanswertothisquestionisno.Eventhoughtheyiarenotfromanor-maldistribution,wecanusethecentrallimittheoremfromAppendixCtoconcludethattheOLSestimatorsareapproximatelynormallydistributed,atleastinlargesamplesizes.167\nd7/14/995:21PMPage168Part1RegressionAnalysiswithCross-SectionalDataFigure5.2Histogramofprateusingthedatain401K.RAW..8.6.4Proportionincell.200102030405060708090100Participationrate(inpercentform)THEOREM5.2(ASYMPTOTICNORMALITYOFOLS)UndertheGauss-MarkovassumptionsMLR.1throughMLR.5,2222(i)n(ˆjj)~ªNormal(0,/aj),where/aj0istheasymptoticvarianceofnn(ˆ);fortheslopecoefficients,a2plimn1rˆ2,wheretherˆaretheresid-jjjijiji1ualsfromregressingxjontheotherindependentvariables.Wesaythatˆjisasymptoticallynormallydistributed(seeAppendixC);22(ii)ˆisaconsistentestimatorofVar(u);(iii)Foreachj,(ˆjj)/se(ˆj)~ªNormal(0,1),(5.7)wherese(ˆj)istheusualOLSstandarderror.168\nd7/14/995:21PMPage169Chapter5MultipleRegressionAnalysis:OLSAsymptoticsTheproofofasymptoticnormalityissomewhatcomplicatedandissketchedintheappendixforthesimpleregressioncase.Part(ii)followsfromthelawoflargenumbers,andpart(iii)followsfromparts(i)and(ii)andtheasymptoticpropertiesdiscussedinAppendixC.Thorem5.2isusefulbecausethenormalityassumptionMLR.6hasbeendropped;theonlyrestrictiononthedistributionoftheerroristhatithasfinitevariance,some-thingwewillalwaysassume.Wehavealsoassumedzeroconditionalmeanandhomoskedasticityofu.Noticehowthestandardnormaldistributionappearsin(5.7),asopposedtothetnk1distribution.Thisisbecausethedistributionisonlyapproximate.Bycontrast,inTheorem4.2,thedistributionoftheratioin(5.7)wasexactlytnk1foranysamplesize.Fromapracticalperspective,thisdifferenceisirrelevant.Infact,itisjustaslegitimatetowrite(ˆjj)/se(ˆj)~ªtnk1,(5.8)sincetnk1approachesthestandardnormaldistributionasthedegreesoffreedomgetslarge.Equation(5.8)tellsusthatttestingandtheconstructionofconfidenceintervalsarecarriedoutexactlyasundertheclassicallinearmodelassumptions.Thismeansthatouranalysisofdependentvariableslikeprateandnarr86doesnothavetochangeatalliftheGauss-Markovassumptionshold:inbothcases,wehaveatleast1,500observations,whichiscertainlyenoughtojustifytheapproximationofthecentrallimittheorem.Ifthesamplesizeisnotverylarge,thenthetdistributioncanbeapoorapproxi-mationtothedistributionofthetstatisticswhenuisnotnormallydistributed.Unfortunately,therearenogeneralprescriptionsonhowbigthesamplesizemustbebeforetheapproximationisgoodenough.Someeconometriciansthinkthatn30issatisfactory,butthiscannotbesufficientforallpossibledistributionsofu.Dependingonthedistributionofu,moreobservationsmaybenecessarybeforethecentrallimittheoremtakeseffect.Further,thequalityoftheapproximationdependsnotjustonn,butonthedf,nk1:withmoreindependentvariablesinthemodel,alargersam-plesizeisusuallyneededtousethetapproximation.Methodsforinferencewithsmalldegreesoffreedomandnonnormalerrorsareoutsidethescopeofthistext.Wewillsimplyusethetstatisticsaswealwayshavewithoutworryingaboutthenormalityassumption.ItisveryimportanttoseethatTheorem5.2doesrequirethehomoskedasticityassumption(alongwiththezeroconditionalmeanassumption).IfVar(yx)isnotcon-stant,theusualtstatisticsandconfidenceintervalsareinvalidnomatterhowlargethesamplesizeis;thecentrallimittheoremdoesnotbailusoutwhenitcomestohet-eroskedasticity.Forthisreason,wedevoteallofChapter8todiscussingwhatcanbedoneinthepresenceofheteroskedasticity.22OneconclusionofTheorem5.2isthatˆisaconsistentestimatorof;wealready22knowfromTheorem3.3thatˆisunbiasedforundertheGauss-Markovassump-tions.Theconsistencyimpliesthatˆisaconsistentestimatorof,whichisimportantinestablishingtheasymptoticnormalityresultinequation(5.7).169\nd7/14/995:21PMPage170Part1RegressionAnalysiswithCross-SectionalDataRememberthatˆappearsinthestandarderrorforeachˆj.Infact,theestimatedvarianceofˆjis2ˆVaˆr(ˆj)2,(5.9)SSTj(1Rj)2whereSSTjisthetotalsumofsquaresofxjinthesample,andRjistheR-squaredfromregressingxjonalloftheotherindependentvariables.InSection3.4,westudiedeachcomponentof(5.9),whichwewillnowexpoundoninthecontextofasymptoticanaly-22sis.Asthesamplesizegrows,ˆconvergesinprobabilitytotheconstant.Further,22Rjapproachesanumberstrictlybetweenzeroandunity(sothat1Rjconvergestosomenumberbetweenzeroandone).ThesamplevarianceofxjisSSTj/n,andsoSSTj/nconvergestoVar(xj)asthesamplesizegrows.ThismeansthatSSTjgrowsatapproxi-22matelythesamerateasthesamplesize:SSTjnj,wherejisthepopulationvari-anceofxj.Whenwecombinethesefacts,wefindthatVaˆr(ˆj)shrinkstozeroattheQUESTION5.2rateof1/n;thisiswhylargersamplesizesInaregressionmodelwithalargesamplesize,whatisanapproxi-arebetter.mate95%confidenceintervalforˆjunderMLR.1throughMLR.5?Whenuisnotnormallydistributed,theWecallthisanasymptoticconfidenceinterval.squarerootof(5.9)issometimescalledtheasymptoticstandarderror,andtstatis-ticsarecalledasymptotictstatistics.BecausethesearethesamequantitieswedealtwithinChapter4,wewilljustcallthemstandarderrorsandtstatistics,withtheunder-standingthatsometimestheyhaveonlylargesamplejustification.Usingtheprecedingargumentabouttheestimatedvariance,wecanwritese(ˆj)cj/n,(5.10)wherecjisapositiveconstantthatdoesnotdependonthesamplesize.Equation(5.10)isonlyanapproximation,butitisausefulruleofthumb:standarderrorscanbeexpectedtoshrinkataratethatistheinverseofthesquarerootofthesamplesize.EXAMPLE5.2(StandardErrorsinaBirthWeightEquation)WeusethedatainBWGHT.RAWtoestimatearelationshipwherelogofbirthweightisthedependentvariable,andcigarettessmokedperday(cigs)andlogoffamilyincomelog(faminc)areindependentvariables.Thetotalnumberofobservationsis1,388.Usingthefirsthalfoftheobservations(694),thestandarderrorforˆcigsisabout.0013.Thestandarderrorusingalloftheobservationsisabout.00086.Theratioofthelatterstandarderrortotheformeris.00086/.0013.662.Thisisprettycloseto694/1,388.707,theratioobtainedfromtheapproximationin(5.10).Inotherwords,equation(5.10)impliesthatthestandarderrorusingthelargersamplesizeshouldbeabout70.7%ofthestandarderrorusingthesmallersample.Thispercentageisprettyclosetothe66.2%weactuallycomputefromtheratioofthestandarderrors.170\nd7/14/995:21PMPage171Chapter5MultipleRegressionAnalysis:OLSAsymptoticsTheasymptoticnormalityoftheOLSestimatorsalsoimpliesthattheFstatisticshaveapproximateFdistributionsinlargesamplesizes.Thus,fortestingexclusionrestrictionsorothermultiplehypotheses,nothingchangesfromwhatwehavedonebefore.OtherLargeSampleTests:TheLagrangeMultiplierStatisticOnceweentertherealmofasymptoticanalysis,thereareotherteststatisticsthatcanbeusedforhypothesistesting.Formostpurposes,thereislittlereasontogobeyondtheusualtandFstatistics:aswejustsaw,thesestatisticshavelargesamplejustificationwithoutthenormalityassumption.Nevertheless,sometimesitisusefultohaveotherwaystotestmultipleexclusionrestrictions,andwenowcovertheLagrangemultiplierLMstatistic,whichhasachievedsomepopularityinmoderneconometrics.Thename“Lagrangemultiplierstatistic”comesfromconstrainedoptimization,atopicbeyondthescopeofthistext.[SeeDavidsonandMacKinnon(1993).]Thenamescorestatistic—whichalsocomesfromoptimizationusingcalculus—isusedaswell.Fortunately,inthelinearregressionframework,itissimpletomotivatetheLMstatisticwithoutdelvingintocomplicatedmathematics.TheformoftheLMstatisticwederiveherereliesontheGauss-Markovassump-tions,thesameassumptionsthatjustifytheFstatisticinlargesamples.Wedonotneedthenormalityassumption.ToderivetheLMstatistic,considertheusualmultipleregressionmodelwithkinde-pendentvariables:y01x1…kxku.(5.11)Wewouldliketotestwhether,say,thelastqofthesevariablesallhavezeropopulationparameters:thenullhypothesisisH0:kq+10,…,k0,(5.12)whichputsqexclusionrestrictionsonthemodel(5.11).AswithFtesting,thealterna-tiveto(5.12)isthatatleastoneoftheparametersisdifferentfromzero.TheLMstatisticrequiresestimationoftherestrictedmodelonly.Thus,assumethatwehaveruntheregressiony˜0˜1x1…˜kqxkqu˜,(5.13)where“~”indicatesthattheestimatesarefromtherestrictedmodel.Inparticular,u˜indicatestheresidualsfromtherestrictedmodel.(Asalways,thisisjustshorthandtoindicatethatweobtaintherestrictedresidualforeachobservationinthesample.)Iftheomittedvariablesxkq1throughxktrulyhavezeropopulationcoefficientsthen,atleastapproximately,u˜shouldbeuncorrelatedwitheachofthesevariablesinthesample.ThissuggestsrunningaregressionoftheseresidualsonthoseindependentvariablesexcludedunderH0,whichisalmostwhattheLMtestdoes.However,itturnsoutthat,togetausableteststatistic,wemustincludealloftheindependentvariables171\nd7/14/995:21PMPage172Part1RegressionAnalysiswithCross-SectionalDataintheregression(thereasonsforthisaretechnicalandunimportant).Thus,weruntheregressionu˜onx1,x2,…,xk.(5.14)Thisisanexampleofanauxiliaryregression,aregressionthatisusedtocomputeateststatisticbutwhosecoefficientsarenotofdirectinterest.Howcanweusetheregressionoutputfrom(5.14)totest(5.12)?If(5.12)istrue,theR-squaredfrom(5.14)shouldbe“close”tozero,subjecttosamplingerror,becauseu˜willbeapproximatelyuncorrelatedwithalltheindependentvariables.Thequestion,asalwayswithhypothesistesting,ishowtodeterminewhenthestatisticislargeenoughtorejectthenullhypothesisatachosensignificancelevel.Itturnsoutthat,underthenullhypothesis,thesamplesizemultipliedbytheusualR-squaredfromtheauxiliaryregression(5.14)isdistributedasymptoticallyasachi-squarerandomvariablewithqdegreesoffreedom.Thisleadstoasimpleprocedurefortestingthejointsignificanceofasetofqindependentvariables.THELAGRANGEMULTIPLIERSTATISTICFORqEXCLUSIONRESTRICTIONS:(i)Regressyontherestrictedsetofindependentvariablesandsavetheresidu-als,u˜.2(ii)Regressu˜onalloftheindependentvariablesandobtaintheR-squared,sayRu(todistinguishitfromtheR-squaredsobtainedwithyasthedependentvari-able).2(iii)ComputeLMnRu[thesamplesizetimestheR-squaredobtainedfromstep(ii)].2(iv)CompareLMtotheappropriatecriticalvalue,c,inaqdistribution;ifLMc,thenullhypothesisisrejected.Evenbetter,obtainthep-valueastheproba-2bilitythataqrandomvariableexceedsthevalueoftheteststatistic.Ifthep-valueislessthanthedesiredsignificancelevel,thenH0isrejected.Ifnot,wefailtorejectH0.TherejectionruleisessentiallythesameasforFtesting.Becauseofitsform,theLMstatisticissometimesreferredtoasthen-R-squaredstatistic.UnlikewiththeFstatistic,thedegreesoffreedomintheunrestrictedmodelplaysnoroleincarryingouttheLMtest.Allthatmattersisthenumberofrestrictions2beingtested(q),thesizeoftheauxiliaryR-squared(Ru),andthesamplesize(n).ThedfintheunrestrictedmodelplaysnorolebecauseoftheasymptoticnatureoftheLM2statistic.ButwemustbesuretomultiplyRubythesamplesizetoobtainLM;aseem-inglylowvalueoftheR-squaredcanstillleadtojointsignificanceifnislarge.Beforegivinganexample,awordofcautionisinorder.Ifinstep(i),wemistak-enlyregressyonalloftheindependentvariablesandobtaintheresidualsfromthisunrestrictedregressiontobeusedinstep(ii),wedonotgetaninterestingstatistic:theresultingR-squaredwillbeexactlyzero!ThisisbecauseOLSchoosestheestimatessothattheresidualsareuncorrelatedinsampleswithallincludedindependentvariables[seeequations(3.13)].Thus,wecanonlytest(5.12)byregressingtherestrictedresid-ualsonalloftheindependentvariables.(Regressingtherestrictedresidualsonthe2restrictedsetofindependentvariableswillalsoproduceR0.)172\nd7/14/995:21PMPage173Chapter5MultipleRegressionAnalysis:OLSAsymptoticsEXAMPLE5.3(EconomicModelofCrime)WeillustratetheLMtestbyusingaslightextensionofthecrimemodelfromExample3.4:narr8601pcnv2avgsen3tottime4ptime865qemp86u,wherenarr86isthenumberoftimesamanwasarrested,pcnvistheproportionofpriorarrestsleadingtoconviction,avgsenisaveragesentenceservedfrompastconvictions,tottimeistotaltimethemanhasspentinprisonpriorto1986sincereachingtheageof18,ptime86ismonthsspentinprisonin1986,andqemp86isnumberofquartersin1986duringwhichthemanwaslegallyemployed.WeusetheLMstatistictotestthenullhypoth-esisthatavgsenandtottimehavenoeffectonnarr86oncetheotherfactorshavebeencontrolledfor.Instep(i),weestimatetherestrictedmodelbyregressingnarr86onpcnv,ptime86,andqemp86;thevariablesavgsenandtottimeareexcludedfromthisregression.Weobtaintheresidualsu˜fromthisregression,2,725ofthem.Next,weruntheregressionu˜onpcnv,ptime86,qemp86,avgsen,andtottime;(5.15)asalways,theorderinwhichwelisttheindependentvariablesisirrelevant.Thissecond2regressionproducesRu,whichturnsouttobeabout.0015.Thismayseemsmall,butwemustmultiplyitbyntogettheLMstatistic:LM2,725(.0015)4.09.The10%criticalvalueinachi-squaredistributionwithtwodegreesoffreedomisabout4.61(roundedtotwodecimalplaces;seeTableG.4).Thus,wefailtorejectthenullhypothesisthatavgsen20andtottime0atthe10%level.Thep-valueisP(24.09).129,sowewouldrejectH0atthe15%level.Asacomparison,theFtestforjointsignificanceofavgsenandtottimeyieldsap-valueofabout.131,whichisprettyclosetothatobtainedusingtheLMstatistic.Thisisnotsur-prisingsince,asymptotically,thetwostatisticshavethesameprobabilityofTypeIerror.(Thatis,theyrejectthenullhypothesiswiththesamefrequencywhenthenullistrue.)Asthepreviousexamplesuggests,withalargesample,werarelyseeimportantdis-crepanciesbetweentheoutcomesofLMandFtests.WewillusetheFstatisticforthemostpartbecauseitiscomputedroutinelybymostregressionpackages.ButyoushouldbeawareoftheLMstatisticasitisusedinappliedwork.OnefinalcommentontheLMstatistic.AswiththeFstatistic,wemustbesuretousethesameobservationsinsteps(i)and(ii).Ifdataaremissingforsomeoftheinde-pendentvariablesthatareexcludedunderthenullhypothesis,theresidualsfromstep(i)shouldbeobtainedfromaregressiononthereduceddataset.5.3ASYMPTOTICEFFICIENCYOFOLSWeknowthat,undertheGauss-Markovassumptions,theOLSestimatorsarebestlin-earunbiased.OLSisalsoasymptoticallyefficientamongacertainclassofestimators173\nd7/14/995:21PMPage174Part1RegressionAnalysiswithCross-SectionalDataundertheGauss-Markovassumptions.Ageneraltreatmentisdifficult[seeWooldridge(1999,Chapter4)].Fornow,wedescribetheresultinthesimpleregressioncase.Inthemodely01xu,(5.16)uhasazeroconditionalmeanunderMLR.3:E(ux)0.Thisopensupavarietyofcon-sistentestimatorsfor0and1;asusual,wefocusontheslopeparameter,1.Letg(x)2beanyfunctionofx;forexample,g(x)xorg(x)1/(1x).Thenuisuncorre-latedwithg(x)(seePropertyCE.5inAppendixB).Letzig(xi)forallobservationsi.Thentheestimatornn˜1(ziz¯)yi(ziz¯)xi(5.17)i1i1isconsistentfor1,providedg(x)andxarecorrelated.(Remember,itispossiblethatg(x)andxareuncorrelatedbecausecorrelationmeasureslineardependence.)Toseethis,wecanpluginyi01xiuiandwrite˜1asnn11˜11n(ziz¯)uin(ziz¯)xi.(5.18)i1i1Now,wecanapplythelawoflargenumberstothenumeratoranddenominator,whichconvergeinprobabilitytoCov(z,u)andCov(z,x),respectively.ProvidedthatCov(z,x)0—sothatzandxarecorrelated—wehaveplim˜11Cov(z,u)/Cov(z,x)1,becauseCov(z,u)0underMLR.3.Itismoredifficulttoshowthat˜1isasymptoticallynormal.Nevertheless,usingargumentssimilartothoseintheappendix,itcanbeshownthatn(˜11)isasymp-22toticallynormalwithmeanzeroandasymptoticvarianceVar(z)/[Cov(z,x)].TheasymptoticvarianceoftheOLSestimatorisobtainedwhenzx,inwhichcase,Cov(z,x)Cov(x,x)Var(x).Therefore,theasymptoticvarianceofn(˜11),222whereˆ1istheOLSestimator,isVar(x)/[Var(x)]/Var(x).Now,theCauchy-2Schwartzinequality(seeAppendixB.4)impliesthat[Cov(z,x)]Var(z)Var(x),whichimpliesthattheasymptoticvarianceofn(ˆ11)isnolargerthanthatofn(˜11).Wehaveshowninthesimpleregressioncasethat,undertheGauss-Markovassumptions,theOLSestimatorhasasmallerasymptoticvariancethananyestimatoroftheform(5.17).[Theestimatorin(5.17)isanexampleofaninstrumentalvariablesestimator,whichwewillstudyextensivelyinChapter15.]Ifthehomo-skedasticityassumptionfails,thenthereareestimatorsoftheform(5.17)thathaveasmallerasymptoticvariancethanOLS.WewillseethisinChapter8.Thegeneralcaseissimilarbutmuchmoredifficultmathematically.Inthekregres-sorcase,theclassofconsistentestimatorsisobtainedbygeneralizingtheOLSfirstorderconditions:174\nd7/14/995:21PMPage175Chapter5MultipleRegressionAnalysis:OLSAsymptoticsngj(xi)(yi˜0˜1xi1…˜kxik)0,j0,1,…,k,(5.19)i1wheregj(xi)denotesanyfunctionofallexplanatoryvariablesforobservationi.Ascanbeseenbycomparing(5.19)withtheOLSfirstorderconditions(3.13),weobtaintheOLSestimatorswheng0(xi)1andgj(xi)xijforj1,2,…,k.Theclassofestima-torsin(5.19)isinfinite,becausewecanuseanyfunctionsofthexijthatwewant.THEOREM5.3(ASYMPTOTICEFFICIENCYOFOLS)UndertheGauss-Markovassumptions,let˜jdenoteestimatorsthatsolveequationsoftheform(5.19)andletˆjdenotetheOLSestimators.Thenforj0,1,2,…,k,theOLSesti-matorshavethesmallestasymptoticvariances:Avarn(ˆjj)Avarn(˜jj).Provingconsistencyoftheestimatorsin(5.19),letaloneshowingtheyareasymptoti-callynormal,ismathematicallydifficult.[SeeWooldridge(1999,Chapter5).]SUMMARYTheclaimsunderlyingthematerialinthischapterarefairlytechnical,buttheirpracti-calimplicationsarestraightforward.WehaveshownthatthefirstfourGauss-MarkovassumptionsimplythatOLSisconsistent.Furthermore,allofthemethodsoftestingandconstructingconfidenceintervalsthatwelearnedinChapter4areapproximatelyvalidwithoutassumingthattheerrorsaredrawnfromanormaldistribution(equiva-lently,thedistributionofygiventheexplanatoryvariablesisnotnormal).ThismeansthatwecanapplyOLSandusepreviousmethodsforanarrayofapplicationswherethedependentvariableisnotevenapproximatelynormallydistributed.WealsoshowedthattheLMstatisticcanbeusedinsteadoftheFstatisticfortestingexclusionrestrictions.Beforeleavingthischapter,weshouldnotethatexamplessuchasExample5.3mayverywellhaveproblemsthatdorequirespecialattention.Foravariablesuchasnarr86,whichiszerooroneformostmeninthepopulation,alinearmodelmaynotbeabletoadequatelycapturethefunctionalrelationshipbetweennarr86andtheexplanatoryvari-ables.Moreover,evenifalinearmodeldoesdescribetheexpectedvalueofarrests,het-eroskedasticitymightbeaproblem.Problemssuchasthesearenotmitigatedasthesamplesizegrows,andwewillreturntotheminlaterchapters.KEYTERMSAsymptoticBiasAuxiliaryRegressionAsymptoticConfidenceIntervalConsistencyAsymptoticNormalityInconsistencyAsymptoticPropertiesLagrangeMultiplierLMStatisticAsymptoticStandardErrorLargeSamplePropertiesAsymptotictStatisticsn-R-squaredStatisticAsymptoticVarianceScoreStatisticAsymptoticallyEfficient175\nd7/14/995:21PMPage176Part1RegressionAnalysiswithCross-SectionalDataPROBLEMS5.1InthesimpleregressionmodelunderMLR.1throughMLR.4,wearguedthattheslopeestimator,ˆ1,isconsistentfor1.Usingˆ0y¯ˆ1x¯1,showthatplimˆ00.[Youneedtousetheconsistencyofˆ1andthelawoflargenumbers,alongwiththefactthat0E(y)1(x1).]5.2Supposethatthemodelpctstck01funds2risktolusatisfiesthefirstfourGauss-Markovassumptions,wherepctstckisthepercentageofaworker’spensioninvestedinthestockmarket,fundsisthenumberofmutualfundsthattheworkercanchoosefrom,andrisktolissomemeasureofrisktolerance(largerrisk-tolmeansthepersonhasahighertoleranceforrisk).Iffundsandrisktolarepositivelycorrelated,whatistheinconsistencyin˜1,theslopecoefficientinthesimpleregressionofpctstckonfunds?5.3ThedatasetSMOKE.RAWcontainsinformationonsmokingbehaviorandothervariablesforarandomsampleofsingleadultsfromtheUnitedStates.Thevariablecigsisthe(average)numberofcigarettessmokedperday.DoyouthinkcigshasanormaldistributionintheU.S.population?Explain.5.4Inthesimpleregressionmodel(5.16),underthefirstfourGauss-Markovassump-tions,weshowedthatestimatorsoftheform(5.17)areconsistentfortheslope,1.Givensuchanestimator,defineanestimatorof0by˜0y¯˜1x¯.Showthatplim˜00.COMPUTEREXERCISES5.5UsethedatainWAGE1.RAWforthisexercise.(i)Estimatetheequationwage01educ2exper3tenureu.Savetheresidualsandplotahistogram.(ii)Repeatpart(i),butwithlog(wage)asthedependentvariable.(iii)WouldyousaythatAssumptionMLR.6isclosertobeingsatisfiedforthelevel-levelmodelorthelog-levelmodel?5.6UsethedatainGPA2.RAWforthisexercise.(i)Usingall4,137observations,estimatetheequationcolgpa01hsperc2satuandreporttheresultsinstandardform.(ii)Reestimatetheequationinpart(i),usingthefirst2,070observations.(iii)Findtheratioofthestandarderrorsonhspercfromparts(i)and(ii).Comparethiswiththeresultfrom(5.10).5.7Inequation(4.42)ofChapter4,computetheLMstatisticfortestingwhethermotheducandfatheducarejointlysignificant.Inobtainingtheresidualsfortherestrictedmodel,besurethattherestrictedmodelisestimatedusingonlythoseobser-vationsforwhichallvariablesintheunrestrictedmodelareavailable(seeExample4.9).176\nd7/14/995:21PMPage177Chapter5MultipleRegressionAnalysis:OLSAsymptoticsAPPENDIX5AWesketchaproofoftheasymptoticnormalityofOLS(Theorem5.2[i])inthesimpleregressioncase.Writethesimpleregressionmodelasinequation(5.16).Then,bytheusualalgebraofsimpleregressionwecanwriten21/2n(ˆ11)(1/sx)[n(xix¯)ui],i12whereweusesxtodenotethesamplevarianceof{xi:i1,2,…,n}.Bythelawof2p2largenumbers(seeAppendixC),sx*xVar(x).AssumptionMLR.4rulesoutnoperfectcollinearity,whichmeansthatVar(x)0(xivariesinthesample,andthereforenn1/21/2xisnotconstantinthepopulation).Next,n(xix¯)uin(xi)uini1i11/2(x¯)[nui],whereE(x)isthepopulationmeanofx.Now{ui}isase-i1n21/2quenceofi.i.d.randomvariableswithmeanzeroandvariance,andsonuii12convergestotheNormal(0,)distributionasn*;thisisjustthecentrallimitthe-oremfromAppendixC.Bythelawoflargenumbers,plim(x¯)0.Astandardresultinasymptotictheoryisthatifplim(wn)0andznhasanasymptoticnormaldis-tribution,thenplim(wnzn)0.[SeeWooldridge(1999,Chapter3)formorediscussion.]n1/2Thisimpliesthat(x¯)[nui]haszeroplim.Next,{(xi)ui:i1,2,…}isi1asequenceofi.i.d.randomvariableswithmeanzero—becauseuandxareuncorrelated22underAssumptionMLR.3—andvariancexbythehomoskedasticityAssumptionn1/222MLR.5.Therefore,n(xi)uihasanasymptoticNormal(0,x)distribution.i1nn1/21/2Wejustshowedthatthedifferencebetweenn(xix¯)uiandn(xi)uii1i1haszeroplim.Aresultinasymptotictheoryisthatifznhasanasymptoticnormaldis-tributionandplim(vnzn)0,thenvnhasthesameasymptoticnormaldistributionasn1/222zn.Itfollowsthatn(xix¯)uialsohasanasymptoticNormal(0,x)distribu-i1tion.Puttingallofthepiecestogethergivesn21/2n(ˆ11)(1/x)[n(xix¯)ui]i1n221/2[(1/sx)(1/x)[n(xix¯)ui],i122andsinceplim(1/sx)1/x,thesecondtermhaszeroplim.Therefore,theasymptotic222222distributionofn(ˆ11)isNormal(0,{x}/{x})Normal(0,/x).This22completestheproofinthesimpleregressioncase,asa1xinthiscase.SeeWooldridge(1999,Chapter4)forthegeneralcase.177\nd7/14/995:33PMPage178ChapterSixMultipleRegressionAnalysis:FurtherIssueshischapterbringstogetherseveralissuesinmultipleregressionanalysisthatweTcouldnotconvenientlycoverinearlierchapters.Thesetopicsarenotasfunda-mentalasthematerialinChapters3and4,buttheyareimportantforapplyingmultipleregressiontoabroadrangeofempiricalproblems.6.1EFFECTSOFDATASCALINGONOLSSTATISTICSInChapter2onbivariateregression,webrieflydiscussedtheeffectsofchangingtheunitsofmeasurementontheOLSinterceptandslopeestimates.WealsoshowedthatchangingtheunitsofmeasurementdidnotaffectR-squared.Wenowreturntotheissueofdatascalingandexaminetheeffectsofrescalingthedependentorindependentvari-ablesonstandarderrors,tstatistics,Fstatistics,andconfidenceintervals.Wewilldiscoverthateverythingweexpecttohappen,doeshappen.Whenvariablesarerescaled,thecoefficients,standarderrors,confidenceintervals,tstatistics,andFstatisticschangeinwaysthatpreserveallmeasuredeffectsandtestingoutcomes.Whilethisisnogreatsurprise—infact,wewouldbeveryworriedifitwerenotthecase—itisusefultoseewhatoccursexplicitly.Often,datascalingisusedforcosmeticpurposes,suchastoreducethenumberofzerosafteradecimalpointinanestimatedcoefficient.Byjudiciouslychoosingunitsofmeasurement,wecanimprovetheappearanceofanestimatedequationwhilechangingnothingthatisessential.Wecouldtreatthisprobleminageneralway,butitismuchbetterillustratedwithexamples.Likewise,thereislittlevaluehereinintroducinganabstractnotation.Webeginwithanequationrelatinginfantbirthweighttocigarettesmokingandfamilyincome:bwgˆhtˆ0ˆ1cigsˆ2faminc,(6.1)wherebwghtischildbirthweight,inounces,cigsisnumberofcigarettessmokedbythemotherwhilepregnant,perday,andfamincisannualfamilyincome,inthousandsofdollars.Theestimatesofthisequation,obtainedusingthedatainBWGHT.RAW,aregiveninthefirstcolumnofTable6.1.Standarderrorsarelistedinparentheses.Theesti-mateoncigssaysthatifawomansmoked5morecigarettesperday,birthweightispre-178\nd7/14/995:33PMPage179Chapter6MultipleRegressionAnalysis:FurtherIssuesTable6.1EffectsofDataScaling(1)(2)(3)DependentVariablebwghtbwghtlbsbwghtIndependentVariablescigs.4634.0289—(.0916)(.0057)packs——9.268(1.832)faminc.0927.0058.0927(.0292)(.0018)(.0292)intercept116.9747.3109116.974(1.049)(0.0656)(1.049)Observations:1,3881,3881,388R-squared:.0298.0298.0298SSR:557,485.512,177.6778557,485.51SER:20.0631.253920.063dictedtobeabout.4634(5)2.317ouncesless.Thetstatisticoncigsis5.03,sothevariableisverystatisticallysignificant.Now,supposethatwedecidetomeasurebirthweightinpounds,ratherthaninounces.Letbwghtlbsbwght/16bebirthweightinpounds.WhathappenstoourOLSstatisticsifweusethisasthedependentvariableinourequation?Itiseasytofindtheeffectonthecoefficientestimatesbysimplemanipulationofequation(6.1).Dividethisentireequationby16:bwgˆht/16ˆ0/16(ˆ1/16)cigs(ˆ2/16)faminc.Sincethelefthandsideisbirthweightinpounds,itfollowsthateachnewcoefficientwillbethecorrespondingoldcoefficientdividedby16.Toverifythis,theregressionofbwghtlbsoncigs,andfamincisreportedincolumn(2)ofTable6.1.Uptofourdigits,theinterceptandslopesincolumn(2)arejustthoseincolumn(1)dividedby16.Forexample,thecoefficientoncigsisnow.0289;thismeansthatifcigswerehigherbyfive,birthweightwouldbe.0289(5).1445poundslower.Intermsofounces,wehave179\nd7/14/995:33PMPage180Part1RegressionAnalysiswithCross-SectionalData.1445(16)2.312,whichisslightlydifferentfromthe2.32weobtainedearlierduetoroundingerror.Thepointis,oncetheeffectsaretransformedintothesameunits,wegetexactlythesameanswer,regardlessofhowthedependentvariableismeasured.Whataboutstatisticalsignificance?Asweexpect,changingthedependentvariablefromouncestopoundshasnoeffectonhowstatisticallyimportanttheindependentvariablesare.Thestandarderrorsincolumn(2)are16timessmallerthanthoseincol-umn(1).Afewquickcalculationsshowthatthetstatisticsincolumn(2)areindeedidenticaltothetstatisticsincolumn(2).Theendpointsfortheconfidenceintervalsincolumn(2)arejusttheendpointsincolumn(1)dividedby16.ThisisbecausetheCIschangebythesamefactorasthestandarderrors.[Rememberthatthe95%CIhereisˆj1.96se(ˆj).]Intermsofgoodness-of-fit,theR-squaredsfromthetworegressionsareidentical,asshouldbethecase.Noticethatthesumofsquaredresiduals,SSR,andthestandarderroroftheregression,SER,dodifferacrossequations.Thesedifferencesareeasilyexplained.Letuˆidenotetheresidualforobservationiintheoriginalequation(6.1).Thentheresidualwhenbwghtlbsisthedependentvariableissimplyuˆi/16.Thus,the22squaredresidualinthesecondequationis(uˆi/16)uˆi/256.Thisiswhythesumofsquaredresidualsincolumn(2)isequaltotheSSRincolumn(1)dividedby256.SinceSERˆSSR/(nk1)SSR/1,385,theSERincolumn(2)is16timessmallerthanthatincolumn(1).Anotherwaytothinkaboutthisisthattheerrorintheequationwithbwghtlbsasthedependentvariablehasastandarddeviation16timessmallerthanthestandarddeviationoftheoriginalerror.Thisdoesnotmeanthatwehavereducedtheerrorbychanginghowbirthweightismeasured;thesmallerSERsimplyreflectsadifferenceinunitsofmeasurement.Next,letusreturnthedependentvariabletoitsoriginalunits:bwghtismeasuredinounces.Instead,letuschangetheunitofmeasurementofoneoftheindependentvari-ables,cigs.Definepackstobethenumberofpacksofcigarettessmokedperday.Thus,packscigs/20.WhathappenstothecoefficientsandotherOLSstatisticsnow?Well,wecanwritebwgˆhtˆ0(20ˆ1)(cigs/20)ˆ2famincˆ0(20ˆ1)packsˆ2faminc.Thus,theinterceptandslopecoefficientonfamincareunchanged,butthecoefficientonpacksis20timesthatoncigs.Thisisintuitivelyappealing.Theresultsfromtheregressionofbwghtonpacksandfamincareincolumn(3)ofTable6.1.Incidentally,rememberthatitwouldmakenosensetoincludebothcigsandpacksinthesameQUESTION6.1equation;thiswouldinduceperfectmulti-Intheoriginalbirthweightequation(6.1),supposethatfaminciscollinearityandwouldhavenointerestingmeasuredindollarsratherthaninthousandsofdollars.Thus,definemeaning.thevariablefincdol1,000faminc.HowwilltheOLSstatisticsOtherthanthecoefficientonpacks,changewhenfincdolissubstitutedforfaminc?Forthepurposesofthereisoneotherstatisticincolumn(3)presentingtheregressionresults,doyouthinkitisbettertomeasureincomeindollarsorinthousandsofdollars?thatdiffersfromthatincolumn(1):thestandarderroronpacksis20timeslargerthanthatoncigsincolumn(1).Thismeansthatthetstatisticfortestingthesignificanceofcigarettesmokingisthesamewhetherwemeasuresmokingintermsofcigarettesorpacks.Thisisonlynatural.180\nd7/14/995:33PMPage181Chapter6MultipleRegressionAnalysis:FurtherIssuesThepreviousexamplespellsoutmostofthepossibilitiesthatarisewhenthedepen-dentandindependentvariablesarerescaled.Rescalingisoftendonewithdollaramountsineconomics,especiallywhenthedollaramountsareverylarge.InChapter2wearguedthat,ifthedependentvariableappearsinlogarithmicform,changingtheunitsofmeasurementdoesnotaffecttheslopecoefficient.Thesameistruehere:changingtheunitsofmeasurementofthedependentvariable,whenitappearsinlogarithmicform,doesnotaffectanyoftheslopeestimates.Thisfollowsfromthesimplefactthatlog(c1yi)log(c1)log(yi)foranyconstantc10.Thenewinterceptwillbelog(c1)ˆ0.Similarly,changingtheunitsofmeasurementofanyxj,wherelog(xj)appearsintheregression,onlyaffectstheintercept.Thiscorrespondstowhatweknowaboutpercentagechangesand,inparticular,elasticities:theyareinvarianttotheunitsofmeasurementofeitheryorthexj.Forexample,ifwehadspecifiedthedepen-dentvariablein(6.1)tobelog(bwght),estimatedtheequation,andthenreestimateditwithlog(bwghtlbs)asthedependentvariable,thecoefficientsoncigsandfamincwouldbethesameinbothregressions;onlytheinterceptwouldbedifferent.BetaCoefficientsSometimesineconometricapplications,akeyvariableismeasuredonascalethatisdifficulttointerpret.Laboreconomistsoftenincludetestscoresinwageequations,andthescaleonwhichthesetestsarescoredisoftenarbitraryandnoteasytointerpret(atleastforeconomists!).Inalmostallcases,weareinterestedinhowaparticularindi-vidual’sscorecompareswiththepopulation.Thus,insteadofaskingabouttheeffectonhourlywageif,say,atestscoreis10pointshigher,itmakesmoresensetoaskwhathappenswhenthetestscoreisonestandarddeviationhigher.Nothingpreventsusfromseeingwhathappenstothedependentvariablewhenanindependentvariableinanestimatedmodelincreasesbyacertainnumberofstandarddeviations,assumingthatwehaveobtainedthesamplestandarddeviation(whichiseasyinmostregressionpackages).Thisisoftenagoodidea.So,forexample,whenwelookattheeffectofastandardizedtestscore,suchastheSATscore,oncollegeGPA,wecanfindthestandarddeviationofSATandseewhathappenswhentheSATscoreincreasesbyoneortwostandarddeviations.Sometimesitisusefultoobtainregressionresultswhenallvariablesinvolved,thedependentaswellasalltheindependentvariables,havebeenstandardized.Avariableisstandardizedinthesamplebysubtractingoffitsmeananddividingbyitsstandarddeviation(seeAppendixC).Thismeansthatwecomputethez-scoreforeveryvariableinthesample.Then,werunaregressionusingthez-scores.Whyisstandardizationuseful?ItiseasiesttostartwiththeoriginalOLSequation,withthevariablesintheiroriginalforms:yiˆ0ˆ1xi1ˆ2xi2…ˆkxikuˆi.(6.2)Wehaveincludedtheobservationsubscriptitoemphasizethatourstandardizationisappliedtoallsamplevalues.Now,ifweaverage(6.2),usethefactthattheuˆihaveazerosampleaverage,andsubtracttheresultfrom(6.2),wegetyiy¯ˆ1(xi1x¯1)ˆ2(xi2x¯2)…ˆk(xikx¯k)uˆi.181\nd7/14/995:33PMPage182Part1RegressionAnalysiswithCross-SectionalDataNow,letˆybethesamplestandarddeviationforthedependentvariable,letˆ1bethesamplesdforx1,letˆ2bethesamplesdforx2,andsoon.Then,simplealgebragivestheequation(yiy¯)/ˆy(ˆ1/ˆy)ˆ1[(xi1x¯1)/ˆ1]…(6.3)(ˆk/ˆy)ˆk[(xikx¯k)/ˆk](uˆi/ˆy).Eachvariablein(6.3)hasbeenstandardizedbyreplacingitwithitsz-score,andthishasresultedinnewslopecoefficients.Forexample,theslopecoefficienton(xi1x¯1)/ˆ1is(ˆ1/ˆy)ˆ1.Thisissimplytheoriginalcoefficient,ˆ1,multipliedbytheratioofthestan-darddeviationofx1tothestandarddeviationofy.Theintercepthasdroppedoutalto-gether.Itisusefultorewrite(6.3),droppingtheisubscriptaszybˆ1z1bˆ2z2…bˆkzkerror,(6.4)wherezydenotesthez-scoreofy,z1isthez-scoreofx1,andsoon.Thenewcoefficientsarebˆj(ˆj/ˆy)ˆjforj1,…,k.(6.5)Thesebˆjaretraditionallycalledstandardizedcoefficientsorbetacoefficients.(Thelatternameismorecommon,whichisunfortunatesincewehavebeenusingbetahattodenotetheusualOLSestimates.)Betacoefficientsreceivetheirinterestingmeaningfromequation(6.4):Ifx1increasesbyonestandarddeviation,thenyˆchangesbybˆ1standarddeviations.Thus,wearemeasuringeffectsnotintermsoftheoriginalunitsofyorthexj,butinstandarddeviationunits.Becauseitmakesthescaleoftheregressorsirrelevant,thisequationputstheexplanatoryvariablesonequalfooting.InastandardOLSequation,itisnotpossibletosimplylookatthesizeofdifferentcoefficientsandconcludethattheexplanatoryvariablewiththelargestcoefficientis“themostimportant.”Wejustsawthatthemagnitudesofcoefficientscanbechangedatwillbychangingtheunitsofmea-surementofthexj.But,wheneachxjhasbeenstandardized,comparingthemagnitudesoftheresultingbetacoefficientsismorecompelling.Toobtainthebetacoefficients,wecanalwaysstandardizey,x1,…,xk,andthenruntheOLSregressionofthez-scoreofyonthez-scoresofx1,…,xk—whereitisnotnec-essarytoincludeanintercept,asitwillbezero.Thiscanbetediouswithmanyinde-pendentvariables.Someregressionpackagesprovidebetacoefficientsviaasimplecommand.Thefollowingexampleillustratestheuseofbetacoefficients.EXAMPLE6.1(EffectsofPollutiononHousingPrices)WeusethedatafromExample4.5(inthefileHPRICE2.RAW)toillustratetheuseofbetacoefficients.Recallthatthekeyindependentvariableisnox,ameasureofthenitrogenoxideintheairovereachcommunity.Onewaytounderstandthesizeofthepollution182\nd7/14/995:33PMPage183Chapter6MultipleRegressionAnalysis:FurtherIssueseffect—withoutgettingintothescienceunderlyingnitrogenoxide’seffectonairquality—istocomputebetacoefficients.(AnalternativeapproachiscontainedinExample4.5:weobtainedapriceelasticitywithrespecttonoxbyusingpriceandnoxinlogarithmicform.)Thepopulationequationisthelevel-levelmodelprice01nox2crime3rooms4dist5stratiou,whereallthevariablesexceptcrimeweredefinedinExample4.5;crimeisthenumberofreportedcrimespercapita.Thebetacoefficientsarereportedinthefollowingequation(soeachvariablehasbeenconvertedtoitsz-score):zpriˆce.340znox.143zcrime.514zrooms.235zdist.270zstratio.Thisequationshowsthataonestandarddeviationincreaseinnoxdecreasespriceby.34standarddeviations;aonestandarddeviationincreaseincrimereducespriceby.14stan-darddeviation.Thus,thesamerelativemovementofpollutioninthepopulationhasalargereffectonhousingpricesthancrimedoes.Sizeofthehouse,asmeasuredbynumberofrooms(rooms),hasthelargeststandardizedeffect.Ifwewanttoknowtheeffectsofeachindependentvariableonthedollarvalueofmedianhouseprice,weshouldusetheunstandardizedvariables.6.2MOREONFUNCTIONALFORMInseveralpreviousexamples,wehaveencounteredthemostpopulardeviceinecono-metricsforallowingnonlinearrelationshipsbetweentheexplainedandexplanatoryvariables:usinglogarithmsforthedependentorindependentvariables.Wehavealsoseenmodelscontainingquadraticsinsomeexplanatoryvariables,butwehaveyettoprovideasystematictreatmentofthem.Inthissection,wecoversomevariationsandextensionsonfunctionalformsthatoftenariseinappliedwork.MoreonUsingLogarithmicFunctionalFormsWebeginbyreviewinghowtointerprettheparametersinthemodellog(price)01log(nox)2roomsu,(6.6)wherethesevariablesaretakenfromExample4.5.Recallthatthroughoutthetextlog(x)isthenaturallogofx.Thecoefficient1istheelasticityofpricewithrespecttonox(pollution).Thecoefficient2isthechangeinlog(price),whenrooms1;aswehaveseenmanytimes,whenmultipliedby100,thisistheapproximatepercentagechangeinprice.Recallthat1002issometimescalledthesemi-elasticityofpricewithrespecttorooms.WhenestimatedusingthedatainHPRICE2.RAW,weobtainlog(priˆce)(9.23)(.718)log(nox)(.306)roomslog(priˆce)(0.19)(.066)log(nox)(.019)rooms(6.7)2n506,R.514.183\nd7/14/995:33PMPage184Part1RegressionAnalysiswithCross-SectionalDataThus,whennoxincreasesby1%,pricefallsby.718%,holdingonlyroomsfixed.Whenroomsincreasesbyone,priceincreasesbyapproximately100(.306)30.6%.Theestimatethatonemoreroomincreasespricebyabout30.6%turnsouttobesomewhatinaccurateforthisapplication.Theapproximationerroroccursbecause,asthechangeinlog(y)becomeslargerandlarger,theapproximation%y100log(y)becomesmoreandmoreinaccurate.Fortunately,asimplecalculationisavailabletocomputetheexactpercentagechange.Todescribetheprocedure,weconsiderthegeneralestimatedmodelloˆg(y)ˆ0ˆ1log(x1)ˆ2x2.(Addingadditionalindependentvariablesdoesnotchangetheprocedure.)Now,fixingx1,wehaveloˆg(y)ˆ2x2.Usingsimplealgebraicpropertiesoftheexponentialandlogarithmicfunctionsgivestheexactpercentagechangeinthepredictedyasˆ%y100[exp(ˆ2x2)1],(6.8)wherethemultiplicationby100turnstheproportionatechangeintoapercentagechange.Whenx21,ˆ%y100[exp(ˆ2)1].(6.9)Appliedtothehousingpriceexamplewithx2roomsandˆ2.306,%priˆce100[exp(.306)1]35.8%,whichisnotablylargerthantheapproximatepercentagechange,30.6%,obtaineddirectlyfrom(6.7).{Incidentally,thisisnotanunbiasedesti-matorbecauseexp()isanonlinearfunction;itis,however,aconsistentestimatorof100[exp(2)1].Thisisbecausetheprobabilitylimitpassesthroughcontinuousfunc-tions,whiletheexpectedvalueoperatordoesnot.SeeAppendixC.}Theadjustmentinequation(6.8)isnotascrucialforsmallpercentagechanges.Forexample,whenweincludethestudent-teacherratioinequation(6.7),itsestimatedcoefficientis.052,whichmeansthatifstratioincreasesbyone,pricedecreasesbyapproximately5.2%.Theexactproportionatechangeisexp(.052)1.051,or5.1%.Ontheotherhand,ifweincreasestratiobyfive,thentheapproximateper-centagechangeinpriceis26%,whiletheexactchangeobtainedfromequation(6.8)is100[exp(.26)1]22.9%.Wehaveseenthatusingnaturallogsleadstocoefficientswithappealinginterpreta-tions,andwecanbeignorantabouttheunitsofmeasurementofvariablesappearinginlogarithmicformbecausetheslopecoefficientsareinvarianttorescalings.Thereareseveralotherreasonslogsareusedsomuchinappliedwork.First,wheny0,mod-elsusinglog(y)asthedependentvariableoftensatisfytheCLMassumptionsmorecloselythanmodelsusingthelevelofy.Strictlypositivevariablesoftenhavecondi-tionaldistributionsthatareheteroskedasticorskewed;takingthelogcanmitigate,ifnoteliminate,bothproblems.Moreover,takinglogsusuallynarrowstherangeofthevariable,insomecasesbyaconsiderableamount.Thismakesestimateslesssensitivetooutlying(orextreme)observationsonthedependentorindependentvariables.Wetakeuptheissueofoutly-ingobservationsinChapter9.184\nd7/14/995:33PMPage185Chapter6MultipleRegressionAnalysis:FurtherIssuesTherearesomestandardrulesofthumbfortakinglogs,althoughnoneiswritteninstone.Whenavariableisapositivedollaramount,thelogisoftentaken.Wehaveseenthisforvariablessuchaswages,salaries,firmsales,andfirmmarketvalue.Variablessuchaspopulation,totalnumberofemployees,andschoolenrollmentoftenappearinlogarithmicform;thesehavethecommonfeatureofbeinglargeintegervalues.Variablesthataremeasuredinyears—suchaseducation,experience,tenure,age,andsoon—usuallyappearintheiroriginalform.Avariablethatisaproportionoraper-cent—suchastheunemploymentrate,theparticipationrateinapensionplan,theper-centageofstudentspassingastandardizedexam,thearrestrateonreportedcrimes—canappearineitheroriginalorlogarithmicform,althoughthereisatendencytousetheminlevelforms.Thisisbecauseanyregressioncoefficientsinvolvingtheoriginalvari-able—whetheritisthedependentorindependentvariable—willhaveapercentagepointchangeinterpretation.(SeeAppendixAforareviewofthedistinctionbetweenapercentagechangeandapercentagepointchange.)Ifweuse,say,log(unem)inaregres-sion,whereunemisthepercentofunemployedindividuals,wemustbeverycarefultodistinguishbetweenapercentagepointchangeandapercentagechange.Remember,ifunemgoesfrom8to9,thisisanincreaseofonepercentagepoint,buta12.5%increasefromtheinitialunemploymentlevel.UsingthelogmeansthatwearelookingattheQUESTION6.2percentagechangeintheunemploymentSupposethattheannualnumberofdrunkdrivingarrestsisdeter-rate:log(9)log(8).118or11.8%,minedbywhichisthelogarithmicapproximationtolog(arrests)01log(pop)2age16_25theactual12.5%increase.otherfactors,Onelimitationofthelogisthatitcan-notbeusedifavariabletakesonzeroorwhereage16_25istheproportionofthepopulationbetween16andnegativevalues.Incaseswhereavariable25yearsofage.Showthat2hasthefollowing(ceterisparibus)inter-pretation:itisthepercentagechangeinarrestswhenthepercentageyisnonnegativebutcantakeonthevalueofthepeopleaged16to25increasesbyonepercentagepoint.0,log(1y)issometimesused.Theper-centagechangeinterpretationsareoftencloselypreserved,exceptforchangesbeginningaty0(wherethepercentagechangeisnotevendefined).Generally,usinglog(1y)andtheninterpretingtheestimatesasifthevariablewerelog(y)isacceptablewhenthedataonyarenotdominatedbyzeros.Anexamplemightbewhereyishoursoftrainingperemployeeforthepopulationofmanufacturingfirms,ifalargefractionoffirmsprovidetrainingtoatleastoneworker.Onedrawbacktousingadependentvariableinlogarithmicformisthatitismoredifficulttopredicttheoriginalvariable.Theoriginalmodelallowsustopredictlog(y),noty.Nevertheless,itisfairlyeasytoturnapredictionforlog(y)intoapredictionfory(seeSection6.4).ArelatedpointisthatitisnotlegitimatetocompareR-squaredsfrommodelswhereyisthedependentvariableinonecaseandlog(y)isthedependentvariableintheother.Thesemeasuresexplainedvariationsindifferentvariables.Wedis-cusshowtocomputecomparablegoodness-of-fitmeasuresinSection6.4.ModelswithQuadraticsQuadraticfunctionsarealsousedquiteofteninappliedeconomicstocapturedecreas-ingorincreasingmarginaleffects.Youmaywanttoreviewpropertiesofquadraticfunc-tionsinAppendixA.185\nd7/14/995:33PMPage186Part1RegressionAnalysiswithCross-SectionalDataInthesimplestcase,ydependsonasingleobservedfactorx,butitdoessoinaqua-draticfashion:2y01x2xu.Forexample,takeywageandxexper.AswediscussedinChapter3,thismodelfallsoutsideofsimpleregressionanalysisbutiseasilyhandledwithmultipleregres-sion.Itisimportanttorememberthat1doesnotmeasurethechangeinywithrespect2tox;itmakesnosensetoholdxfixedwhilechangingx.Ifwewritetheestimatedequa-tionas2yˆˆ0ˆ1xˆ2x(6.10)thenwehavetheapproximationyˆ(ˆ12ˆ2x)x,soyˆ/xˆ12ˆ2x.(6.11)Thissaysthattheslopeoftherelationshipbetweenxandydependsonthevalueofx;theestimatedslopeisˆ12ˆ2x.Ifwepluginx0,weseethatˆ1canbeinterpretedastheapproximateslopeingoingfromx0tox1.Afterthat,thesecondterm,2ˆ2x,mustbeaccountedfor.Ifweareonlyinterestedincomputingthepredictedchangeinygivenastartingvalueforxandachangeinx,wecoulduse(6.10)directly:thereisnoreasontousethecalculusapproximationatall.However,weareusuallymoreinterestedinquicklysum-marizingtheeffectofxony,andtheinterpretationofˆ1andˆ2inequation(6.11)pro-videsthatsummary.Typically,wemightplugtheaveragevalueofxinthesample,orsomeotherinterestingvalues,suchasthemedianorthelowerandupperquartilevalues.Inmanyapplications,ˆ1ispositive,andˆ2isnegative.Forexample,usingthewagedatainWAGE1.RAW,weobtain2wageˆ(3.73)(.298)exper(.0061)exper2wageˆ(0.35)(.041)exper(.0009)exper(6.12)2n526,R.093.Thisestimatedequationimpliesthatexperhasadiminishingeffectonwage.Thefirstyearofexperienceisworthroughly30centsperhour(.298dollars).Thesecondyearofexperienceisworthless[about.2982(.0061)(1).286,or28.6cents,accordingtheapproximationin(6.11)withx1].Ingoingfrom10to11yearsofexperience,wageispredictedtoincreasebyabout.2982(.0061)(10).176,or17.6cents.Andsoon.2Whenthecoefficientonxispositive,andthecoefficientonxisnegative,thequa-dratichasaparabolicshape.Thereisalwaysapositivevalueofx,wheretheeffectofxonyiszero;beforethispoint,xhasapositiveeffectony;afterthispoint,xhasanegativeeffectony.Inpractice,itcanbeimportanttoknowwherethisturningpointis.186\nd7/14/995:33PMPage187Chapter6MultipleRegressionAnalysis:FurtherIssuesIntheestimatedequation(6.10)withˆ10andˆ20,theturningpoint(ormax-imumofthefunction)isalwaysachievedatthecoefficientonxovertwicetheabsolute2valueofthecoefficientonx:*xˆ1/(2ˆ2).(6.13)**Inthewageexample,xexperis.298/[2(.0061)]24.4.(Notehowwejustdroptheminussignon.0061indoingthiscalculation.)Thisquadraticrelationshipisillus-tratedinFigure6.1.Inthewageequation(6.12),thereturntoexperiencebecomeszeroatabout24.4years.Whatshouldwemakeofthis?Thereareatleastthreepossibleexplanations.First,itmaybethatfewpeopleinthesamplehavemorethan24yearsofexperience,andsothepartofthecurvetotherightof24canbeignored.Thecostofusingaqua-dratictocapturediminishingeffectsisthatthequadraticmusteventuallyturnaround.Ifthispointisbeyondallbutasmallpercentageofthepeopleinthesample,thenthisisnotofmuchconcern.ButinthedatasetWAGE1.RAW,about28%ofthepeopleinthesamplehavemorethan24yearsofexperience;thisistoohighapercentagetoignore.Itispossiblethatthereturntoexperreallybecomenegativeatsomepoint,butitishardtobelievethatthishappensat24yearsofexperience.Amorelikelypossibil-Figure6.1Quadraticrelationshipbetweenwageandexper.wage7.373.7324.4exper187\nd7/14/995:33PMPage188Part1RegressionAnalysiswithCross-SectionalDataityisthattheestimatedeffectofexperonwageisbiased,becausewehavecontrolledfornootherfactors,orbecausethefunctionalrelationshipbetweenwageandexperinequation(6.12)isnotentirelycorrect.Problem6.9asksyoutoexplorethispossibil-itybycontrollingforeducation,inadditiontousinglog(wage)asthedependentvari-able.Whenamodelhasadependentvariableinlogarthmicformandanexplanatoryvari-ableenteringasaquadratic,somecareisneededinmakingausefulinterpretation.ThefollowingexamplealsoshowsthequadraticcanhaveaU-shape,ratherthanaparabolicshape.AU-shapearisesintheequation(6.10)whenˆ1isnegativeandˆ2ispositive;thiscapturesanincreasingeffectofxony.EXAMPLE6.2(EffectsofPollutiononHousingPrices)WemodifythehousingpricemodelfromExample4.5toincludeaquadraticterminrooms:log(price)01log(nox)2log(dist)3rooms2(6.14)4rooms5stratiou.ThemodelestimatedusingthedatainHPRICE2.RAWislog(priceˆ)(13.39)(.902)log(nox)(.087)log(dist)log(priˆce)(0.57)(.115)log(nox)(.043)log(dist)2(.545)rooms(.062)rooms(.048)stratio2(.165)rooms(.013)rooms(.006)stratio2n506,R.603.2Thequadratictermroomshasatstatisticofabout4.77,andsoitisverystatisticallysig-nificant.Butwhataboutinterpretingtheeffectofroomsonlog(price)?Initially,theeffectappearstobestrange.Sincethecoefficientonroomsisnegativeandthecoefficienton2roomsispositive,thisequationliterallyimpliesthat,atlowvaluesofrooms,anadditionalroomhasanegativeeffectonlog(price).Atsomepoint,theeffectbecomespositive,andthequadraticshapemeansthatthesemi-elasticityofpricewithrespecttoroomsisincreas-ingasroomsincreases.ThissituationisshowninFigure6.2.Weobtaintheturnaroundvalueofroomsusingequation(6.13)(eventhoughˆ1isneg-ativeandˆ2ispositive).Theabsolutevalueofthecoefficientonrooms,.545,dividedby2*twicethecoefficientonrooms,.062,givesrooms.545/[2(.062)]4.4;thispointislabeledinFigure6.2.Dowereallybelievethatstartingatthreeroomsandincreasingtofourroomsactuallyreducesahouse’sexpectedvalue?Probablynot.Itturnsoutthatonlyfiveofthe506com-munitiesinthesamplehavehousesaveraging4.4roomsorless,about1%ofthesample.Thisissosmallthatthequadratictotheleftof4.4can,forpracticalpurposes,beignored.Totherightof4.4,weseethataddinganotherroomhasanincreasingeffectontheper-centagechangeinprice:ˆlog(price){[.5452(.062)]rooms}rooms188\nd7/14/995:33PMPage189Chapter6MultipleRegressionAnalysis:FurtherIssuesFigure6.2log(price)asaquadraticfunctionofrooms.log(price)4.4roomsandso%ˆprice100{[.5452(.062)]rooms}rooms(54.512.4rooms)rooms.Thus,anincreaseinroomsfrom,say,fivetosixincreasespricebyabout54.512.4(5)7.5%;theincreasefromsixtosevenincreasespricebyroughly54.512.4(6)19.9%.Thisisaverystrongincreasingeffect.Therearemanyotherpossibilitiesforusingquadraticsalongwithlogarithms.Forexample,anextensionof(6.14)thatallowsanonconstantelasticitybetweenpriceandnoxis2log(price)01log(nox)2[log(nox)]2(6.15)3crime4rooms5rooms6stratiou.189\nd7/14/995:33PMPage190Part1RegressionAnalysiswithCross-SectionalDataIf20,then1istheelasticityofpricewithrespecttonox.Otherwise,thiselastic-itydependsonthelevelofnox.Toseethis,wecancombinetheargumentsforthepar-tialeffectsinthequadraticandlogarithmicmodelstoshowthat%price[122log(nox)]%nox,(6.16)andthereforetheelasticityofpricewithrespecttonoxis122log(nox),sothatitdependsonlog(nox).Finally,otherpolynomialtermscanbeincludedinregressionmodels.Certainlythequadraticisseenmostoften,butacubicandevenaquartictermappearnowandthen.Anoftenreasonablefunctionalformforatotalcostfunctionis23cost01quantity2quantity3quantityu.Estimatingsuchamodelcausesnocomplications.Interpretingtheparametersismoreinvolved(thoughstraightforwardusingcalculus);wedonotstudythesemodelsfurther.ModelswithInteractionTermsSometimesitisnaturalforthepartialeffect,elasticity,orsemi-elasticityofthedepen-dentvariablewithrespecttoanexplanatoryvariabletodependonthemagnitudeofyetanotherexplanatoryvariable.Forexample,inthemodelprice01sqrft2bdrms3sqrftbdrms4bthrmsu,thepartialeffectofbdrmsonprice(holdingallothervariablesfixed)isprice23sqrft.(6.17)bdrmsIf30,then(6.17)impliesthatanadditionalbedroomyieldsahigherincreaseinhousingpriceforlargerhouses.Inotherwords,thereisaninteractioneffectbetweensquarefootageandnumberofbedrooms.Insummarizingtheeffectofbdrmsonprice,wemustevaluate(6.17)atinterestingvaluesofsqrft,suchasthemeanvalue,orthelowerandupperquartilesinthesample.Whetherornot3iszeroissomethingwecaneasilytest.EXAMPLE6.3(EffectsofAttendanceonFinalExamPerformance)Amodeltoexplainthestandardizedoutcomeonafinalexam(stndfnl)intermsofper-centageofclassesattended,priorcollegegradepointaverage,andACTscoreis2stndfnl01atndrte2priGPA3ACT4priGPA2(6.18)5ACT6priGPAatndrteu.(WeusethestandardizedexamscoreforthereasonsdiscussedinSection6.1:itiseasiertointerpretastudent’sperformancerelativetotherestoftheclass.)Inadditiontoquadratics190\nd7/14/995:33PMPage191Chapter6MultipleRegressionAnalysis:FurtherIssuesinpriGPAandACT,thismodelincludesaninteractionbetweenpriGPAandtheattendancerate.Theideaisthatclassattendancemighthaveadifferenteffectforstudentswhohaveperformeddifferentlyinthepast,asmeasuredbypriGPA.Weareinterestedintheeffectsofattendanceonfinalexamscore:stndfnl/atndrte16priGPA.Usingthe680observationsinATTEND.RAW,forstudentsinmicroeconomicprinciples,theestimatedequationisstndˆfnl(2.05)(.0067)atndrte(1.63)priGPA(.128)ACTstndˆfnl(1.36)(.0102)atndrte(0.48)priGPA(.098)ACT22(.296)priGPA(.0045)ACT(.0056)priGPAatndrte22(6.19)(.101)priGPA(.0022)ACT(.0043)priGPAatndrte22n680,R.229,R¯.222.Wemustinterpretthisequationwithextremecare.Ifwesimplylookatthecoefficientonatndrte,wewillincorrectlyconcludethatattendancehasanegativeeffectonfinalexamscore.ButthiscoefficientsupposedlymeasurestheeffectwhenpriGPA0,whichisnotinteresting(inthissample,thesmallestpriorGPAisabout.86).Wemustalsotakecarenottolookseparatelyattheestimatesof1and6andconcludethat,becauseeachtstatisticisinsignificant,wecannotrejectH0:10,60.Infact,thep-valuefortheFtestofthisjointhypothesisis.014,sowecertainlyrejectH0atthe5%level.Thisisagoodexam-pleofwherelookingatseparatetstatisticswhentestingajointhypothesiscanleadonefarastray.Howshouldweestimatethepartialeffectofatndrteonstndfnl?Wemustpluginin-terestingvaluesofpriGPAtoobtainthepartialeffect.ThemeanvalueofpriGPAinthesampleis2.59,soatthemeanpriGPA,theeffectofatndrteonstndfnlis.0067.0056(2.59).0078.Whatdoesthismean?Becauseatndrteismeasuredasapercent,itmeansthata10percentagepointincreaseinatndrteincreasesstndˆfnlby.078standarddeviationsfromthemeanfinalexamscore.Howcanwetellwhethertheestimate.0078isstatisticallydifferentfromzero?Weneedtoreruntheregression,wherewereplacepriGPAatndrtewith(priGPA2.59)atndrte.Thisgives,asthenewcoefficientonatndrte,theestimatedeffectatpriGPA2.59,alongwithitsstandarderror;nothingelseintheregressionchanges.(WedescribedthisdeviceinSection4.4.)Runningthisnewregressiongivesthestandarderrorofˆ1ˆ6(2.59).0078as.0026,whichyieldst.0078/.00263.Therefore,attheaverageQUESTION6.3priGPA,weconcludethatattendancehasaIfweaddtheterm7ACTatndrtetoequation(6.18),whatisthestatisticallysignificantpositiveeffectonfinalpartialeffectofatndrteonstndfnl?examscore.Thingsareevenmorecomplicatedfor2findingtheeffectofpriGPAonstndfnlbecauseofthequadratictermpriGPA.TofindtheeffectatthemeanvalueofpriGPAandthemeanattendancerate,.82,wewouldreplace22priGPAwith(priGPA2.59)andpriGPAatndrtewithpriGPA(atndrte.82).Thecoef-ficientonpriGPAbecomesthepartialeffectatthemeanvalues,andwewouldhaveitsstandarderror.(SeeProblem6.14.)191\nd7/14/995:33PMPage192Part1RegressionAnalysiswithCross-SectionalData6.3MOREONGOODNESS-OF-FITANDSELECTIONOFREGRESSORS2Untilnow,wehavenotfocusedmuchonthesizeofRinevaluatingourregressionmodels,becausebeginningstudentstendtoputtoomuchweightonR-squared.Aswewillseenow,choosingasetofexplanatoryvariablesbasedonthesizeoftheR-squaredcanleadtononsensicalmodels.InChapter10,wewilldiscoverthatR-squaredsobtainedfromtimeseriesregressionscanbeartificiallyhighandcanresultinmislead-ingconclusions.2NothingabouttheclassicallinearmodelassumptionsrequiresthatRbeaboveany2particularvalue;Rissimplyanestimateofhowmuchvariationinyisexplainedbyx1,x2,…,xkinthepopulation.WehaveseenseveralregressionsthathavehadprettysmallR-squareds.Whilethismeansthatwehavenotaccountedforseveralfactorsthataffecty,thisdoesnotmeanthatthefactorsinuarecorrelatedwiththeindependentvariables.ThezeroconditionalmeanassumptionMLR.3iswhatdetermineswhetherwegetunbi-asedestimatorsoftheceterisparibuseffectsoftheindependentvariables,andthesizeoftheR-squaredhasnodirectbearingonthis.Remember,though,thattherelativechangeintheR-squared,whenvariablesareaddedtoanequation,isveryuseful:theFstatisticin(4.41)fortestingthejointsignif-icancecruciallydependsonthedifferenceinR-squaredsbetweentheunrestrictedandrestrictedmodels.AdjustedR-SquaredMostregressionpackageswillreport,alongwiththeR-squared,astatisticcalledtheadjustedR-squared.SincetheadjustedR-squaredisreportedinmuchappliedwork,andsinceithassomeusefulfeatures,wecoveritinthissubsection.ToseehowtheusualR-squaredmightbeadjusted,itisusefullywrittenas2R1(SSR/n)/(SST/n),(6.20)whereSSRisthesumofsquaredresidualsandSSTisthetotalsumofsquares;com-paredwithequation(3.28),allwehavedoneisdividebothSSRandSSTbyn.This22expressionrevealswhatRisactuallyestimating.Defineyasthepopulationvariance2ofyandletudenotethepopulationvarianceoftheerrorterm,u.(Untilnow,wehave22usedtodenoteu,butitishelpfultobemorespecifichere.)Thepopulation22R-squaredisdefinedas1u/y;thisistheproportionofthevariationinyinthe2populationexplainedbytheindependentvariables.ThisiswhatRissupposedtobeestimating.22RestimatesubySSR/n,whichweknowtobebiased.SowhynotreplaceSSR/nwithSSR/(nk1)?Also,wecanuseSST/(n1)inplaceofSST/n,astheformer2istheunbiasedestimatorofy.Usingtheseestimators,wearriveattheadjustedR-squared:2R¯1[SSR/(nk1)]/[SST/(n1)]2(6.21)1ˆ/[SST/(n1)],192\nd7/14/995:33PMPage193Chapter6MultipleRegressionAnalysis:FurtherIssues2sinceˆSSR/(nk1).BecauseofthenotationusedtodenotetheadjustedR-squared,itissometimescalledR-barsquared.TheadjustedR-squaredissometimescalledthecorrectedR-squared,butthisisnot22agoodnamebecauseitimpliesthatR¯issomehowbetterthanRasanestimatorofthe2populationR-squared.Unfortunately,R¯isnotgenerallyknowntobeabetterestima-22tor.ItistemptingtothinkthatR¯correctsthebiasinRforestimatingthepopulationR-squared,butitdoesnot:theratiooftwounbiasedestimatorsisnotanunbiasedesti-mator.2TheprimaryattractivenessofR¯isthatitimposesapenaltyforaddingadditional2independentvariablestoamodel.WeknowthatRcanneverfallwhenanewindepen-dentvariableisaddedtoaregressionequation:thisisbecauseSSRnevergoesup(and2usuallyfalls)asmoreindependentvariablesareadded.ButtheformulaforR¯showsthatitdependsexplicitlyonk,thenumberofindependentvariables.Ifanindependentvariableisaddedtoaregression,SSRfalls,butsodoesthedfintheregression,nk1.SSR/(nk1)cangoupordownwhenanewindependentvariableisaddedtoaregression.Aninterestingalgebraicfactisthefollowing:ifweaddanewindependentvariable2toaregressionequation,R¯increasesif,andonlyif,thetstatisticonthenewvariable2isgreaterthanoneinabsolutevalue.(AnextensionofthisisthatR¯increaseswhenagroupofvariablesisaddedtoaregressionif,andonlyif,theFstatisticforjointsig-nificanceofthenewvariablesisgreaterthanunity.)Thus,weseeimmediatelythat2usingR¯todecidewhetheracertainindependentvariable(orsetofvariables)belongsinamodelgivesusadifferentanswerthanstandardtorFtesting(sinceatorFstatis-ticofunityisnotstatisticallysignificantattraditionalsignificancelevels).22ItissometimesusefultohaveaformulaforR¯intermsofR.Simplealgebragives22R¯1(1R)(n1)/(nk1).(6.22)22Forexample,ifR.30,n51,andk10,thenR¯1.70(50)/40.125.Thus,22forsmallnandlargek,R¯canbesubstantiallybelowR.Infact,iftheusualR-squared2issmall,andnk1issmall,R¯canactuallybenegative!Forexample,youcanplug222inR.10,n51,andk10toverifythatR¯.125.AnegativeR¯indicatesaverypoormodelfitrelativetothenumberofdegreesoffreedom.TheadjustedR-squaredissometimesreportedalongwiththeusualR-squaredin22regressions,andsometimesR¯isreportedinplaceofR.Itisimportanttoremember222thatitisR,notR¯,thatappearsintheFstatisticin(4.41).ThesameformulawithR¯r2andR¯urisnotvalid.UsingAdjustedR-SquaredtoChooseBetweenNonnestedModelsInSection4.5,welearnedhowtocomputeanFstatisticfortestingthejointsignifi-canceofagroupofvariables;thisallowsustodecide,ataparticularsignificancelevel,whetheratleastonevariableinthegroupaffectsthedependentvariable.Thistestdoesnotallowustodecidewhichofthevariableshasaneffect.Insomecases,wewantto193\nd7/14/995:33PMPage194Part1RegressionAnalysiswithCross-SectionalDatachooseamodelwithoutredundantindependentvariables,andtheadjustedR-squaredcanhelpwiththis.InthemajorleaguebaseballsalaryexampleinSection4.4,wesawthatneitherhrunsyrnorrbisyrwasindividuallysignificant.Thesetwovariablesarehighlycorre-lated,sowemightwanttochoosebetweenthemodelslog(salary)01years2gamesyr3bavg4hrunsyruandlog(salary)01years2gamesyr3bavg4rbisyru.Thesetwoexamplesarenonnestedmodels,becauseneitherequationisaspecialcaseoftheother.TheFstatisticswestudiedinChapter4onlyallowustotestnestedmod-els:onemodel(therestrictedmodel)isaspecialcaseoftheothermodel(theunre-strictedmodel).Seeequations(4.32)and(4.28)forexamplesofrestrictedandunrestrictedmodels.OnepossibilityistocreateacompositemodelthatcontainsallexplanatoryvariablesfromtheoriginalmodelsandthentotesteachmodelagainstthegeneralmodelusingtheFtest.Theproblemwiththisprocessisthateitherbothmod-elsmightberejected,orneithermodelmightberejected(ashappenswiththemajorleaguebaseballsalaryexampleinSection4.4).Thus,itdoesnotalwaysprovideawaytodistinguishbetweenmodelswithnonnestedregressors.2Inthebaseballplayersalaryregression,R¯fortheregressioncontaininghrunsyris2.6211,andR¯fortheregressioncontainingrbisyris.6226.Thus,basedontheadjustedR-squared,thereisaveryslightpreferenceforthemodelwithrbisyr.Butthedifferenceispracticallyverysmall,andwemightobtainadifferentanswerbycontrollingforsomeofthevariablesinProblem4.16.(Becausebothnonnestedmodelscontainfiveparameters,theusualR-squaredcanbeusedtodrawthesameconclusion.)2ComparingR¯tochooseamongdifferentnonnestedsetsofindependentvariablescanbevaluablewhenthesevariablesrepresentdifferentfunctionalforms.ConsidertwomodelsrelatingR&Dintensitytofirmsales:rdintens01log(sales)u.(6.23)2rdintens01sales2salesu.(6.24)Thefirstmodelcapturesadiminishingreturnbyincludingsalesinlogarithmicform;thesecondmodeldoesthisbyusingaquadratic.Thus,thesecondmodelcontainsonemoreparameterthanthefirst.Whenequation(6.23)isestimatedusingthe32observationsonchemicalfirmsin22RDCHEM.RAW,Ris.061,andRforequation(6.24)is.148.Therefore,itappearsthatthequadraticfitsmuchbetter.ButacomparisonoftheusualR-squaredsisunfairtothefirstmodelbecauseitcontainsonelessparameterthan(6.24).Thatis,(6.23)isamoreparsimoniousmodelthan(6.24).Everythingelsebeingequal,simplermodelsarebetter.SincetheusualR-squared22doesnotpenalizemorecomplicatedmodels,itisbettertouseR¯.R¯for(6.23)is.030,2whileR¯for(6.24)is.090.Thus,evenafteradjustingforthedifferenceindegreesoffreedom,thequadraticmodelwinsout.Thequadraticmodelisalsopreferredwhenprofitmarginisaddedtoeachregression.194\nd7/14/995:33PMPage195Chapter6MultipleRegressionAnalysis:FurtherIssues2ThereisanimportantlimitationinusingR¯tochoosebetweennonnestedmodels:wecannotuseittochoosebetweendifferentfunctionalformsforthedependentvariable.Thisisunfortunate,becauseweoftenwanttodecideonwhetheryorlog(y)(ormaybesomeothertransformation)shouldbeusedasthedependentvariablebasedonQUESTION6.4goodness-of-fit.ButneitherR2norR¯2canExplainwhychoosingamodelbymaximizingR¯2orminimizingˆbeusedforthis.Thereasonissimple:(thestandarderroroftheregression)isthesamething.theseR-squaredsmeasuretheexplainedproportionofthetotalvariationinwhat-everdependentvariableweareusingintheregression,anddifferentfunctionsofthedependentvariablewillhavedifferentamountsofvariationtoexplain.Forexample,thetotalvariationsinyandlog(y)arenotthesame.ComparingtheadjustedR-squaredsfromregressionswiththesedifferentformsofthedependentvariablesdoesnottellusany-thingaboutwhichmodelfitsbetter;theyarefittingtwoseparatedependentvariables.EXAMPLE6.4(CEOCompensationandFirmPerformance)ConsidertwoestimatedmodelsrelatingCEOcompensationtofirmperformance:salˆary(830.63)(.0163)sales(19.63)roesalˆary(223.90)(.0089)sales(11.08)roe(6.25)22n209,R.029,R¯.020andlsalˆary(4.36)(.275)lsales(.0179)roelsalˆary(0.29)(.033)lsales(.0040)roe(6.26)22n209,R.282,R¯.275,whereroeisthereturnonequitydiscussedinChapter2.Forsimplicity,lsalaryandlsalesdenotethenaturallogsofsalaryandsales.Wealreadyknowhowtointerpretthesedif-ferentestimatedequations.Butcanwesaythatonemodelfitsbetterthantheother?TheR-squaredforequation(6.25)showsthatsalesandroeexplainonlyabout2.9%ofthevariationinCEOsalaryinthesample.Bothsalesandroehavemarginalstatisticalsig-nificance.Equation(6.26)showsthatlog(sales)androeexplainabout28.2%ofthevariationinlog(salary).Intermsofgoodness-of-fit,thismuchhigherR-squaredwouldseemtoimplythatmodel(6.26)ismuchbetter,butthisisnotnecessarilythecase.Thetotalsumofsquaresforsalaryinthesampleis391,732,982,whilethetotalsumofsquaresforlog(salary)isonly66.72.Thus,thereismuchlessvariationinlog(salary)thatneedstobeexplained.22Atthispoint,wecanusefeaturesotherthanRorR¯todecidebetweenthesemodels.Forexample,log(sales)androearemuchmorestatisticallysignificantin(6.26)thanaresalesandroein(6.25),andthecoefficientsin(6.26)areprobablyofmoreinterest.Tobesure,however,wewillneedtomakeavalidgoodness-of-fitcomparison.195\nd7/14/995:33PMPage196Part1RegressionAnalysiswithCross-SectionalDataInSection6.4,wewillofferagoodness-of-fitmeasurethatdoesallowustocom-paremodelswhereyappearsinbothlevelandlogform.ControllingforTooManyFactorsinRegressionAnalysisInmanyoftheexampleswehavecovered,andcertainlyinourdiscussionofomittedvariablesbiasinChapter3,wehaveworriedaboutomittingimportantfactorsfromamodelthatmightbecorrelatedwiththeindependentvariables.Itisalsopossibletocon-trolfortoomanyvariablesinaregressionanalysis.Ifweoveremphasizegoodness-of-fit,weopenourselvestocontrollingforfactorsinaregressionmodelthatshouldnotbecontrolledfor.Toavoidthismistake,weneedtoremembertheceterisparibusinterpretationofmultipleregressionmodels.Toillustratethisissue,supposewearedoingastudytoassesstheimpactofstatebeertaxesontrafficfatalities.Theideaisthatahighertaxonbeerwillreducealcoholconsumption,andlikewisedrunkdriving,resultinginfewertrafficfatalities.Tomea-suretheceterisparibuseffectoftaxesonfatalities,wecanmodelfatalitiesasafunc-tionofseveralfactors,includingthebeertax:fatalities01tax2miles3percmale4perc16_21…,wheremilesistotalmilesdriven,percmaleispercentofthestatepopulationthatismale,andperc16_21ispercentofthepopulationbetweenages16and21,andsoon.Noticehowwehavenotincludedavariablemeasuringpercapitabeerconsumption.Arewecommittinganomittedvariableserror?Theanswerisno.Ifwecontrolforbeerconsumptioninthisequation,thenhowwouldbeertaxesaffecttrafficfatalities?Intheequationfatalities01tax2beercons…,1measuresthedifferenceinfatalitiesduetoaonepercentagepointincreaseintax,holdingbeerconsfixed.Itisdifficulttounderstandwhythiswouldbeinteresting.Weshouldnotbecontrollingfordifferencesinbeerconsacrossstates,unlesswewanttotestforsomesortofindirecteffectofbeertaxes.Otherfactors,suchasgenderandagedistribution,shouldbecontrolledfor.Theissueofwhetherornottocontrolforcertainfactorsisnotalwaysclear-cut.Forexample,Betts(1995)studiestheeffectofhighschoolqualityonsubsequentearnings.Hepointsoutthat,ifbetterschoolqualityresultsinmoreeducation,thencontrollingforeducationintheregressionalongwithmeasuresofqualitywillunderestimatethereturntoquality.Bettsdoestheanalysiswithandwithoutyearsofeducationintheequationtogetarangeofestimatedeffectsforqualityofschooling.ToseeexplicitlyhowfocusingonhighR-squaredscanleadtotrouble,considerthehousingpriceexamplefromSection4.5thatillustratesthetestingofmultiplehypothe-ses.Inthatcase,wewantedtotesttherationalityofhousingpriceassessments.Weregressedlog(price)onlog(assess),log(lotsize),log(sqrft),andbdrmsandtestedwhetherthelatterthreevariableshadzeropopulationcoefficientswhilelog(assess)hadacoefficientofunity.Butwhatifwewanttoestimateahedonicpricemodel,asinExample4.8,wherethemarginalvaluesofvarioushousingattributesareobtained?Shouldweincludelog(assess)intheequation?TheadjustedR-squaredfromtheregres-196\nd7/14/995:33PMPage197Chapter6MultipleRegressionAnalysis:FurtherIssuessionwithlog(assess)is.762,whiletheadjustedR-squaredwithoutitis.630.Basedongoodness-of-fitonly,weshouldincludelog(assess).Butthisisincorrectifourgoalistodeterminetheeffectsoflotsize,squarefootage,andnumberofbedroomsonhous-ingvalues.Includinglog(assess)intheequationamountstoholdingonemeasureofvaluefixedandthenaskinghowmuchanadditionalbedroomwouldchangeanothermeasureofvalue.Thismakesnosenseforvaluinghousingattributes.Ifwerememberthatdifferentmodelsservedifferentpurposes,andwefocusontheceterisparibusinterpretationofregression,thenwewillnotincludethewrongfactorsinaregressionmodel.AddingRegressorstoReducetheErrorVarianceWehavejustseensomeexamplesofwherecertainindependentvariablesshouldnotbeincludedinaregressionmodel,eventhoughtheyarecorrelatedwiththedependentvari-able.FromChapter3,weknowthataddinganewindependentvariabletoaregressioncanexacerbatethemulticollinearityproblem.Ontheotherhand,sincewearetakingsomethingoutoftheerrorterm,addingavariablegenerallyreducestheerrorvariance.Generally,wecannotknowwhicheffectwilldominate.However,thereisonecasethatisobvious:weshouldalwaysincludeindependentvariablesthataffectyandareuncorrelatedwithalloftheindependentvariablesofinterest.Thereasonforthisinclusionissimple:addingsuchavariabledoesnotinducemulticollinearityinthepopulation(andthereforemulticollinearityinthesampleshouldbenegligible),butitwillreducetheerrorvariance.Inlargesamplesizes,thestandarderrorsofallOLSestimatorswillbereduced.Asanexample,considerestimatingtheindividualdemandforbeerasafunctionoftheaveragecountybeerprice.Itmaybereasonabletoassumethatindividualcharac-teristicsareuncorrelatedwithcounty-levelprices,andsoasimpleregressionofbeerconsumptiononcountypricewouldsufficeforestimatingtheeffectofpriceonindi-vidualdemand.Butitispossibletogetamorepreciseestimateofthepriceelasticityofbeerdemandbyincludingindividualcharacteristics,suchasageandamountofedu-cation.Ifthesefactorsaffectdemandandareuncorrelatedwithprice,thenthestandarderrorofthepricevariablewillbesmaller,atleastinlargesamples.Unfortunately,caseswherewehaveinformationonadditionalexplanatoryvariablesthatareuncorrelatedwiththeexplanatoryvariablesofinterestarerareinthesocialsci-ences.Butitisworthrememberingthatwhenthesevariablesareavailable,theycanbeincludedinamodeltoreducetheerrorvariancewithoutinducingmulticollinearity.6.4PREDICTIONANDRESIDUALANALYSISInChapter3,wedefinedtheOLSpredictedorfittedvaluesandtheOLSresiduals.Predictionsarecertainlyuseful,buttheyaresubjecttosamplingvariation,sincetheyareobtainedusingtheOLSestimators.Thus,inthissection,weshowhowtoobtainconfidenceintervalsforapredictionfromtheOLSregressionline.FromChapters3and4,weknowthattheresidualsareusedtoobtainthesumofsquaredresidualsandtheR-squared,sotheyareimportantforgoodness-of-fitandtest-ing.Sometimeseconomistsstudytheresidualsforparticularobservationstolearnaboutindividuals(orfirms,houses,etc.)inthesample.197\nd7/14/995:33PMPage198Part1RegressionAnalysiswithCross-SectionalDataConfidenceIntervalsforPredictionsSupposewehaveestimatedtheequationyˆˆ0ˆ1x1ˆ2x2…ˆkxk.(6.27)Whenwepluginparticularvaluesoftheindependentvariables,weobtainapredictionfory,whichisanestimateoftheexpectedvalueofygiventheparticularvaluesfortheexplanatoryvariables.Foremphasis,letc1,c2,…,ckdenoteparticularvaluesforeachofthekindependentvariables;thesemayormaynotcorrespondtoanactualdatapointinoursample.Theparameterwewouldliketoestimateis001c12c2…kck(6.28)E(yx1c1,x2c2,…,xkck).Theestimatorof0isˆ0ˆ0ˆ1c1ˆ2c2…ˆkck.(6.29)Inpractice,thisiseasytocompute.Butwhatifwewantsomemeasureoftheuncer-taintyinthispredictedvalue?Itisnaturaltoconstructaconfidenceintervalfor0,whichiscenteredatˆ0.Toobtainaconfidenceintervalfor0,weneedastandarderrorforˆ0.Then,withalargedf,wecanconstructa95%confidenceintervalusingtheruleofthumbˆ02se(ˆ0).(Asalways,wecanusetheexactpercentilesinatdistribution.)Howdoweobtainthestandarderrorofˆ0?Thisisthesameproblemweencoun-teredinSection4.4:weneedtoobtainastandarderrorforalinearcombinationoftheOLSestimators.Here,theproblemisevenmorecomplicated,becausealloftheOLSestimatorsgenerallyappearinˆ0(unlesssomecjarezero).Nevertheless,thesametrickthatweusedinSection4.4willworkhere.Write001c1…kckandplugthisintotheequationy01x1…kxkutoobtainy01(x1c1)2(x2c2)…k(xkck)u.(6.30)Inotherwords,wesubtractthevaluecjfromeachobservationonxj,andthenweruntheregressionofyion(xi1c1),…,(xikck),i1,2,…,n.(6.31)Thepredictedvaluein(6.29)and,moreimportantly,itsstandarderror,areobtainedfromtheintercept(orconstant)inregression(6.31).Asanexample,weobtainaconfidenceintervalforapredictionfromacollegeGPAregression,whereweusehighschoolinformation.198\nd7/14/995:33PMPage199Chapter6MultipleRegressionAnalysis:FurtherIssuesEXAMPLE6.5(ConfidenceIntervalforPredictedCollegeGPA)UsingthedatainGPA2.RAW,weobtainthefollowingequationforpredictingcollegeGPA:colˆgpa(1.493)(.00149)sat(.01386)hsperccolˆgpa(0.075)(.00007)sat(.00056)hsperc2(.06088)hsize(.00546)hsize2(6.32)(.01650)hsize(.00227)hsize22n4,137,R.278,R¯.277,ˆ.560,wherewehavereportedestimatestoseveraldigitstoreduceround-offerror.Whatispre-dictedcollegeGPA,whensat1,200,hsperc30,andhsize5(whichmeans500)?Thisiseasytogetbypluggingthesevaluesintoequation(6.32):colˆgpa2.70(roundedtotwodigits).Unfortunately,wecannotuseequation(6.32)directlytogetaconfidenceintervalfortheexpectedcolgpaatthegivenvaluesoftheindependentvariables.Onesim-plewaytoobtainaconfidenceintervalistodefineanewsetofindependentvariables:2sat0sat1,200,hsperc0hsperc30,hsize0hsize5,andhsizesq0hsize25.Whenweregresscolgpaonthesenewindependentvariables,wegetcolˆgpa(2.700)(.00149)sat0(.01386)hsperc0colˆgpa(0.020)(.00007)sat0(.00056)hsperc0(.06088)hsize0(.00546)hsizesq0(.01650)hsize0(.00227)hsizesq022n4,137,R.278,R¯.277,ˆ.560.Theonlydifferencebetweenthisregressionandthatin(6.32)istheintercept,whichisthepredictionwewant,alongwithitsstandarderror,.020.Itisnotanaccidentthattheslopecoefficents,theirstandarderrors,R-squared,andsoonarethesameasbefore;thispro-videsawaytocheckthatthepropertransformationsweredone.Wecaneasilyconstructa95%confidenceintervalfortheexpectedcollegeGPA:2.701.96(.020)orabout2.66to2.74.Thisconfidenceintervalisrathernarrowduetotheverylargesamplesize.Becausethevarianceoftheinterceptestimatorissmallestwheneachexplanatoryvariablehaszerosamplemean(seeQuestion2.5forthesimpleregressioncase),itfol-lowsfromtheregressionin(6.31)thatthevarianceofthepredictionissmallestatthemeanvaluesofthexj.(Thatis,cjx¯jforallj.)Thisresultisnottoosurprising,sincewehavethemostfaithinourregressionlinenearthemiddleofthedata.Asthevaluesofthecjgetfartherawayfromthex¯j,Var(yˆ)getslargerandlarger.ThepreviousmethodallowsustoputaconfidenceintervalaroundtheOLSesti-mateofE(yx1,…,xk),foranyvaluesoftheexplanatoryvariables.Butthisisnotthesameasobtainingaconfidenceintervalforanew,asyetunknown,outcomeony.Informingaconfidenceintervalforanoutcomeony,wemustaccountforanotherveryimportantsourceofvariation:thevarianceintheunobservederror.199\nd7/14/995:33PMPage200Part1RegressionAnalysiswithCross-SectionalData0Letydenotethevalueforwhichwewouldliketoconstructaconfidenceinterval,0whichwesometimescallapredictioninterval.Forexample,ycouldrepresentaper-00sonorfirmnotinouroriginalsample.Letx1,…,xkbethenewvaluesoftheindepen-0dentvariables,whichweassumeweobserve,andletubetheunobservederror.Therefore,wehave00000y01x12x2…kxku.(6.33)00Asbefore,ourbestpredictionofyistheexpectedvalueofygiventheexplana-00toryvariables,whichweestimatefromtheOLSregressionline:yˆˆ0ˆ1x10000ˆ2x2…ˆkxk.Thepredictionerrorinusingyˆtopredictyis000000eˆyyˆ(01x1…kxk)uyˆ0.(6.34)000000Now,E(yˆ)E(ˆ0)E(ˆ1)x1E(ˆ2)x2…E(ˆk)xk01x1…kxk,becausetheˆjareunbiased.(Asbefore,theseexpectationsareallconditionalonthe00samplevaluesoftheindependentvariables.)Becauseuhaszeromean,E(eˆ)0.Wehaveshowedthattheexpectedpredictionerroriszero.000Infindingthevarianceofeˆ,notethatuisuncorrelatedwitheachˆj,becauseuisuncorrelatedwiththeerrorsinthesampleusedtoobtaintheˆj.Bybasicpropertiesof00covariance(seeAppendixB),uandyˆareuncorrelated.Therefore,thevarianceofthepredictionerror(conditionalonallin-samplevaluesoftheindependentvariables)isthesumofthevariances:00002Var(eˆ)Var(yˆ)Var(u)Var(yˆ),(6.35)200whereVar(u)istheerrorvariance.Therearetwosourcesofvarianceineˆ.The0firstisthesamplingerrorinyˆ,whicharisesbecausewehaveestimatedthej.Because0eachˆjhasavarianceproportionalto1/n,wherenisthesamplesize,Var(yˆ)ispro-0portionalto1/n.Thismeansthat,forlargesamples,Var(yˆ)canbeverysmall.Bycon-2trast,isthevarianceoftheerrorinthepopulation;itdoesnotchangewiththesample2size.Inmanyexamples,willbethedominanttermin(6.35).0Undertheclassicallinearmodelassumptions,theˆjanduarenormallydistributed,0andsoeˆisalsonormallydistributed(conditionalonallsamplevaluesoftheexplana-0toryvariables).Earlier,wedescribedhowtoobtainanunbiasedestimatorofVar(yˆ),2andweobtainedourunbiasedestimatorofinChapter3.Byusingtheseestimators,0wecandefinethestandarderrorofeˆas0221/2se(eˆ){[se(yˆ0)]ˆ}.(6.36)00Usingthesamereasoningforthetstatisticsoftheˆj,eˆ/se(eˆ)hasatdistributionwithn(k1)degreesoffreedom.Therefore,00P[t.025eˆ/se(eˆ)t.025].95,thwheret.025isthe97.5percentileinthetnk1distribution.Forlargenk1,000rememberthatt.0251.96.Pluggingineˆyyˆandrearranginggivesa95%0predictionintervalfory:200\nd7/14/995:33PMPage201Chapter6MultipleRegressionAnalysis:FurtherIssues00yˆt.025se(eˆ);(6.37)00asusual,exceptforsmalldf,agoodruleofthumbisyˆ2se(eˆ).Thisiswiderthan02theconfidenceintervalforyˆitself,becauseofˆin(6.36);itoftenismuchwiderto0reflectthefactorsinuthatwehavenotcontrolledfor.EXAMPLE6.6(ConfidenceIntervalforFutureCollegeGPA)Supposewewanta95%CIforthefuturecollegeGPAforahighschoolstudentwithsat1,200,hsperc30,andhsize5.Remember,inExample6.5weobtainedaconfi-denceintervalfortheexpectedGPA;nowwemustaccountfortheunobservedfactorsin0theerrorterm.WehaveeverythingweneedtoobtainaCIforcolgpa.se(yˆ).020andˆ0221/20.560andso,from(6.36),se(eˆ)[(.020)(.560)].560.Noticehowsmallse(yˆ)00isrelativetoˆ:virtuallyallofthevariationineˆcomesfromthevariationinu.The95%CIis2.701.96(.560)orabout1.60to3.80.Thisisawideconfidenceinterval,anditshowsthat,basedonthefactorsusedintheregression,wecannotsignificantlynarrowthelikelyrangeofcollegeGPA.ResidualAnalysisSometimesitisusefultoexamineindividualobservationstoseewhethertheactualvalueofthedependentvariableisaboveorbelowthepredictedvalue;thatis,toexam-inetheresidualsfortheindividualobservations.Thisprocessiscalledresidualanaly-sis.Economistshavebeenknowntoexaminetheresidualsfromaregressioninordertoaidinthepurchaseofahome.Thefollowinghousingpriceexampleillustratesresidualanalysis.Housingpriceisrelatedtovariousobservablecharacteristicsofthehouse.Wecanlistallofthecharacteristicsthatwefindimportant,suchassize,numberofbed-rooms,numberofbathrooms,andsoon.Wecanuseasampleofhousestoestimatearelationshipbetweenpriceandattributes,whereweendupwithapredictedvalueandanactualvalueforeachhouse.Then,wecanconstructtheresiduals,uˆiyiyˆi.Thehousewiththemostnegativeresidualis,atleastbasedonthefactorswehavecontrolledfor,themostunderpricedonerelativetoitscharacteristics.Italsomakessensetocom-puteaconfidenceintervalforwhatthefuturesellingpriceofthehomecouldbe,usingthemethoddescribedinequation(6.37).UsingthedatainHPRICE1.RAW,werunaregressionofpriceonlotsize,sqrft,andstbdrms.Inthesampleof88homes,themostnegativeresidualis120.206,forthe81house.Therefore,theaskingpriceforthishouseis$120,206belowitspredictedprice.Therearemanyotherusesofresidualanalysis.Onewaytoranklawschoolsistoregressmedianstartingsalaryonavarietyofstudentcharacteristics(suchasmedianLSATscoresofenteringclass,mediancollegeGPAofenteringclass,andsoon)andtoobtainapredictedvalueandresidualforeachlawschool.Thelawschoolwiththelargestresidualhasthehighestpredictedvalueadded.(Ofcourse,thereisstillmuchuncertaintyabouthowanindividual’sstartingsalarywouldcomparewiththemedianforalawschooloverall.)Theseresidualscanbeusedalongwiththecostsofattending201\nd7/14/995:33PMPage202Part1RegressionAnalysiswithCross-SectionalDataeachlawschooltodeterminethebestvalue;thiswouldrequireanappropriatedis-countingoffutureearnings.Residualanalysisalsoplaysaroleinlegaldecisions.ANewYorkTimesarticleenti-tled“JudgeSaysPupil’sPoverty,NotSegregation,HurtsScores”(6/28/95)describesanimportantlegalcase.TheissuewaswhetherthepoorperformanceonstandardizedtestsintheHartfordSchoolDistrict,relativetoperformanceinsurroundingsuburbs,wasduetopoorschoolqualityatthehighlysegregatedschools.Thejudgeconcludedthat“thedisparityintestscoresdoesnotindicatethatHartfordisdoinganinadequateorpoorjobineducatingitsstudentsorthatitsschoolsarefailing,becausethepre-QUESTION6.5dictedscoresbasedupontherelevantHowmightyouuseresidualanalysistodeterminewhichmoviesocioeconomicfactorsareaboutatthelev-actorsareoverpaidrelativetoboxofficeproduction?elsthatonewouldexpect.”Thisconclu-sionisalmostcertainlybasedonare-gressionanalysisofaverageormedianscoresonsocioeconomiccharacteristicsofvar-iousschooldistrictsinConnecticut.Thejudge’sconclusionsuggeststhat,giventhepovertylevelsofstudentsatHartfordschools,theactualtestscoresweresimilartothosepredictedfromaregressionanalysis:theresidualforHartfordwasnotsufficientlynegativetoconcludethattheschoolsthemselveswerethecauseoflowtestscores.PredictingyWhenlog(y)IstheDependentVariableSincethenaturallogtransformationisusedsooftenforthedependentvariableinempiricaleconomics,wedevotethissubsectiontotheissueofpredictingywhenlog(y)isthedependentvariable.Asabyproduct,wewillobtainagoodness-of-fitmeasureforthelogmodelthatcanbecomparedwiththeR-squaredfromthelevelmodel.Toobtainaprediction,itisusefultodefinelogylog(y);thisemphasizesthatitisthelogofythatispredictedinthemodellogy01x12x2…kxku.(6.38)Inthisequation,thexjmightbetransformationsofothervariables;forexample,wecouldhavex1log(sales),x2log(mktval),x3ceotenintheCEOsalaryexample.GiventheOLSestimators,weknowhowtopredictlogyforanyvalueoftheinde-pendentvariables:loˆgyˆ0ˆ1x1ˆ2x2…ˆkxk.(6.39)Now,sincetheexponentialundoesthelog,ourfirstguessforpredictingyistosimplyexponentiatethepredictedvalueforlog(y):yˆexp(loˆgy).Thisdoesnotwork;infact,itwillsystematicallyunderestimatetheexpectedvalueofy.Infact,ifmodel(6.38)fol-lowstheCLMassumptionsMLR.1throughMLR.6,itcanbeshownthat2E(yx)exp(/2)exp(01x12x2…kxk),2wherexdenotestheindependentvariablesandisthevarianceofu.[Ifu~22Normal(0,),thentheexpectedvalueofexp(u)isexp(/2).]Thisequationshowsthatasimpleadjustmentisneededtopredicty:202\nd7/14/995:33PMPage203Chapter6MultipleRegressionAnalysis:FurtherIssues2yˆexp(ˆ/2)exp(loˆgy),(6.40)22whereˆissimplytheunbiasedestimatorof.Sinceˆ,thestandarderrorofthe2regression,isalwaysreported,obtainingpredictedvaluesforyiseasy.Becauseˆ220,exp(ˆ/2)1.Forlargeˆ,thisadjustmentfactorcanbesubstantiallylargerthanunity.Thepredictionin(6.40)isnotunbiased,butitisconsistent.Therearenounbiasedpredictionsofy,andinmanycases,(6.40)workswell.However,itdoesrelyonthenor-malityoftheerrorterm,u.InChapter5,weshowedthatOLShasdesirableproperties,evenwhenuisnotnormallydistributed.Therefore,itisusefultohaveapredictionthatdoesnotrelyonnormality.Ifwejustassumethatuisindependentoftheexplanatoryvariables,thenwehaveE(yx)0exp(01x12x2…kxk),(6.41)where0istheexpectedvalueofexp(u),whichmustbegreaterthanunity.Givenanestimateˆ0,wecanpredictyasyˆˆ0exp(loˆgy),(6.42)whichagainsimplyrequiresexponentiatingthepredictedvaluefromthelogmodelandmultiplyingtheresultbyˆ0.Itturnsoutthataconsistentestimatorofˆ0iseasilyobtained.PREDICTINGyWHENTHEDEPENDENTVARIABLEISlog(y):(i)Obtainthefittedvaluesloˆgyifromtheregressionoflogyonx1,…,xk.(ii)Foreachobservationi,createmˆiexp(loˆgyi).(iii)Nowregressyonthesinglevariablemˆwithoutanintercept;thatis,performasimpleregressionthroughtheorigin.Thecoefficientonmˆ,theonlycoefficientthereis,istheestimateof0.Onceˆ0isobtained,itcanbeusedalongwithpredictionsoflogytopredicty.Thestepsareasfollows:(i)Forgivenvaluesofx1,x2,…,xk,obtainloˆgyfrom(6.39).(ii)Obtainthepredictionyˆfrom(6.42).EXAMPLE6.7(PredictingCEOSalaries)Themodelofinterestislog(salary)01log(sales)2log(mktval)3ceotenu,sothat1and2areelasticitiesand1003isasemi-elasticity.TheestimatedequationusingCEOSAL2.RAWis203\nd7/14/995:33PMPage204Part1RegressionAnalysiswithCross-SectionalDatalsalˆary(4.504)(.163)lsales(.109)lmktval(.0117)ceotenlsalˆary(0.257)(.039)lsales(.050)lmktval(.0053)ceoten(6.43)2n177,R.318,where,forclarity,weletlsalarydenotethelogofsalary,andsimilarlyforlsalesandlmktval.Next,weobtainmˆiexp(lsalˆaryi)foreachobservationinthesample.Regressingsalaryonmˆ(withoutaconstant)producesˆ01.117.Wecanusethisvalueofˆ0alongwith(6.43)topredictsalaryforanyvaluesofsales,mktval,andceoten.Letusfindthepredictionforsales5,000(whichmeans$5billion,sincesalesisinmillionsofdollars),mktval10,000(or$10billion),andceoten10.From(6.43),thepredictionforlsalaryis4.504.163log(5,000).109log(10,000).0117(10)7.013.Thepredictedsalaryistherefore1.117exp(7.013)1,240.967,or$1,240,967.Ifweforgettomultiplybyˆ01.117,wegetapredictionof$1,110,983.Wecanusethepreviousmethodofobtainingpredictionstodeterminehowwellthemodelwithlog(y)asthedependentvariableexplainsy.Wealreadyhavemeasuresformodelswhenyisthedependentvariable:theR-squaredandtheadjustedR-squared.Thegoalistofindagoodness-of-fitmeasureinthelog(y)modelthatcanbecomparedwithanR-squaredfromamodelwhereyisthedependentvariable.Thereareseveralwaystofindthismeasure,butwepresentanapproachthatiseasytoimplement.Afterrunningtheregressionofyonmˆthroughtheorigininstep(iii),weobtainthefittedvaluesforthisregression,yˆiˆ0mˆi.Then,wefindthesamplecorre-lationbetweenyˆiandtheactualyiinthesample.ThesquareofthiscanbecomparedwiththeR-squaredwegetbyusingyasthedependentvariableinalinearregressionmodel.RememberthattheR-squaredinthefittedequationyˆˆ0ˆ1x1…ˆkxkisjustthesquaredcorrelationbetweentheyiandtheyˆi(seeSection3.2).EXAMPLE6.8(PredictingCEOSalaries)Afterstep(iii)intheprecedingprocedure,weobtainthefittedvaluessalaˆryiˆ0mˆi.Thesimplecorrelationbetweensalaryiandsalaˆryiinthesampleis.493;thesquareofthisvalueisabout.243.Thisisourmeasureofhowmuchsalaryvariationisexplainedbythelogmodel;itisnottheR-squaredfrom(6.43),whichis.318.Supposeweestimateamodelwithallvariablesinlevels:salary01sales2mktval3ceotenu.TheR-squaredobtainedfromestimatingthismodelusingthesame177observationsis.201.Thus,thelogmodelexplainsmoreofthevariationinsalary,andsowepreferitongoodness-of-fitgrounds.Thelogmodelisalsochosenbecauseitseemsmorerealisticandtheparametersareeasiertointerpret.204\nd7/14/995:33PMPage205Chapter6MultipleRegressionAnalysis:FurtherIssuesSUMMARYInthischapter,wehavecoveredsomeimportantmultipleregressionanalysistopics.Section6.1showedthatachangeintheunitsofmeasurementofanindependentvariablechangestheOLScoefficientintheexpectedmanner:ifxjismultipliedbyc,itscoefficientisdividedbyc.Ifthedependentvariableismultipliedbyc,allOLScoeffi-cientsaremultipliedbyc.NeithertnorFstatisticsareaffectedbychangingtheunitsofmeasurementofanyvariables.Wediscussedbetacoefficients,whichmeasuretheeffectsoftheindependentvari-ablesonthedependentvariableinstandarddeviationunits.ThebetacoefficientsareobtainedfromastandardOLSregressionafterthedependentandindependentvariableshavebeentransformedintoz-scores.Aswehaveseeninseveralexamples,thelogarithmicfunctionalformprovidescoefficientswithpercentageeffectinterpretations.Wediscusseditsadditionaladvan-tagesinSection6.2.Wealsosawhowtocomputetheexactpercentageeffectwhenacoefficientinalog-levelmodelislarge.Modelswithquadraticsallowforeitherdimin-ishingorincreasingmarginaleffects.Modelswithinteractionsallowthemarginaleffectofoneexplanatoryvariabletodependuponthelevelofanotherexplanatoryvariable.2WeintroducedtheadjustedR-squared,R¯,asanalternativetotheusualR-squared2formeasuringgoodness-of-fit.WhileRcanneverfallwhenanothervariableisadded2toaregression,R¯penalizesthenumberofregressorsandcandropwhenanindepen-2dentvariableisadded.ThismakesR¯preferableforchoosingbetweennonnestedmod-22elswithdifferentnumbersofexplanatoryvariables.NeitherRnorR¯canbeusedtocomparemodelswithdifferentdependentvariables.Nevertheless,itisfairlyeasytoobtaingoodness-of-fitmeasuresforchoosingbetweenyandlog(y)asthedependentvariable,asshowninSection6.4.InSection6.3,wediscussedthesomewhatsubtleproblemofrelyingtoomuchon22RorR¯inarrivingatafinalmodel:itispossibletocontrolfortoomanyfactorsinaregressionmodel.Forthisreason,itisimportanttothinkaheadaboutmodelspecifica-tion,particularlytheceterisparibusnatureofthemultipleregressionequation.Explanatoryvariablesthataffectyandareuncorrelatedwithalltheotherexplanatoryvariablescanbeusedtoreducetheerrorvariancewithoutinducingmulticollinearity.InSection6.4,wedemonstratedhowtoobtainaconfidenceintervalforapredic-tionmadefromanOLSregressionline.Wealsoshowedhowaconfidenceintervalcanbeconstructedforafuture,unknownvalueofy.Occasionally,wewanttopredictywhenlog(y)isusedasthedependentvariableinaregressionmodel.Section6.4explainsthissimplemethod.Finally,wearesometimesinterestedinknowingaboutthesignandmagnitudeoftheresidualsforparticularobser-vations.Residualanalysiscanbeusedtodeterminewhetherparticularmembersofthesamplehavepredictedvaluesthatarewellaboveorwellbelowtheactualoutcomes.KEYTERMSAdjustedR-SquaredNonnestedModelsBetaCoefficientsPopulationR-SquaredInteractionEffectPredictions205\nd7/14/995:33PMPage206Part1RegressionAnalysiswithCross-SectionalDataPredictionErrorResidualAnalysisPredictionIntervalStandardizedCoefficientsQuadraticFunctionsVarianceofthePredictionErrorPROBLEMS6.1ThefollowingequationwasestimatedusingthedatainCEOSAL1.RAW:2log(salaryˆ)(4.322)(.276)log(sales)(.0215)roe(.00008)roe2log(salaryˆ)(.324)(.033)log(sales)(.0129)roe(.00026)roe2n209,R.282.Thisequationallowsroetohaveadiminishingeffectonlog(salary).Isthisgeneralitynecessary?Explainwhyorwhynot.6.2Letˆ0,ˆ1,…,ˆkbetheOLSestimatesfromtheregressionofyionxi1,…,xik,i1,2,…,n.Fornonzeroconstantsc1,…,ck,arguethattheOLSinterceptandslopesfromtheregressionofc0yionc1xi1,…,ckxik,i1,2,…,n,aregivenby˜0c0ˆ0,˜1(c0/c1)ˆ1,…,˜k(c0/ck)ˆk.(Hint:Usethefactthattheˆjsolvethefirstorderconditionsin(3.13),andthe˜jmustsolvethefirstorderconditionsinvolvingtherescaleddependentandindependentvariables.)6.3UsingthedatainRDCHEM.RAW,thefollowingequationwasobtainedbyOLS:2rdinˆtens(2.613)(.00030)sales(.0000000070)sales2rdinˆtens(0.429)(.00014)sales(.0000000037)sales2n32,R.1484.(i)Atwhatpointdoesthemarginaleffectofsalesonrdintensbecomeneg-ative?(ii)Wouldyoukeepthequadraticterminthemodel?Explain.(iii)Definesalesbilassalesmeasuredinbillionsofdollars:salesbil2sales/1,000.Rewritetheestimatedequationwithsalesbilandsalesbilastheindependentvariables.Besuretoreportstandarderrorsandthe222R-squared.[Hint:Notethatsalesbilsales/(1,000).](iv)Forthepurposeofreportingtheresults,whichequationdoyouprefer?6.4Thefollowingmodelallowsthereturntoeducationtodependuponthetotalamountofbothparents’education,calledpareduc:log(wage)01educ2educpareduc3exper4tenureu.(i)Showthat,indecimalform,thereturntoanotheryearofeducationinthismodelislog(wage)/educ12pareduc.Whatsigndoyouexpectfor2?Why?(ii)UsingthedatainWAGE2.RAW,theestimatedequationis206\nd7/14/995:33PMPage207Chapter6MultipleRegressionAnalysis:FurtherIssueslog(wˆage)(5.65)(.047)educ(.00078)educpareduclog(wˆage)(0.13)(.010)educ(.00021)educpareduc(.019)exper(.010)tenure(.004)exper(.003)tenure2n722,R.169.(Only722observationscontainfullinformationonparents’education.)Interpretthecoefficientontheinteractionterm.Itmighthelptochoosetwospecificvaluesforpareduc—forexample,pareduc32ifbothparentshaveacollegeeducation,orpareduc24ifbothparentshaveahighschooleducation—andtocomparetheestimatedreturntoeduc.(iii)Whenpareducisaddedasaseparatevariabletotheequation,weget:log(wˆage)(4.94)(.097)educ(.033)pareduc(.0016)educpareduclog(wˆage)(0.38)(.027)educ(.017)pareduc(.0012)educpareduc(.020)exper(.010)tenure(.004)exper(.003)tenure2n722,R.174.Doestheestimatedreturntoeducationnowdependpositivelyonparenteducation?Testthenullhypothesisthatthereturntoeducationdoesnotdependonparenteducation.6.5InExample4.2,wherethepercentageofstudentsreceivingapassingscoreonath10grademathexam(math10)isthedependentvariable,doesitmakesensetoincludethsci11—thepercentageof11graderspassingascienceexam—asanadditionalexplanatoryvariable?26.6WhenatndrteandACTatndrteareaddedtotheequationestimatedin(6.19),theR-squaredbecomes.232.Aretheseadditionaltermsjointlysignificantatthe10%level?Wouldyouincludetheminthemodel?6.7Thefollowingthreeequationswereestimatedusingthe1,534observationsin401K.RAW:praˆte(80.29)(5.44)mrate(.269)age(.00013)totemppraˆte(0.78)(0.52)mrate(.045)age(.00004)totemp22R.100,R¯.098.praˆte(97.32)(5.02)mrate(.314)age(2.66)log(totemp)praˆte(1.95)(0.51)mrate(.044)age(0.28)log(totemp)22R.144,R¯.142.praˆte(80.62)(5.34)mrate(.290)age(.00043)totemppraˆte(0.78)(0.52)mrate(.045)age(.00009)totemp2).0000000039)totemp2(.0000000010)totemp22R.108,R¯.106.Whichofthesethreemodelsdoyouprefer.Why?207\nd7/14/995:33PMPage208Part1RegressionAnalysiswithCross-SectionalDataCOMPUTEREXERCISES6.8UsethedatainHPRICE3.RAW,onlyfortheyear1981,toanswerthefollowingquestions.Thedataareforhousesthatsoldduring1981inNorthAndover,MA;1981wastheyearconstructionbeganonalocalgarbageincinerator.(i)Tostudytheeffectsoftheincineratorlocationonhousingprice,con-siderthesimpleregressionmodellog(price)01log(dist)u,wherepriceishousingpriceindollarsanddistisdistancefromthehousetotheincineratormeasuredinfeet.Interpretingthisequationcausally,whatsigndoyouexpectfor1ifthepresenceoftheincinera-tordepresseshousingprices?Estimatethisequationandinterprettheresults.(ii)Tothesimpleregressionmodelinpart(i),addthevariableslog(inst),log(area),log(land),rooms,baths,andage,whereinstisdistancefromthehometotheinterstate,areaissquarefootageofthehouse,landisthelotsizeinsquarefeet,roomsistotalnumberofrooms,bathsisnum-berofbathrooms,andageisageofthehouseinyears.Nowwhatdoyouconcludeabouttheeffectsoftheincinerator?Explainwhy(i)and(ii)giveconflictingresults.2(iii)Add[log(inst)]tothemodelfrompart(ii).Nowwhathappens?Whatdoyouconcludeabouttheimportanceoffunctionalform?(iv)Isthesquareoflog(dist)significantwhenyouaddittothemodelfrompart(iii)?6.9UsethedatainWAGE1.RAWforthisexercise.(i)UseOLStoestimatetheequation2log(wage)01educ2exper3experuandreporttheresultsusingtheusualformat.2(ii)Isexperstatisticallysignificantatthe1%level?(iii)Usingtheapproximation%waˆge100(ˆ22ˆ3exper)exper,findtheapproximatereturntothefifthyearofexperience.Whatistheapproximatereturntothetwentiethyearofexperience?(iv)Atwhatvalueofexperdoesadditionalexperienceactuallylowerpre-dictedlog(wage)?Howmanypeoplehavemoreexperienceinthissam-ple?6.10Consideramodelwherethereturntoeducationdependsupontheamountofworkexperience(andviceversa):log(wage)01educ2exper3educexperu.(i)Showthatthereturntoanotheryearofeducation(indecimalform),holdingexperfixed,is13exper.208\nd7/14/995:33PMPage209Chapter6MultipleRegressionAnalysis:FurtherIssues(ii)Statethenullhypothesisthatthereturntoeducationdoesnotdependonthelevelofexper.Whatdoyouthinkistheappropriatealternative?(iii)UsethedatainWAGE2.RAWtotestthenullhypothesisin(ii)againstyourstatedalternative.(iv)Let1denotethereturntoeducation(indecimalform),whenexper10:11103.Obtainˆ1anda95%confidenceintervalfor1.(Hint:Write11103andplugthisintotheequation;thenre-arrange.Thisgivestheregressionforobtainingtheconfidenceintervalfor1.)6.11UsethedatainGPA2.RAWforthisexercise.(i)Estimatethemodel2sat01hsize2hsizeu,wherehsizeissizeofgraduatingclass(inhundreds),andwritetheresultsintheusualform.Isthequadratictermstatisticallysignificant?(ii)Usingtheestimatedequationfrompart(i),whatisthe“optimal”highschoolsize?Justifyyouranswer.(iii)Isthisanalysisrepresentativeoftheacademicperformanceofallhighschoolseniors?Explain.(iv)Findtheestimatedoptimalhighschoolsize,usinglog(sat)asthedependentvariable.Isitmuchdifferentfromwhatyouobtainedinpart(ii)?6.12UsethehousingpricedatainHPRICE1.RAWforthisexercise.(i)Estimatethemodellog(price)01log(lotsize)2log(sqrft)3bdrmsuandreporttheresultsintheusualOLSformat.(ii)Findthepredictedvalueoflog(price),whenlotsize20,000,sqrft2,500,andbdrms4.UsingthemethodsinSection6.4,findthepre-dictedvalueofpriceatthesamevaluesoftheexplanatoryvariables.(iii)Forexplainingvariationinprice,decidewhetheryoupreferthemodelfrompart(i)orthemodelprice01lotsize2sqrft3bdrmsu.6.13UsethedatainVOTE1.RAWforthisexercise.(i)Consideramodelwithaninteractionbetweenexpenditures:voteA01prtystrA2expendA3expendB4expendAexpendBu.WhatisthepartialeffectofexpendBonvoteA,holdingprtystrAandexpendAfixed?WhatisthepartialeffectofexpendAonvoteA?Istheexpectedsignfor4obvious?(ii)Estimatetheequationinpart(i)andreporttheresultsintheusualform.Istheinteractiontermstatisticallysignificant?(iii)FindtheaverageofexpendAinthesample.FixexpendAat300(for$300,000).Whatistheestimatedeffectofanother$100,000dollarsspentbyCandidateBonvoteA?Isthisalargeeffect?209\nd7/14/995:33PMPage210Part1RegressionAnalysiswithCross-SectionalData(iv)NowfixexpendBat100.WhatistheestimatedeffectofexpendA100onvoteA.Doesthismakesense?(v)NowestimateamodelthatreplacestheinteractionwithshareA,CandidateA’spercentageshareoftotalcampaignexpenditures.DoesitmakesensetoholdbothexpendAandexpendBfixed,whilechangingshareA?(vi)(Requirescalculus)Inthemodelfrompart(v),findthepartialeffectofexpendBonvoteA,holdingprtystrAandexpendAfixed.EvaluatethisatexpendA300andexpendB0andcommentontheresults.6.14UsethedatainATTEND.RAWforthisexercise.(i)InthemodelofExample6.3,arguethatstndfnl/priGPA224priGPA6atndrte.Useequation(6.19)toestimatethepartialeffect,whenpriGPA2.59andatndrte.82.Interpretyourestimate.(ii)Showthattheequationcanbewrittenas2stndfnl01atndrte2priGPA3ACT4(priGPA2.59)25ACT6priGPA(atndrte.82)u,where2224(2.59)6(.82).(Notethattheintercepthaschanged,butthisisunimportant.)Usethistoobtainthestandarderrorofˆ2frompart(i).6.15UsethedatainHPRICE1.RAWforthisexercise.(i)Estimatethemodelprice01lotsize2sqrft3bdrmsuandreporttheresultsintheusualform,includingthestandarderroroftheregression.Obtainpredictedprice,whenwepluginlotsize10,000,sqrft2,300,andbdrms4;roundthispricetothenearestdollar.(ii)Runaregressionthatallowsyoutoputa95%confidenceintervalaroundthepredictedvalueinpart(i).Notethatyourpredictionwilldif-fersomewhatduetoroundingerror.0(iii)Letpricebetheunknownfuturesellingpriceofthehousewiththe0characteristicsusedinparts(i)and(ii).Finda95%CIforpriceandcommentonthewidthofthisconfidenceinterval.210\n\nd7/14/995:55PMPage211ChapterSevenMultipleRegressionAnalysiswithQualitativeInformation:Binary(orDummy)Variablesnpreviouschapters,thedependentandindependentvariablesinourmultipleregres-Isionmodelshavehadquantitativemeaning.Justafewexamplesincludehourlywagerate,yearsofeducation,collegegradepointaverage,amountofairpollution,leveloffirmsales,andnumberofarrests.Ineachcase,themagnitudeofthevariableconveysusefulinformation.Inempiricalwork,wemustalsoincorporatequalitativefactorsintoregressionmodels.Thegenderorraceofanindividual,theindustryofafirm(manufacturing,retail,etc.),andtheregionintheUnitedStateswhereacityislocated(south,north,west,etc.)areallconsideredtobequalitativefactors.Mostofthischapterisdedicatedtoqualitativeindependentvariables.Afterwedis-cusstheappropriatewaystodescribequalitativeinformationinSection7.1,weshowhowqualitativeexplanatoryvariablescanbeeasilyincorporatedintomultipleregres-sionmodelsinSections7.2,7.3,and7.4.Thesesectionscoveralmostallofthepopu-larwaysthatqualitativeindependentvariablesareusedincross-sectionalregressionanalysis.InSection7.5,wediscussabinarydependentvariable,whichisaparticularkindofqualitativedependentvariable.Themultipleregressionmodelhasaninterestinginter-pretationinthiscaseandiscalledthelinearprobabilitymodel.Whilemuchmalignedbysomeeconometricians,thesimplicityofthelinearprobabilitymodelmakesituse-fulinmanyempiricalcontexts.WewilldescribeitsdrawbacksinSection7.5,buttheyareoftensecondaryinempiricalwork.7.1DESCRIBINGQUALITATIVEINFORMATIONQualitativefactorsoftencomeintheformofbinaryinformation:apersonisfemaleormale;apersondoesordoesnotownapersonalcomputer;afirmoffersacertainkindofemployeepensionplanoritdoesnot;astateadministerscapitalpunishmentoritdoesnot.Inalloftheseexamples,therelevantinformationcanbecapturedbydefin-ingabinaryvariableorazero-onevariable.Ineconometrics,binaryvariablesaremostcommonlycalleddummyvariables,althoughthisnameisnotespeciallydescriptive.Indefiningadummyvariable,wemustdecidewhicheventisassignedthevalueoneandwhichisassignedthevaluezero.Forexample,inastudyofindividualwagedeter-211\nd7/14/995:55PMPage212Part1RegressionAnalysiswithCross-SectionalDatamination,wemightdefinefemaletobeabinaryvariabletakingonthevalueoneforfemalesandthevaluezeroformales.Thenameinthiscaseindicatestheeventwiththevalueone.Thesameinformationiscap-turedbydefiningmaletobeoneiftheper-QUESTION7.1sonismaleandzeroifthepersonisSupposethat,inastudycomparingelectionoutcomesbetweenfemale.EitheroftheseisbetterthanusingDemocraticandRepublicancandidates,youwishtoindicatethegenderbecausethisnamedoesnotmakeitpartyofeachcandidate.Isanamesuchaspartyawisechoiceforabinaryvariableinthiscase?Whatwouldbeabettername?clearwhenthedummyvariableisone:doesgender1correspondtomaleorfemale?Whatwecallourvariablesisunimportantforgettingregressionresults,butitalwayshelpstochoosenamesthatclarifyequationsandexpositions.Supposeinthewageexamplethatwehavechosenthenamefemaletoindicategen-der.Further,wedefineabinaryvariablemarriedtoequaloneifapersonismarriedandzeroifotherwise.Table7.1givesapartiallistingofawagedatasetthatmightresult.WeseethatPerson1isfemaleandnotmarried,Person2isfemaleandmarried,Person3ismaleandnotmarried,andsoon.Whydoweusethevalueszeroandonetodescribequalitativeinformation?Inasense,thesevaluesarearbitrary:anytwodifferentvalueswoulddo.Therealbenefitofcapturingqualitativeinformationusingzero-onevariablesisthatitleadstoregressionmodelswheretheparametershaveverynaturalinterpretations,aswewillseenow.Table7.1APartialListingoftheDatainWAGE1.RAWpersonwageeducexperfemalemarried13.101121023.2412221133.001120046.008440155.301270152511.56165015263.5014510212\nd7/14/995:55PMPage213Chapter7MultipleRegressionAnalysisWithQualitativeInformation:Binary(orDummy)Variables7.2ASINGLEDUMMYINDEPENDENTVARIABLEHowdoweincorporatebinaryinformationintoregressionmodels?Inthesimplestcase,withonlyasingledummyexplanatoryvariable,wejustadditasanindependentvariableintheequation.Forexample,considerthefollowingsimplemodelofhourlywagedetermination:wage00female1educu.(7.1)Weuse0astheparameteronfemaleinordertohighlighttheinterpretationofthepa-rametersmultiplyingdummyvariables;later,wewillusewhatevernotationismostconvenient.Inmodel(7.1),onlytwoobservedfactorsaffectwage:genderandeducation.Sincefemale1whenthepersonisfemale,andfemale0whenthepersonismale,theparameter0hasthefollowinginterpretation:0isthedifferenceinhourlywagebetweenfemalesandmales,giventhesameamountofeducation(andthesameerrortermu).Thus,thecoefficient0determineswhetherthereisdiscriminationagainstwomen:if00,then,forthesamelevelofotherfactors,womenearnlessthanmenonaverage.Intermsofexpectations,ifweassumethezeroconditionalmeanassumptionE(ufemale,educ)0,then0E(wagefemale1,educ)E(wagefemale0,educ).Sincefemale1correspondstofemalesandfemale0correspondstomales,wecanwritethismoresimplyas0E(wagefemale,educ)E(wagemale,educ).(7.2)Thekeyhereisthatthelevelofeducationisthesameinbothexpectations;thediffer-ence,0,isduetogenderonly.Thesituationcanbedepictedgraphicallyasaninterceptshiftbetweenmalesandfemales.InFigure7.1,thecase00isshown,sothatmenearnafixedamountmoreperhourthanwomen.Thedifferencedoesnotdependontheamountofeducation,andthisexplainswhythewage-educationprofilesforwomenandmenareparallel.Atthispoint,youmaywonderwhywedonotalsoincludein(7.1)adummyvari-able,saymale,whichisoneformalesandzeroforfemales.Thereasonisthatthiswouldberedundant.In(7.1),theinterceptformalesis0,andtheinterceptforfemalesis00.Sincetherearejusttwogroups,weonlyneedtwodifferentintercepts.Thismeansthat,inadditionto0,weneedtouseonlyonedummyvariable;wehavecho-sentoincludethedummyvariableforfemales.Usingtwodummyvariableswouldintroduceperfectcollinearitybecausefemalemale1,whichmeansthatmaleisaperfectlinearfunctionoffemale.Includingdummyvariablesforbothgendersisthesimplestexampleoftheso-calleddummyvariabletrap,whichariseswhentoomanydummyvariablesdescribeagivennumberofgroups.Wewilldiscussthisproblemlater.In(7.1),wehavechosenmalestobethebasegrouporbenchmarkgroup,thatis,thegroupagainstwhichcomparisonsaremade.Thisiswhy0istheinterceptfor213\nd7/14/995:55PMPage214Part1RegressionAnalysiswithCross-SectionalDataFigure7.1Graphofwage=00female1educfor00.wagemen:wage=educ01women:wage=()+educ001slope=10000educmales,and0isthedifferenceininterceptsbetweenfemalesandmales.Wecouldchoosefemalesasthebasegroupbywritingthemodelaswage00male1educu,wheretheinterceptforfemalesis0andtheinterceptformalesis00;thisimpliesthat000and000.Inanyapplication,itdoesnotmatterhowwechoosethebasegroup,butitisimportanttokeeptrackofwhichgroupisthebasegroup.Someresearchersprefertodroptheoverallinterceptinthemodelandtoincludedummyvariablesforeachgroup.Theequationwouldthenbewage0male0female1educu,wheretheinterceptformenis0andtheinterceptforwomenis0.Thereisnodummyvariabletrapinthiscasebecausewedonothaveanoverallintercept.However,thisformulationhaslittletooffer,sincetestingforadifferenceintheinterceptsismoredifficult,andthereisnogenerallyagreeduponwaytocomputeR-squaredinregressionswithoutanintercept.Therefore,wewillalwaysincludeanoverallinterceptforthebasegroup.214\nd7/14/995:55PMPage215Chapter7MultipleRegressionAnalysisWithQualitativeInformation:Binary(orDummy)VariablesNothingmuchchangeswhenmoreexplanatoryvariablesareinvolved.Takingmalesasthebasegroup,amodelthatcontrolsforexperienceandtenureinadditiontoeducationiswage00female1educ2exper3tenureu.(7.3)Ifeduc,exper,andtenureareallrelevantproductivitycharacteristics,thenullhypoth-esisofnodifferencebetweenmenandwomenisH0:00.ThealternativethatthereisdiscriminationagainstwomenisH1:00.Howcanweactuallytestforwagediscrimination?Theanswerissimple:justesti-matethemodelbyOLS,exactlyasbefore,andusetheusualtstatistic.NothingchangesaboutthemechanicsofOLSorthestatisticaltheorywhensomeoftheindependentvariablesaredefinedasdummyvariables.Theonlydifferencewithwhatwehavedoneupuntilnowisintheinterpretationofthecoefficientonthedummyvariable.EXAMPLE7.1(HourlyWageEquation)UsingthedatainWAGE1.RAW,weestimatemodel(7.3).Fornow,weusewage,ratherthanlog(wage),asthedependentvariable:(waˆge1.57)(1.81)female(.572)educwaˆge(0.72)(0.26)female(.049)educ(.025)exper(.141)tenure(7.4)(.012)exper(.021)tenure2n526,R.364.Thenegativeintercept—theinterceptformen,inthiscase—isnotverymeaningful,sincenoonehasclosetozeroyearsofeduc,exper,andtenureinthesample.Thecoefficientonfemaleisinteresting,becauseitmeasurestheaveragedifferenceinhourlywagebetweenawomanandaman,giventhesamelevelsofeduc,exper,andtenure.Ifwetakeawomanandamanwiththesamelevelsofeducation,experience,andtenure,thewomanearns,onaverage,$1.81lessperhourthantheman.(Recallthattheseare1976wages.)Itisimportanttorememberthat,becausewehaveperformedmultipleregressionandcontrolledforeduc,exper,andtenure,the$1.81wagedifferentialcannotbeexplainedbydifferentaveragelevelsofeducation,experience,ortenurebetweenmenandwomen.Wecanconcludethatthedifferentialof$1.81isduetogenderorfactorsassociatedwithgen-derthatwehavenotcontrolledforintheregression.Itisinformativetocomparethecoefficientonfemaleinequation(7.4)totheestimatewegetwhenallotherexplanatoryvariablesaredroppedfromtheequation:waˆge(7.10)(2.51)femalewaˆge(0.21)(0.30)female(7.5)2n526,R.116.215\nd7/14/995:55PMPage216Part1RegressionAnalysiswithCross-SectionalDataThecoefficientsin(7.5)haveasimpleinterpretation.Theinterceptistheaveragewageformeninthesample(letfemale0),somenearn$7.10perhouronaverage.Thecoeffi-cientonfemaleisthedifferenceintheaveragewagebetweenwomenandmen.Thus,theaveragewageforwomeninthesampleis7.102.514.59,or$4.59perhour.(Incidentally,thereare274menand252womeninthesample.)Equation(7.5)providesasimplewaytocarryoutacomparison-of-meanstestbetweenthetwogroups,whichinthiscasearemenandwomen.Theestimateddifference,2.51,hasatstatisticof8.37,whichisverystatisticallysignificant(and,ofcourse,$2.51iseco-nomicallylargeaswell).Generally,simpleregressiononaconstantandadummyvariableisastraightforwardwaytocomparethemeansoftwogroups.Fortheusualttesttobevalid,wemustassumethatthehomoskedasticityassumptionholds,whichmeansthatthepopulationvarianceinwagesformenisthesameasthatforwomen.Theestimatedwagedifferentialbetweenmenandwomenislargerin(7.5)thanin(7.4)because(7.5)doesnotcontrolfordifferencesineducation,experience,andtenure,andthesearelower,onaverage,forwomenthanformeninthissample.Equation(7.4)givesamorereliableestimateoftheceterisparibusgenderwagegap;itstillindicatesaverylargedifferential.Inmanycases,dummyindependentvariablesreflectchoicesofindividualsorothereconomicunits(asopposedtosomethingpredetermined,suchasgender).Insuchsitu-ations,thematterofcausalityisagainacentralissue.Inthefollowingexample,wewouldliketoknowwhetherpersonalcomputerownershipcausesahighercollegegradepointaverage.EXAMPLE7.2(EffectsofComputerOwnershiponCollegeGPA)Inordertodeterminetheeffectsofcomputerownershiponcollegegradepointaverage,weestimatethemodelcolGPA00PC1hsGPA2ACTu,wherethedummyvariablePCequalsoneifastudentownsapersonalcomputerandzerootherwise.TherearevariousreasonsPCownershipmighthaveaneffectoncolGPA.Astu-dent’sworkmightbeofhigherqualityifitisdoneonacomputer,andtimecanbesavedbynothavingtowaitatacomputerlab.Ofcourse,astudentmightbemoreinclinedtoplaycomputergamesorsurftheInternetifheorsheownsaPC,soitisnotobviousthat0ispos-itive.ThevariableshsGPA(highschoolGPA)andACT(achievementtestscore)areusedascon-trols:itcouldbethatstrongerstudents,asmeasuredbyhighschoolGPAandACTscores,aremorelikelytoowncomputers.WecontrolforthesefactorsbecausewewouldliketoknowtheaverageeffectoncolGPAifastudentispickedatrandomandgivenapersonalcomputer.UsingthedatainGPA1.RAW,weobtainˆcolGPA(1.26)(.157)PC(.447)hsGPA(.0087)ACTˆcolGPA(0.33)(.057)PC(.094)hsGPA(.0105)ACT(7.6)2n141,R.219.216\nd7/14/995:55PMPage217Chapter7MultipleRegressionAnalysisWithQualitativeInformation:Binary(orDummy)VariablesThisequationimpliesthatastudentwhoownsaPChasapredictedGPAabout.16pointhigherthanacomparablestudentwithoutaPC(remember,bothcolGPAandhsGPAareonafour-pointscale).Theeffectisalsoverystatisticallysignificant,withtPC.157/.0572.75.WhathappensifwedrophsGPAandACTfromtheequation?Clearly,droppingthelat-tervariableshouldhaveverylittleeffect,asitscoefficientandtstatisticareverysmall.ButhsGPAisverysignificant,andsodroppingitcouldaffecttheestimateofPC.RegressingcolGPAonPCgivesanestimateonPCequaltoabout.170,withastandarderrorof.063;inthiscase,ˆPCanditststatisticdonotchangebymuch.Intheexercisesattheendofthechapter,youwillbeaskedtocontrolforotherfactorsintheequationtoseeifthecomputerownershipeffectdisappears,orifitatleastgetsnotablysmaller.Eachofthepreviousexamplescanbeviewedashavingrelevanceforpolicyanaly-sis.Inthefirstexample,wewereinterestedingenderdiscriminationintheworkforce.Inthesecondexample,wewereconcernedwiththeeffectofcomputerownershiponcollegeperformance.Aspecialcaseofpolicyanalysisisprogramevaluation,wherewewouldliketoknowtheeffectofeconomicorsocialprogramsonindividuals,firms,neighborhoods,cities,andsoon.Inthesimplestcase,therearetwogroupsofsubjects.Thecontrolgroupdoesnotparticipateintheprogram.Theexperimentalgrouportreatmentgroupdoestakepartintheprogram.Thesenamescomefromliteratureintheexperimentalsciences,andtheyshouldnotbetakenliterally.Exceptinrarecases,thechoiceofthecontrolandtreatmentgroupsisnotrandom.However,insomecases,multipleregressionanalysiscanbeusedtocontrolforenoughotherfactorsinordertoestimatethecausaleffectoftheprogram.EXAMPLE7.3(EffectsofTrainingGrantsonHoursofTraining)Usingthe1988dataforMichiganmanufacturingfirmsinJTRAIN.RAW,weobtainthefol-lowingestimatedequation:hrseˆmp(46.67)(26.25)grant(.98)log(sales)hrseˆmp(43.41)(5.59)grant(3.54)log(sales)(6.07)log(employ)(7.7)(3.88)log(employ)2n105,R.237.Thedependentvariableishoursoftrainingperemployee,atthefirmlevel.Thevariablegrantisadummyvariableequaltooneifthefirmreceivedajobtraininggrantfor1988andzerootherwise.Thevariablessalesandemployrepresentannualsalesandnumberofemployees,respectively.Wecannotenterhrsempinlogarithmicform,becausehrsempiszerofor29ofthe105firmsusedintheregression.217\nd7/14/995:55PMPage218Part1RegressionAnalysiswithCross-SectionalDataThevariablegrantisverystatisticallysignificant,withtgrant4.70.Controllingforsalesandemployment,firmsthatreceivedagranttrainedeachworker,onaverage,26.25hoursmore.Sincetheaveragenumberofhoursofperworkertraininginthesampleisabout17,withamaximumvalueof164,granthasalargeeffectontraining,asisexpected.Thecoefficientonlog(sales)issmallandveryinsignificant.Thecoefficientonlog(employ)meansthat,ifafirmis10%larger,ittrainsitsworkersabout.61hourless.Itststatisticis1.56,whichisonlymarginallystatisticallysignificant.Aswithanyotherindependentvariable,weshouldaskwhetherthemeasuredeffectofaqualitativevariableiscausal.Inequation(7.7),isthedifferenceintrainingbetweenfirmsthatreceivegrantsandthosethatdonotduetothegrant,orisgrantreceiptsim-plyanindicatorofsomethingelse?Itmightbethatthefirmsreceivinggrantswouldhave,onaverage,trainedtheirworkersmoreevenintheabsenceofagrant.Nothinginthisanalysistellsuswhetherwehaveestimatedacausaleffect;wemustknowhowthefirmsreceivinggrantsweredetermined.Wecanonlyhopewehavecontrolledforasmanyfactorsaspossiblethatmightberelatedtowhetherafirmreceivedagrantandtoitslevelsoftraining.WewillreturntopolicyanalysiswithdummyvariablesinSection7.6,aswellasinlaterchapters.InterpretingCoefficientsonDummyExplanatoryVariablesWhentheDependentVariableIslog(y)Acommonspecificationinappliedworkhasthedependentvariableappearinginloga-rithmicform,withoneormoredummyvariablesappearingasindependentvariables.Howdoweinterpretthedummyvariablecoefficientsinthiscase?Notsurprisingly,thecoefficientshaveapercentageinterpretation.EXAMPLE7.4(HousingPriceRegression)UsingthedatainHPRICE1.RAW,weobtaintheequationlog(priˆce)(5.56)(.168)log(lotsize)(.707)log(sqrft)log(priˆce)(0.65)(.038)log(lotsize)(.093)log(sqrft)(.027)bdrms(.054)colonial(7.8)(.029)bdrms(.045)colonial2n88,R.649.Allthevariablesareself-explanatoryexceptcolonial,whichisabinaryvariableequaltooneifthehouseisofthecolonialstyle.Whatdoesthecoefficientoncolonialmean?Forgivenlevelsoflotsize,sqrft,andbdrms,thedifferenceinlog(ˆprice)betweenahouseofcolonialstyleandthatofanotherstyleis.054.Thismeansthatacolonialstylehouseispredictedtosellforabout5.4%more,holdingotherfactorsfixed.218\nd7/14/995:55PMPage219Chapter7MultipleRegressionAnalysisWithQualitativeInformation:Binary(orDummy)VariablesThisexampleshowsthat,whenlog(y)isthedependentvariableinamodel,thecoefficientonadummyvariable,whenmultipliedby100,isinterpretedasthepercent-agedifferenceiny,holdingallotherfactorsfixed.Whenthecoefficientonadummyvariablesuggestsalargeproportionatechangeiny,theexactpercentagedifferencecanbeobtainedexactlyaswiththesemi-elasticitycalculationinSection6.2.EXAMPLE7.5(LogHourlyWageEquation)LetusreestimatethewageequationfromExample7.1,usinglog(wage)asthedependentvariableandaddingquadraticsinexperandtenure:log(wˆage)(.417)(.297)female(.080)educ(.029)experlog(wˆage)(.099)(.036)female(.007)educ(.005)exper22(.00058)exper(.032)tenure(.00059)tenure22(7.9)(.00010)exper(.007)tenure(.00023)tenure2n526,R.441.UsingthesameapproximationasinExample7.4,thecoefficientonfemaleimpliesthat,forthesamelevelsofeduc,exper,andtenure,womenearnabout100(.297)29.7%lessthanmen.Wecandobetterthanthisbycomputingtheexactpercentagedifferenceinpredictedwages.Whatwewantistheproportionatedifferenceinwagesbetweenfemalesandmales,holdingotherfactorsfixed:(waˆgeFwaˆgeM)/waˆgeM.Whatwehavefrom(7.9)islog(ˆwageF)log(ˆwageM).297.Exponentiatingandsubtractingonegives(waˆgeFwaˆgeM)/waˆgeMexp(.297)1.257.Thismoreaccurateestimateimpliesthatawoman’swageis,onaverage,25.7%belowacomparableman’swage.IfwehadmadethesamecorrectioninExample7.4,wewouldhaveobtainedexp(.054)1.0555,orabout5.6%.ThecorrectionhasasmallereffectinExample7.4thaninthewageexample,becausethemagnitudeofthecoefficientonthedummyvariableismuchsmallerin(7.8)thanin(7.9).Generally,ifˆ1isthecoefficientonadummyvariable,sayx1,whenlog(y)isthedependentvariable,theexactpercentagedifferenceinthepredictedywhenx11ver-suswhenx10is100[exp(ˆ1)1].(7.10)Theestimateˆ1canbepositiveornegative,anditisimportanttopreserveitssignincomputing(7.10).219\nd7/14/995:55PMPage220Part1RegressionAnalysiswithCross-SectionalData7.3USINGDUMMYVARIABLESFORMULTIPLECATEGORIESWecanuseseveraldummyindependentvariablesinthesameequation.Forexample,wecouldaddthedummyvariablemarriedtoequation(7.9).Thecoefficientonmar-riedgivesthe(approximate)proportionaldifferentialinwagesbetweenthosewhoareandarenotmarried,holdinggender,educ,exper,andtenurefixed.Whenweestimatethismodel,thecoefficientonmarried(withstandarderrorinparentheses)is.053(.041),andthecoefficientonfemalebecomes.290(.036).Thus,the“marriagepre-mium”isestimatedtobeabout5.3%,butitisnotstatisticallydifferentfromzero(t1.29).Animportantlimitationofthismodelisthatthemarriagepremiumisassumedtobethesameformenandwomen;thisisrelaxedinthefollowingexample.EXAMPLE7.6(LogHourlyWageEquation)Letusestimateamodelthatallowsforwagedifferencesamongfourgroups:marriedmen,marriedwomen,singlemen,andsinglewomen.Todothis,wemustselectabasegroup;wechoosesinglemen.Then,wemustdefinedummyvariablesforeachoftheremaininggroups.Callthesemarrmale,marrfem,andsingfem.Puttingthesethreevariablesinto(7.9)(and,ofcourse,droppingfemale,sinceitisnowredundant)giveslog(wˆage)(.321)(.213)marrmale(.198)marrfemlog(wˆage)(.100)(.055)marrmale(.058)marrfem2(.110)singfem(.079)educ(.027)exper(.00054)exper2(.056)singfem(.007)educ(.005)exper(.00011)exper(7.11)2(.029)tenure(.00053)tenure2(.007)tenure(.00023)tenure2n526,R.461.Allofthecoefficients,withtheexceptionofsingfem,havetstatisticswellabovetwoinabsolutevalue.Thetstatisticforsingfemisabout1.96,whichisjustsignificantatthe5%levelagainstatwo-sidedalternative.Tointerpretthecoefficientsonthedummyvariables,wemustrememberthatthebasegroupissinglemales.Thus,theestimatesonthethreedummyvariablesmeasurethepro-portionatedifferenceinwagerelativetosinglemales.Forexample,marriedmenareesti-matedtoearnabout21.3%morethansinglemen,holdinglevelsofeducation,experience,andtenurefixed.[Themorepreciseestimatefrom(7.10)isabout23.7%.]Amarriedwoman,ontheotherhand,earnsapredicted19.8%lessthanasinglemanwiththesamelevelsoftheothervariables.Sincethebasegroupisrepresentedbytheinterceptin(7.11),wehaveincludeddummyvariablesforonlythreeofthefourgroups.Ifweweretoaddadummyvariableforsinglemalesto(7.11),wewouldfallintothedummyvariabletrapbyintroducingperfectcollinearity.Someregressionpackageswillautomaticallycorrectthismistakeforyou,while220\nd7/14/995:55PMPage221Chapter7MultipleRegressionAnalysisWithQualitativeInformation:Binary(orDummy)Variablesotherswilljusttellyouthereisperfectcollinearity.Itisbesttocarefullyspecifythedummyvariables,becauseitforcesustoproperlyinterpretthefinalmodel.Eventhoughsinglemenisthebasegroupin(7.11),wecanusethisequationtoobtaintheestimateddifferencebetweenanytwogroups.Sincetheoverallinterceptiscommontoallgroups,wecanignorethatinfindingdifferences.Thus,theestimatedproportionatedif-ferencebetweensingleandmarriedwomenis.110(.198).088,whichmeansthatsinglewomenearnabout8.8%morethanmarriedwomen.Unfortunately,wecannotuseequation(7.11)fortestingwhethertheestimateddifferencebetweensingleandmarriedwomenisstatisticallysignificant.Knowingthestandarderrorsonmarrfemandsingfemisnotenoughtocarryoutthetest(seeSection4.4).Theeasiestthingtodoistochooseoneofthesegroupstobethebasegroupandtoreestimatetheequation.Nothingsubstantivechanges,butwegettheneededestimateanditsstandarderrordirectly.Whenweusemar-riedwomenasthebasegroup,weobtainlog(wageˆ)(.123)(.411)marrmale(.198)singmale(.088)singfem…,log(wageˆ)(.106)(.056)marrmale(.058)singmale(.052)singfem…,where,ofcourse,noneoftheunreportedcoefficientsorstandarderrorshavechanged.Theestimateonsingfemis,asexpected,.088.Now,wehaveastandarderrortogoalongwiththisestimate.Thetstatisticforthenullthatthereisnodifferenceinthepopulationbetweenmarriedandsinglewomenistsingfem.088/.0521.69.Thisismarginalevi-denceagainstthenullhypothesis.Wealsoseethattheestimateddifferencebetweenmar-riedmenandmarriedwomenisverystatisticallysignificant(tmarrmale7.34).Thepreviousexampleillustratesageneralprincipleforincludingdummyvariablestoindicatedifferentgroups:iftheregressionmodelistohavedifferentinterceptsfor,sayggroupsorcategories,weneedtoincludeg1dummyvariablesinthemodelalongwithanintercept.Theinterceptforthebasegroupistheoverallinterceptinthemodel,andthedummyvariablecoefficientforaparticulargrouprepresentstheesti-QUESTION7.2mateddifferenceininterceptsbetweenthatInthebaseballsalarydatafoundinMLB1.RAW,playersaregivengroupandthebasegroup.Includinggoneofsixpositions:frstbase,scndbase,thrdbase,shrtstop,outfield,dummyvariablesalongwithaninterceptorcatcher.Toallowforsalarydifferentialsacrossposition,without-willresultinthedummyvariabletrap.Anfieldersasthebasegroup,whichdummyvariableswouldyouincludeasindependentvariables?alternativeistoincludegdummyvariablesandtoexcludeanoverallintercept.Thisisnotadvisablebecausetestingfordifferencesrelativetoabasegroupbecomesdifficult,andsomeregressionpackagesalterthewaytheR-squarediscomputedwhentheregres-siondoesnotcontainanintercept.IncorporatingOrdinalInformationbyUsingDummyVariablesSupposethatwewouldliketoestimatetheeffectofcitycreditratingsonthemunici-palbondinterestrate(MBR).Severalfinancialcompanies,suchasMoody’sInvestmentServiceandStandardandPoor’s,ratethequalityofdebtforlocalgovernments,where221\nd7/14/995:55PMPage222Part1RegressionAnalysiswithCross-SectionalDatatheratingsdependonthingslikeprobabilityofdefault.(Localgovernmentspreferlowerinterestratesinordertoreducetheircostsofborrowing.)Forsimplicity,supposethatrankingsrangefromzerotofour,withzerobeingtheworstcreditratingandfourbeingthebest.Thisisanexampleofanordinalvariable.CallthisvariableCRforcon-creteness.Thequestionweneedtoaddressis:HowdoweincorporatethevariableCRintoamodeltoexplainMBR?OnepossibilityistojustincludeCRaswewouldincludeanyotherexplanatoryvariable:MBR01CRotherfactors,wherewedonotexplicitlyshowwhatotherfactorsareinthemodel.Then1istheper-centagepointchangeinMBRwhenCRincreasesbyoneunit,holdingotherfactorsfixed.Unfortunately,itisratherhardtointerpretaone-unitincreaseinCR.Weknowthequantitativemeaningofanotheryearofeducation,oranotherdollarspentperstu-dent,butthingslikecreditratingstypicallyhaveonlyordinalmeaning.WeknowthataCRoffourisbetterthanaCRofthree,butisthedifferencebetweenfourandthreethesameasthedifferencebetweenoneandzero?Ifnot,thenitmightnotmakesensetoassumethataone-unitincreaseinCRhasaconstanteffectonMBR.Abetterapproach,whichwecanimplementbecauseCRtakesonrelativelyfewval-ues,istodefinedummyvariablesforeachvalueofCR.Thus,letCR11ifCR1,andCR10otherwise;CR21ifCR2,andCR20otherwise.Andsoon.Effectively,wetakethesinglecreditratingandturnitintofivecategories.Then,wecanestimatethemodelMBR01CR12CR23CR34CR4otherfactors.(7.12)Followingourruleforincludingdummyvariablesinamodel,weincludefourdummyvariablessincewehavefivecategories.Theomittedcategoryhereisacreditratingofzero,andsoitisthebasegroup.(Thisiswhywedonotneedtodefineadummyvari-ableforthiscategory.)Thecoefficientsareeasytointerpret:1isthedifferenceinMBR(otherfactorsfixed)betweenamunicipal-itywithacreditratingofoneandamunic-QUESTION7.3ipalitywithacreditratingofzero;2istheInmodel(7.12),howwouldyoutestthenullhypothesisthatcreditdifferenceinMBRbetweenamunicipalityratinghasnoeffectonMBR?withacreditratingoftwoandamunici-palitywithacreditratingofzero;andsoon.Themovementbetweeneachcreditratingisallowedtohaveadifferenteffect,sousing(7.12)ismuchmoreflexiblethansimplyputtingCRinasasinglevariable.Oncethedummyvariablesaredefined,estimating(7.12)isstraightforward.EXAMPLE7.7(EffectsofPhysicalAttractivenessonWage)HamermeshandBiddle(1994)usedmeasuresofphysicalattractivenessinawageequation.Eachpersoninthesamplewasrankedbyaninterviewerforphysicalattractiveness,using222\nd7/14/995:55PMPage223Chapter7MultipleRegressionAnalysisWithQualitativeInformation:Binary(orDummy)Variablesfivecategories(homely,quiteplain,average,goodlooking,andstrikinglybeautifulorhand-some).Becausetherearesofewpeopleatthetwoextremes,theauthorsputpeopleintooneofthreegroupsfortheregressionanalysis:average,belowaverage,andaboveaver-age,wherethebasegroupisaverage.Usingdatafromthe1977QualityofEmploymentSurvey,aftercontrollingfortheusualproductivitycharacteristics,HamermeshandBiddleestimatedanequationformen:logˆ(wage)ˆ0(.164)belavg(.016)abvavgotherfactorslogˆ(wage)ˆ0(.046)belavg(.033)abvavgotherfactors2n700,R¯.403andanequationforwomen:logˆ(wage)ˆ0(.124)belavg(.035)abvavgotherfactorslogˆ(wage)ˆ0(.066)belavg(.049)abvavgotherfactors2n409,R¯.330.Theotherfactorscontrolledforintheregressionsincludeeducation,experience,tenure,maritalstatus,andrace;seeTable3inHamermeshandBiddle’spaperforamorecompletelist.Inordertosavespace,thecoefficientsontheothervariablesarenotreportedinthepaperandneitheristheintercept.Formen,thosewithbelowaveragelooksareestimatedtoearnabout16.4%lessthananaveragelookingmanwhoisthesameinotherrespects(includingeducation,experience,tenure,maritalstatus,andrace).Theeffectisstatisticallydifferentfromzero,witht3.57.Similarly,menwithaboveaveragelooksearnanestimated1.6%more,althoughtheeffectisnotstatisticallysignificant(t.5).Awomanwithbelowaveragelooksearnsabout12.4%lessthananotherwisecom-parableaveragelookingwoman,witht1.88.Aswasthecaseformen,theestimateonabvavgisnotstatisticallydifferentfromzero.Insomecases,theordinalvariabletakesontoomanyvaluessothatadummyvari-ablecannotbeincludedforeachvalue.Forexample,thefileLAWSCH85.RAWcon-tainsdataonmedianstartingsalariesforlawschoolgraduates.Oneofthekeyexplanatoryvariablesistherankofthelawschool.Sinceeachlawschoolhasadiffer-entrank,weclearlycannotincludeadummyvariableforeachrank.Ifwedonotwishtoputtherankdirectlyintheequation,wecanbreakitdownintocategories.Thefol-lowingexampleshowshowthisisdone.EXAMPLE7.8(EffectsofLawSchoolRankingsonStartingSalaries)Definethedummyvariablestop10,r11_25,r26_40,r41_60,r61_100totakeonthevalueunitywhenthevariablerankfallsintotheappropriaterange.Weletschoolsrankedbelow100bethebasegroup.Theestimatedequationis223\nd7/14/995:55PMPage224Part1RegressionAnalysiswithCross-SectionalDatalog(saˆlary)(9.17)(.700)top10(.594)r11_25(.375)r26_40(0.41)(.053)(.039)(.034)(.263)r41_60(.132)r61_100(.0057)LSAT(.028)(.021)(.0031)(7.13)(.014)GPA(.036)log(libvol)(.0008)log(cost)(.074)(.026)(.0251)22n136,R.911,R¯.905.Weseeimmediatelythatallofthedummyvariablesdefiningthedifferentranksareverystatisticallysignificant.Theestimateonr61_100meansthat,holdingLSAT,GPA,libvol,andcostfixed,themediansalaryatalawschoolrankedbetween61and100isabout13.2%higherthanthatatalawschoolrankedbelow100.Thedifferencebetweenatop10schoolandabelow100schoolisquitelarge.Usingtheexactcalculationgiveninequation(7.10)givesexp(.700)11.014,andsothepredictedmediansalaryismorethan100%higheratatop10schoolthanitisatabelow100school.Asanindicationofwhetherbreakingtherankintodifferentgroupsisanimprovement,wecancomparetheadjustedR-squaredin(7.13)withtheadjustedR-squaredfrominclud-ingrankasasinglevariable:theformeris.905andthelatteris.836,sotheadditionalflex-ibilityof(7.13)iswarranted.Interestingly,oncetherankisputintothe(admittedlysomewhatarbitrary)givencate-gories,alloftheothervariablesbecomeinsignificant.Infact,atestforjointsignificanceofLSAT,GPA,log(libvol),andlog(cost)givesap-valueof.055,whichisborderlinesignificant.Whenrankisincludedinitsoriginalform,thep-valueforjointsignificanceiszerotofourdecimalplaces.Onefinalcommentaboutthisexample.Inderivingthepropertiesofordinaryleastsquares,weassumedthatwehadarandomsample.Thecurrentapplicationviolatesthatassumptionbecauseofthewayrankisdefined:aschool’sranknecessarilydependsontherankoftheotherschoolsinthesample,andsothedatacannotrepresentindependentdrawsfromthepopulationofalllawschools.Thisdoesnotcauseanyseriousproblemspro-videdtheerrortermisuncorrelatedwiththeexplanatoryvariables.7.4INTERACTIONSINVOLVINGDUMMYVARIABLESInteractionsAmongDummyVariablesJustasvariableswithquantitativemeaningcanbeinteractedinregressionmodels,socandummyvariables.WehaveeffectivelyseenanexampleofthisinExample7.6,wherewedefinedfourcategoriesbasedonmaritalstatusandgender.Infact,wecanrecastthatmodelbyaddinganinteractiontermbetweenfemaleandmar-riedtothemodelwherefemaleandmarriedappearseparately.Thisallowsthemarriagepremiumtodependongender,justasitdidinequation(7.11).Forpur-posesofcomparison,theestimatedmodelwiththefemale-marriedinteractiontermis224\nd7/14/995:55PMPage225Chapter7MultipleRegressionAnalysisWithQualitativeInformation:Binary(orDummy)Variableslog(ˆwage)(.321)(.110)female(.213)marriedlog(ˆwage)(.100)(.056)female(.055)married(7.14)(.301)femalemarried…,(.072)femalemarried…,wheretherestoftheregressionisnecessarilyidenticalto(7.11).Equation(7.14)showsexplicitlythatthereisastatisticallysignificantinteractionbetweengenderandmaritalstatus.Thismodelalsoallowsustoobtaintheestimatedwagedifferentialamongallfourgroups,butherewemustbecarefultopluginthecorrectcombinationofzerosandones.Settingfemale0andmarried0correspondstothegroupsinglemen,whichisthebasegroup,sincethiseliminatesfemale,married,andfemalemarried.Wecanfindtheinterceptformarriedmenbysettingfemale0andmarried1in(7.14);thisgivesaninterceptof.321.213.534.Andsoon.Equation(7.14)isjustadifferentwayoffindingwagedifferentialsacrossallgen-der-maritalstatuscombinations.Ithasnorealadvantagesover(7.11);infact,equation(7.11)makesiteasiertotestfordifferentialsbetweenanygroupandthebasegroupofsinglemen.EXAMPLE7.9(EffectsofComputerUsageonWages)Krueger(1993)estimatestheeffectsofcomputerusageonwages.Hedefinesadummyvari-able,whichwecallcompwork,equaltooneifanindividualusesacomputeratwork.Anotherdummyvariable,comphome,equalsoneifthepersonusesacomputerathome.Using13,379peoplefromthe1989CurrentPopulationSurvey,Krueger(1993,Table4)obtainslog(ˆwage)ˆ0(.177)compwork(.070)comphomelog(ˆwage)ˆ0(.009)compwork(.019)comphome(7.15)(.017)compworkcomphomeotherfactors.(.023)compworkcomphomeotherfactors.(Theotherfactorsarethestandardonesforwageregressions,includingeducation,experi-ence,gender,andmaritalstatus;seeKrueger’spaperfortheexactlist.)Kruegerdoesnotreporttheinterceptbecauseitisnotofanyimportance;allweneedtoknowisthatthebasegroupconsistsofpeoplewhodonotuseacomputerathomeoratwork.Itisworthnotic-ingthattheestimatedreturntousingacomputeratwork(butnotathome)isabout17.7%.(Themorepreciseestimateis19.4%.)Similarly,peoplewhousecomputersathomebutnotatworkhaveabouta7%wagepremiumoverthosewhodonotuseacomputeratall.Thedifferentialbetweenthosewhouseacomputeratbothplaces,relativetothosewhouseacomputerinneitherplace,isabout26.4%(obtainedbyaddingallthreecoefficientsandmultiplyingby100),orthemorepreciseestimate30.2%obtainedfromequation(7.10).Theinteractiontermin(7.15)isnotstatisticallysignificant,norisitverybigeconomi-cally.Butitiscausinglittleharmbybeingintheequation.225\nd7/14/995:55PMPage226Part1RegressionAnalysiswithCross-SectionalDataAllowingforDifferentSlopesWehavenowseenseveralexamplesofhowtoallowdifferentinterceptsforanynum-berofgroupsinamultipleregressionmodel.Therearealsooccasionsforinteractingdummyvariableswithexplanatoryvariablesthatarenotdummyvariablestoallowfordifferencesinslopes.Continuingwiththewageexample,supposethatwewishtotestwhetherthereturntoeducationisthesameformenandwomen,allowingforaconstantwagedifferentialbetweenmenandwomen(adifferentialforwhichwehavealreadyfoundevidence).Forsimplicity,weincludeonlyeducationandgenderinthemodel.Whatkindofmodelallowsforaconstantwagedifferentialaswellasdifferentreturnstoeducation?Considerthemodellog(wage)(00female)(11female)educu.(7.16)Ifweplugfemale0into(7.16),thenwefindthattheinterceptformalesis0,andtheslopeoneducationformalesis1.Forfemales,wepluginfemale1;thus,theinterceptforfemalesis00,andtheslopeis11.Therefore,0measuresthedifferenceininterceptsbetweenwomenandmen,and1measuresthedifferenceinthereturntoeducationbetweenwomenandmen.Twoofthefourcasesforthesignsof0and1arepresentedinFigure7.2.Figure7.2Graphsofequation(7.16).(a)00,10;(b)00,10.wagewagemenwomenmenwomen(a)educ(b)educ226\nd7/14/995:55PMPage227Chapter7MultipleRegressionAnalysisWithQualitativeInformation:Binary(orDummy)VariablesGraph(a)showsthecasewheretheinterceptforwomenisbelowthatformen,andtheslopeofthelineissmallerforwomenthanformen.Thismeansthatwomenearnlessthanmenatalllevelsofeducation,andthegapincreasesaseducgetslarger.Ingraph(b),theinterceptforwomenisbelowthatformen,buttheslopeoneducationislargerforwomen.Thismeansthatwomenearnlessthanmenatlowlevelsofeduca-tion,butthegapnarrowsaseducationincreases.Atsomepoint,awomanearnsmorethanaman,giventhesamelevelsofeducation(andthispointiseasilyfoundgiventheestimatedequation).Howcanweestimatemodel(7.16)?InordertoapplyOLS,wemustwritethemodelwithaninteractionbetweenfemaleandeduc:log(wage)00female1educ1femaleeducu.(7.17)Theparameterscannowbeestimatedfromtheregressionoflog(wage)onfemale,educ,andfemaleeduc.Obtainingtheinteractiontermiseasyinanyregressionpackage.Donotbedauntedbytheoddnatureoffemaleeduc,whichiszeroforanymaninthesam-pleandequaltothelevelofeducationforanywomaninthesample.Animportanthypothesisisthatthereturntoeducationisthesameforwomenandmen.Intermsofmodel(7.17),thisisstatedasH0:10,whichmeansthattheslopeoflog(wage)withrespecttoeducisthesameformenandwomen.Notethatthishypothesisputsnorestrictionsonthedifferenceinintercepts,0.Awagedifferentialbetweenmenandwomenisallowedunderthisnull,butitmustbethesameatalllev-elsofeducation.ThissituationisdescribedbyFigure7.1.Wearealsointerestedinthehypothesisthataveragewagesareidenticalformenandwomenwhohavethesamelevelsofeducation.Thismeansthat0and1mustbothbezerounderthenullhypothesis.Inequation(7.17),wemustuseanFtesttotestH0:00,10.Inthemodelwithjustaninterceptdifference,werejectthishypoth-esisbecauseH0:00issoundlyrejectedagainstH1:00.EXAMPLE7.10(LogHourlyWageEquation)Weaddquadraticsinexperienceandtenureto(7.17):logˆ(wage)(.389)(.227)female(.082)educlogˆ(wage)(.119)(.168)female(.008)educ2(.0056)femaleeduc(.029)exper(.00058)exper2(.0131)femalec(.005)exper(.00011)exper(7.18)2(.032)tenure(.00059)tenure2(.007)tenure(.00024)tenure2n526,R.441.Theestimatedreturntoeducationformeninthisequationis.082,or8.2%.Forwomen,itis.082.0056.0764,orabout7.6%.Thedifference,.56%,orjustoverone-half227\nd7/14/995:55PMPage228Part1RegressionAnalysiswithCross-SectionalDataapercentagepointlessforwomen,isnoteconomicallylargenorstatisticallysignificant:thetstatisticis.0056/.0131.43.Thus,weconcludethatthereisnoevidenceagainstthehypothesisthatthereturntoeducationisthesameformenandwomen.Thecoefficientonfemale,whileremainingeconomicallylarge,isnolongersignificantatconventionallevels(t1.35).Itscoefficientandtstatisticintheequationwithouttheinteractionwere.297and8.25,respectively[seeequation(7.9)].Shouldwenowconcludethatthereisnostatisticallysignificantevidenceoflowerpayforwomenatthesamelevelsofeduc,exper,andtenure?Thiswouldbeaseriouserror.Sincewehaveaddedtheinteractionfemaleeductotheequation,thecoefficientonfemaleisnowesti-matedmuchlesspreciselythanitwasinequation(7.9):thestandarderrorhasincreasedbyalmostfive-fold(.168/.0364.67).Thereasonforthisisthatfemaleandfemaleeducarehighlycorrelatedinthesample.Inthisexample,thereisausefulwaytothinkaboutthemulticollinearity:inequation(7.17)andthemoregeneralequationestimatedin(7.18),0measuresthewagedifferentialbetweenwomenandmenwheneduc0.Asthereisnooneinthesamplewithevenclosetozeroyearsofeducation,itisnotsurpris-ingthatwehaveadifficulttimeestimatingthedifferentialateduc0(noristhediffer-entialatzeroyearsofeducationveryinformative).Moreinterestingwouldbetoestimatethegenderdifferentialat,say,theaverageeducationlevelinthesample(about12.5).Todothis,wewouldreplacefemaleeducwithfemale(educ12.5)andreruntheregression;thisonlychangesthecoefficientonfemaleanditsstandarderror.(SeeExer-cise7.15.)IfwecomputetheFstatisticforH0:00,10,weobtainF34.33,whichisahugevalueforanFrandomvariablewithnumeratordf2anddenominatordf518:thep-valueiszerotofourdecimalplaces.Intheend,weprefermodel(7.9),whichallowsforaconstantwagedifferentialbetweenwomenandmen.Asamorecomplicatedexamplein-QUESTION7.4volvinginteractions,wenowlookattheef-Howwouldyouaugmentthemodelestimatedin(7.18)toallowthefectsofraceandcityracialcompositiononreturntotenuretodifferbygender?majorleaguebaseballplayersalaries.EXAMPLE7.11(EffectsofRaceonBaseballPlayerSalaries)Thefollowingequationisestimatedforthe330majorleaguebaseballplayersforwhichcityracialcompositionstatisticsareavailable.Thevariablesblackandhispanarebinaryindica-torsfortheindividualplayers.(Thebasegroupiswhiteplayers.)Thevariablepercblckisthepercentageoftheteam’scitythatisblack,andperchispisthepercentageofHispanics.Theothervariablesmeasureaspectsofplayerproductivityandlongevity.Here,weareinterestedinraceeffectsaftercontrollingfortheseotherfactors.Inadditiontoincludingblackandhispanintheequation,weaddtheinteractionsblackpercblckandhispanperchisp.Theestimatedequationis228\nd7/14/995:55PMPage229Chapter7MultipleRegressionAnalysisWithQualitativeInformation:Binary(orDummy)Variableslog(saˆlary)(10.34)(.0673)years(.0089)gamesyrlog(saˆlary)(2.18)(.0129)years(.0034)gamesyr(.00095)bavg(.0146)hrunsyr(.0045)rbisyr(.00151)bavg(.0164)hrunsyr(.0076)rbisyr(.0072)runsyr(.0011)fldperc(.0075)allstar(7.19)(.0046)runsyr(.0021)fldperc(.0029)allstar(.198)black(.190)hispan(.0125)blackpercblck(.125)black(.153)hispan(.0050)blackpercblck2(.0201)hispanperchisp,n330,R.638.2(.0098)hispanperchisp,n330,R.638.First,weshouldtestwhetherthefourracevariables,black,hispan,blackpercblck,andhispanperchisparejointlysignificant.Usingthesame330players,theR-squaredwhenthefourracevariablesaredroppedis.626.Sincetherearefourrestrictionsanddf33013intheunrestrictedmodel,theFstatisticisabout2.63,whichyieldsap-valueof.034.Thus,thesevariablesarejointlysignificantatthe5%level(thoughnotatthe1%level).Howdoweinterpretthecoefficientsontheracevariables?Inthefollowingdiscussion,allproductivityfactorsareheldfixed.First,considerwhathappensforblackplayers,hold-ingperchispfixed.Thecoefficient.198onblackliterallymeansthat,ifablackplayerisinacitywithnoblacks(percblck0),thentheblackplayerearnsabout19.8%lessthanacomparablewhiteplayer.Aspercblckincreases—whichmeansthewhitepopulationdecreases,sinceperchispisheldfixed—thesalaryofblacksincreasesrelativetothatforwhites.Inacitywith10%blacks,log(salary)forblackscomparedtothatforwhitesis.198.0125(10).073,sosalaryisabout7.3%lessforblacksthanforwhitesinsuchacity.Whenpercblck20,blacksearnabout5.2%morethanwhites.Thelargestper-centageofblacksinacityisabout74%(Detroit).Similarly,HispanicsearnlessthanwhitesincitieswithalowpercentageofHispanics.ButwecaneasilyfindthevalueofperchispthatmakesthedifferentialbetweenwhitesandHispanicsequalzero:itmustmake.190.0201perchisp0,whichgivesperchisp9.45.ForcitiesinwhichthepercentofHispanicsislessthan9.45%,Hispanicsarepredictedtoearnlessthanwhites(foragivenblackpopulation),andtheoppositeistrueifthenum-berofHispanicsisabove9.45%.Twelveofthetwenty-twocitiesrepresentedinthesam-plehaveHispanicpopulationsthatarelessthan6%ofthetotalpopulation.ThelargestpercentageofHispanicsisabout31%.Howdoweinterpretthesefindings?WecannotsimplyclaimdiscriminationexistsagainstblacksandHispanics,becausetheestimatesimplythatwhitesearnlessthanblacksandHispanicsincitiesheavilypopulatedbyminorities.Theimportanceofcitycompositiononsalariesmightbeduetoplayerpreferences:perhapsthebestblackplayerslivedispro-portionatelyincitieswithmoreblacksandthebestHispanicplayerstendtobeincitieswithmoreHispanics.Theestimatesin(7.19)allowustodeterminethatsomerelationshipispre-sent,butwecannotdistinguishbetweenthesetwohypotheses.229\nd7/14/995:55PMPage230Part1RegressionAnalysiswithCross-SectionalDataTestingforDifferencesinRegressionFunctionsAcrossGroupsThepreviousexamplesillustratethatinteractingdummyvariableswithotherindepen-dentvariablescanbeapowerfultool.Sometimes,wewishtotestthenullhypothesisthattwopopulationsorgroupsfollowthesameregressionfunction,againstthealter-nativethatoneormoreoftheslopesdifferacrossthegroups.Wewillalsoseeexam-plesofthisinChapter13,whenwediscusspoolingdifferentcrosssectionsovertime.Supposewewanttotestwhetherthesameregressionmodeldescribescollegegradepointaveragesformaleandfemalecollegeathletes.Theequationiscumgpa01sat2hsperc3tothrsu,wheresatisSATscore,hspercishighschoolrankpercentile,andtothrsistotalhoursofcollegecourses.Weknowthat,toallowforaninterceptdifference,wecanincludeadummyvariableforeithermalesorfemales.Ifwewantanyoftheslopestodependongender,wesimplyinteracttheappropriatevariablewith,say,female,andincludeitintheequation.Ifweareinterestedintestingwhetherthereisanydifferencebetweenmenandwomen,thenwemustallowamodelwheretheinterceptandallslopescanbedifferentacrossthetwogroups:cumgpa00female1sat1femalesat2hsperc(7.20)2femalehsperc3tothrs3femaletothrsu.Theparameter0isthedifferenceintheinterceptbetweenwomenandmen,1istheslopedifferencewithrespecttosatbetweenwomenandmen,andsoon.ThenullhypothesisthatcumgpafollowsthesamemodelformalesandfemalesisstatedasH0:00,10,20,30.(7.21)Ifoneofthejisdifferentfromzero,thenthemodelisdifferentformenandwomen.UsingthespringsemesterdatafromthefileGPA3.RAW,thefullmodelisestimatedascumˆgpa(1.48)(.353)female(.0011)sat(.00075)femalesatcumˆgpa(0.21)(.411)female(.0002)sat(.00039)femalesat(.0085)hsperc(.00055)femalehsperc(.0023)tothrs(.0014)hsperc(.00316)femalehsperc(.0009)tothrs(7.22)(.00012)femaletothrs(.00163)femaletothrs22n366,R.406,R¯.394.Thefemaledummyvariableandnoneoftheinteractiontermsareverysignificant;onlythefemalesatinteractionhasatstatisticclosetotwo.Butweknowbetterthantorelyontheindividualtstatisticsfortestingajointhypothesissuchas(7.21).TocomputetheFstatistic,wemustestimatetherestrictedmodel,whichresultsfromdroppingfemale230\nd7/14/995:55PMPage231Chapter7MultipleRegressionAnalysisWithQualitativeInformation:Binary(orDummy)Variables22andalloftheinteractions;thisgivesanR(therestrictedR)ofabout.352,sotheFsta-tisticisabout8.14;thep-valueiszerotofivedecimalplaces,whichcausesustosoundlyreject(7.21).Thus,menandwomenathletesdofollowdifferentGPAmodels,eventhougheachtermin(7.22)thatallowswomenandmentobedifferentisindivid-uallyinsignificantatthe5%level.Thelargestandarderrorsonfemaleandtheinteractiontermsmakeitdifficulttotellexactlyhowmenandwomendiffer.Wemustbeverycarefulininterpretingequation(7.22)because,inobtainingdifferencesbetweenwomenandmen,theinteractiontermsmustbetakenintoaccount.Ifwelookonlyatthefemalevariable,wewouldwronglyconcludethatcumgpaisabout.353lessforwomenthanformen,holdingotherfactorsfixed.Thisistheestimateddifferenceonlywhensat,hsperc,andtothrsareallsettozero,whichisnotaninterestingscenario.Atsat1,100,hsperc10,andtothrs50,thepredicteddifferencebetweenawomanandamanis.353.00075(1,100).00055(10).00012(50).461.Thatis,thefemaleathleteispredictedtohaveaGPAthatisalmostone-halfapointhigherthanthecomparablemaleathlete.Inamodelwiththreevariables,sat,hsperc,andtothrs,itisprettysimpletoaddalloftheinteractionstotestforgroupdifferences.Insomecases,manymoreexplanatoryvariablesareinvolved,andthenitisconvenienttohaveadifferentwaytocomputethestatistic.ItturnsoutthatthesumofsquaredresidualsformoftheFstatisticcanbecom-putedeasilyevenwhenmanyindependentvariablesareinvolved.Inthegeneralmodelwithkexplanatoryvariablesandanintercept,supposewehavetwogroups,callthemg1andg2.Wewouldliketotestwhethertheinterceptandallslopesarethesameacrossthetwogroups.Writethemodelasyg,0g,1x1g,2x2…g,kxku,(7.23)forg1andg2.Thehypothesisthateachbetain(7.23)isthesameacrossthetwogroupsinvolvesk1restrictions(intheGPAexample,k14).Theunrestrictedmodel,whichwecanthinkofashavingagroupdummyvariableandkinteractiontermsinadditiontotheinterceptandvariablesthemselves,hasn2(k1)degreesoffree-dom.[IntheGPAexample,n2(k1)3662(4)358.]Sofar,thereisnoth-ingnew.Thekeyinsightisthatthesumofsquaredresidualsfromtheunrestrictedmodelcanbeobtainedfromtwoseparateregressions,oneforeachgroup.LetSSR1bethesumofsquaredresidualsobtainedestimating(7.23)forthefirstgroup;thisinvolvesn1observations.LetSSR2bethesumofsquaredresidualsobtainedfromestimatingthemodelusingthesecondgroup(n2observations).Inthepreviousexample,ifgroup1isfemales,thenn190andn2276.Now,thesumofsquaredresidualsfortheunre-strictedmodelissimplySSRurSSR1SSR2.Therestrictedsumofsquaredresidu-alsisjusttheSSRfrompoolingthegroupsandestimatingasingleequation.Oncewehavethese,wecomputetheFstatisticasusual:[SSR(SSR1SSR2)][n2(k1)]F(7.24)SSR1SSR2k1wherenisthetotalnumberofobservations.ThisparticularFstatisticisusuallycalledtheChowstatisticineconometrics.231\nd7/14/995:55PMPage232Part1RegressionAnalysiswithCross-SectionalDataToapplytheChowstatistictotheGPAexample,weneedtheSSRfromthere-gressionthatpooledthegroupstogether:thisisSSRr85.515.TheSSRforthe90womeninthesampleisSSR119.603,andtheSSRforthemenisSSR258.752.Thus,SSRur19.60358.75278.355.TheFstatisticis[(85.51578.355)/78.355](358/4)8.18;ofcourse,subjecttoroundingerror,thisiswhatwegetusingtheR-squaredformofthetestinthemodelswithandwithouttheinteractionterms.(Awordofcaution:thereisnosimpleR-squaredformofthetestifseparateregressionshavebeenestimatedforeachgroup;theR-squaredformofthetestcanbeusedonlyifinteractionshavebeenincludedtocreatetheunrestrictedmodel.)OneimportantlimitationoftheChowtest,regardlessofthemethodusedtoimple-mentit,isthatthenullhypothesisallowsfornodifferencesatallbetweenthegroups.Inmanycases,itismoreinterestingtoallowforaninterceptdifferencebetweenthegroupsandthentotestforslopedifferences;wesawoneexampleofthisinthewageequationinExample7.10.Todothis,wemustusetheapproachofputtinginteractionsdirectlyintheequationandtestingjointsignificanceofallinteractions(withoutrestrict-ingtheintercepts).IntheGPAexample,wenowtakethenulltobeH0:10,20,30.(0isnotrestrictedunderthenull.)TheFstatisticforthesethreerestrictionsisabout1.53,whichgivesap-valueequalto.205.Thus,wedonotrejectthenullhypothesis.Failuretorejectthehypothesisthattheparametersmultiplyingtheinteractiontermsareallzerosuggeststhatthebestmodelallowsforaninterceptdifferenceonly:cumˆgpa(1.39)(.310)female(.0012)sat(.0084)hsperccumˆgpa(0.18)(.059)female(.0002)SAT(.0012)hsperc(.0025)tothrs(7.25)(.0007)tothrs22n366,R.398,R¯.392.Theslopecoefficientsin(7.25)areclosetothoseforthebasegroup(males)in(7.22);droppingtheinteractionschangesverylittle.However,femalein(7.25)ishighlysig-nificant:itststatisticisover5,andtheestimateimpliesthat,atgivenlevelsofsat,hsperc,andtothrs,afemaleathletehasapredictedGPAthatis.31pointshigherthanamaleathlete.Thisisapracticallyimportantdifference.7.5ABINARYDEPENDENTVARIABLE:THELINEARPROBABILITYMODELBynowwehavelearnedmuchaboutthepropertiesandapplicabilityofthemultiplelin-earregressionmodel.Inthelastseveralsections,westudiedhow,throughtheuseofbinaryindependentvariables,wecanincorporatequalitativeinformationasexplanatoryvariablesinamultipleregressionmodel.Inallofthemodelsupuntilnow,thedepen-dentvariableyhashadquantitativemeaning(forexample,yisadollaramount,atestscore,apercent,orthelogsofthese).Whathappensifwewanttousemultipleregres-siontoexplainaqualitativeevent?232\nd7/14/995:55PMPage233Chapter7MultipleRegressionAnalysisWithQualitativeInformation:Binary(orDummy)VariablesInthesimplestcase,andonethatoftenarisesinpractice,theeventwewouldliketoexplainisabinaryoutcome.Inotherwords,ourdependentvariable,y,takesononlytwovalues:zeroandone.Forexample,ycanbedefinedtoindicatewhetheranadulthasahighschooleducation;orycanindicatewhetheracollegestudentusedillegaldrugsduringagivenschoolyear;orycanindicatewhetherafirmwastakenoverbyanotherfirmduringagivenyear.Ineachoftheseexamples,wecanlety1denoteoneoftheoutcomesandy0theotheroutcome.Whatdoesitmeantowritedownamultipleregressionmodel,suchasy01x1…kxku,(7.26)whenyisabinaryvariable?Sinceycantakeononlytwovalues,jcannotbeinter-pretedasthechangeinygivenaone-unitincreaseinxj,holdingallotherfactorsfixed:yeitherchangesfromzerotooneorfromonetozero.Nevertheless,thejstillhaveusefulinterpretations.IfweassumethatthezeroconditionalmeanassumptionMLR.3holds,thatis,E(ux1,…,xk)0,thenwehave,asalways,E(yx)01x1…kxk.wherexisshorthandforalloftheexplanatoryvariables.Thekeypointisthatwhenyisabinaryvariabletakingonthevalueszeroandone,itisalwaystruethatP(y1x)E(yx):theprobabilityof“success”—thatis,theprobabilitythaty1—isthesameastheexpectedvalueofy.Thus,wehavetheimpor-tantequationP(y1x)01x1…kxk,(7.27)whichsaysthattheprobabilityofsuccess,sayp(x)P(y1x),isalinearfunctionofthexj.Equation(7.27)isanexampleofabinaryresponsemodel,andP(y1x)isalsocalledtheresponseprobability.(WewillcoverotherbinaryresponsemodelsinChapter17.)Becauseprobabilitiesmustsumtoone,P(y0x)1P(y1x)isalsoalinearfunctionofthexj.Themultiplelinearregressionmodelwithabinarydependentvariableiscalledthelinearprobabilitymodel(LPM)becausetheresponseprobabilityislinearinthepara-metersj.IntheLPM,jmeasuresthechangeintheprobabilityofsuccesswhenxjchanges,holdingotherfactorsfixed:P(y1x)jxj.(7.28)Withthisinmind,themultipleregressionmodelcanallowustoestimatetheeffectofvariousexplanatoryvariablesonqualitativeevents.ThemechanicsofOLSarethesameasbefore.Ifwewritetheestimatedequationasyˆˆ0ˆ1x1…ˆkxk,wemustnowrememberthatyˆisthepredictedprobabilityofsuccess.Therefore,ˆ0isthepredictedprobabilityofsuccesswheneachxjissettozero,whichmayormaynot233\nd7/14/995:55PMPage234Part1RegressionAnalysiswithCross-SectionalDatabeinteresting.Theslopecoefficientˆ1measuresthepredictedchangeintheprobabil-ityofsuccesswhenx1increasesbyoneunit.Inordertocorrectlyinterpretalinearprobabilitymodel,wemustknowwhatcon-stitutesa“success.”Thus,itisagoodideatogivethedependentvariableanamethatdescribestheeventy1.Asanexample,letinlf(“inthelaborforce”)beabinaryvari-ableindicatinglaborforceparticipationbyamarriedwomanduring1975:inlf1ifthewomanreportsworkingforawageoutsidethehomeatsomepointduringtheyear,andzerootherwise.Weassumethatlaborforceparticipationdependsonothersourcesofincome,includinghusband’searnings(nwifeinc,measuredinthousandsofdollars),yearsofeducation(educ),pastyearsoflabormarketexperience(exper),age,numberofchildrenlessthansixyearsold(kidslt6),andnumberofkidsbetween6and18yearsofage(kidsge6).UsingthedatafromMroz(1987),weestimatethefollowinglinearprobabilitymodel,where428ofthe753womeninthesamplereportbeinginthelaborforceatsomepointduring1975:inˆlf(.586)(.0034)nwifeinc(.038)educ(.039)experinˆlf(.154)(.0014)nwifei(.007)educ(.006)exper2(.00060)exper(.016)age(.262)kidslt6(.0130)kidsge6(7.29)(.00018)exper(.002)age(.034)kidslt6(.0132)kidsge62n753,R.264.Usingtheusualtstatistics,allvariablesin(7.29)exceptkidsge6arestatisticallysignif-icant,andallofthesignificantvariableshavetheeffectswewouldexpectbasedoneco-nomictheory(orcommonsense).Tointerprettheestimates,wemustrememberthatachangeintheindependentvari-ablechangestheprobabilitythatinlf1.Forexample,thecoefficientoneducmeansthat,everythingelsein(7.29)heldfixed,anotheryearofeducationincreasestheprob-abilityoflaborforceparticipationby.038.Ifwetakethisequationliterally,10moreyearsofeducationincreasestheprobabilityofbeinginthelaborforceby.038(10).38,whichisaprettylargeincreaseinaprobability.TherelationshipbetweentheprobabilityoflaborforceparticipationandeducisplottedinFigure7.3.Theotherindependentvariablesarefixedatthevaluesnwifeinc50,exper5,age30,kidslt61,andkidsge60forillustrationpurposes.Thepredictedprobabilityisnegativeuntileducationequals3.84years.Thisshouldnotcausetoomuchconcernbecause,inthissample,nowomanhaslessthanfiveyearsofeducation.Thelargestreportededucationis17years,andthisleadstoapredictedprobabilityof.5.Ifwesettheotherindependentvariablesatdifferentvalues,therangeofpredictedprobabilitieswouldchange.Butthemarginaleffectofanotheryearofeducationontheprobabilityoflaborforceparticipationisalways.038.Thecoefficientonnwifeincimpliesthat,ifnwifeinc10(whichmeansanincreaseof$10,000),theprobabilitythatawomanisinthelaborforcefallsby.034.Thisisnotanespeciallylargeeffectgiventhatanincreaseinincomeof$10,000isverysignificantintermsof1975dollars.Experiencehasbeenenteredasaquadratictoallowtheeffectofpastexperiencetohaveadiminishingeffectonthelaborforceparticipa-tionprobability.Holdingotherfactorsfixed,theestimatedchangeintheprobabilityisapproximatedas.0392(.0006)exper.039.0012exper.Thepointatwhichpast234\nd7/14/995:55PMPage235Chapter7MultipleRegressionAnalysisWithQualitativeInformation:Binary(orDummy)VariablesFigure7.3Estimatedrelationshipbetweentheprobabilityofbeinginthelaborforceandyearsofeducation,withotherexplanatoryvariablesfixed.ProbabilityofLaborForceParticipation.5slope=.03803.84educ–.146experiencehasnoeffectontheprobabilityoflaborforceparticipationis.039/.001232.5,whichisahighlevelofexperience:only13ofthe753womeninthesamplehavemorethan32yearsofexperience.Unlikethenumberofolderchildren,thenumberofyoungchildrenhasahugeimpactonlaborforceparticipation.Havingoneadditionalchildlessthansixyearsoldreducestheprobabilityofparticipationby.262,atgivenlevelsoftheothervariables.Inthesample,justunder20%ofthewomenhaveatleastoneyoungchild.Thisexampleillustrateshoweasylinearprobabilitymodelsaretoestimateandinterpret,butitalsohighlightssomeshortcomingsoftheLPM.First,itiseasytoseethat,ifweplugincertaincombinationsofvaluesfortheindependentvariablesinto(7.29),wecangetpredictionseitherlessthanzeroorgreaterthanone.Sincethesearepredictedprobabilities,andprobabilitiesmustbebetweenzeroandone,thiscanbealit-tleembarassing.Forexample,whatwoulditmeantopredictthatawomanisinthelaborforcewithaprobabilityof.10?Infact,ofthe753womeninthesample,16ofthefit-tedvaluesfrom(7.29)arelessthanzero,and17ofthefittedvaluesaregreaterthanone.Arelatedproblemisthataprobabilitycannotbelinearlyrelatedtotheindependentvariablesforalltheirpossiblevalues.Forexample,(7.29)predictsthattheeffectofgoingfromzerochildrentooneyoungchildreducestheprobabilityofworkingby.262.Thisisalsothepredicteddropifthewomangoesfromhaveoneyoungchildtotwo.Itseemsmorerealisticthatthefirstsmallchildwouldreducetheprobabilitybyalargeamount,butthensubsequentchildrenwouldhaveasmallermarginaleffect.Infact,235\nd7/14/995:55PMPage236Part1RegressionAnalysiswithCross-SectionalDatawhentakentotheextreme,(7.29)impliesthatgoingfromzerotofouryoungchildrenreducestheprobabilityofworkingbyinˆlf.262(kidslt6).262(4)1.048,whichisimpossible.Evenwiththeseproblems,thelinearprobabilitymodelisusefulandoftenappliedineconomics.Itusuallyworkswellforvaluesoftheindependentvariablesthatareneartheaveragesinthesample.Inthelaborforceparticipationexample,therearenowomeninthesamplewithfouryoungchildren;infact,onlythreewomenhavethreeyoungchildren.Over96%ofthewomenhaveeithernoyoungchildrenoronesmallchild,andsoweshouldprobablyrestrictattentiontothiscasewheninterpretingtheestimatedequation.Predictedprobabilitiesoutsidetheunitintervalarealittletroublingwhenwewanttomakepredictions,butthisisrarelycentraltoananalysis.Usually,wewanttoknowtheceterisparibuseffectofcertainvariablesontheprobability.Duetothebinarynatureofy,thelinearprobabilitymodeldoesviolateoneoftheGauss-Markovassumptions.Whenyisabinaryvariable,itsvariance,conditionalonx,isVar(yx)p(x)[1p(x)],(7.30)wherep(x)isshorthandfortheprobabilityofsuccess:p(x)01x1…kxk.Thismeansthat,exceptinthecasewheretheprobabilitydoesnotdependonanyoftheindependentvariables,theremustbeheteroskedasticityinalinearprobabilitymodel.WeknowfromChapter3thatthisdoesnotcausebiasintheOLSestimatorsofthej.ButwealsoknowfromChapters4and5thathomoskedasticityiscrucialforjustifyingtheusualtandFstatistics,eveninlargesamples.Becausethestandarderrorsin(7.29)arenotgenerallyvalid,weshouldusethemwithcaution.WewillshowhowtocorrectthestandarderrorsforheteroskedasticityinChapter8.Itturnsoutthat,inmanyappli-cations,theusualOLSstatisticsarenotfaroff,anditisstillacceptableinappliedworktopresentastandardOLSanalysisofalinearprobabilitymodel.EXAMPLE7.12(ALinearProbabilityModelofArrests)Letarr86beabinaryvariableequaltounityifamanwasarrestedduring1986,andzerootherwise.ThepopulationisagroupofyoungmeninCaliforniabornin1960or1961whohaveatleastonearrestpriorto1986.Alinearprobabilitymodelfordescribingarr86isarr8601pcnv2avgsen3tottime4ptime865qemp86u,wherepcnvistheproportionofpriorarreststhatledtoaconviction,avgsenistheaveragesentenceservedfrompriorconvictions(inmonths),tottimeismonthsspentinprisonsinceage18priorto1986,ptime86ismonthsspentinprisonin1986,andqemp86isthenum-berofquarters(0to4)thatthemanwaslegallyemployedin1986.ThedataweuseareinCRIME1.RAW,thesamedatasetusedforExample3.5.Hereweuseabinarydependentvariable,becauseonly7.2%ofthemeninthesamplewerearrestedmorethanonce.About27.7%ofthemenwerearrestedatleastonceduring1986.Theestimatedequationis236\nd7/14/995:55PMPage237Chapter7MultipleRegressionAnalysisWithQualitativeInformation:Binary(orDummy)Variablesarrˆ86(.441)(.162)pcnv(.0061)avgsen(.0023)tottimearrˆ86(.017)(.021)pcnv(.0065)avgsen(.0050)tottime(.022)ptime86(.043)qemp86(7.31)(.005)ptime86(.005)qemp862n2,725,R.0474.Theintercept,.441,isthepredictedprobabilityofarrestforsomeonewhohasnotbeenconvicted(andsopcnvandavgsenarebothzero),hasspentnotimeinprisonsinceage18,spentnotimeinprisonin1986,andwasunemployedduringtheentireyear.Thevari-ablesavgsenandtottimeareinsignificantbothindividuallyandjointly(theFtestgivesp-value.347),andavgsenhasacounterintuitivesigniflongersentencesaresupposedtodetercrime.Grogger(1991),usingasupersetofthesedataanddifferenteconometricmethods,foundthattottimehasastatisticallysignificantpositiveeffectonarrestsandcon-cludedthattottimeisameasureofhumancapitalbuiltupincriminalactivity.Increasingtheprobabilityofconvictiondoeslowertheprobabilityofarrest,butwemustbecarefulwheninterpretingthemagnitudeofthecoefficient.Thevariablepcnvisaproportionbetweenzeroandone;thus,changingpcnvfromzerotooneessentiallymeansachangefromnochanceofbeingconvictedtobeingconvictedwithcertainty.Eventhislargechangereducestheprobabilityofarrestonlyby.162;increasingpcnvby.5decreasestheprobabilityofarrestby.081.Theincarcerativeeffectisgivenbythecoefficientonptime86.Ifamanisinprison,hecannotbearrested.Sinceptime86ismeasuredinmonths,sixmoremonthsinprisonreducestheprobabilityofarrestby.022(6).132.Equation(7.31)givesanotherexampleofwherethelinearprobabilitymodelcannotbetrueoverallrangesoftheindependentvariables.Ifamanisinprisonall12monthsof1986,hecannotbearrestedin1986.Settingallothervariablesequaltozero,thepredictedprobabilityofarrestwhenptime8612is.441.022(12).177,whichisnotzero.Nevertheless,ifwestartfromtheunconditionalprobabilityofarrest,.277,12monthsinprisonreducestheprobabilitytoessentiallyzero:.277.022(12).013.Finally,employmentreducestheprobabilityofarrestinasignificantway.Allotherfac-torsfixed,amanemployedinallfourquartersis.172lesslikelytobearrestedthanamanwhowasnotemployedatall.Wecanalsoincludedummyindependentvariablesinmodelswithdummydepen-dentvariables.Thecoefficientmeasuresthepredicteddifferenceinprobabilitywhenthedummyvariablegoesfromzerotoone.Forexample,ifweaddtworacedummies,blackandhispan,tothearrestequation,weobtainarrˆ86(.380)(.152)pcnv(.0046)avgsen(.0026)tottimearrˆ86(.019)(.021)pcnv(.0064)avgsen(.0049)tottime(.024)ptime86(.038)qemp86(.170)black(.096)hispan(7.32)(.005)ptime86(.005)qemp86(.024)black(.021)hispan2n2,725,R.0682.237\nd7/14/995:55PMPage238Part1RegressionAnalysiswithCross-SectionalDataThecoefficientonblackmeansthat,allotherfactorsbeingequal,ablackmanhasa.17higherchanceofbeingarrestedthanawhiteman(thebasegroup).Anotherwaytosaythisisthattheprobabilityofarrestis17percentagepointshigherforblacksthanQUESTION7.5forwhites.ThedifferenceisstatisticallyWhatisthepredictedprobabilityofarrestforablackmanwithnosignificantaswell.Similarly,Hispanicpriorconvictions—sothatpcnv,avgsen,tottime,andptime86areallzero—whowasemployedallfourquartersin1986?Doesthisseemmenhavea.096higherchanceofbeingreasonable?arrestedthanwhitemen.7.6MOREONPOLICYANALYSISANDPROGRAMEVALUATIONWehaveseensomeexamplesofmodelscontainingdummyvariablesthatcanbeuse-fulforevaluatingpolicy.Example7.3gaveanexampleofprogramevaluation,wheresomefirmsreceivedjobtraininggrantsandothersdidnot.Aswementionedearlier,wemustbecarefulwhenevaluatingprogramsbecauseinmostexamplesinthesocialsciencesthecontrolandtreatmentgroupsarenotrandomlyassigned.ConsideragaintheHolzeretal.(1993)study,wherewearenowinterestedintheeffectofthejobtraininggrantsonworkerproductivity(asopposedtoamountofjobtraining).Theequationofinterestislog(scrap)01grant2log(sales)3log(employ)u,wherescrapisthefirm’sscraprate,andthelattertwovariablesareincludedascon-trols.Thebinaryvariablegrantindicateswhetherthefirmreceivedagrantin1988forjobtraining.Beforewelookattheestimates,wemightbeworriedthattheunobservedfactorsaffectingworkerproductivity—suchasaveragelevelsofeducation,ability,experience,andtenure—mightbecorrelatedwithwhetherthefirmreceivesagrant.Holzeretal.pointoutthatgrantsweregivenonafirst-come,first-servebasis.Butthisisnotthesameasgivingoutgrantsrandomly.Itmightbethatfirmswithlessproductiveworkerssawanopportunitytoimproveproductivityandthereforeweremorediligentinapply-ingforthegrants.UsingthedatainJTRAIN.RAWfor1988—whenfirmsactuallywereeligibletoreceivethegrants—weobtainlog(sˆcrap)(4.99)(.052)grant(.455)log(sales)log(sˆcrap)(4.66)(.431)grant(.373)log(sales)(.639)log(employ)(7.33)(.365)log(employ)2n50,R.072.(17outofthe50firmsreceivedatraininggrant,andtheaveragescraprateis3.47acrossallfirms.)Thepointestimateof.052ongrantmeansthat,forgivensalesandemploy,firmsreceivingagranthavescrapratesabout5.2%lowerthanfirmswithoutgrants.Thisisthedirectionoftheexpectedeffectifthetraininggrantsareeffective,but238\nd7/14/995:55PMPage239Chapter7MultipleRegressionAnalysisWithQualitativeInformation:Binary(orDummy)Variablesthetstatisticisverysmall.Thus,fromthiscross-sectionalanalysis,wemustconcludethatthegrantshadnoeffectonfirmproductivity.WewillreturntothisexampleinChapter9andshowhowaddinginformationfromaprioryearleadstoamuchdiffer-entconclusion.Evenincaseswherethepolicyanalysisdoesnotinvolveassigningunitstoacon-trolgroupandatreatmentgroup,wemustbecarefultoincludefactorsthatmightbesystematicallyrelatedtothebinaryindependentvariableofinterest.Agoodexampleofthisistestingforracialdiscrimination.Raceissomethingthatisnotdeterminedbyanindividualorbygovernmentadministrators.Infact,racewouldappeartobetheperfectexampleofanexogenousexplanatoryvariable,giventhatitisdeterminedatbirth.However,forhistoricalreasons,thisisnotthecase:therearesystematicdifferencesinbackgroundsacrossrace,andthesedifferencescanbeimportantintestingforcurrentdiscrimination.Asanexample,considertestingfordiscriminationinloanapprovals.Ifwecancol-lectdataon,say,individualmortgageapplications,thenwecandefinethedummydependentvariableapprovedasequaltooneifamortgageapplicationwasapproved,andzerootherwise.Asystematicdifferenceinapprovalratesacrossracesisanindica-tionofdiscrimination.However,sinceapprovaldependsonmanyotherfactors,includ-ingincome,wealth,creditratings,andageneralabilitytopaybacktheloan,wemustcontrolforthemiftherearesystematicdifferencesinthesefactorsacrossrace.Alinearprobabilitymodeltotestfordiscriminationmightlooklikethefollowing:approved01nonwhite2income3wealth4credrateotherfactors.DiscriminationagainstminoritiesisindicatedbyarejectionofH0:10infavorofH0:10,because1istheamountbywhichtheprobabilityofanonwhitegettinganapprovaldiffersfromtheprobabilityofawhitegettinganapproval,giventhesamelev-elsofothervariablesintheequation.Ifincome,wealth,andsoonaresystematicallydifferentacrossraces,thenitisimportanttocontrolforthesefactorsinamultipleregressionanalysis.Anotherproblemthatoftenarisesinpolicyandprogramevaluationisthatindivid-uals(orfirmsorcities)choosewhetherornottoparticipateincertainbehaviorsorpro-grams.Forexample,individualschoosetouseillegaldrugsordrinkalcohol.Ifwewanttoexaminetheeffectsofsuchbehaviorsonunemploymentstatus,earnings,orcriminalbehavior,weshouldbeconcernedthatdrugusagemightbecorrelatedwithotherfac-torsthatcanaffectemploymentandcriminaloutcomes.ChildreneligibleforprogramssuchasHeadStartparticipatebasedonparentaldecisions.SincefamilybackgroundplaysaroleinHeadStartdecisionsandaffectsstudentoutcomes,weshouldcontrolforthesefactorswhenexaminingtheeffectsofHeadStart[see,forexample,CurrieandThomas(1995)].Individualsselectedbyemployersorgovernmentagenciestopartici-pateinjobtrainingprogramscanparticipateornot,andthisdecisionisunlikelytoberandom[see,forexample,Lynch(1991)].Citiesandstateschoosewhethertoimple-mentcertainguncontrollaws,anditislikelythatthisdecisionissystematicallyrelatedtootherfactorsthataffectviolentcrime[see,forexample,KleckandPatterson(1993)].Thepreviousparagraphgivesexamplesofwhataregenerallyknownasself-selectionproblemsineconomics.Literally,thetermcomesfromthefactthatindividu-alsself-selectintocertainbehaviorsorprograms:participationisnotrandomlydeter-239\nd7/14/995:55PMPage240Part1RegressionAnalysiswithCross-SectionalDatamined.Thetermisusedgenerallywhenabinaryindicatorofparticipationmightbesystematicallyrelatedtounobservedfactors.Thus,ifwewritethesimplemodely01particu,(7.34)whereyisanoutcomevariableandparticisabinaryvariableequaltounityiftheindi-vidual,firm,orcityparticipatesinabehavior,aprogram,orhasacertainkindoflaw,thenweareworriedthattheaveragevalueofudependsonparticipation:E(upartic1)E(upartic0).Asweknow,thiscausesthesimpleregressionestimatorof1tobebiased,andsowewillnotuncoverthetrueeffectofparticipation.Thus,theself-selectionproblemisanotherwaythatanexplanatoryvariable(particinthiscase)canbeendogenous.Bynowweknowthatmultipleregressionanalysiscan,tosomedegree,alleviatetheself-selectionproblem.Factorsintheerrortermin(7.34)thatarecorrelatedwithparticcanbeincludedinamultipleregressionequation,assuming,ofcourse,thatwecancollectdataonthesefactors.Unfortunately,inmanycases,weareworriedthatunobservedfactorsarerelatedtoparticipation,inwhichcasemultipleregressionpro-ducesbiasedestimators.Withstandardmultipleregressionanalysisusingcross-sectionaldata,wemustbeawareoffindingspuriouseffectsofprogramsonoutcomevariablesduetotheself-selectionproblem.AgoodexampleofthisiscontainedinCurrieandCole(1993).TheseauthorsexaminetheeffectofAFDC(aidforfamilieswithdependentchildren)participationonthebirthweightofachild.Evenaftercontrollingforavarietyoffam-ilyandbackgroundcharacteristics,theauthorsobtainOLSestimatesthatimplypartic-ipationinAFDClowersbirthweight.Astheauthorspointout,itishardtobelievethatAFDCparticipationitselfcauseslowerbirthweight.[SeeCurrie(1995)foradditionalexamples.]UsingadifferenteconometricmethodthatwewilldiscussinChapter15,CurrieandColefindevidenceforeithernoeffectorapositiveeffectofAFDCpartici-pationonbirthweight.Whentheself-selectionproblemcausesstandardmultipleregressionanalysistobebiasedduetoalackofsufficientcontrolvariables,themoreadvancedmethodscoveredinChapters13,14,and15canbeusedinstead.SUMMARYInthischapter,wehavelearnedhowtousequalitativeinformationinregressionanalysis.Inthesimplestcase,adummyvariableisdefinedtodistinguishbetweentwogroups,andthecoefficientestimateonthedummyvariableestimatestheceterisparibusdifferencebetweenthetwogroups.Allowingformorethantwogroupsisaccomplishedbydefiningasetofdummyvariables:ifthereareggroups,theng1dummyvariablesareincludedinthemodel.Allestimatesonthedummyvariablesareinterpretedrelativetothebaseorbenchmarkgroup(thegroupforwhichnodummyvariableisincludedinthemodel).Dummyvariablesarealsousefulforincorporatingordinalinformation,suchasacreditorabeautyrating,inregressionmodels.Wesimplydefineasetofdummyvari-ablesrepresentingdifferentoutcomesoftheordinalvariable,allowingoneofthecate-goriestobethebasegroup.240\nd7/14/995:55PMPage241Chapter7MultipleRegressionAnalysisWithQualitativeInformation:Binary(orDummy)VariablesDummyvariablescanbeinteractedwithquantitativevariablestoallowslopedif-ferencesacrossdifferentgroups.Intheextremecase,wecanalloweachgrouptohaveitsownslopeoneveryvariable,aswellasitsownintercept.TheChowtestcanbeusedtodetectwhetherthereareanydifferencesacrossgroups.Inmanycases,itismoreinterestingtotestwhether,afterallowingforaninterceptdifference,theslopesfortwodifferentgroupsarethesame.AstandardFtestcanbeusedforthispurposeinanunre-strictedmodelthatincludesinteractionsbetweenthegroupdummyandallvariables.Thelinearprobabilitymodel,whichissimplyestimatedbyOLS,allowsustoexplainabinaryresponseusingregressionanalysis.TheOLSestimatesarenowinterpretedaschangesintheprobabilityof“success”(y1),givenaone-unitincreaseinthecorre-spondingexplanatoryvariable.TheLPMdoeshavesomedrawbacks:itcanproducepre-dictedprobabilitiesthatarelessthanzeroorgreaterthanone,itimpliesaconstantmarginaleffectofeachexplanatoryvariablethatappearsinitsoriginalform,anditcon-tainsheteroskedasticity.Thefirsttwoproblemsareoftennotseriouswhenweareobtain-ingestimatesofthepartialeffectsoftheexplanatoryvariablesforthemiddlerangesofthedata.HeteroskedasticitydoesinvalidatetheusualOLSstandarderrorsandteststatis-tics,but,aswewillseeinthenextchapter,thisiseasilyfixedinlargeenoughsamples.Weendedthischapterwithadiscussionofhowbinaryvariablesareusedtoevalu-atepoliciesandprograms.Asinallregressionanalysis,wemustrememberthatpro-gramparticipation,orsomeotherbinaryregressorwithpolicyimplications,mightbecorrelatedwithunobservedfactorsthataffectthedependentvariable,resultingintheusualomittedvariablesbias.KEYTERMSBaseGroupInteractionTermBenchmarkGroupInterceptShiftBinaryVariableLinearProbabilityModel(LPM)ChowStatisticOrdinalVariableControlGroupPolicyAnalysisDifferenceinSlopesProgramEvaluationDummyVariableTrapResponseProbabilityDummyVariablesSelf-selectionExperimentalGroupTreatmentGroupPROBLEMS7.1UsingthedatainSLEEP75.RAW(seealsoProblem3.3),weobtaintheestimatedequationsleˆep(3,840.83)(.163)totwrk(11.71)educ(8.70)agesleˆep(235.11)(.018)totwrk(5.86)educ(11.21)age2(.128)age(87.75)male2(.134)age(34.33)male22n706,R.123,R¯.117.241\nd7/14/995:55PMPage242Part1RegressionAnalysiswithCross-SectionalDataThevariablesleepistotalminutesperweekspentsleepingatnight,totwrkistotalweeklyminutesspentworking,educandagearemeasuredinyears,andmaleisagen-derdummy.(i)Allotherfactorsbeingequal,isthereevidencethatmensleepmorethanwomen?Howstrongistheevidence?(ii)Isthereastatisticallysignificanttradeoffbetweenworkingandsleep-ing?Whatistheestimatedtradeoff?(iii)Whatotherregressiondoyouneedtoruntotestthenullhypothesisthat,holdingotherfactorsfixed,agehasnoeffectonsleeping?7.2ThefollowingequationswereestimatedusingthedatainBWGHT.RAW:log(bˆwght)(4.66)(.0044)cigs(.0093)log(faminc)(.016)paritylog(bˆwght)(0.22)(.0009)cigs(.0059)log(faminc)(.006)parity(.027)male(.055)white(.010)male(.013)white2n1,388,R.0472andlog(bˆwght)(4.65)(.0052)cigs(.0110)log(faminc)(.017)paritylog(bˆwght)(0.38)(.0010)cigs(.0085)log(faminc)(.006)parity(.034)male(.045)white(.0030)motheduc(.0032)fatheduc(.011)male(.015)white(.0030)motheduc(.0026)fatheduc2n1,191,R.0493.ThevariablesaredefinedasinExample4.9,butwehaveaddedadummyvariableforwhetherthechildismaleandadummyvariableindicatingwhetherthechildisclassi-fiedaswhite.(i)Inthefirstequation,interpretthecoefficientonthevariablecigs.Inpar-ticular,whatistheeffectonbirthweightfromsmoking10moreciga-rettesperday?(ii)Howmuchmoreisawhitechildpredictedtoweighthananonwhitechild,holdingtheotherfactorsinthefirstequationfixed?Isthediffer-encestatisticallysignificant?(iii)Commentontheestimatedeffectandstatisticalsignificanceofmotheduc.(iv)Fromthegiveninformation,whyareyouunabletocomputetheFsta-tisticforjointsignificanceofmotheducandfatheduc?WhatwouldyouhavetodotocomputetheFstatistic?7.3UsingthedatainGPA2.RAW,thefollowingequationwasestimated:2saˆt(1,028.10)(19.30)hsize(2.19)hsize(45.09)female2saˆt1,02(6.29)1(3.83)hsize(0.53)hsize5(4.29)female(169.81)black(62.31)femaleblack0(12.71)black(18.15)femaleblack2n4,137,R.0858.242\nd7/14/995:55PMPage243Chapter7MultipleRegressionAnalysisWithQualitativeInformation:Binary(orDummy)VariablesThevariablesatisthecombinedSATscore,hsizeissizeofthestudent’shighschoolgraduatingclass,inhundreds,femaleisagenderdummyvariable,andblackisaracedummyvariableequaltooneforblacks,andzerootherwise.2(i)Istherestrongevidencethathsizeshouldbeincludedinthemodel?Fromthisequation,whatistheoptimalhighschoolsize?(ii)Holdinghsizefixed,whatistheestimateddifferenceinSATscorebetweennonblackfemalesandnonblackmales?Howstatisticallysig-nificantisthisestimateddifference?(iii)WhatistheestimateddifferenceinSATscorebetweennonblackmalesandblackmales?Testthenullhypothesisthatthereisnodifferencebetweentheirscores,againstthealternativethatthereisadifference.(iv)WhatistheestimateddifferenceinSATscorebetweenblackfemalesandnonblackfemales?Whatwouldyouneedtodototestwhetherthedifferenceisstatisticallysignificant?7.4Anequationexplainingchiefexecutiveofficersalaryislog(saˆlary)(4.59)(.257)log(sales)(.011)roe(.158)financelog(saˆlary)(0.30)(.032)log(sales)(.004)roe(.089)finance(.181)consprod(.283)utility(.085)consprod(.099)utility2n209,R.357.ThedatausedareinCEOSAL1.RAW,wherefinance,consprod,andutilityarebinaryvariablesindicatingthefinancial,consumerproducts,andutilitiesindustries.Theomit-tedindustryistransportation.(i)Computetheapproximatepercentagedifferenceinestimatedsalarybetweentheutilityandtransportationindustries,holdingsalesandroefixed.Isthedifferencestatisticallysignificantatthe1%level?(ii)Useequation(7.10)toobtaintheexactpercentagedifferenceinesti-matedsalarybetweentheutilityandtransportationindustriesandcom-parethiswiththeanswerobtainedinpart(i).(iii)Whatistheapproximatepercentagedifferenceinestimatedsalarybetweentheconsumerproductsandfinanceindustries?Writeanequa-tionthatwouldallowyoutotestwhetherthedifferenceisstatisticallysignificant.7.5InExample7.2,letnoPCbeadummyvariableequaltooneifthestudentdoesnotownaPC,andzerootherwise.(i)IfnoPCisusedinplaceofPCinequation(7.6),whathappenstotheinterceptintheestimatedequation?WhatwillbethecoefficientonnoPC?(Hint:WritePC1noPCandplugthisintotheequationcolˆGPAˆ0ˆ0PCˆ1hsGPAˆ2ACT.)(ii)WhatwillhappentotheR-squaredifnoPCisusedinplaceofPC?(iii)ShouldPCandnoPCbothbeincludedasindependentvariablesinthemodel?Explain.7.6Totesttheeffectivenessofajobtrainingprogramonthesubsequentwagesofworkers,wespecifythemodel243\nd7/14/995:55PMPage244Part1RegressionAnalysiswithCross-SectionalDatalog(wage)01train2educ3experu,wheretrainisabinaryvariableequaltounityifaworkerparticipatedintheprogram.Thinkoftheerrortermuascontainingunobservedworkerability.Iflessableworkershaveagreaterchanceofbeingselectedfortheprogram,andyouuseanOLSanalysis,whatcanyousayaboutthelikelybiasintheOLSestimatorof1?(Hint:ReferbacktoChapter3.)7.7Intheexampleinequation(7.29),supposethatwedefineoutlftobeoneifthewomanisoutofthelaborforce,andzerootherwise.(i)Ifweregressoutlfonalloftheindependentvariablesinequation(7.29),whatwillhappentotheinterceptandslopeestimates?(Hint:inlf1outlf.Plugthisintothepopulationequationinlf01nwifeinc2educ…andrearrange.)(ii)Whatwillhappentothestandarderrorsontheinterceptandslopeesti-mates?(iii)WhatwillhappentotheR-squared?7.8Supposeyoucollectdatafromasurveyonwages,education,experience,andgen-der.Inaddition,youaskforinformationaboutmarijuanausage.Theoriginalquestionis:“Onhowmanyseparateoccasionslastmonthdidyousmokemarijuana?”(i)Writeanequationthatwouldallowyoutoestimatetheeffectsofmari-juanausageonwage,whilecontrollingforotherfactors.Youshouldbeabletomakestatementssuchas,“Smokingmarijuanafivemoretimespermonthisestimatedtochangewagebyx%.”(ii)Writeamodelthatwouldallowyoutotestwhetherdrugusagehasdif-ferenteffectsonwagesformenandwomen.Howwouldyoutestthattherearenodifferencesintheeffectsofdrugusageformenandwomen?(iii)Supposeyouthinkitisbettertomeasuremarijuanausagebyputtingpeopleintooneoffourcategories:nonuser,lightuser(1to5timespermonth),moderateuser(6to10timespermonth),andheavyuser(morethan10timespermonth).Nowwriteamodelthatallowsyoutoesti-matetheeffectsofmarijuanausageonwage.(iv)Usingthemodelinpart(iii),explainindetailhowtotestthenullhypothesisthatmarijuanausagehasnoeffectonwage.Beveryspecificandincludeacarefullistingofdegreesoffreedom.(v)Whataresomepotentialproblemswithdrawingcausalinferenceusingthesurveydatathatyoucollected?COMPUTEREXERCISES7.9UsethedatainGPA1.RAWforthisexercise.(i)Addthevariablesmothcollandfathcolltotheequationestimatedin(7.6)andreporttheresultsintheusualform.Whathappenstotheesti-matedeffectofPCownership?IsPCstillstatisticallysignificant?244\nd7/14/995:55PMPage245Chapter7MultipleRegressionAnalysisWithQualitativeInformation:Binary(orDummy)Variables(ii)Testforjointsignificanceofmothcollandfathcollintheequationfrompart(i)andbesuretoreportthep-value.2(iii)AddhsGPAtothemodelfrompart(i)anddecidewhetherthisgener-alizationisneeded.7.10UsethedatainWAGE2.RAWforthisexercise.(i)Estimatethemodellog(wage)01educ2exper3tenure4married5black6south7urbanuandreporttheresultsintheusualform.Holdingotherfactorsfixed,whatistheapproximatedifferenceinmonthlysalarybetweenblacksandnonblacks?Isthisdifferencestatisticallysignificant?22(ii)Addthevariablesexperandtenuretotheequationandshowthattheyarejointlyinsignificantateventhe20%level.(iii)Extendtheoriginalmodeltoallowthereturntoeducationtodependonraceandtestwhetherthereturntoeducationdoesdependonrace.(iv)Again,startwiththeoriginalmodel,butnowallowwagestodifferacrossfourgroupsofpeople:marriedandblack,marriedandnonblack,singleandblack,andsingleandnonblack.Whatistheestimatedwagedifferentialbetweenmarriedblacksandmarriednonblacks?7.11Amodelthatallowsmajorleaguebaseballplayersalarytodifferbypositionislog(salary)01years2gamesyr3bavg4hrunsyr5rbisyr6runsyr7fldperc8allstar9frstbase10scndbase11thrdbase12shrtstop13catcheru,whereoutfieldisthebasegroup.(i)Statethenullhypothesisthat,controllingforotherfactors,catchersandoutfieldersearn,onaverage,thesameamount.TestthishypothesisusingthedatainMLB1.RAWandcommentonthesizeoftheestimatedsalarydifferential.(ii)Stateandtestthenullhypothesisthatthereisnodifferenceinaveragesalaryacrosspositions,onceotherfactorshavebeencontrolledfor.(iii)Aretheresultsfromparts(i)and(ii)consistent?Ifnot,explainwhatishappening.7.12UsethedatainGPA2.RAWforthisexercise.(i)Considertheequation2colgpa01hsize2hsize3hsperc4sat5female6athleteu,wherecolgpaiscumulativecollegegradepointaverage,hsizeissizeofhighschoolgraduatingclass,inhundreds,hspercisacademicpercentileingraduatingclass,satiscombinedSATscore,femaleisabinarygen-dervariable,andathleteisabinaryvariable,whichisoneforstudent-athletes.Whatareyourexpectationsforthecoefficientsinthisequation?Whichonesareyouunsureabout?245\nd7/14/995:55PMPage246Part1RegressionAnalysiswithCross-SectionalData(ii)Estimatetheequationinpart(i)andreporttheresultsintheusualform.WhatistheestimatedGPAdifferentialbetweenathletesandnonath-letes?Isitstatisticallysignificant?(iii)Dropsatfromthemodelandreestimatetheequation.Nowwhatistheestimatedeffectofbeinganathlete?Discusswhytheestimateisdiffer-entthanthatobtainedinpart(ii).(iv)Inthemodelfrompart(i),allowtheeffectofbeinganathletetodifferbygenderandtestthenullhypothesisthatthereisnoceterisparibusdifferencebetweenwomenathletesandwomennonathletes.(v)Doestheeffectofsatoncolgpadifferbygender?Justifyyouranswer.7.13InProblem4.2,weaddedthereturnonthefirm’sstock,ros,toamodelexplain-ingCEOsalary;rosturnedouttobeinsignificant.Now,defineadummyvariable,ros-neg,whichisequaltooneifros0andequaltozeroifros0.UseCEOSAL1.RAWtoestimatethemodellog(salary)01log(sales)2roe3rosnegu.Discusstheinterpretationandstatisticalsignificanceofˆ3.7.14UsethedatainSLEEP75.RAWforthisexercise.Theequationofinterestis2sleep01totwrk2educ3age4age5yngkidu.(i)Estimatethisequationseparatelyformenandwomenandreporttheresultsintheusualform.Aretherenotabledifferencesinthetwoesti-matedequations?(ii)ComputetheChowtestforequalityoftheparametersinthesleepequa-tionformenandwomen.Usetheformofthetestthataddsmaleandtheinteractiontermsmaletotwrk,…,maleyngkidandusesthefullsetofobservations.Whataretherelevantdfforthetest?Shouldyourejectthenullatthe5%level?(iii)Nowallowforadifferentinterceptformalesandfemalesanddeterminewhethertheinteractiontermsinvolvingmalearejointlysignificant.(iv)Giventheresultsfromparts(ii)and(iii),whatwouldbeyourfinalmodel?7.15UsethedatainWAGE1.RAWforthisexercise.(i)Useequation(7.18)toestimatethegenderdifferentialwheneduc12.5.Comparethiswiththeestimateddifferentialwheneduc0.(ii)Runtheregressionusedtoobtain(7.18),butwithfemale(educ12.5)replacingfemaleeduc.Howdoyouintepretthecoefficientonfemalenow?(iii)Isthecoefficientonfemaleinpart(ii)statisticallysignificant?Comparethiswith(7.18)andcomment.7.16UsethedatainLOANAPP.RAWforthisexercise.Thebinaryvariabletobeexplainedisapprove,whichisequaltooneifamortgageloantoanindividualwasapproved.Thekeyexplanatoryvariableiswhite,adummyvariableequaltooneiftheapplicantwaswhite.TheotherapplicantsinthedatasetareblackandHispanic.246\nd7/14/995:55PMPage247Chapter7MultipleRegressionAnalysisWithQualitativeInformation:Binary(orDummy)VariablesTotestfordiscriminationinthemortgageloanmarket,alinearprobabilitymodelcanbeused:approve01whiteotherfactors.(i)Ifthereisdiscriminationagainstminorities,andtheappropriatefactorshavebeencontrolledfor,whatisthesignof1?(ii)Regressapproveonwhiteandreporttheresultsintheusualform.Interpretthecoefficientonwhite.Isitstatisticallysignificant?Isitprac-ticallylarge?(iii)Ascontrols,addthevariableshrat,obrat,loanprc,unem,male,married,dep,sch,cosign,chist,pubrec,mortlat1,mortlat2,andvr.Whathappenstothecoefficientonwhite?Istherestillevidenceofdis-criminationagainstnonwhites?(iv)Nowallowtheeffectofracetointeractwiththevariablemeasuringotherobligationsasapercentofincome(obrat).Istheinteractiontermsignificant?(v)Usingthemodelfrompart(iv),whatistheeffectofbeingwhiteontheprobabilityofapprovalwhenobrat32,whichisroughlythemeanvalueinthesample?Obtaina95%confidenceintervalforthiseffect.247\n\nd7/14/996:18PMPage248ChapterEightHeteroskedasticityhehomoskedasticityassumption,introducedinChapter3formultipleregres-Tsion,statesthatthevarianceoftheunobservableerror,u,conditionalontheexplanatoryvariables,isconstant.Homoskedasticityfailswheneverthevarianceoftheunobservableschangesacrossdifferentsegmentsofthepopulation,whicharedeterminedbythedifferentvaluesoftheexplanatoryvariables.Forexample,inasav-ingsequation,heteroskedasticityispresentifthevarianceoftheunobservedfactorsaffectingsavingsincreaseswithincome.InChapters3and4,wesawthathomoskedasticityisneededtojustifytheusualttests,Ftests,andconfidenceintervalsforOLSestimationofthelinearregressionmodel,evenwithlargesamplesizes.Inthischapter,wediscusstheavailableremedieswhenhet-eroskedasticityoccurs,andwealsoshowhowtotestforitspresence.Webeginbybrieflyreviewingtheconsequencesofheteroskedasticityforordinaryleastsquaresestimation.8.1CONSEQUENCESOFHETEROSKEDASTICITYFOROLSConsideragainthemultiplelinearregressionmodel:y01x12x2…kxku.(8.1)InChapter3,weprovedunbiasednessoftheOLSestimatorsˆ0,ˆ1,ˆ2,…,ˆkunderthefirstfourGauss-Markovassumptions,MLR.1throughMLR.4.InChapter5,weshowedthatthesamefourassumptionsimplyconsistencyofOLS.Thehomoskedasticityassump-2tionMLR.5,statedintermsoftheerrorvarianceasVar(ux1,x2,…,xk),playednoroleinshowingwhetherOLSwasunbiasedorconsistent.ItisimportanttorememberthatheteroskedasticitydoesnotcausebiasorinconsistencyintheOLSestimatorsofthej,whereassomethinglikeomittinganimportantvariablewouldhavethiseffect.Ifheteroskedasticitydoesnotcausebiasorinconsistency,whydidweintroduceitasoneoftheGauss-Markovassumptions?RecallfromChapter3thattheestimatorsofthevariances,Var(ˆj),arebiasedwithoutthehomoskedasticityassumption.SincetheOLSstandarderrorsarebaseddirectlyonthesevariances,theyarenolongervalidforconstructingconfidenceintervalsandtstatistics.TheusualOLStstatisticsdonothavetdistributionsinthepresenceofheteroskedasticity,andtheproblemisnotresolvedby248\nd7/14/996:18PMPage249Chapter8Heteroskedasticityusinglargesamplesizes.Similarly,FstatisticsarenolongerFdistributed,andtheLMstatisticnolongerhasanasymptoticchi-squaredistribution.Insummary,thestatisticsweusedtotesthypothesesundertheGauss-Markovassumptionsarenotvalidinthepresenceofheteroskedasticity.WealsoknowthattheGauss-Markovtheorem,whichsaysthatOLSisbestlinearunbiased,reliescruciallyonthehomoskedasticityassumption.IfVar(ux)isnotcon-stant,OLSisnolongerBLUE.Inaddition,OLSisnolongerasymptoticallyefficientintheclassofestimatorsdescribedinTheorem5.3.AswewillseeinSection8.4,itispossibletofindestimatorsthataremoreefficientthanOLSinthepresenceofhet-eroskedasticity(althoughitrequiresknowingtheformoftheheteroskedasticity).Withrelativelylargesamplesizes,itmightnotbesoimportanttoobtainanefficientestima-tor.Inthenextsection,weshowhowtheusualOLSteststatisticscanbemodifiedsothattheyarevalid,atleastasymptotically.8.2HETEROSKEDASTICITY-ROBUSTINFERENCEAFTEROLSESTIMATIONSincetestinghypothesesissuchanimportantcomponentofanyeconometricanalysisandtheusualOLSinferenceisgenerallyfaultyinthepresenceofheteroskedasticity,wemustdecideifweshouldentirelyabandonOLS.Fortunately,OLSisstilluseful.Inthelasttwodecades,econometricianshavelearnedhowtoadjuststandarderrors,t,F,andLMstatisticssothattheyarevalidinthepresenceofheteroskedasticityofunknownform.Thisisveryconvenientbecauseitmeanswecanreportnewstatisticsthatwork,regardlessofthekindofheteroskedasticitypresentinthepopulation.Themethodsinthissectionareknownasheteroskedasticity-robustproceduresbecausetheyarevalid—atleastinlargesamples—whetherornottheerrorshaveconstantvariance,andwedonotneedtoknowwhichisthecase.Webeginbysketchinghowthevariances,Var(ˆj),canbeestimatedinthepresenceofheteroskedasticity.Acarefulderivationofthetheoryiswell-beyondthescopeofthistext,buttheapplicationofheteroskedasticity-robustmethodsisveryeasynowbecausemanystatisticsandeconometricspackagescomputethesestatisticsasanoption.First,considerthemodelwithasingleindependentvariable,whereweincludeanisubscriptforemphasis:yi01xiui.WeassumethroughoutthatthefirstfourGauss-Markovassumptionshold.Iftheerrorscontainheteroskedasticity,then2Var(uixi)i,2whereweputanisubscriptontoindicatethatthevarianceoftheerrordependsupontheparticularvalueofxi.WritetheOLSestimatorasn(xix¯)uii1ˆ11n.2(xix¯)i1249\nd7/14/996:18PMPage250Part1RegressionAnalysiswithCross-SectionalDataUnderAssumptionsMLR.1throughMLR.4(thatis,withoutthehomoskedasticityassumption),andconditioningonthevaluesxiinthesample,wecanusethesameargu-mentsfromChapter2toshowthatn22(xix¯)ii1Var(ˆ1),(8.2)2SSTxn222whereSSTx(xix¯)isthetotalsumofsquaresofthexi.Wheniforalli,i12thisformulareducestotheusualform,/SSTx.Equation(8.2)explicitlyshowsthat,forthesimpleregressioncase,thevarianceformuladerivedunderhomoskedasticityisnolongervalidwhenheteroskedasticityispresent.Sincethestandarderrorofˆ1isbaseddirectlyonestimatingVar(ˆ1),weneedawaytoestimateequation(8.2)whenheteroskedasticityispresent.White(1980)showedhowthiscanbedone.LetuˆidenotetheOLSresidualsfromtheinitialregressionofyonx.ThenavalidestimatorofVar(ˆ1),forheteroskedasticityofanyform(includinghomoskedasticity),isn22(xix¯)uˆii1,(8.3)2SSTxwhichiseasilycomputedfromthedataaftertheOLSregression.Inwhatsenseis(8.3)avalidestimatorofVar(ˆ1)?Thisisprettysubtle.Briefly,itcanbeshownthatwhenequation(8.3)ismultipliedbythesamplesizen,itconverges2222inprobabilitytoE[(xix)ui]/(x),whichistheprobabilitylimitofntimes(8.2).Ultimately,thisiswhatisnecessaryforjustifyingtheuseofstandarderrorstoconstructconfidenceintervalsandtstatistics.Thelawoflargenumbersandthecentrallimitthe-oremplaykeyrolesinestablishingtheseconvergences.YoucanrefertoWhite’sorigi-nalpaperfordetails,butthatpaperisquitetechnical.SeealsoWooldridge(1999,Chapter4).Asimilarformulaworksinthegeneralmultipleregressionmodely01x1…kxku.ItcanbeshownthatavalidestimatorofVar(ˆj),underAssumptionsMLR.1throughMLR.4,isn22rˆijuˆii1Varˆ(ˆj),(8.4)2SSTjthwhererˆijdenotestheiresidualfromregressingxjonallotherindependentvariables,andSSRjisthesumofsquaredresidualsfromthisregression(seeSection3.2forthepartiallingoutarepresentationoftheOLSestimates).Thesquarerootofthequantity250\nd7/14/996:18PMPage251Chapter8Heteroskedasticityin(8.4)iscalledtheheteroskedasticity-robuststandarderrorforˆj.Ineconometrics,theserobuststandarderrorsareusuallyattributedtoWhite(1980).Earlierworksinsta-tistics,notablythosebyEicker(1967)andHuber(1967),pointedtothepossibilityofobtainingsuchrobuststandarderrors.Inappliedwork,thesearesometimescalledWhite,Huber,orEickerstandarderrors(orsomehyphenatedcombinationofthesenames).Wewilljustrefertothemasheteroskedasticity-robuststandarderrors,orevenjustrobuststandarderrorswhenthecontextisclear.Sometimes,asadegreeoffreedomcorrection,(8.4)ismultipliedbyn/(nk1)beforetakingthesquareroot.Thereasoningforthisadjustmentisthat,ifthesquared2OLSresidualsuˆiwerethesameforallobservationsi—thestrongestpossibleformofhomoskedasticityinasample—wewouldgettheusualOLSstandarderrors.Othermodificationsof(8.4)arestudiedinMacKinnonandWhite(1985).Sinceallformshaveonlyasymptoticjustificationandtheyareasymptoticallyequivalent,noformisuniformlypreferredaboveallothers.Typically,weusewhateverformiscomputedbytheregressionpackageathand.Onceheteroskedasticity-robuststandarderrorsareobtained,itissimpletoconstructaheteroskedasticity-robusttstatistic.Recallthatthegeneralformofthetstatisticisestimatehypothesizedvaluet.(8.5)standarderrorSincewearestillusingtheOLSestimatesandwehavechosenthehypothesizedvalueaheadoftime,theonlydifferencebetweentheusualOLStstatisticandtheheteroskedasticity-robusttstatisticisinhowthestandarderroriscomputed.EXAMPLE8.1(LogWageEquationwithHeteroskedasticity-RobustStandardErrors)WeestimatethemodelinExample7.6,butwereporttheheteroskedasticity-robuststan-darderrorsalongwiththeusualOLSstandarderrors.Someoftheestimatesarereportedtomoredigitssothatwecancomparetheusualstandarderrorswiththeheteroskedasticity-robuststandarderrors:logˆ(wage)(.321)(.213)marrmale(.198)marrfem(.110)singfemlogˆ(wage)(.100)(.055)marrmale(.058)marrfem(.056)singfemlogˆ(wage)[.109][.057]marrmale[.058]marrfem[.057]singfem2(.0789)educ(.0268)exper(.00054)exper2(.0067)educ(.0055)exper(.00011)exper(8.6)2[.0074]educ[.0051]exper[.00011]exper2(.0291)tenure(.00053)tenure2(.0068)tenure(.00023)tenure2[.0069]tenure[.00024]tenure2n526,R.461.251\nd7/14/996:18PMPage252Part1RegressionAnalysiswithCross-SectionalDataTheusualOLSstandarderrorsareinparentheses,(),belowthecorrespondingOLSesti-mate,andtheheteroskedasticity-robuststandarderrorsareinbrackets,[].Thenumbersinbracketsaretheonlynewthings,sincetheequationisstillestimatedbyOLS.Severalthingsareapparentfromequation(8.6).First,inthisparticularapplication,anyvariablethatwasstatisticallysignficantusingtheusualtstatisticisstillstatisticallysignifi-cantusingtheheteroskedasticity-robusttstatistic.Thisisbecausethetwosetsofstandarderrorsarenotverydifferent.(Theassociatedp-valueswilldifferslightlybecausetherobusttstatisticsarenotidenticaltotheusual,nonrobust,tstatistics.)Thelargestrelativechangeinstandarderrorsisforthecoefficientoneduc:theusualstandarderroris.0067,andtherobuststandarderroris.0074.Still,therobuststandarderrorimpliesarobusttstatisticabove10.Equation(8.6)alsoshowsthattherobuststandarderrorscanbeeitherlargerorsmallerthantheusualstandarderrors.Forexample,therobuststandarderroronexperis.0051,whereastheusualstandarderroris.0055.Wedonotknowwhichwillbelargeraheadoftime.Asanempiricalmatter,therobuststandarderrorsareoftenfoundtobelargerthantheusualstandarderrors.Beforeleavingthisexample,wemustemphasizethatwedonotknow,atthispoint,whetherheteroskedasticityisevenpresentinthepopulationmodelunderlyingequation(8.6).Allwehavedoneisreport,alongwiththeusualstandarderrors,thosethatarevalid(asymptotically)whetherornotheteroskedasticityispresent.Wecanseethatnoimportantconclusionsareoverturnedbyusingtherobuststandarderrorsinthisexample.Thisoftenhappensinappliedwork,butinothercasesthedifferencesbetweentheusualandrobuststandarderrorsaremuchlarger.Asanexampleofwherethedifferencesaresubstantial,seeProblem8.7.Atthispoint,youmaybeaskingthefollowingquestion:Iftheheteroskedasticity-robuststandarderrorsarevalidmoreoftenthantheusualOLSstandarderrors,whydowebotherwiththeusualstandarderrorsatall?Thisisavalidquestion.Onereasontheyarestillusedincross-sectionalworkisthat,ifthehomoskedasticityassumptionholdsandtheerrorsarenormallydistributed,thentheusualtstatisticshaveexacttdistribu-tions,regardlessofthesamplesize(seeChapter4).Therobuststandarderrorsandrobusttstatisticsarejustifiedonlyasthesamplesizebecomeslarge.Withsmallsam-plesizes,therobusttstatisticscanhavedistributionsthatarenotveryclosetothetdis-tribution,whichwouldcouldthrowoffourinference.Inlargesamplesizes,wecanmakeacaseforalwaysreportingonlytheheteroskedasticity-robuststandarderrorsincross-sectionalapplications,andthisprac-ticeisbeingfollowedmoreandmoreinappliedwork.Itisalsocommontoreportbothstandarderrors,asinequation(8.6),sothatareadercandeterminewhetheranycon-clusionsaresensitivetothestandarderrorinuse.ItisalsopossibletoobtainFandLMstatisticsthatarerobusttoheteroskedastic-ityofanunknown,arbitraryform.Theheteroskedasticity-robustFstatistic(orasimpletransformationofit)isalsocalledaheteroskedasticity-robustWaldstatistic.Ageneraltreatmentofthisstatisticisbeyondthescopeofthistext.Nevertheless,sincemanystatisticspackagesnowcomputetheseroutinely,itisusefultoknowthat252\nd7/14/996:18PMPage253Chapter8Heteroskedasticityheteroskedasticity-robustFandLMstatisticsareavailable.[SeeWooldridge(1999)fordetails.]EXAMPLE8.2(Heteroskedasticity-RobustFStatistic)UsingthedataforthespringsemesterinGPA3.RAW,weestimatethefollowingequation:cuˆmgpa(1.47)(.00114)sat(.00857)hsperc(.00250)tothrscuˆmgpa(0.23)(.00018)sat(.00124)hsperc(.00073)tothrscuˆmgpa[0.22][.00019]sat[.00140]hsperc[.00073]tothrs(.303)female(.128)black(.059)white(8.7)(.059)female(.147)black(.141)white[.059]female[.118]black[.110]white22n366,R.4006,R¯.3905.Again,thedifferencesbetweentheusualstandarderrorsandtheheteroskedasticity-robuststandarderrorsarenotverybig,anduseoftherobusttstatisticsdoesnotchangethesta-tisticalsignificanceofanyindependentvariable.Jointsignificancetestsarenotmuchaffectedeither.Supposewewishtotestthenullhypothesisthat,aftertheotherfactorsarecontrolledfor,therearenodifferencesincumgpabyrace.ThisisstatedasH0:black0,white0.TheusualFstatisticiseasilyobtained,oncewehavetheR-squaredfromtherestrictedmodel;thisturnsouttobe.3983.TheFstatisticisthen[(.4006.3983)/(1.4006)](359/2).69.Ifheteroskedasticityispresent,thisversionofthetestisinvalid.Theheteroskedasticity-robustversionhasnosimpleform,butitcanbecomputedusingcer-tainstatisticalpackages.Thevalueoftheheteroskedasticity-robustFstatisticturnsouttobe.75,whichdiffersonlyslightlyfromthenonrobustversion.Thep-valuefortherobusttestis.474,whichisnotclosetostandardsignificancelevels.Wefailtorejectthenullhypothesisusingeithertest.ComputingHeteroskedasticity-RobustLMTestsNotallregressionpackagescomputeFstatisticsthatarerobusttoheteroskedasticity.Therefore,itissometimesconvenienttohaveawayofobtainingatestofmultipleexclusionrestrictionsthatisrobusttohet-eroskedasticityanddoesnotrequireapar-QUESTION8.1ticularkindofeconometricsoftware.ItEvaluatethefollowingstatement:Theheteroskedasticity-robustturnsoutthataheteroskedasticity-robuststandarderrorsarealwaysbiggerthantheusualstandarderrors.LMstatisticiseasilyobtainedusingvirtu-allyanyregressionpackage.ToillustratecomputationoftherobustLMstatistic,considerthemodely01x12x23x34x45x5u,253\nd7/14/996:18PMPage254Part1RegressionAnalysiswithCross-SectionalDataandsupposewewouldliketotestH0:40,50.ToobtaintheusualLMstatistic,wewouldfirstestimatetherestrictedmodel(thatis,themodelwithoutx4andx5)toobtaintheresiduals,u˜.Then,wewouldregressu˜onalloftheindependentvariablesand22theLMnRu˜,whereRu˜istheusualR-squaredfromthisregression.Obtainingaversionthatisrobusttoheteroskedasticityrequiresmorework.OnewaytocomputethestatisticrequiresonlyOLSregressions.Weneedtheresiduals,sayr˜1,fromtheregressionofx4onx1,x2,x3.Also,weneedtheresiduals,sayr˜2,fromtheregressionofx5onx1,x2,x3.Thus,weregresseachoftheindependentvariablesexcludedunderthenullonalloftheincludedindependentvariables.Wekeeptheresid-ualseachtime.Thefinalstepappearsodd,butitis,afterall,justacomputationaldevice.Runtheregressionof1onr˜1u˜,r˜2u˜,(8.8)withoutanintercept.Yes,weactuallydefineadependentvariableequaltothevalueoneforallobservations.Weregressthisontotheproductsr˜1u˜andr˜2u˜.TherobustLMsta-tisticturnsouttobenSSR1,whereSSR1isjusttheusualsumofsquaredresidualsfromregression(8.8).Thereasonthisworksissomewhattechnical.Basically,thisisdoingfortheLMtestwhattherobuststandarderrorsdoforthettest.[SeeWooldridge(1991b)orDavidsonandMacKinnon(1993)foramoredetaileddiscussion.]Wenowsummarizethecomputationoftheheteroskedasticity-robustLMstatisticinthegeneralcase.AHETEROSKEDASTICITY-ROBUSTLMSTATISTIC:1.Obtaintheresidualsu˜fromtherestrictedmodel.2.Regresseachoftheindependentvariablesexcludedunderthenullonalloftheincludedindependentvariables;ifthereareqexcludedvariables,thisleadstoqsetsofresiduals(r˜1,r˜2,…,r˜q).3.Findtheproductsbetweeneachr˜jandu˜(forallobservations).4.Runtheregressionof1onr˜1u˜,r˜2u˜,…,r˜qu˜,withoutanintercept.Theheteroskedasticity-robustLMstatisticisnSSR1,whereSSR1isjusttheusualsumofsquaredresidualsfromthisfinalregression.UnderH0,LMisdistributed2approximatelyasq.OncetherobustLMstatisticisobtained,therejectionruleandcomputationofp-valuesisthesameasfortheusualLMstatisticinSection5.2.EXAMPLE8.3(Heteroskedasticity-RobustLMStatistic)WeusethedatainCRIME1.RAWtotestwhethertheaveragesentencelengthservedforpastconvictionsaffectsthenumberofarrestsinthecurrentyear(1986).Theestimatedmodelis254\nd7/14/996:18PMPage255Chapter8Heteroskedasticity2(narr86ˆ)(.567)(.136)pcnv(.0178)avgsen(.00052)avgsen2(narr86ˆ)(.036)(.040)pcnv(.0097)avgsen(.00030)avgsen2(narr86ˆ)[.040][.034]pcnv[.0101]avgsen[.00021]avgsen(.0394)ptime86(.0505)qemp86(.00148)inc86(.0087)ptime86(.0144)qemp86(.00034)inc86(8.9)[.0062]ptime86[.0142]qemp86[.00023]inc86(.325)black(.193)hispan(.045)black(.040)hispan[.058]black[.040]hispan2n2,725,R.0728.Inthisexample,therearemoresubstantialdifferencesbetweensomeoftheusualstandard2errorsandtherobuststandarderrors.Forexample,theusualtstatisticonavgsenisabout21.73,whiletherobusttstatisticisabout2.48.Thus,avgsenismoresignificantusingtherobuststandarderror.Theeffectofavgsenonnarr86issomewhatdifficulttoreconcile.Sincetherelationshipisquadratic,wecanfigureoutwhereavgsenhasapositiveeffectonnarr86andwheretheeffectbecomesnegative.Theturningpointis.0178/[2(.00052)]17.12;recallthatthisismeasuredinmonths.Literally,thismeansthatnarr86ispositivelyrelatedtoavgsenwhenavgsenislessthan17months;thenavgsenhastheexpecteddeterrenteffectafter17months.Toseewhetheraveragesentencelengthhasastatisticallysignificanteffectonnarr86,wemusttestthejointhypothesisH0:avgsen0,avgsen20.UsingtheusualLMstatistic(seeSection5.2),weobtainLM3.54;inachi-squaredistributionwithtwodf,thisyieldsap-value.170.Thus,wedonotrejectH0ateventhe15%level.Theheteroskedasticity-robustLMstatisticisLM4.00(roundingtotwodecimalplaces),withap-value.135.ThisisstillnotverystrongevidenceagainstH0;avgsendoesnotappeartohaveastrongeffectonnarr86.[Incidentally,whenavgsenappearsalonein(8.9),thatis,withoutthequa-draticterm,itsusualtstatisticis.658,anditsrobusttstatisticis.592.]8.3TESTINGFORHETEROSKEDASTICITYTheheteroskedasticity-robuststandarderrorsprovideasimplemethodforcomputingtstatisticsthatareasymptoticallytdistributedwhetherornotheteroskedasticityispres-ent.Wehavealsoseenthatheteroskedasticity-robustFandLMstatisticsareavailable.Implementingthesetestsdoesnotrequireknowingwhetherornotheteroskedasticityispresent.Nevertheless,therearestillsomegoodreasonsforhavingsimpleteststhatcandetectitspresence.First,aswementionedintheprevioussection,theusualtstatisticshaveexacttdistributionsundertheclassicallinearmodelassumptions.Forthisreason,manyeconomistsstillprefertoseetheusualOLSstandarderrorsandteststatisticsreported,unlessthereisevidenceofheteroskedasticity.Second,ifheteroskedasticityispresent,theOLSestimatorisnolongerthebestlinearunbiasedestimator.AswewillseeinSection8.4,itispossibletoobtainabetterestimatorthanOLSwhentheformofheteroskedasticityisknown.255\nd7/14/996:18PMPage256Part1RegressionAnalysiswithCross-SectionalDataManytestsforheteroskedasticityhavebeensuggestedovertheyears.Someofthem,whilehavingtheabilitytodetectheteroskedasticity,donotdirectlytesttheassumptionthatthevarianceoftheerrordoesnotdependupontheindependentvari-ables.Wewillrestrictourselvestomoremoderntests,whichdetectthekindofhet-eroskedasticitythatinvalidatestheusualOLSstatistics.Thisalsohasthebenefitofputtingalltestsinthesameframework.Asusual,westartwiththelinearmodely01x12x2…kxku,(8.10)whereAssumptionsMLR.1throughMLR.4aremaintainedinthissection.Inparticu-lar,weassumethatE(ux1,x2,…,xk)0,sothatOLSisunbiasedandconsistent.WetakethenullhypothesistobethatAssumptionMLR.5istrue:2H0:Var(ux1,x2,…,xk).(8.11)Thatis,weassumethattheidealassumptionofhomoskedasticityholds,andwerequirethedatatotellusotherwise.Ifwecannotreject(8.11)atasufficientlysmallsignifi-cancelevel,weusuallyconcludethatheteroskedasticityisnotaproblem.However,rememberthatweneveracceptH0;wesimplyfailtorejectit.Becauseweareassumingthatuhasazeroconditionalexpectation,Var(ux)2E(ux),andsothenullhypothesisofhomoskedasticityisequivalentto222H0:E(ux1,x2,…,xk)E(u).Thisshowsthat,inordertotestforviolationofthehomoskedasticityassumption,we2wanttotestwhetheruisrelated(inexpectedvalue)tooneormoreoftheexplanatory2variables.IfH0isfalse,theexpectedvalueofu,giventheindependentvariables,canbeanyfunctionofthexj.Asimpleapproachistoassumealinearfunction:2u01x12x2…kxkv,(8.12)wherevisanerrortermwithmeanzerogiventhexj.Paycloseattentiontothedepen-dentvariableinthisequation:itisthesquareoftheerrorintheoriginalregressionequation,(8.10).ThenullhypothesisofhomoskedasticityisH0:12…k0.(8.13)Underthenullhypothesis,itisoftenreasonabletoassumethattheerrorin(8.12),v,isindependentofx1,x2,…,xk.Then,weknowfromSection5.2thateithertheForLM2statisticsfortheoverallsignificanceoftheindependentvariablesinexplainingucanbeusedtotest(8.13).Bothstatisticswouldhaveasymptoticjustification,eventhough222ucannotbenormallydistributed.(Forexample,ifuisnormallydistributed,thenu/22isdistributedas1.)Ifwecouldobservetheuinthesample,thenwecouldeasilycom-2putethisstatisticbyrunningtheOLSregressionofuonx1,x2,…,xk,usingallnobser-vations.256\nd7/14/996:18PMPage257Chapter8HeteroskedasticityAswehaveemphasizedbefore,weneverknowtheactualerrorsinthepopulationmodel,butwedohaveestimatesofthem:theOLSresidual,uˆi,isanestimateoftheerroruiforobservationi.Thus,wecanestimatetheequation2uˆ01x12x2…kxkerror(8.14)andcomputetheForLMstatisticsforthejointsignificanceofx1,…,xk.ItturnsoutthatusingtheOLSresidualsinplaceoftheerrorsdoesnotaffectthelargesampledistribu-tionoftheForLMstatistics,althoughshowingthisisprettycomplicated.TheFandLMstatisticsbothdependontheR-squaredfromregression(8.14);call2thisRuˆ2todistinguishitfromtheR-squaredinestimatingequation(8.10).Then,theFstatisticis2Ruˆ2/kF,(8.15)2(1Ruˆ2)/(nk1)wherekisthenumberofregressorsin(8.14);thisisthesamenumberofindependentvariablesin(8.10).Computing(8.15)byhandisrarelynecessary,sincemostregressionpackagesautomaticallycomputetheFstatisticforoverallsignificanceofaregression.ThisFstatistichas(approximately)anFk,nk1distributionunderthenullhypothesisofhomoskedasticity.TheLMstatisticforheteroskedasticityisjustthesamplesizetimestheR-squaredfrom(8.14):2LMnRuˆ2.(8.16)2Underthenullhypothesis,LMisdistributedasymptoticallyask.Thisisalsoveryeasytoobtainafterrunningregression(8.14).TheLMversionofthetestistypicallycalledtheBreusch-Pagantestforhet-eroskedasticity(BPtest).BreuschandPagan(1980)suggestedadifferentformofthetestthatassumestheerrorsarenormallydistributed.Koenker(1983)suggestedtheformoftheLMstatisticin(8.16),anditisgenerallypreferredduetoitsgreaterapplic-ability.WesummarizethestepsfortestingforheteroskedasticityusingtheBPtest:THEBREUSCH-PAGANTESTFORHETEROSKEDASTICITY.1.Estimatethemodel(8.10)byOLS,asusual.ObtainthesquaredOLSresiduals,2uˆ(oneforeachobservation).22.Runtheregressionin(8.14).KeeptheR-squaredfromthisregression,Ruˆ2.3.FormeithertheFstatisticortheLMstatisticandcomputethep-value(usingthe2Fk,nk1distributionintheformercaseandthekdistributioninthelattercase).Ifthep-valueissufficientlysmall,thatis,belowthechosensignificancelevel,thenwerejectthenullhypothesisofhomoskedasticity.IftheBPtestresultsinasmallenoughp-value,somecorrectivemeasureshouldbetaken.Onepossibilityistojustusetheheteroskedasticity-robuststandarderrorsand257\nd7/14/996:18PMPage258Part1RegressionAnalysiswithCross-SectionalDatateststatisticsdiscussedintheprevioussection.AnotherpossibilityisdiscussedinSection8.4.EXAMPLE8.4(HeteroskedasticityinHousingPriceEquations)WeusethedatainHPRICE1.RAWtotestforheteroskedasticityinasimplehousingpriceequation.Theestimatedequationusingthelevelsofallvariablesis(priˆce21.77)(.00207)lotsize(.123)sqrft(13.85)bdrmspriˆce(29.48)(.00064)lotsize(.013)sqrft0(9.01)bdrms(8.17)2n88,R.672.Thisequationtellsusnothingaboutwhethertheerrorinthepopulationmodelishet-eroskedastic.WeneedtoregressthesquaredOLSresidualsontheindependentvariables.22TheR-squaredfromtheregressionofuˆonlotsize,sqrft,andbdrmsisRuˆ2.1601.Withn88andk3,thisproducesanFstatisticforsignificanceoftheindependentvariablesofF[.1601/(1.1601)](84/3)5.34.Theassociatedp-valueis.002,whichisstrongevidenceagainstthenull.TheLMstatisticis88(.1601)14.09;thisgivesap-value2.0028(usingthe3distribution),givingessentiallythesameconclusionastheFstatistic.Thismeansthattheusualstandarderrorsreportedin(8.17)arenotreliable.InChapter6,wementionedthatonebenefitofusingthelogarithmicfunctionalformforthedependentvariableisthatheteroskedasticityisoftenreduced.Inthecurrentappli-cation,letusputprice,lotsize,andsqrftinlogarithmicform,sothattheelasticitiesofprice,withrespecttolotsizeandsqrft,areconstant.Theestimatedequationislog(priˆce)(5.61)(.168)log(lotsize)(.700)log(sqrft)(.037)bdrmslog(priˆce)5(.65)(.038)log(lotsize)(.093)log(sqrft)(.028)bdrms(8.18)2n88,R.643.RegressingthesquaredOLSresidualsfromthisregressiononlog(lotsize),log(sqrft),and2bdrmsgivesRuˆ2.0480.Thus,F1.41(p-value.245),andLM4.22(p-value.239).Therefore,wefailtorejectthenullhypothesisofhomoskedasticityinthemodelwiththelogarithmicfunctionalforms.Theoccurrenceoflessheteroskedasticitywiththedepen-dentvariableinlogarithmicformhasbeennoticedinmanyempiricalapplications.IfwesuspectthatheteroskedasticityQUESTION8.2dependsonlyuponcertainindependentConsiderwageequation(7.11),whereyouthinkthatthecondi-variables,wecaneasilymodifythetionalvarianceoflog(wage)doesnotdependoneduc,exper,orBreusch-Pagantest:wesimplyregressuˆ2tenure.However,youareworriedthatthevarianceoflog(wage)dif-onwhateverindependentvariableswefersacrossthefourdemographicgroupsofmarriedmales,marriedchooseandcarryouttheappropriateForfemales,singlemales,andsinglefemales.Whatregressionwouldyouruntotestforheteroskedasticity?Whatarethedegreesoffree-LMtest.RememberthattheappropriatedomintheFtest?degreesoffreedomdependsuponthenum-258\nd7/14/996:18PMPage259Chapter8Heteroskedasticity2berofindependentvariablesintheregressionwithuˆasthedependentvariable;thenumberofindependentvariablesshowingupinequation(8.10)isirrelevant.Ifthesquaredresidualsareregressedononlyasingleindependentvariable,thetestforheteroskedasticityisjusttheusualtstatisticonthevariable.Asignificanttstatisticsuggeststhatheteroskedasticityisaproblem.TheWhiteTestforHeteroskedasticityInChapter5,weshowedthattheusualOLSstandarderrorsandteststatisticsareasymptoticallyvalid,providedalloftheGauss-Markovassumptionshold.Itturnsout2thatthehomoskedasticityassumption,Var(u1x1,…,xk),canbereplacedwiththe2weakerassumptionthatthesquarederror,u,isuncorrelatedwithalltheindependent2variables(xj),thesquaresoftheindependentvariables(xj),andallthecrossproducts(xjxhforjh).ThisobservationmotivatedWhite(1980)toproposeatestforhet-eroskedasticitythataddsthesquaresandcrossproductsofalloftheindependentvari-ablestoequation(8.14).ThetestisexplicitlyintendedtotestforformsofheteroskedasticitythatinvalidatetheusualOLSstandarderrorsandteststatistics.Whenthemodelcontainsk3independentvariables,theWhitetestisbasedonanestimationof2222uˆ01x12x23x34x15x26x3(8.19)7x1x28x1x39x2x3error.ComparedwiththeBreusch-Pagantest,thisequationhassixmoreregressors.TheWhitetestforheteroskedasticityistheLMstatisticfortestingthatallofthejinequa-tion(8.19)arezero,exceptfortheintercept.Thus,ninerestrictionsarebeingtestedinthiscase.WecanalsouseanFtestofthishypothesis;bothtestshaveasymptoticjusti-fication.Withonlythreeindependentvariablesintheoriginalmodel,equation(8.19)hasnineindependentvariables.Withsixindependentvariablesintheoriginalmodel,theWhiteregressionwouldgenerallyinvolve27regressors(unlesssomeareredundant).ThisabundanceofregressorsisaweaknessinthepureformoftheWhitetest:itusesmanydegreesoffreedomformodelswithjustamoderatenumberofindependentvari-ables.ItispossibletoobtainatestthatiseasiertoimplementthantheWhitetestandmoreconservingondegreesoffreedom.Tocreatethetest,recallthatthedifferencebetweentheWhiteandBreusch-Pagantestsisthattheformerincludesthesquaresandcrossproductsoftheindependentvariables.Wecanachievethesamethingbyusingfewerfunctionsoftheindependentvariables.OnesuggestionistousetheOLSfittedvaluesinatestforheteroskedasticity.Rememberthatthefittedvaluesaredefined,foreachobservationi,byyˆiˆ0ˆ1xi1ˆ2xi2…ˆkxik.Thesearejustlinearfunctionsoftheindependentvariables.Ifwesquarethefittedval-ues,wegetaparticularfunctionofallthesquaresandcrossproductsoftheindepen-dentvariables.Thissuggeststestingforheteroskedasticitybyestimatingtheequation259\nd7/14/996:18PMPage260Part1RegressionAnalysiswithCross-SectionalData22uˆ01yˆ2yˆerror,(8.20)whereyˆstandsforthefittedvalues.Itisimportantnottoconfuseyˆandyinthisequa-tion.Weusethefittedvaluesbecausetheyarefunctionsoftheindependentvariables(andtheestimatedparameters);usingyin(8.20)doesnotproduceavalidtestforhet-eroskedasticity.WecanusetheForLMstatisticforthenullhypothesisH0:10,20inequa-tion(8.20).Thisresultsintworestrictionsintestingthenullofhomoskedasticity,regardlessofthenumberofindependentvariablesintheoriginalmodel.Conservingondegreesoffreedominthiswayisoftenagoodidea,anditalsomakesthetesteasytoimplement.Sinceyˆisanestimateoftheexpectedvalueofy,giventhexj,using(8.20)totestforheteroskedasticityisusefulincaseswherethevarianceisthoughttochangewiththeleveloftheexpectedvalue,E(yx).Thetestfrom(8.20)canbeviewedasaspecialcaseoftheWhitetest,sinceequation(8.20)canbeshowntoimposerestrictionsontheparametersinequation(8.19).ASPECIALCASEOFTHEWHITETESTFORHETEROSKEDASTICITY:1.Estimatethemodel(8.10)byOLS,asusual.ObtaintheOLSresidualsuˆandthe2fittedvaluesyˆ.ComputethesquaredOLSresidualsuˆandthesquaredfittedval-2uesyˆ.2.Runtheregressioninequation(8.20).KeeptheR-squaredfromthisregression,2Ruˆ2.3.FormeithertheForLMstatisticandcomputethep-value(usingtheF2,n3dis-2tributionintheformercaseandthe2distributioninthelattercase).EXAMPLE8.5(SpecialFormoftheWhiteTestintheLogHousingPriceEquation)WeapplythespecialcaseoftheWhitetesttoequation(8.18),whereweusetheLMformofthestatistic.Theimportantthingtorememberisthatthechi-squaredistributionalways22hastwodf.Theregressionofuˆonlpriˆce,(lpriˆce),wherelpriˆcedenotesthefittedvalues2from(8.18),producesRuˆ2.0392;thus,LM88(.0392)3.45,andthep-value.178.ThisisstrongerevidenceofheteroskedasticitythanisprovidedbytheBreusch-Pagantest,butwestillfailtorejecthomoskedasticityateventhe15%level.Beforeleavingthissection,weshoulddiscussoneimportantcaveat.Wehaveinter-pretedarejectionusingoneoftheheteroskedasticitytestsasevidenceofheteroskedas-ticity.ThisisappropriateprovidedwemaintainAssumptionsMLR.1throughMLR.4.But,ifMLR.3isviolated—inparticular,ifthefunctionalformofE(yx)ismisspeci-fied—thenatestforheteroskedastcitycanrejectH0,evenifVar(yx)isconstant.Forexample,ifweomitoneormorequadratictermsinaregressionmodelorusethelevelmodelwhenweshouldusethelog,atestforheteroskedasticitycanbesignificant.This260\nd7/14/996:18PMPage261Chapter8Heteroskedasticityhasledsomeeconomiststoviewtestsforheteroskedasticityasgeneralmisspecificationtests.However,therearebetter,moredirecttestsforfunctionalformmisspecification,andwewillcoversomeoftheminSection9.1.Itisbettertouseexplicittestsforfunc-tionalformfirst,sincefunctionalformmisspecificationismoreimportantthanhet-eroskedasticity.Then,oncewearesatisfiedwiththefunctionalform,wecantestforheteroskedasticity.8.4WEIGHTEDLEASTSQUARESESTIMATIONIfheteroskedasticityisdetectedusingoneofthetestsinSection8.3,weknowfromSection8.2thatonepossibleresponseistouseheteroskedasticity-robuststatisticsafterestimationbyOLS.Beforethedevelopmentofheteroskedasticity-robuststatistics,theresponsetoafindingofheteroskedasticitywastomodelandestimateitsspecificform.Aswewillsee,thisleadstoamoreefficientestimatorthanOLS,anditproducestandFstatisticsthathavetandFdistributions.Whilethisseemsattractive,itactuallyrequiresmoreworkonourpartbecausewemustbeveryspecificaboutthenatureofanyheteroskedasticity.TheHeteroskedasticityIsKnownuptoaMultiplicativeConstantLetxdenotealltheexplanatoryvariablesinequation(8.10)andassumethat2Var(ux)h(x),(8.21)whereh(x)issomefunctionoftheexplanatoryvariablesthatdeterminesthehet-eroskedasticity.Sincevariancesmustbepositive,h(x)0forallpossiblevaluesoftheindependentvariables.Weassumeinthissubsectionthatthefunctionh(x)isknown.2Thepopulationparameterisunknown,butwewillbeabletoestimateitfromadatasample.2Forarandomdrawingfromthepopulation,wecanwriteiVar(uixi)22h(xi)hi,whereweagainusethenotationxitodenoteallindependentvariablesforobservationi,andhichangeswitheachobservationbecausetheindependentvari-ableschangeacrossobservations.Forexample,considerthesimplesavingsfunctionsavi01inciui(8.22)2Var(uiinci)inci.(8.23)Here,h(inc)inc:thevarianceoftheerrorisproportionaltothelevelofincome.Thismeansthat,asincomeincreases,thevariabilityinsavingsincreases.(If10,theexpectedvalueofsavingsalsoincreaseswithincome.)Becauseincisalwayspositive,thevarianceinequation(8.23)isalwaysguaranteedtobepositive.Thestandarddevi-ationofui,conditionaloninci,isinci.Howcanweusetheinformationinequation(8.21)toestimatethej?Essentially,wetaketheoriginalequation,261\nd7/14/996:18PMPage262Part1RegressionAnalysiswithCross-SectionalDatayi01xi12xi2…kxikui,(8.24)whichcontainsheteroskedasticerrors,andtransformitintoanequationthathashomoskedasticerrors(andsatisfiestheotherGauss-Markovassumptions).Sincehiisjustafunctionofxi,ui/hihasazeroexpectedvalueconditionalonxi.Further,since222Var(uixi)E(uixi)hi,thevarianceofui/hi(conditionalonxi)is:2222E(ui/hi)E(ui)/hi(hi)/hi,wherewehavesuppressedtheconditioningonxiforsimplicity.Wecandivideequation(8.24)byhitogetyi/hi0/hi1(xi1/hi)2(xi2/hi)…(8.25)k(xik/hi)(ui/hi)ory*i0xi*01x*i1…kx*iku*,i(8.26)wherex*i01/hiandtheotherstarredvariablesdenotethecorrespondingoriginalvariablesdividedbyhi.Equation(8.26)looksalittlepeculiar,buttheimportantthingtorememberisthatwederiveditsowecouldobtainestimatorsofthejthathavebetterefficiencyproper-tiesthanOLS.Theintercept0intheoriginalequation(8.24)isnowmultiplyingthevariablexi*01/hi.Eachslopeparameterinjmultipliesanewvariablethatrarelyhasausefulinterpretation.Thisshouldnotcauseproblemsifwerecallthat,forinter-pretingtheparametersandthemodel,wealwayswanttoreturntotheoriginalequation(8.24).Intheprecedingsavingsexample,thetransformedequationlookslikesavi/inci0(1/inci)1inciu*,iwhereweusethefactthatinci/inciinci.Nevertheless,1isthemarginalpropen-sitytosaveoutofincome,aninterpretationweobtainfromequation(8.22).Equation(8.26)islinearinitsparameters(soitsatisfiesMLR.1),andtherandomsamplingassumptionhasnotchanged.Further,u*hasazeromeanandaconstantvari-i2ance(),conditionalonx*.ThismeansthatiftheoriginalequationsatisfiesthefirstifourGauss-Markovassumptions,thenthetransformedequation(8.26)satisfiesallfiveGauss-Markovassumptions.Also,ifuihasanormaldistribution,thenu*hasanormali2distributionwithvariance.Therefore,thetransformedequationsatisfiestheclassi-callinearmodelassumptions(MLR.1throughMLR.6),iftheoriginalmodeldoesso,exceptforthehomoskedasticityassumption.SinceweknowthatOLShasappealingproperties(isBLUE,forexample)undertheGauss-Markovassumptions,thediscussioninthepreviousparagraphsuggestsestimat-ingtheparametersinequation(8.26)byordinaryleastsquares.Theseestimators,*,0*,…,1*,willbedifferentfromtheOLSestimatorsintheoriginalequation.Thek*jareexamplesofgeneralizedleastsquares(GLS)estimators.Inthiscase,theGLS262\nd7/14/996:18PMPage263Chapter8Heteroskedasticityestimatorsareusedtoaccountforheteroskedasticityintheerrors.WewillencounterotherGLSestimatorsinChapter12.Sinceequation(8.26)satisfiesalloftheidealassumptions,standarderrors,tstatis-tics,andFstatisticscanallbeobtainedfromregressionsusingthetransformedvari-ables.Thesumofsquaredresidualsfrom(8.26)dividedbythedegreesoffreedomis2anunbiasedestimatorof.Further,theGLSestimators,becausetheyarethebestlin-earunbiasedestimatorsofthej,arenecessarilymoreefficientthantheOLSestima-torsˆjobtainedfromtheuntransformedequation.Essentially,afterwehavetransformedthevariables,wesimplyusestandardOLSanalysis.Butwemustremem-bertointerprettheestimatesinlightoftheoriginalequation.TheR-squaredthatisobtainedfromestimating(8.26),whileusefulforcomputingFstatistics,isnotespeciallyinformativeasagoodness-of-fitmeasure:ittellsushowmuchvariationiny*isexplainedbythexj*,andthisisseldomverymeaningful.TheGLSestimatorsforcorrectingheteroskedasticityarecalledweightedleastsquares(WLS)estimators.Thisnamecomesfromthefactthatthe*minimizethejweightedsumofsquaredresiduals,whereeachsquaredresidualisweightedby1/hi.Theideaisthatlessweightisgiventoobservationswithahighererrorvariance;OLSgiveseachobservationthesameweightbecauseitisbestwhentheerrorvarianceisidenticalforallpartitionsofthepopulation.Mathematically,theWLSestimatorsarethevaluesofthebjthatmaken2(yib0b1xi1b2xi2…bkxik)/hi(8.27)i1assmallaspossible.Bringingthesquarerootof1/hiinsidethesquaredresidualshowsthattheweightedsumofsquaredresidualsisidenticaltothesumofsquaredresidualsinthetransformedvariables:n2(y*ib0x*i0b1xi*1b2x*i2…bkx*ik).i1ItfollowsthattheWLSestimatorsthatminimize(8.27)aresimplytheOLSestimatorsfrom(8.26).Aweightedleastsquaresestimatorcanbedefinedforanysetofpositiveweights.OLSisthespecialcasethatgivesequalweighttoallobservations.Theefficientproce-dure,GLS,weightseachsquaredresidualbytheinverseoftheconditionalvarianceofuigivenxi.Obtainingthetransformedvariablesinordertoperformweightedleastsquarescanbetedious,andthechanceofmakingmistakesisnontrivial.Fortunately,mostmodernregressionpackageshaveafeaturefordoingweightedleastsquares.Typically,alongwiththedependentandindependentvariablesintheoriginalmodel,wejustspecifytheweightingfunction.Inadditiontomakingmistakeslesslikely,thisforcesustointer-pretweightedleastsquaresestimatesintheoriginalmodel.Infact,wecanwriteouttheestimatedequationintheusualway.TheestimatesandstandarderrorswillbedifferentfromOLS,butthewayweinterpretthoseestimates,standarderrors,andteststatisticsisthesame.263\nd7/14/996:18PMPage264Part1RegressionAnalysiswithCross-SectionalDataEXAMPLE8.6(FamilySavingEquation)Table8.1containsestimatesofsavingfunctionsfromthedatasetSAVING.RAW(on100familiesfrom1970).Weestimatethesimpleregressionmodel(8.22)byOLSandbyweightedleastsquares,assuminginthelattercasethatthevarianceisgivenby(8.23).Wethenaddvariablesforfamilysize,ageofthehouseholdhead,yearsofeducationforthehouseholdhead,andadummyvariableindicatingwhetherthehouseholdheadisblack.Inthesimpleregressionmodel,theOLSestimateofthemarginalpropensitytosave(MPS)is.147,withatstatisticof2.53.(ThestandarderrorsinTable8.1forOLSarethenonrobuststandarderrors.Ifwereallythoughtheteroskedasticitywasaproblem,wewouldprobablycomputetheheteroskedasticity-robuststandarderrorsaswell;wewillnotdothathere.)TheWLSestimateoftheMPSissomewhathigher:.172,witht3.02.ThestandarderrorsoftheOLSandWLSestimatesareverysimilarforthiscoefficient.Theinterceptesti-matesareverydifferentforOLSandWLS,butthisshouldcausenoconcernsincethetsta-tisticsarebothverysmall.FindingfairlylargechangesincoefficientsthatareinsignificantisnotuncommonwhencomparingOLSandWLSestimates.TheR-squaredsincolumns(1)and(2)arenotcomparable.Table8.1DependentVariable:savIndependent(1)(2)(3)(4)VariablesOLSWLSOLSWLSinc.147.172.109.101(.058)(.057)(.071)(.077)size——67.666.87(222.96)(168.43)educ——151.82139.48(117.25)(100.54)age——.28621.75(50.031)(41.31)black——518.39137.28(1,308.06)(844.59)intercept124.84124.951,605.421,854.81(655.39)(480.86)(2,830.71)(2,351.80)Observations100100100100R-Squared.0621.0853.0828.1042264\nd7/14/996:18PMPage265Chapter8HeteroskedasticityAddingdemographicvariablesreducestheMPSwhetherOLSorWLSisused;thestan-darderrorsalsoincreasebyafairamount(duetomulticollinearitythatisinducedbyaddingtheseadditionalvariables).Itiseasytosee,usingeithertheOLSorWLSestimates,thatnoneoftheadditionalvariablesisindividuallysignificant.Aretheyjointlysignificant?TheFtestbasedontheOLSestimatesusestheR-squaredsfromcolumns(1)and(3).With94dfintheunrestrictedmodelandfourrestrictions,theFstatisticisF[(.0828.0621)/(1.0828)](94/4).53andp-value.715.TheFtest,usingtheWLSestimates,usestheR-squaredsfromcolumns(2)and(4):F.50andp-value.739.Thus,usingeitherOLSorWLS,thedemographicvariablesarejointlyinsignificant.Thissuggeststhatthesimpleregressionmodelrelatingsavingstoincomeissufficient.Whatshouldwechooseasourbestestimateofthemarginalpropensitytosave?Inthiscase,itdoesnotmattermuchwhetherweusetheOLSestimateof.147ortheWLSesti-mateof.172.Remember,botharejustestimatesfromarelativelysmallsample,andtheOLS95%confidenceintervalcontainstheWLSestimate,andviceversa.Inpractice,werarelyknowhowthevariancedependsonaparticularindependentvariableinasimpleform.Forexample,inthesavingsequationthatincludesalldemo-graphicvariables,howdoweknowthatthevarianceofsavdoesnotchangewithageoreducationlevels?Inmostapplications,weareunsureaboutVar(yx1,x2…,xk).QUESTION8.3ThereisonecasewheretheweightsUsingtheOLSresidualsobtainedfromtheOLSregressionreportedneededforWLSarisenaturallyfroman2incolumn(1)ofTable8.1,theregressionofuˆonincyieldsatsta-underlyingeconometricmodel.Thishap-tisticonincof.96.IsthereanyneedtouseweightedleastsquaresinExample8.6?penswhen,insteadofusingindividualleveldata,weonlyhaveaveragesofdataacrosssomegrouporgeographicregion.Forexample,supposeweareinterestedindeterminingtherelationshipbetweentheamountaworkercontributestohisorher401(k)pensionplanasafunctionoftheplangenerosity.Letidenoteaparticularfirmandletedenoteanemployeewithinthefirm.Asimplemodeliscontribi,e01earnsi,e2agei,e3mrateiui,e,(8.28)wherecontribi,eistheannualcontributionbyemployeeewhoworksforfirmi,earnsi,eisannualearningsforthisperson,andagei,eistheperson’sage.Thevariablemrateiistheamountthefirmputsintoanemployee’saccountforeverydollartheemployeecon-tributes.If(8.28)satisfiestheGauss-Markovassumptions,thenwecouldestimateit,givenasampleonindividualsacrossvariousemployers.Suppose,however,thatweonlyhaveaveragevaluesofcontributions,earnings,andagebyemployer.Inotherwords,indi-vidual-leveldataarenotavailable.Thus,letcontribidenoteaveragecontributionforpeopleatfirmi,andsimilarlyforearnsiandagei.Letmidenotethenumberofemploy-eesateachfirm;weassumethatthisisaknownquantity.Then,ifweaverageequation(8.28)acrossallemployeesatfirmi,weobtainthefirm-levelequationcontribi01earnsi2agei3mrateiui,(8.29)265\nd7/14/996:18PMPage266Part1RegressionAnalysiswithCross-SectionalDatami1whereu¯imiui,eistheaverageerroracrossallemployeesinfirmi.Ifwehavene1firmsinoursample,then(8.29)isjustastandardmultiplelinearregressionmodelthatcanbeestimatedbyOLS.Theestimatorsareunbiasediftheoriginalmodel(8.28)sat-isfiestheGauss-Markovassumptionsandtheindividualerrorsui,eareindependentofthefirm’ssize,mi(becausethentheexpectedvalueofu¯i,giventheexplanatoryvariablesin(8.29),iszero).Iftheequationattheindividuallevelsatisfiesthehomoskedasticityassumption,thenthefirm-levelequation(8.29)musthaveheteroskedasticity.Infact,ifVar(ui,e)22foralliande,thenVar(u¯i)/mi.Inotherwords,forlargerfirms,thevarianceoftheerrortermu¯idecreaseswithfirmsize.Inthiscase,hi1/mi,andsothemosteffi-cientprocedureisweightedleastsquares,withweightsequaltothenumberofemploy-eesatthefirm(1/himi).Thisensuresthatlargerfirmsreceivemoreweight.Thisgivesusanefficientwayofestimatingtheparametersintheindividual-levelmodelwhenweonlyhaveaveragesatthefirmlevel.Asimilarweightingariseswhenweareusingpercapitadataatthecity,county,state,orcountrylevel.Iftheindividual-levelequationsatisfiestheGauss-Markovassumptions,thentheerrorinthepercapitaequationhasavarianceproportionaltooneoverthesizeofthepopulation.Therefore,weightedleastsquareswithweightsequaltothepopulationisappropriate.Forexample,supposewehavecity-leveldataonpercapitabeerconsumption(inounces),thepercentageofpeopleinthepopulationover21yearsold,averageadulteducationlevels,averageincomelevels,andthecitypriceofbeer.Thenthecity-levelmodelbeerpc0+1perc212avgeduc2incpc2priceucanbeestimatedbyweightedleastsquares,withtheweightsbeingthecitypopu-lation.Theadvantageofweightingbyfirmsize,citypopulation,andsoonreliesontheunderlyingindividualequationbeinghomoskedastic.Ifheteroskedasticityexistsattheindividuallevel,thentheproperweightingdependsontheformoftheheteroskedastic-ity.Thisisonereasonwhymoreandmoreresearcherssimplycomputerobuststandarderrorsandteststatisticswhenestimatingmodelsusingpercapitadata.Analternativeistoweightbypopulationbuttoreporttheheteroskedasticity-robuststatisticsintheWLSestimation.Thisensuresthat,whiletheestimationisefficientiftheindividual-levelmodelsatisfiestheGauss-Markovassumptions,anyheteroskedasticityattheindividuallevelisaccountedforthroughrobustinference.TheHeteroskedasticityFunctionMustBeEstimated:FeasibleGLSIntheprevioussubsection,wesawsomeexamplesofwheretheheteroskedasticityisknownuptoamultiplicativeform.Inmostcases,theexactformofheteroskedasticityisnotobvious.Inotherwords,itisdifficulttofindthefunctionh(xi)oftheprevioussection.Nevertheless,inmanycaseswecanmodelthefunctionhandusethedatatoestimatetheunknownparametersinthismodel.Thisresultsinanestimateofeachhi,denotedashˆi.UsinghˆiinsteadofhiintheGLStransformationyieldsanestimatorcalled266\nd7/14/996:18PMPage267Chapter8HeteroskedasticitythefeasibleGLS(FGLS)estimator.FeasibleGLSissometimescalledestimatedGLS,orEGLS.Therearemanywaystomodelheteroskedasticity,butwewillstudyoneparticular,fairlyflexibleapproach.Assumethat2Var(ux)exp(01x12x2…kxk),(8.30)wherex1,x2,…,xkaretheindependentvariablesappearingintheregressionmodel[seeequation(8.1)],andthejareunknownparameters.Otherfunctionsofthexjcanappear,butwewillfocusprimarilyon(8.30).Inthenotationoftheprevioussubsection,h(x)exp(01x12x2…kxk).Youmaywonderwhywehaveusedtheexponentialfunctionin(8.30).Afterall,whentestingforheteroskedasticityusingtheBreusch-Pagantest,weassumedthathet-eroskedasticitywasalinearfunctionofthexj.Linearalternativessuchas(8.12)arefinewhentestingforheteroskedasticity,buttheycanbeproblematicwhencorrectingforheteroskedasticityusingweightedleastsquares.Wehaveencounteredthereasonforthisproblembefore:linearmodelsdonotensurethatpredictedvaluesarepositive,andourestimatedvariancesmustbepositiveinordertoperformWLS.Iftheparametersjwereknown,thenwewouldjustapplyWLS,asintheprevioussubsection.Thisisnotveryrealistic.Itisbettertousethedatatoestimatethesepara-meters,andthentousetheseestimatestoconstructweights.Howcanweestimatethej?Essentially,wewilltransformthisequationintoalinearformthat,withslightmod-ification,canbeestimatedbyOLS.Underassumption(8.30),wecanwrite22uexp(01x12x2…kxk)v,wherevhasameanequaltounity,conditionalonx(x1,x2,…,xk).Ifweassumethatvisactuallyindependentofx,wecanwrite2log(u)01x12x2…kxke,(8.31)whereehasazeromeanandisindependentofx;theinterceptinthisequationisdif-ferentfrom0,butthisisnotimportant.Thedependentvariableisthelogofthesquarederror.Since(8.31)satisfiestheGauss-Markovassumptions,wecangetunbiasedesti-matorsofthejbyusingOLS.Asusual,wemustreplacetheunobserveduwiththeOLSresiduals.Therefore,weruntheregressionof2log(uˆ)onx1,x2,…,xk.(8.32)Actually,whatweneedfromthisregressionarethefittedvalues;callthesegˆi.Then,theestimatesofhiaresimplyhˆiexp(gˆi).(8.33)WenowuseWLSwithweights1/hˆi.Wesummarizethesteps.267\nd7/14/996:18PMPage268Part1RegressionAnalysiswithCross-SectionalDataAFEASIBLEGLSPROCEDURETOCORRECTFORHETEROSKEDASTICITY:1.Runtheregressionofyonx1,x2,...,xkandobtaintheresiduals,uˆ.22.Createlog(uˆ)byfirstsquaringtheOLSresidualsandthentakingthenaturallog.3.Runtheregressioninequation(8.32)andobtainthefittedvalues,gˆ.4.Exponentiatethefittedvaluesfrom(8.32):hˆexp(gˆ).5.Estimatetheequationy01x1…kxkubyWLS,usingweights1/hˆ.IfwecouldusehiratherthanhˆiintheWLSprocedure,weknowthatourestimatorswouldbeunbiased;infact,theywouldbethebestlinearunbiasedestimators,assum-ingthatwehaveproperlymodeledtheheteroskedasticity.HavingtoestimatehiusingthesamedatameansthattheFGLSestimatorisnolongerunbiased(soitcannotbeBLUE,either).Nevertheless,theFGLSestimatorisconsistentandasymptoticallymoreefficientthanOLS.Thisisdifficulttoshowbecauseofestimationofthevariancepara-meters.Butifweignorethis—asitturnsoutwemay—theproofissimilartoshowingthatOLSisefficientintheclassofestimatorsinTheorem5.3.Atanyrate,forlargesamplesizes,FGLSisanattractivealternativetoOLSwhenthereisevidenceofhet-eroskedasticitythatinflatesthestandarderrorsoftheOLSestimates.WemustrememberthattheFGLSestimatorsareestimatorsoftheparametersintheequationy01x1…kxku.JustastheOLSestimatesmeasurethemarginalimpactofeachxjony,sodotheFGLSestimates.WeusetheFGLSestimatesinplaceoftheOLSestimatesbecausetheyaremoreefficientandhaveassociatedteststatisticswiththeusualtandFdistributions,atleastinlargesamples.Ifwehavesomedoubtaboutthevariancespecifiedinequation(8.30),wecanuseheteroskedasticity-robuststandarderrorsandteststatisticsinthetransformedequation.Anotherusefulalternativeforestimatinghiistoreplacetheindependentvariablesinregression(8.32)withtheOLSfittedvaluesandtheirsquares.Inotherwords,obtainthegˆiasthefittedvaluesfromtheregressionof22log(uˆ)onyˆ,yˆ(8.34)andthenobtainthehˆiexactlyasinequation(8.33).Thischangesonlystep(3)inthepreviousprocedure.Ifweuseregression(8.32)toestimatethevariancefunction,youmaybewonder-ingifwecansimplytestforheteroskedasticityusingthissameregression(anForLMtestcanbeused).Infact,Park(1966)suggestedthis.Unfortunately,whencomparedwiththetestsdiscussedinSection8.3,theParktesthassomeproblems.First,thenullhypothesismustbesomethingstrongerthanhomoskedasticity:effectively,uandxmustbeindependent.ThisisnotrequiredintheBreusch-PaganorWhitetests.Second,usingtheOLSresidualsuˆinplaceofuin(8.32)cancausetheFstatistictodeviatefromthe268\nd7/14/996:18PMPage269Chapter8HeteroskedasticityFdistribution,eveninlargesamplesizes.Thisisnotanissueintheothertestswehavecovered.Forthesereasons,theParktestisnotrecommendedwhentestingforhet-eroskedasticity.Thereasonthatregression(8.32)workswellforweightedleastsquaresisthatweonlyneedconsistentestimatorsofthej,andregression(8.32)certainlydeliversthose.EXAMPLE8.7(DemandforCigarettes)WeusethedatainSMOKE.RAWtoestimateademandfunctionfordailycigarettecon-sumption.Sincemostpeopledonotsmoke,thedependentvariable,cigs,iszeroformostobservations.Alinearmodelisnotidealbecauseitcanresultinnegativepredictedvalues.Nevertheless,wecanstilllearnsomethingaboutthedeterminantsofcigarettesmokingbyusingalinearmodel.Theequationestimatedbyordinaryleastsquares,withtheusualOLSstandarderrorsinparentheses,is0(ciˆgs3.64)(.880)log(income)0(.751)log(cigpric)ciˆgs(24.08)(.728)log(income)(5.773)log(cigpric)2(.501)educ(.771)age(.0090)age(2.83)restaurn2(8.35)(.167)educ(.160)age(.0017)age(1.11)restaurn2n807,R.0526,wherecigsisnumberofcigarettessmokedperday,incomeisannualincome,cigpricistheperpackpriceofcigarettes(incents),educisyearsofschooling,ageismeasuredinyears,andrestaurnisabinaryindicatorequaltounityifthepersonresidesinastatewithrestau-rantsmokingrestrictions.Sincewearealsogoingtodoweightedleastsquares,wedonotreporttheheteroskedasticity-robuststandarderrorsforOLS.(Incidentally,13outofthe807fittedvaluesarelessthanzero;thisislessthan2%ofthesampleandisnotamajorcauseforconcern.)Neitherincomenorcigarettepriceisstatisticallysignificantin(8.35),andtheireffectsarenotpracticallylarge.Forexample,ifincomeincreasesby10%,cigsispredictedtoincreaseby(.880/100)(10).088,orlessthanone-tenthofacigaretteperday.Themag-nitudeofthepriceeffectissimilar.Eachyearofeducationreducestheaveragecigarettessmokedperdaybyone-half,andtheeffectisstatisticallysignificant.Cigarettesmokingisalsorelatedtoage,inaquadraticfashion.Smokingincreaseswithageupuntilage.771/[2(.009)]42.83,andthensmokingdecreaseswithage.Bothtermsinthequadraticarestatisticallysignificant.Thepresenceofarestrictiononsmokinginrestaurantsdecreasescigarettesmokingbyalmostthreecigarettesperday,onaverage.Dotheerrorsunderlyingequation(8.35)containheteroskedasticity?TheBreusch-PaganregressionofthesquaredOLSresidualsontheindependentvariablesin(8.35)[see2equation(8.14)]producesRuˆ2.040.ThissmallR-squaredmayseemtoindicatenohet-eroskedasticity,butwemustremembertocomputeeithertheForLMstatistic.Ifthe2samplesizeislarge,aseeminglysmallRuˆ2canresultinaverystrongrejectionof269\nd7/14/996:18PMPage270Part1RegressionAnalysiswithCross-SectionalDatahomoskedasticity.TheLMstatisticisLM807(.040)32.28,andthisistheoutcomeof2a6randomvariable.Thep-valueislessthan.000015,whichisverystrongevidenceofheteroskedasticity.Therefore,weestimatetheequationusingthepreviousfeasibleGLSprocedure.Theestimatedequationis0(ciˆgs5.64)(1.30)log(income)02.94)log(cigpric)ciˆgs(17.80)(.44)log(income)(4.46)log(cigpric)2(.463)educ(.482)age(.0056)age(3.46)restaurn2(8.36)(.120)educ(.097)age(.0009)age(.80)restaurn2n807,R.1134.Theincomeeffectisnowstatisticallysignificantandlargerinmagnitude.Thepriceeffectisalsonotablybigger,butitisstillstatisticallyinsignificant.(Onereasonforthisisthatcigpricvariesonlyacrossstatesinthesample,andsothereismuchlessvariationinlog(cigpric)thaninlog(income),educ,andage.)Theestimatesontheothervariableshave,naturally,changedsomewhat,butthebasicstoryisstillthesame.Cigarettesmokingisnegativelyrelatedtoschooling,hasaquadraticrelationshipwithage,andisnegativelyaffectedbyrestaurantsmokingrestrictions.WemustbealittlecarefulincomputingFstatisticsfortestingmultiplehypothesesafterestimationbyWLS.(ThisistruewhetherthesumofsquaredresidualsorR-squaredformoftheFstatisticisused.)Itisimportantthatthesameweightsbeusedtoestimatetheunrestrictedandrestrictedmodels.WeshouldfirstestimatetheunrestrictedmodelbyOLS.Oncewehaveobtainedtheweights,wecanusethemtoestimatetherestrictedmodelaswell.TheFstatisticcanbecomputedasusual.Fortunately,manyregressionpackageshaveasimplecom-mandfortestingjointrestrictionsafterQUESTION8.4WLSestimation,soweneednotperformSupposethatthemodelforheteroskedasticityinequation(8.30)istherestrictedregressionourselves.notcorrect,butweusethefeasibleGLSprocedurebasedonthisExample8.7hintsatanissuethatvariance.WLSisstillconsistent,buttheusualstandarderrors,tsta-sometimesarisesinapplicationsoftistics,andsoonwillnotbevalid,evenasymptotically.Whatcanwedoinstead?[Hint:Seeequation(8.26),whereu*containshet-iweightedleastsquares:theOLSandWLSeroskedasticityifVar(ux)2h(x).]estimatescanbesubstantiallydifferent.Thisisnotsuchabigprobleminthedemandforcigarettesequationbecauseallthecoefficientsmaintainthesamesigns,andthebiggestchangesareonvariablesthatwerestatisticallyinsignificantwhentheequa-tionwasestimatedbyOLS.TheOLSandWLSestimateswillalwaysdifferduetosam-plingerror.Theissueiswhethertheirdifferenceisenoughtochangeimportantconclusions.IfOLSandWLSproducestatisticallysignificantestimatesthatdifferinsign—forexample,theOLSpriceelasticityispositiveandsignificant,whiletheWLSpriceelas-ticityisnegativeandsignficant—orthedifferenceinmagnitudesoftheestimatesispracticallylarge,weshouldbesuspicious.Typically,thisindicatesthatoneoftheother270\nd7/14/996:18PMPage271Chapter8HeteroskedasticityGauss-Markovassumptionsisfalse,particularlythezeroconditionalmeanassumptionontheerror(MLR.3).CorrelationbetweenuandanyindependentvariablecausesbiasandinconsistencyinOLSandWLS,andthebiaseswillusuallybedifferent.TheHausmantest[Hausman(1978)]canbeusedtoformallycomparetheOLSandWLSestimatestoseeiftheydifferbymorethanthesamplingerrorsuggests.Thistestisbeyondthescopeofthistext.Inmanycases,aninformal“eyeballing”oftheestimatesissufficienttodetectaproblem.8.5THELINEARPROBABILITYMODELREVISITEDAswesawinSection7.6,whenthedependentvariableyisabinaryvariable,themodelmustcontainheteroskedasticity,unlessalloftheslopeparametersarezero.Wearenowinapositiontodealwiththisproblem.ThesimplestwaytodealwithheteroskedasticityinthelinearprobabilitymodelistocontinuetouseOLSestimation,buttoalsocomputerobuststandarderrorsinteststa-tistics.ThisignoresthefactthatweactuallyknowtheformofheteroskedasticityfortheLPM.Nevertheless,OLSestimatesoftheLPMissimpleandoftenproducessatisfac-toryresults.EXAMPLE8.8(LaborForceParticipationofMarriedWomen)InthelaborforceparticipationexampleinSection7.6[seeequation(7.29)],wereportedtheusualOLSstandarderrors.Nowwecomputetheheteroskedasticity-robuststandarderrorsaswell.Thesearereportedinbracketsbelowtheusualstandarderrors:inˆlf(.586)(.0034)nwifeinc(.038)educ(.039)experinˆlf(.154)(.0014)nwifeinc(.007)educ(.006)experinˆlf[.151][.0015]nwifeinc[.007]educ[.006]exper26(.00060)exper(.016)age(.262)kidslt6(.0130)kidsge6(8.37)2(.00018)exper(.002)age(.034)kidslt6(.0132)kidslt62[.00019]exper[.002]age[.032]kidslt6[.0135]kidslt62n753,R.264.SeveraloftherobustandOLSstandarderrorsarethesametothereporteddegreeofpre-cision;inallcasesthedifferencesarepracticallyverysmall.Therefore,whilehet-eroskedasticityisaproblemintheory,itisnotinpractice,atleastnotforthisexample.ItoftenturnsoutthattheusualOLSstandarderrorsandteststatisticsaresimilartotheirheteroskedasticity-robustcounterparts.Furthermore,itrequiresaminimalefforttocom-puteboth.Generally,theOLSesimatorsareinefficientintheLPM.RecallthattheconditionalvarianceofyintheLPMis271\nd7/14/996:18PMPage272Part1RegressionAnalysiswithCross-SectionalDataVar(yx)p(x)[1p(x)],(8.38)wherep(x)01x1…kxk(8.39)istheresponseprobability(probabilityofsuccess,y1).Itseemsnaturaltouseweightedleastsquares,butthereareacoupleofhitches.Theprobabilityp(x)clearlydependsontheunknownpopulationparameters,j.Nevertheless,wedohaveunbiasedestimatorsoftheseparameters,namelytheOLSestimators.WhentheOLSestimatorsarepluggedintoequation(8.39),weobtaintheOLSfittedvalues.Thus,foreachobser-vationi,Var(yixi)isestimatedbyhˆiyˆi(1yˆi),(8.40)whereyˆiistheOLSfittedvalueforobservationi.NowweapplyfeasibleGLS,justasinSection8.4.Unfortunately,beingabletoestimatehiforeachidoesnotmeanthatwecanpro-ceeddirectlywithWLSestimation.TheproblemisonethatwebrieflydiscussedinSection7.6:thefittedvaluesyˆineednotfallintheunitinterval.Ifeitheryˆi0oryˆi1,equation(8.40)showsthathˆiwillbenegative.SinceWLSproceedsbymultiplyingobservationiby1/hˆi,themethodwillfailifhˆiisnegative(orzero)foranyobserva-tion.Inotherwords,alloftheweightsforWLSmustbepositive.Insomecases,0yˆi1foralli,inwhichcaseWLScanbeusedtoestimatetheLPM.Incaseswithmanyobservationsandsmallprobabilitiesofsuccessorfailure,itisverycommontofindsomefittedvaluesoutsidetheunitinterval.Ifthishappens,asitdoesinthelaborforceparticipationexampleinequation(8.37),itiseasiesttoaban-donWLSandtoreporttheheteroskedasticity-robuststatistics.Analternativeistoadjustthosefittedvaluesthatarelessthanzeroorgreaterthanunity,andthentoapplyWLS.Onesuggestionistosetyˆi.01ifyˆi0andyˆi.99ifyˆi1.Unfortunately,thisrequiresanarbitrarychoiceonthepartoftheresearcher—forexample,whynotuse.001and.999astheadjustedvalues?Ifmanyfittedvaluesareoutsidetheunitinterval,theadjustmenttothefittedvaluescanaffecttheresults;inthissituation,itisprobablybesttojustuseOLS.ESTIMATINGTHELINEARPROBABILITYMODELBYWEIGHTEDLEASTSQUARES:1.EstimatethemodelbyOLSandobtainthefittedvalues,yˆ.2.Determinewhetherallofthefittedvaluesareinsidetheunitinterval.Ifso,pro-ceedtostep(3).Ifnot,someadjustmentisneededtobringallfittedvaluesintotheunitinterval.3.Constructtheestimatedvariancesinequation(8.40).4.Estimatetheequationy01x1…kxkubyWLS,usingweights1/hˆ.272\nd7/14/996:18PMPage273Chapter8HeteroskedasticityEXAMPLE8.9(DeterminantsofPersonalComputerOwnership)WeusethedatainGPA1.RAWtoestimatetheprobabilityofowningacomputer.LetPCdenoteabinaryindicatorequaltounityifthestudentownsacomputer,andzeroother-wise.ThevariablehsGPAishighschoolGPA,ACTisachievementtestscore,andparcollisabinaryindicatorequaltounityifatleastoneparentattendedcollege.(Separatecollegeindicatorsforthemotherandthefatherdonotyieldindividuallysignificantresults,astheseareprettyhighlycorrelated.)TheequationestimatedbyOLSis(PˆC.0004)(.065)hsGPA(.0006)ACT(.221)parcollPˆC(.4905)(.137)hsGPA(.0155)ACT(.093)parcollPˆC[.4888][.139]hsGPA[.0158]ACT[.087]parcoll(8.41)2n141,R.0415.JustaswithExample8.8,therearenostrikingdifferencesbetweentheusualandrobuststandarderrors.Nevertheless,wealsoestimatethemodelbyWLS.BecausealloftheOLSfittedvaluesareinsidetheunitinterval,noadjustmentsareneeded:PˆC(.026)(.033)hsGPA(.0043)ACT(.215)parcollPˆC(.477)(.130)hsGPA(.0155)ACT(.086)parcoll(8.42)2n141,R.0464.TherearenoimportantdifferencesintheOLSandWLSestimates.Theonlysignificantexplanatoryvariableisparcoll,andinbothcasesweestimatethattheprobabilityofPCownershipisabout.22higher,ifatleastoneparentattendedcollege.SUMMARYWebeganbyreviewingthepropertiesofordinaryleastsquaresinthepresenceofhet-eroskedasticity.HeteroskedasticitydoesnotcausebiasorinconsistencyintheOLSesti-mators,buttheusualstandarderrorsandteststatisticsarenolongervalid.Weshowedhowtocomputeheteroskedasticity-robuststandarderrorsandtstatistics,somethingthatisroutinelydonebymanyregressionpackages.Mostregressionpackagesalsocomputeaheteroskedasticity-robust,F-typestatistic.Wediscussedtwocommonwaystotestforheteroskedasticity:theBreusch-PagantestandaspecialcaseoftheWhitetest.BothofthesestatisticsinvolveregressingthesquaredOLSresidualsoneithertheindependentvariables(BP)orthefittedandsquaredfittedvalues(White).AsimpleFtestisasymptoticallyvalid;therearealsoLagrangemultiplierversionsofthetests.OLSisnolongerthebestlinearunbiasedestimatorinthepresenceofhet-eroskedasticity.Whentheformofheteroskedasticityisknown,generalizedleast273\nd7/14/996:18PMPage274Part1RegressionAnalysiswithCross-SectionalDatasquares(GLS)estimationcanbeused.ThisleadstoweightedleastsquaresasameansofobtainingtheBLUEestimator.TheteststatisticsfromtheWLSestimationareeitherexactlyvalidwhentheerrortermisnormallydistributedorasymptoticallyvalidundernonnormality.Thisassumes,ofcourse,thatwehavethepropermodelofheteroskedas-ticity.Morecommonly,wemustestimateamodelfortheheteroskedasticitybeforeapply-ingWLS.TheresultingfeasibleGLSestimatorisnolongerunbiased,butitisconsis-tentandasymptoticallyefficient.TheusualstatisticsfromtheWLSregressionareasymptoticallyvalid.Wediscussedamethodtoensurethattheestimatedvariancesarestrictlypositiveforallobservations,somethingneededtoapplyWLS.AswediscussedinChapter7,thelinearprobabilitymodelforabinarydependentvariablenecessarilyhasaheteroskedasticerrorterm.Asimplewaytodealwiththisproblemistocomputeheteroskedasticity-robuststatistics.Alternatively,ifallthefittedvalues(thatis,theestimatedprobabilities)arestrictlybetweenzeroandone,weightedleastsquarescanbeusedtoobtainasymptoticallyefficientestimators.KEYTERMSBreusch-PaganTestforHeteroskedasticity-RobustFStatisticHeteroskedasticity(BPTest)Heteroskedasticity-RobustLMStatisticFeasibleGLS(FGLS)EstimatorHeteroskedasticity-RobusttStatisticGeneralizedLeastSquares(GLS)WeightedLeastSquares(WLS)EstimatorsEstimatorsHeteroskedasticityofUnknownFormWhiteTestforHeteroskedasticityHeteroskedasticity-RobustStandardErrorPROBLEMS8.1Whichofthefollowingareconsequencesofheteroskedasticity?(i)TheOLSestimators,ˆj,areinconsistent.(ii)TheusualFstatisticnolongerhasanFdistribution.(iii)TheOLSestimatorsarenolongerBLUE.8.2Consideralinearmodeltoexplainmonthlybeerconsumption:beer01inc2price3educ4femaleuE(uinc,price,educ,female)022Var(uinc,price,educ,female)inc.Writethetransformedequationthathasahomoskedasticerrorterm.8.3TrueorFalse:WLSispreferredtoOLS,whenanimportantvariablehasbeenomittedfromthemodel.8.4UsingthedatainGPA3.RAW,thefollowingequationwasestimatedforthefallandsecondsemesterstudents:274\nd7/14/996:18PMPage275Chapter8Heteroskedasticity(trmˆgpa2.12)(.900)crsgpa(.193)cumgpa(.0014)tothrs2trmgpa(.55)(.175)crsgpa(.064)cumgpa(.0012)tothrs2trmgpa[.55][.166]crsgpa[.074]cumgpa[.0012]tothrs(.0018)sat(.0039)hsperc(.351)female(.157)season(.0002)sat(.0018)hsperc(.085)female(.098)season[.0002]sat[.0019]hsperc[.079]female[.080]season2n269,R.465.Here,trmgpaistermGPA,crsgpaisaweightedaverageofoverallGPAincoursestaken,tothrsistotalcredithourspriortothesemester,satisSATscore,hspercisgrad-uatingpercentileinhighschoolclass,femaleisagenderdummy,andseasonisadummyvariableequaltounityifthestudent’ssportisinseasonduringthefall.Theusualandheteroskedasticity-robuststandarderrorsarereportedinparenthesesandbrackets,respectively.(i)Dothevariablescrsgpa,cumgpa,andtothrshavetheexpectedesti-matedeffects?Whichofthesevariablesarestatisticallysignificantatthe5%level?Doesitmatterwhichstandarderrorsareused?(ii)WhydoesthehypothesisH0:crsgpa1makesense?Testthishypoth-esisagainstthetwo-sidedalternativeatthe5%level,usingbothstan-darderrors.Describeyourconclusions.(iii)Testwhetherthereisanin-seasoneffectontermGPA,usingbothstan-darderrors.Doesthesignificancelevelatwhichthenullcanberejecteddependonthestandarderrorused?8.5Thevariablesmokesisabinaryvariableequaltooneifapersonsmokes,andzerootherwise.UsingthedatainSMOKE.RAW,weestimatealinearprobabilitymodelforsmokes:smoˆkes(.656)(.069)log(cigpric)(.012)log(income)(.029)educsmoˆkes(.855)(.204)log(cigpric)(.026)log(income)(.006)educsmoˆkes[.856][.207]log(cigpric)[.026]log(income)[.006]educ2(.020)age(.00026)age(.101)restaurn(.026)white2(.006)age(.00006)age(.039)restaurn(.052)white2[.005]age[.00006]age[.038]restaurn[.050]white2n807,R.062.Thevariablewhiteequalsoneiftherespondentiswhite,andzerootherwise;theotherindependentvariablesaredefinedinExample8.7.Boththeusualandheteroskedasticity-robuststandarderrorsarereported.(i)Arethereanyimportantdifferencesbetweenthetwosetsofstandarderrors?(ii)Holdingotherfactorsfixed,ifeducationincreasesbyfouryears,whathappenstotheestimatedprobabilityofsmoking?(iii)Atwhatpointdoesanotheryearofagereducetheprobabilityofsmok-ing?(iv)Interpretthecoefficientonthebinaryvariablerestaurn(adummyvari-ableequaltooneifthepersonlivesinastatewithrestaurantsmokingrestrictions).275\nd7/14/996:18PMPage276Part1RegressionAnalysiswithCross-SectionalData(v)Personnumber206inthedatasethasthefollowingcharacteristics:cigpric67.44,income6,500,educ16,age77,restaurn0,white0,andsmokes0.Computethepredictedprobabilityofsmokingforthispersonandcommentontheresult.COMPUTEREXERCISES8.6UsethedatainSLEEP75.RAWtoestimatethefollowingsleepequation:2sleep01totwrk2educ3age4age5yngkid6maleu.(i)Writedownamodelthatallowsthevarianceofutodifferbetweenmenandwomen.Thevarianceshouldnotdependonotherfactors.(ii)Estimatetheparametersofthemodelforheteroskedasticty.(YouhavetoestimatethesleepequationbyOLS,first,toobtaintheOLSresidu-als.)Istheestimatedvarianceofuhigherformenorforwomen?(iii)Isthevarianceofustatisticallydifferentformenandforwomen?8.7(i)UsethedatainHPRICE1.RAWtoobtaintheheteroskedasticity-robuststan-darderrorsforequation(8.17).Discussanyimportantdifferenceswiththeusualstandarderrors.(ii)Repeatpart(i)forequation(8.18).(iii)Whatdoesthisexamplesuggestaboutheteroskedasticityandthetrans-formationusedforthedependentvariable?8.8ApplythefullWhitetestforheteroskedasticity[seeequation(8.19)]toequation(8.18).Usingthechi-squareformofthestatistic,obtainthep-value.Whatdoyoucon-clude?8.9UseVOTE1.RAWforthisexercise.(i)EstimateamodelwithvoteAasthedependentvariableandprtystrA,democA,log(expendA),andlog(expendB)asindependentvariables.ObtaintheOLSresiduals,uˆi,andregresstheseonalloftheindependent2variables.ExplainwhyyouobtainR0.(ii)NowcomputetheBreusch-Pagantestforheteroskedasticity.UsetheFstatisticversionandreportthep-value.(iii)ComputethespecialcaseoftheWhitetestforheteroskedasticity,againusingtheFstatisticform.Howstrongistheevidenceforheteroskedas-ticitynow?8.10UsethedatainPNTSPRD.RAWforthisexercise.(i)ThevariablesprdcvrisabinaryvariableequaltooneiftheLasVegaspointspreadforacollegebasketballgamewascovered.Theexpectedvalueofsprdcvr,say,istheprobabilitythatthespreadiscoveredinarandomlyselectedgame.TestH0:.5againstH1:.5atthe10%significancelevelanddiscussyourfindings.(Hint:Thisiseasilydoneusingattestbyregressingsprdcvronaninterceptonly.)(ii)Howmanygamesinthesampleof553wereplayedonaneutralcourt?276\nd7/14/996:18PMPage277Chapter8Heteroskedasticity(iii)Estimatethelinearprobabilitymodelsprdcvr01favhome2neutral3fav254und25uandreporttheresultsintheusualform.(ReporttheusualOLSstandarderrorsandtheheteroskedasticity-robuststandarderrors.)Whichvari-ableismostsignificant,bothpracticallyandstatistically?(iv)Explainwhy,underthenullhypothesisH0:12340,thereisnoheteroskedasticityinthemodel.(v)UsetheusualFstatistictotestthehypothesisinpart(iv).Whatdoyouconclude?(vi)Giventhepreviousanalysis,wouldyousaythatitispossibletosys-tematicallypredictwhethertheLasVegasspreadwillbecoveredusinginformationavailablepriortothegame?8.11InExample7.12,weestimatedalinearprobabilitymodelforwhetherayoungmanwasarrestedduring1986:arr8601pcnv2avgsen3tottime4ptime865qemp86u.(i)EstimatethismodelbyOLSandverifythatallfittedvaluesarestrictlybetweenzeroandone.Whatarethesmallestandlargestfittedvalues?(ii)Estimatetheequationbyweightedleastsquares,asdiscussedinSection8.5.(iii)UsetheWLSestimatestodeterminewhetheravgsenandtottimearejointlysignificantatthe5%level.8.12UsethedatainLOANAPP.RAWforthisexercise.(i)Estimatetheequationinpart(iii)ofProblem7.16,computingtheheteroskedasticity-robuststandarderrors.Comparethe95%confidenceintervalonwhitewiththenonrobustconfidenceinterval.(ii)Obtainthefittedvaluesfromtheregressioninpart(i).Areanyofthemlessthanzero?Areanyofthemgreaterthanone?Whatdoesthismeanaboutapplyingweightedleastsquares?277\nChapterTenBasicRegressionAnalysiswithTimeSeriesDatanthischapter,webegintostudythepropertiesofOLSforestimatinglinearregressionImodelsusingtimeseriesdata.InSection10.1,wediscusssomeconceptualdiffer-encesbetweentimeseriesandcross-sectionaldata.Section10.2providessomeexam-plesoftimeseriesregressionsthatareoftenestimatedintheempiricalsocialsciences.WethenturnourattentiontothefinitesamplepropertiesoftheOLSestimatorsandstatetheGauss-Markovassumptionsandtheclassicallinearmodelassumptionsfortimeseriesregression.Whiletheseassumptionshavefeaturesincommonwiththoseforthecross-sectionalcase,theyalsohavesomesignificantdifferencesthatwewillneedtohighlight.Inaddition,wereturntosomeissuesthatwetreatedinregressionwithcross-sectionaldata,suchashowtouseandinterpretthelogarithmicfunctionalformanddummyvariables.TheimportanttopicsofhowtoincorporatetrendsandaccountforseasonalityinmultipleregressionaretakenupinSection10.5.10.1THENATUREOFTIMESERIESDATAAnobviouscharacteristicoftimeseriesdatawhichdistinguishesitfromcross-sectionaldataisthatatimeseriesdatasetcomeswithatemporalordering.Forexample,inChapter1,webrieflydiscussedatimeseriesdatasetonemployment,theminimumwage,andothereconomicvariablesforPuertoRico.Inthisdataset,wemustknowthatthedatafor1970immediatelyprecedethedatafor1971.Foranalyzingtimeseriesdatainthesocialsciences,wemustrecognizethatthepastcaneffectthefuture,butnotviceversa(unlikeintheStarTrekuniverse).Toemphasizetheproperorderingoftimeseriesdata,Table10.1givesapartiallistingofthedataonU.S.inflationandunemploymentratesinPHILLIPS.RAW.Anotherdifferencebetweencross-sectionalandtimeseriesdataismoresubtle.InChapters3and4,westudiedstatisticalpropertiesoftheOLSestimatorsbasedonthenotionthatsampleswererandomlydrawnfromtheappropriatepopulation.Understandingwhycross-sectionaldatashouldbeviewedasrandomoutcomesisfairlystraightforward:adifferentsampledrawnfromthepopulationwillgenerallyyielddif-ferentvaluesoftheindependentanddependentvariables(suchaseducation,experi-ence,wage,andsoon).Therefore,theOLSestimatescomputedfromdifferentrandomsampleswillgenerallydiffer,andthisiswhyweconsidertheOLSestimatorstoberan-domvariables.311\nPart2RegressionAnalysiswithTimeSeriesDataTable10.1PartialListingofDataonU.S.InflationandUnemploymentRates,1948–1996YearInflationUnemployment19488.13.819491.25.919501.35.319517.93.319942.66.119952.85.619963.05.4Howshouldwethinkaboutrandomnessintimeseriesdata?Certainly,economictimeseriessatisfytheintuitiverequirementsforbeingoutcomesofrandomvariables.Forexample,todaywedonotknowwhattheDowJonesIndustrialAveragewillbeatitscloseattheendofthenexttradingday.WedonotknowwhattheannualgrowthinoutputwillbeinCanadaduringthecomingyear.Sincetheoutcomesofthesevariablesarenotforeknown,theyshouldclearlybeviewedasrandomvariables.Formally,asequenceofrandomvariablesindexedbytimeiscalledastochasticprocessoratimeseriesprocess.(“Stochastic”isasynonymforrandom.)Whenwecollectatimeseriesdataset,weobtainonepossibleoutcome,orrealization,ofthesto-chasticprocess.Wecanonlyseeasinglerealization,becausewecannotgobackintimeandstarttheprocessoveragain.(Thisisanalogoustocross-sectionalanalysiswherewecancollectonlyonerandomsample.)However,ifcertainconditionsinhistoryhadbeendifferent,wewouldgenerallyobtainadifferentrealizationforthestochasticprocess,andthisiswhywethinkoftimeseriesdataastheoutcomeofrandomvariables.Thesetofallpossiblerealizationsofatimeseriesprocessplaystheroleofthepopulationincross-sectionalanalysis.10.2EXAMPLESOFTIMESERIESREGRESSIONMODELSInthissection,wediscusstwoexamplesoftimeseriesmodelsthathavebeenusefulinempiricaltimeseriesanalysisandthatareeasilyestimatedbyordinaryleastsquares.WewillstudyadditionalmodelsinChapter11.312\nChapter10BasicRegressionAnalysiswithTimeSeriesDataStaticModelsSupposethatwehavetimeseriesdataavailableontwovariables,sayyandz,whereytandztaredatedcontemporaneously.Astaticmodelrelatingytozisyt01ztut,t1,2,…,n.(10.1)Thename“staticmodel”comesfromthefactthatwearemodelingacontemporaneousrelationshipbetweenyandz.Usually,astaticmodelispostulatedwhenachangeinzattimetisbelievedtohaveanimmediateeffectony:yt1zt,whenut0.Staticregressionmodelsarealsousedwhenweareinterestedinknowingthetradeoffbetweenyandz.AnexampleofastaticmodelisthestaticPhillipscurve,givenbyinft01unemtut,(10.2)whereinftistheannualinflationrateandunemtistheunemploymentrate.ThisformofthePhillipscurveassumesaconstantnaturalrateofunemploymentandconstantinfla-tionaryexpectations,anditcanbeusedtostudythecontemporaneoustradeoffbetweenthem.[See,forexample,Mankiw(1994,Section11.2).]Naturally,wecanhaveseveralexplanatoryvariablesinastaticregressionmodel.Letmrdrtetdenotethemurdersper10,000peopleinaparticularcityduringyeart,letconvrtetdenotethemurderconvictionrate,letunemtbethelocalunemploymentrate,andletyngmletbethefractionofthepopulationconsistingofmalesbetweentheagesof18and25.Then,astaticmultipleregressionmodelexplainingmurderratesismrdrtet01convrtet2unemt3yngmletut.(10.3)Usingamodelsuchasthis,wecanhopetoestimate,forexample,theceterisparibuseffectofanincreaseintheconvictionrateoncriminalactivity.FiniteDistributedLagModelsInafinitedistributedlag(FDL)model,weallowoneormorevariablestoaffectywithalag.Forexample,forannualobservations,considerthemodelgfrt00pet1pet12pet2ut,(10.4)wheregfrtisthegeneralfertilityrate(childrenbornper1,000womenofchildbearingage)andpetistherealdollarvalueofthepersonaltaxexemption.Theideaistoseewhether,intheaggregate,thedecisiontohavechildrenislinkedtothetaxvalueofhav-ingachild.Equation(10.4)recognizesthat,forbothbiologicalandbehavioralreasons,decisionstohavechildrenwouldnotimmediatelyresultfromchangesinthepersonalexemption.Equation(10.4)isanexampleofthemodelyt00zt1zt12zt2ut,(10.5)313\nPart2RegressionAnalysiswithTimeSeriesDatawhichisanFDLofordertwo.Tointerpretthecoefficientsin(10.5),supposethatzisaconstant,equaltoc,inalltimeperiodsbeforetimet.Attimet,zincreasesbyoneunittoc1andthenrevertstoitspreviouslevelattimet1.(Thatis,theincreaseinzistemporary.)Moreprecisely,…,zt2c,zt1c,ztc1,zt1c,zt2c,….Tofocusontheceterisparibuseffectofzony,wesettheerrortermineachtimeperiodtozero.Then,yt100c1c2c,yt00(c1)1c2c,yt100c1(c1)2c,yt200c1c2(c1),yt300c1c2c,andsoon.Fromthefirsttwoequations,ytyt10,whichshowsthat0istheimmediatechangeinyduetotheone-unitincreaseinzattimet.0isusuallycalledtheimpactpropensityorimpactmultiplier.Similarly,1yt1yt1isthechangeinyoneperiodafterthetemporarychange,and2yt2yt1isthechangeinytwoperiodsafterthechange.Attimet3,yhasrevertedbacktoitsinitiallevel:yt3yt1.Thisisbecausewehaveassumedthatonlytwolagsofzappearin(10.5).Whenwegraphthejasafunctionofj,weobtainthelagdistribution,whichsummarizesthedynamiceffectthatatemporaryincreaseinzhasony.ApossiblelagdistributionfortheFDLofordertwoisgiveninFigure10.1.(Ofcourse,wewouldneverknowtheparametersj;instead,wewillestimatethejandthenplottheestimatedlagdistribution.)ThelagdistributioninFigure10.1impliesthatthelargesteffectisatthefirstlag.Thelagdistributionhasausefulinterpretation.Ifwestandardizetheinitialvalueofyatyt10,thelagdistributiontracesoutallsubsequentvaluesofyduetoaone-unit,temporaryincreaseinz.Wearealsointerestedinthechangeinyduetoapermanentincreaseinz.Beforetimet,zequalstheconstantc.Attimet,zincreasespermanentlytoc1:zsc,standzsc1,st.Again,settingtheerrorstozero,wehaveyt100c1c2c,yt00(c1)1c2c,yt100(c1)1(c1)2c,yt200(c1)1(c1)2(c1),andsoon.Withthepermanentincreaseinz,afteroneperiod,yhasincreasedby01,andaftertwoperiods,yhasincreasedby012.Therearenofurtherchangesinyaftertwoperiods.Thisshowsthatthesumofthecoefficientsoncurrentandlaggedz,012,isthelong-runchangeinygivenapermanentincreaseinzandiscalledthelong-runpropensity(LRP)orlong-runmultiplier.TheLRPisoftenofinterestindistributedlagmodels.314\nChapter10BasicRegressionAnalysiswithTimeSeriesDataFigure10.1Alagdistributionwithtwononzerolags.Themaximumeffectisatthefirstlag.coefficient()j1234lagAsanexample,inequation(10.4),0measurestheimmediatechangeinfertilityduetoaone-dollarincreaseinpe.Aswementionedearlier,therearereasonstobelievethat0issmall,ifnotzero.But1or2,orboth,mightbepositive.Ifpepermanentlyincreasesbyonedollar,then,aftertwoyears,gfrwillhavechangedby012.Thismodelassumesthattherearenofurtherchangesaftertwoyears.Whetherornotthisisactuallythecaseisanempiricalmatter.Afinitedistributedlagmodeloforderqiswrittenasyt00zt1zt1…qztqut.(10.6)Thiscontainsthestaticmodelasaspecialcasebysetting1,2,…,qequaltozero.Sometimes,aprimarypurposeforestimatingadistributedlagmodelistotestwhetherzhasalaggedeffectony.Theimpactpropensityisalwaysthecoefficientonthecon-temporaneousz,0.Occasionally,weomitztfrom(10.6),inwhichcasetheimpactpropensityiszero.Thelagdistributionisagainthejgraphedasafunctionofj.Thelong-runpropensityisthesumofallcoefficientsonthevariablesztj:LRP01…q.(10.7)315\nPart2RegressionAnalysiswithTimeSeriesDataBecauseoftheoftensubstantialcorrelationinzatdifferentlags—thatis,duetomulti-collinearityin(10.6)—itcanbedifficulttoobtainpreciseestimatesoftheindividualj.Interestingly,evenwhenthejcannotbepreciselyestimated,wecanoftengetgoodQUESTION10.1estimatesoftheLRP.Wewillseeanexam-Inanequationforannualdata,supposethatplelater.Wecanhavemorethanoneexplanatoryintt1.6.48inft.15inft1.32inft2ut,variableappearingwithlags,orwecanaddwhereintisaninterestrateandinfistheinflationrate,whatarethecontemporaneousvariablestoanFDLimpactandlong-runpropensities?model.Forexample,theaverageeducationlevelforwomenofchildbearingagecouldbeaddedto(10.4),whichallowsustoaccountforchangingeducationlevelsforwomen.AConventionAbouttheTimeIndexWhenmodelshavelaggedexplanatoryvariables(and,aswewillseeinthenextchap-ter,modelswithlaggedy),confusioncanariseconcerningthetreatmentofinitialobser-vations.Forexample,ifin(10.5),weassumethattheequationholds,startingatt1,thentheexplanatoryvariablesforthefirsttimeperiodarez1,z0,andz1.Ourconven-tionwillbethatthesearetheinitialvaluesinoursample,sothatwecanalwaysstartthetimeindexatt1.Inpractice,thisisnotveryimportantbecauseregressionpack-agesautomaticallykeeptrackoftheobservationsavailableforestimatingmodelswithlags.Butforthisandthenextfewchapters,weneedsomeconventionconcerningthefirsttimeperiodbeingrepresentedbytheregressionequation.10.3FINITESAMPLEPROPERTIESOFOLSUNDERCLASSICALASSUMPTIONSInthissection,wegiveacompletelistingofthefinitesample,orsmallsample,prop-ertiesofOLSunderstandardassumptions.Wepayparticularattentiontohowtheassumptionsmustbealteredfromourcross-sectionalanalysistocovertimeseriesregressions.UnbiasednessofOLSThefirstassumptionsimplystatesthatthetimeseriesprocessfollowsamodelwhichislinearinitsparameters.ASSUMPTIONTS.1(LINEARINPARAMETERS)Thestochasticprocess{(xt1,xt2,…,xtk,yt):t1,2,…,n}followsthelinearmodelyt01xt1…kxtkut,(10.8)where{ut:t1,2,…,n}isthesequenceoferrorsordisturbances.Here,nisthenumberofobservations(timeperiods).316\nChapter10BasicRegressionAnalysiswithTimeSeriesDataTable10.2ExampleofXfortheExplanatoryVariablesinEquation(10.3)tconvrteunemyngmle1.46.074.122.42.071.123.42.063.114.47.062.095.48.060.106.50.059.117.55.058.128.56.059.13Inthenotationxtj,tdenotesthetimeperiod,andjis,asusual,alabeltoindicateoneofthekexplanatoryvariables.Theterminologyusedincross-sectionalregressionapplieshere:ytisthedependentvariable,explainedvariable,orregressand;thextjaretheindependentvariables,explanatoryvariables,orregressors.WeshouldthinkofAssumptionTS.1asbeingessentiallythesameasAssumptionMLR.1(thefirstcross-sectionalassumption),butwearenowspecifyingalinearmodelfortimeseriesdata.TheexamplescoveredinSection10.2canbecastintheformof(10.8)byappropriatelydefiningxtj.Forexample,equation(10.5)isobtainedbysettingxt1zt,xt2zt1,andxt3zt2.Inordertostateanddiscussseveraloftheremainingassumptions,weletxt(xt1,xt2,…,xtk)denotethesetallindependentvariablesintheequationattimet.Further,Xdenotesthecollectionofallindependentvariablesforalltimeperiods.ItisusefultothinkofXasbeinganarray,withnrowsandkcolumns.Thisreflectshowtimeseriesthdataarestoredineconometricsoftwarepackages:thetrowofXisxt,consistingofallindependentvariablesfortimeperiodt.Therefore,thefirstrowofXcorrespondstot1,thesecondrowtot2,andthelastrowtotn.AnexampleisgiveninTable10.2,usingn8andtheexplanatoryvariablesinequation(10.3).ThenextassumptionisthetimeseriesanalogofAssumptionMLR.3,anditalsodropstheassumptionofrandomsamplinginAssumptionMLR.2.ASSUMPTIONTS.2(ZEROCONDITIONALMEAN)Foreacht,theexpectedvalueoftheerrorut,giventheexplanatoryvariablesforalltimeperiods,iszero.Mathematically,317\nPart2RegressionAnalysiswithTimeSeriesDataE(utX)0,t1,2,…,n.(10.9)Thisisacrucialassumption,andweneedtohaveanintuitivegraspofitsmeaning.Asinthecross-sectionalcase,itiseasiesttoviewthisassumptionintermsofuncorrelat-edness:AssumptionTS.2impliesthattheerrorattimet,ut,isuncorrelatedwitheachexplanatoryvariableineverytimeperiod.Thefactthatthisisstatedintermsofthecon-ditionalexpectationmeansthatwemustalsocorrectlyspecifythefunctionalrelation-shipbetweenytandtheexplanatoryvariables.IfutisindependentofXandE(ut)0,thenAssumptionTS.2automaticallyholds.Giventhecross-sectionalanalysisfromChapter3,itisnotsurprisingthatwerequireuttobeuncorrelatedwiththeexplanatoryvariablesalsodatedattimet:incon-ditionalmeanterms,E(utxt1,…,xtk)E(utxt)0.(10.10)When(10.10)holds,wesaythatthextjarecontemporaneouslyexogenous.Equation(10.10)impliesthatutandtheexplanatoryvariablesarecontemporaneouslyuncorre-lated:Corr(xtj,ut)0,forallj.AssumptionTS.2requiresmorethancontemporaneousexogeneity:utmustbeuncorrelatedwithxsj,evenwhenst.Thisisastrongsenseinwhichtheexplanatoryvariablesmustbeexogenous,andwhenTS.2holds,wesaythattheexplanatoryvari-ablesarestrictlyexogenous.InChapter11,wewilldemonstratethat(10.10)issuffi-cientforprovingconsistencyoftheOLSestimator.ButtoshowthatOLSisunbiased,weneedthestrictexogeneityassumption.Inthecross-sectionalcase,wedidnotexplicitlystatehowtheerrortermfor,say,personi,ui,isrelatedtotheexplanatoryvariablesforotherpeopleinthesample.Thereasonthiswasunnecessaryisthat,withrandomsampling(AssumptionMLR.2),uiisautomaticallyindependentoftheexplanatoryvariablesforobservationsotherthani.Inatimeseriescontext,randomsamplingisalmostneverappropriate,sowemustexplic-itlyassumethattheexpectedvalueofutisnotrelatedtotheexplanatoryvariablesinanytimeperiods.ItisimportanttoseethatAssumptionTS.2putsnorestrictiononcorrelationintheindependentvariablesorintheutacrosstime.AssumptionTS.2onlysaysthattheaver-agevalueofutisunrelatedtotheindependentvariablesinalltimeperiods.AnythingthatcausestheunobservablesattimettobecorrelatedwithanyoftheexplanatoryvariablesinanytimeperiodcausesAssumptionTS.2tofail.Twoleadingcandidatesforfailureareomittedvariablesandmeasurementerrorinsomeoftheregressors.But,thestrictexogeneityassumptioncanalsofailforother,lessobviousreasons.Inthesimplestaticregressionmodelyt01ztut,AssumptionTS.2requiresnotonlythatutandztareuncorrelated,butthatutisalsouncorrelatedwithpastandfuturevaluesofz.Thishastwoimplications.First,zcanhavenolaggedeffectony.Ifzdoeshavealaggedeffectony,thenweshouldestimateadistributedlagmodel.Amoresubtlepointisthatstrictexogeneityexcludesthepos-318\nChapter10BasicRegressionAnalysiswithTimeSeriesDatasibilitythatchangesintheerrortermtodaycancausefuturechangesinz.Thiseffec-tivelyrulesoutfeedbackfromyonfuturevaluesofz.Forexample,considerasimplestaticmodeltoexplainacity’smurderrateintermsofpoliceofficerspercapita:mrdrtet01polpctut.Itmaybereasonabletoassumethatutisuncorrelatedwithpolpctandevenwithpastvaluesofpolpct;forthesakeofargument,assumethisisthecase.Butsupposethatthecityadjuststhesizeofitspoliceforcebasedonpastvaluesofthemurderrate.Thismeansthat,say,polpct1mightbecorrelatedwithut(sinceahigherutleadstoahighermrdrtet).Ifthisisthecase,AssumptionTS.2isgenerallyviolated.Therearesimilarconsiderationsindistributedlagmodels.Usuallywedonotworrythatutmightbecorrelatedwithpastzbecausewearecontrollingforpastzinthemodel.Butfeedbackfromutofuturezisalwaysanissue.Explanatoryvariablesthatarestrictlyexogenouscannotreacttowhathashappenedtoyinthepast.Afactorsuchastheamountofrainfallinanagriculturalproductionfunctionsatisfiesthisrequirement:rainfallinanyfutureyearisnotinfluencedbytheoutputduringthecurrentorpastyears.Butsomethingliketheamountoflaborinputmightnotbestrictlyexogenous,asitischosenbythefarmer,andthefarmermayadjusttheamountoflaborbasedonlastyear’syield.Policyvariables,suchasgrowthinthemoneysupply,expendituresonwelfare,highwayspeedlimitsareofteninfluencedbywhathashappenedtotheoutcomevariableinthepast.Inthesocialsciences,manyexplanatoryvariablesmayverywellviolatethestrictexogeneityassumption.EventhoughAssumptionTS.2canbeunrealistic,webeginwithitinordertoconcludethattheOLSestimatorsareunbiased.MosttreatmentsofstaticandfinitedistributedlagmodelsassumeTS.2bymakingthestrongerassumptionthattheexplanatoryvariablesarenonrandom,orfixedinrepeatedsamples.Thenonrandomnessassumptionisobviouslyfalsefortimeseriesobservations;AssumptionTS.2hastheadvantageofbeingmorereal-isticabouttherandomnatureofthextj,whileitisolatesthenecessaryassumptionabouthowutandtheexplanatoryvariablesarerelatedinorderforOLStobeunbiased.ThelastassumptionneededforunbiasednessofOLSisthestandardnoperfectcollinearityassumption.ASSUMPTIONTS.3(NOPERFECTCOLLINEARITY)Inthesample(andthereforeintheunderlyingtimeseriesprocess),noindependentvariableisconstantoraperfectlinearcombinationoftheothers.Wediscussedthisassumptionatlengthinthecontextofcross-sectionaldatainChapter3.Theissuesareessentiallythesamewithtimeseriesdata.Remember,AssumptionTS.3doesallowtheexplanatoryvariablestobecorrelated,butitrulesoutperfectcorrelationinthesample.THEOREM10.1(UNBIASEDNESSOFOLS)UnderAssumptionsTS.1,TS.2,andTS.3,theOLSestimatorsareunbiasedconditionalonX,andthereforeunconditionallyaswell:E(ˆj)j,j0,1,…,k.319\nPart2RegressionAnalysiswithTimeSeriesDataTheproofofthistheoremisessentiallytheQUESTION10.2sameasthatforTheorem3.1inChapter3,IntheFDLmodelyt00zt1zt1ut,whatdoweneedandsoweomitit.Whencomparingtoassumeaboutthesequence{z0,z1,…,zn}inorderforAs-Theorem10.1toTheorem3.1,wehavesumptionTS.3tohold?beenabletodroptherandomsamplingassumptionbyassumingthat,foreacht,uthaszeromeangiventheexplanatoryvariablesatalltimeperiods.Ifthisassumptiondoesnothold,OLScannotbeshowntobeunbiased.Theanalysisofomittedvariablesbias,whichwecoveredinSection3.3,isessen-tiallythesameinthetimeseriescase.Inparticular,Table3.2andthediscussionsur-roundingitcanbeusedasbeforetodeterminethedirectionsofbiasduetoomittedvariables.TheVariancesoftheOLSEstimatorsandtheGauss-MarkovTheoremWeneedtoaddtwoassumptionstoroundouttheGauss-Markovassumptionsfortimeseriesregressions.Thefirstoneisfamiliarfromcross-sectionalanalysis.ASSUMPTIONTS.4(HOMOSKEDASTICITY)2ConditionalonX,thevarianceofutisthesameforallt:Var(utX)Var(ut),t1,2,…,n.ThisassumptionmeansthatVar(utX)cannotdependonX—itissufficientthatutandXareindependent—andthatVar(ut)mustbeconstantovertime.WhenTS.4doesnothold,wesaythattheerrorsareheteroskedastic,justasinthecross-sectionalcase.Forexam-ple,consideranequationfordeterminingthree-month,T-billrates(i3t)basedontheinflationrate(inft)andthefederaldeficitasapercentageofgrossdomesticproduct(deft):i3t01inft2deftut.(10.11)Amongotherthings,AssumptionTS.4requiresthattheunobservablesaffectinginter-estrateshaveaconstantvarianceovertime.Sincepolicyregimechangesareknowntoaffectthevariabilityofinterestrates,thisassumptionmightverywellbefalse.Further,itcouldbethatthevariabilityininterestratesdependsonthelevelofinflationorrela-tivesizeofthedeficit.Thiswouldalsoviolatethehomoskedasticityassumption.WhenVar(utX)doesdependonX,itoftendependsontheexplanatoryvariablesattimet,xt.InChapter12,wewillseethatthetestsforheteroskedasticityfromChapter8canalsobeusedfortimeseriesregressions,atleastundercertainassumptions.ThefinalGauss-Markovassumptionfortimeseriesanalysisisnew.ASSUMPTIONTS.5(NOSERIALCORRELATION)ConditionalonX,theerrorsintwodifferenttimeperiodsareuncorrelated:Corr(ut,usX)0,forallts.320\nChapter10BasicRegressionAnalysiswithTimeSeriesDataTheeasiestwaytothinkofthisassumptionistoignoretheconditioningonX.Then,AssumptionTS.5issimplyCorr(ut,us)0,forallts.(10.12)(ThisishowthenoserialcorrelationassumptionisstatedwhenXistreatedasnonran-dom.)WhenconsideringwhetherAssumptionTS.5islikelytohold,wefocusonequa-tion(10.12)becauseofitssimpleinterpretation.When(10.12)isfalse,wesaythattheerrorsin(10.8)sufferfromserialcorrela-tion,orautocorrelation,becausetheyarecorrelatedacrosstime.Considerthecaseoferrorsfromadjacenttimeperiods.Supposethat,whenut10then,onaverage,theerrorinthenexttimeperiod,ut,isalsopositive.ThenCorr(ut,ut1)0,andtheerrorssufferfromserialcorrelation.Inequation(10.11)thismeansthat,ifinterestratesareunexpectedlyhighforthisperiod,thentheyarelikelytobeaboveaverage(forthegivenlevelsofinflationanddeficits)forthenextperiod.Thisturnsouttobeareasonablecharacterizationfortheerrortermsinmanytimeseriesapplications,whichwewillseeinChapter12.Fornow,weassumeTS.5.Importantly,AssumptionTS.5assumesnothingabouttemporalcorrelationintheindependentvariables.Forexample,inequation(10.11),inftisalmostcertainlycorre-latedacrosstime.ButthishasnothingtodowithwhetherTS.5holds.Anaturalquestionthatarisesis:InChapters3and4,whydidwenotassumethattheerrorsfordifferentcross-sectionalobservationsareuncorrelated?Theanswercomesfromtherandomsamplingassumption:underrandomsampling,uianduhareindependentforanytwoobservationsiandh.Itcanalsobeshownthatthisistrue,con-ditionalonallexplanatoryvariablesinthesample.Thus,forourpurposes,serialcorre-lationisonlyanissueintimeseriesregressions.AssumptionsTS.1throughTS.5aretheappropriateGauss-Markovassumptionsfortimeseriesapplications,buttheyhaveotherusesaswell.Sometimes,TS.1throughTS.5aresatisfiedincross-sectionalapplications,evenwhenrandomsamplingisnotareasonableassumption,suchaswhenthecross-sectionalunitsarelargerelativetothepopulation.Itispossiblethatcorrelationexists,say,acrosscitieswithinastate,butaslongastheerrorsareuncorrelatedacrossthosecities,AssumptionTS.5holds.Butweareprimarilyinterestedinapplyingtheseassumptionstoregressionmodelswithtimeseriesdata.THEOREM10.2(OLSSAMPLINGVARIANCES)UnderthetimeseriesGauss-MarkovassumptionsTS.1throughTS.5,thevarianceofˆj,conditionalonX,is22Var(ˆjX)/[SSTj(1Rj)],j1,…,k,(10.13)2whereSSTjisthetotalsumofsquaresofxtjandRjistheR-squaredfromtheregressionofxjontheotherindependentvariables.321\nPart2RegressionAnalysiswithTimeSeriesDataEquation(10.13)istheexactvariancewederivedinChapter3underthecross-sectionalGauss-Markovassumptions.SincetheproofisverysimilartotheoneforTheorem3.2,weomitit.ThediscussionfromChapter3aboutthefactorscausinglargevariances,includingmulticollinearityamongtheexplanatoryvariables,appliesimme-diatelytothetimeseriescase.TheusualestimatoroftheerrorvarianceisalsounbiasedunderAssumptionsTS.1throughTS.5,andtheGauss-Markovtheoremholds.THEOREM10.3(UNBIASEDESTIMATIONOF2)2UnderAssumptionsTS.1throughTS.5,theestimatorˆSSR/dfisanunbiasedestimator2of,wheredfnk1.THEOREM10.4(GAUSS-MARKOVTHEOREM)UnderAssumptionsTS.1throughTS.5,theOLSestimatorsarethebestlinearunbiasedesti-matorsconditionalonX.ThebottomlinehereisthatOLShasQUESTION10.3thesamedesirablefinitesamplepropertiesIntheFDLmodelyt00zt1zt1ut,explainthenatureunderTS.1throughTS.5thatithasunderofanymulticollinearityintheexplanatoryvariables.MLR.1throughMLR.5.InferenceUndertheClassicalLinearModelAssumptionsInordertousetheusualOLSstandarderrors,tstatistics,andFstatistics,weneedtoaddafinalassumptionthatisanalogoustothenormalityassumptionweusedforcross-sectionalanalysis.ASSUMPTIONTS.6(NORMALITY)TheerrorsutareindependentofXandareindependentlyandidenticallydistributedas2Normal(0,).AssumptionTS.6impliesTS.3,TS.4,andTS.5,butitisstrongerbecauseoftheindependenceandnormalityassumptions.THEOREM10.5(NORMALSAMPLINGDISTRIBUTIONS)UnderAssumptionsTS.1throughTS.6,theCLMassumptionsfortimeseries,theOLSesti-matorsarenormallydistributed,conditionalonX.Further,underthenullhypothesis,eachtstatistichasatdistribution,andeachFstatistichasanFdistribution.Theusualconstruc-tionofconfidenceintervalsisalsovalid.322\nChapter10BasicRegressionAnalysiswithTimeSeriesDataTheimplicationsofTheorem10.5areofutmostimportance.Itimpliesthat,whenAssumptionsTS.1throughTS.6hold,everythingwehavelearnedaboutestimationandinferenceforcross-sectionalregressionsappliesdirectlytotimeseriesregressions.Thus,tstatisticscanbeusedfortestingstatisticalsignificanceofindividualexplanatoryvariables,andFstatisticscanbeusedtotestforjointsignificance.Justasinthecross-sectionalcase,theusualinferenceproceduresareonlyasgoodastheunderlyingassumptions.Theclassicallinearmodelassumptionsfortimeseriesdataaremuchmorerestrictivethanthoseforthecross-sectionaldata—inparticular,thestrictexogeneityandnoserialcorrelationassumptionscanbeunrealistic.Nevertheless,theCLMframeworkisagoodstartingpointformanyapplications.EXAMPLE10.1(StaticPhillipsCurve)Todeterminewhetherthereisatradeoff,onaverage,betweenunemploymentandinfla-tion,wecantestH0:10againstH0:10inequation(10.2).Iftheclassicallinearmodelassumptionshold,wecanusetheusualOLStstatistic.UsingannualdatafortheUnitedStatesinPHILLIPS.RAW,fortheyears1948through1996,weobtaininˆft(1.42)(.468)unemtinˆft(1.72)(.289)unemt(10.14)22n49,R.053,R¯.033.Thisequationdoesnotsuggestatradeoffbetweenunemandinf:ˆ10.Thetstatisticforˆ1isabout1.62,whichgivesap-valueagainstatwo-sidedalternativeofabout.11.Thus,ifanything,thereisapositiverelationshipbetweeninflationandunemployment.Therearesomeproblemswiththisanalysisthatwecannotaddressindetailnow.InChapter12,wewillseethattheCLMassumptionsdonothold.Inaddition,thestaticPhillipscurveisprobablynotthebestmodelfordeterminingwhetherthereisashort-runtradeoffbetweeninflationandunemployment.MacroeconomistsgenerallyprefertheexpectationsaugmentedPhillipscurve,asimpleexampleofwhichisgiveninChapter11.Asasecondexample,weestimateequation(10.11)usinganualdataontheU.S.economy.EXAMPLE10.2(EffectsofInflationandDeficitsonInterestRates)ThedatainINTDEF.RAWcomefromthe1997EconomicReportofthePresidentandspantheyears1948through1996.Thevariablei3isthethree-monthT-billrate,infistheannualinflationratebasedontheconsumerpriceindex(CPI),anddefisthefederalbudgetdeficitasapercentageofGDP.Theestimatedequationis323\nPart2RegressionAnalysiswithTimeSeriesDatai3ˆt(1.25)(.613)inft(.700)defti3ˆt(0.44)(.076)inft(.118)deft(10.15)22n49,R.697,R¯.683.Theseestimatesshowthatincreasesininflationandtherelativesizeofthedeficitworktogethertoincreaseshort-terminterestrates,bothofwhichareexpectedfrombasiceco-nomics.Forexample,aceterisparibusonepercentagepointincreaseintheinflationrateincreasesi3by.613points.Bothinfanddefareverystatisticallysignificant,assuming,ofcourse,thattheCLMassumptionshold.10.4FUNCTIONALFORM,DUMMYVARIABLES,ANDINDEXNUMBERSAllofthefunctionalformswelearnedaboutinearlierchapterscanbeusedintimeseriesregressions.Themostimportantoftheseisthenaturallogarithm:timeseriesregressionswithconstantpercentageeffectsappearofteninappliedwork.EXAMPLE10.3(PuertoRicanEmploymentandtheMinimumWage)AnnualdataonthePuertoRicanemploymentrate,minimumwage,andothervariablesareusedbyCastillo-FreedmanandFreedman(1992)tostudytheeffectsoftheU.S.minimumwageonemploymentinPuertoRico.Asimplifiedversionoftheirmodelislog(prepopt)01log(mincovt)2log(usgnpt)ut,(10.16)whereprepoptistheemploymentrateinPuertoRicoduringyeart(ratioofthoseworkingtototalpopulation),usgnptisrealU.S.grossnationalproduct(inbillionsofdollars),andmincovmeasurestheimportanceoftheminimumwagerelativetoaveragewages.Inpar-ticular,mincov(avgmin/avgwage)avgcov,whereavgministheaverageminimumwage,avgwageistheaverageoverallwage,andavgcovistheaveragecoveragerate(thepro-portionofworkersactuallycoveredbytheminimumwagelaw).Usingdatafortheyears1950through1987gives(log(preˆpopt)1.05)(.154)log(mincovt)(.012)log(usgnpt)log(preˆpopt)(0.77)(.065)log(mincovt)(.089)log(usgnpt)(10.17)22n38,R.661,R¯.641.Theestimatedelasticityofprepopwithrespecttomincovis.154,anditisstatisticallysig-nificantwitht2.37.Therefore,ahigherminimumwagelowerstheemploymentrate,somethingthatclassicaleconomicspredicts.TheGNPvariableisnotstatisticallysignificant,butthischangeswhenweaccountforatimetrendinthenextsection.324\nChapter10BasicRegressionAnalysiswithTimeSeriesDataWecanuselogarithmicfunctionalformsindistributedlagmodels,too.Forexam-ple,forquarterlydata,supposethatmoneydemand(Mt)andgrossdomesticproduct(GDPt)arerelatedbylog(Mt)00log(GDPt)1log(GDPt1)2log(GDPt2)3log(GDPt3)4log(GDPt4)ut.Theimpactpropensityinthisequation,0,isalsocalledtheshort-runelasticity:itmeasurestheimmediatepercentagechangeinmoneydemandgivena1%increaseinGDP.Thelong-runpropensity,01…4,issometimescalledthelong-runelasticity:itmeasuresthepercentageincreaseinmoneydemandafterfourquartersgivenapermanent1%increaseinGDP.Binaryordummyindependentvariablesarealsoquiteusefulintimeseriesappli-cations.Sincetheunitofobservationistime,adummyvariablerepresentswhether,ineachtimeperiod,acertaineventhasoccurred.Forexample,forannualdata,wecanindicateineachyearwhetheraDemocratoraRepublicanispresidentoftheUnitedStatesbydefiningavariabledemoct,whichisunityifthepresidentisaDemocrat,andzerootherwise.Or,inlookingattheeffectsofcapitalpunishmentonmurderratesinTexas,wecandefineadummyvariableforeachyearequaltooneifTexashadcapitalpunishmentduringthatyear,andzerootherwise.Oftendummyvariablesareusedtoisolatecertainperiodsthatmaybesystemati-callydifferentfromotherperiodscoveredbyadataset.EXAMPLE10.4(EffectsofPersonalExemptiononFertilityRates)Thegeneralfertilityrate(gfr)isthenumberofchildrenborntoevery1,000womenofchild-bearingage.Fortheyears1913through1984,theequation,gfrt01pet2ww2t3pilltut,explainsgfrintermsoftheaveragerealdollarvalueofthepersonaltaxexemption(pe)andtwobinaryvariables.Thevariableww2takesonthevalueunityduringtheyears1941through1945,whentheUnitedStateswasinvolvedinWorldWarII.Thevariablepillisunityfrom1963on,whenthebirthcontrolpillwasmadeavailableforcontraception.UsingthedatainFERTIL3.RAW,whichweretakenfromthearticlebyWhittington,Alm,andPeters(1990),givesgfˆrt(98.68)(.083)pet(24.24)ww2t(31.59)pilltgfˆrt9(3.21)(.030)pet2(7.46)ww2t3(4.08)pillt(10.18)22n72,R.473,R¯.450.Eachvariableisstatisticallysignificantatthe1%levelagainstatwo-sidedalternative.WeseethatthefertilityratewaslowerduringWorldWarII:givenpe,therewereabout24fewerbirthsforevery1,000womenofchildbearingage,whichisalargereduction.(From1913through1984,gfrrangedfromabout65to127.)Similarly,thefertilityratehasbeensubstantiallylowersincetheintroductionofthebirthcontrolpill.325\nPart2RegressionAnalysiswithTimeSeriesDataThevariableofeconomicinterestispe.Theaveragepeoverthistimeperiodis$100.40,rangingfromzeroto$243.83.Thecoefficientonpeimpliesthata12-dollarincreaseinpeincreasesgfrbyaboutonebirthper1,000womenofchildbearingage.Thiseffectishardlytrivial.InSection10.2,wenotedthatthefertilityratemayreacttochangesinpewithalag.Estimatingadistributedlagmodelwithtwolagsgivesgfˆrt(95.87)(.073)pet(.0058)pet1(.034)pet2gfˆrt9(3.28)(.126)pet(.1557)pet1(.126)pet2(22.13)ww2t(31.30)pillt(10.19)(10.73)ww2t0(3.98)pillt22n70,R.499,R¯.459.Inthisregression,weonlyhave70observationsbecausewelosetwowhenwelagpetwice.Thecoefficientsonthepevariablesareestimatedveryimprecisely,andeachoneisindividuallyinsignificant.Itturnsoutthatthereissubstantialcorrelationbetweenpet,pet1,andpet2,andthismulticollinearitymakesitdifficulttoestimatetheeffectateachlag.However,pet,pet1,andpet2arejointlysignificant:theFstatistichasap-value.012.Thus,pedoeshaveaneffectongfr[aswealreadysawin(10.18)],butwedonothavegoodenoughestimatestodeterminewhetheritiscontemporaneousorwithaone-ortwo-yearlag(orsomeofeach).Actually,pet1andpet2arejointlyinsignificantinthisequation(p-value.95),soatthispoint,wewouldbejustifiedinusingthestaticmodel.Butforillustrativepurposes,letusobtainaconfidenceintervalforthelong-runpropensityinthismodel.TheestimatedLRPin(10.19)is.073.0058.034.101.However,wedonothaveenoughinformationin(10.19)toobtainthestandarderrorofthisestimate.ToobtainthestandarderroroftheestimatedLRP,weusethetricksuggestedinSection4.4.Let0012denotetheLRPandwrite0intermsof0,1,and2as0012.Next,substitutefor0inthemodelgfrt00pet1pet12pet2…togetgfrt0(012)pet1pet12pet2…00pet1(pet1pet)2(pet2pet)….Fromthislastequation,wecanobtainˆ0anditsstandarderrorbyregressinggfrtonpet,(pet1pet),(pet2pet),ww2t,andpillt.Thecoefficientandassociatedstandarderroronpetarewhatweneed.Runningthisregressiongivesˆ0.101asthecoefficientonpet(aswealreadyknewfromabove)andse(ˆ0).030[whichwecouldnotcomputefrom(10.19)].Therefore,thetstatisticforˆ0isabout3.37,soˆ0isstatisticallydifferentfromzeroatsmallsignificancelevels.Eventhoughnoneoftheˆjisindividuallysignificant,theLRPisverysignificant.The95%confidenceintervalfortheLRPisabout.041to.160.Whittington,Alm,andPeters(1990)allowforfurtherlagsbutrestrictthecoefficientstohelpalleviatethemulticollinearityproblemthathindersestimationoftheindividualj.(SeeProblem10.6foranexampleofhowtodothis.)ForestimatingtheLRP,whichwould326\nChapter10BasicRegressionAnalysiswithTimeSeriesDataseemtobeofprimaryinteresthere,suchrestrictionsareunnecessary.Whittington,Alm,andPetersalsocontrolforadditionalvariables,suchasaveragefemalewageandtheunemploymentrate.Binaryexplanatoryvariablesarethekeycomponentinwhatiscalledaneventstudy.Inaneventstudy,thegoalistoseewhetheraparticulareventinfluencessomeoutcome.Economistswhostudyindustrialorganizationhavelookedattheeffectsofcertaineventsonfirmstockprices.Forexample,Rose(1985)studiedtheeffectsofnewtruckingregulationsonthestockpricesoftruckingcompanies.AsimpleversionofanequationusedforsucheventstudiesisfmRt01Rt2dtut,fmwhereRtisthestockreturnforfirmfduringperiodt(usuallyaweekoramonth),Rtisthemarketreturn(usuallycomputedforabroadstockmarketindex),anddtisadummyvariableindicatingwhentheeventoccurred.Forexample,ifthefirmisanair-line,dtmightdenotewhethertheairlineexperiencedapublicizedaccidentornearacci-mdentduringweekt.IncludingRtintheequationcontrolsforthepossibilitythatbroadmarketmovementsmightcoincidewithairlineaccidents.Sometimes,multipledummyvariablesareused.Forexample,iftheeventistheimpositionofanewregulationthatmightaffectacertainfirm,wemightincludeadummyvariablethatisoneforafewweeksbeforetheregulationwaspubliclyannouncedandaseconddummyvariableforafewweeksaftertheregulationwasannounced.Thefirstdummyvariablemightdetectthepresenceofinsideinformation.Beforewegiveanexampleofaneventstudy,weneedtodiscussthenotionofanindexnumberandthedifferencebetweennominalandrealeconomicvariables.Anindexnumbertypicallyaggregatesavastamountofinformationintoasinglequantity.Indexnumbersareusedregularlyintimeseriesanalysis,especiallyinmacroeconomicapplications.Anexampleofanindexnumberistheindexofindustrialproduction(IIP),computedmonthlybytheBoardofGovernorsoftheFederalReserve.TheIIPisamea-sureofproductionacrossabroadrangeofindustries,and,assuch,itsmagnitudeinaparticularyearhasnoquantitativemeaning.InordertointerpretthemagnitudeoftheIIP,wemustknowthebaseperiodandthebasevalue.Inthe1997EconomicReportofthePresident(ERP),thebaseyearis1987,andthebasevalueis100.(SettingIIPto100inthebaseperiodisjustaconvention;itmakesjustasmuchsensetosetIIP1in1987,andsomeindexesaredefinedwithoneasthebasevalue.)BecausetheIIPwas107.7in1992,wecansaythatindustrialproductionwas7.7%higherin1992thanin1987.WecanusetheIIPinanytwoyearstocomputethepercentagedifferenceinindustrialoutputduringthosetwoyears.Forexample,sinceIIP61.4in1970andIIP85.7in1979,industrialproductiongrewbyabout39.6%duringthe1970s.Itiseasytochangethebaseperiodforanyindexnumber,andsometimeswemustdothistogiveindexnumbersreportedwithdifferentbaseyearsacommonbaseyear.Forexample,ifwewanttochangethebaseyearoftheIIPfrom1987to1982,wesim-plydividetheIIPforeachyearbythe1982valueandthenmultiplyby100tomakethebaseperiodvalue100.Generally,theformulais327\nPart2RegressionAnalysiswithTimeSeriesDatanewindext100(oldindext/oldindexnewbase),(10.20)whereoldindexnewbaseistheoriginalvalueoftheindexinthenewbaseyear.Forexam-ple,withbaseyear1987,theIIPin1992is107.7;ifwechangethebaseyearto1982,theIIPin1992becomes100(107.7/81.9)131.5(becausetheIIPin1982was81.9).Anotherimportantexampleofanindexnumberisapriceindex,suchastheconsumerpriceindex(CPI).WealreadyusedtheCPItocomputeannualinflationratesinExample10.1.Aswiththeindustrialproductionindex,theCPIisonlymeaningfulwhenwecom-pareitacrossdifferentyears(ormonths,ifweareusingmonthlydata).Inthe1997ERP,CPI38.8in1970,andCPI130.7in1990.Thus,thegeneralpricelevelgrewbyalmost237%overthistwenty-yearperiod.(In1997,theCPIisdefinedsothatitsaveragein1982,1983,and1984equals100;thus,thebaseperiodislistedas1982–1984.)Inadditiontobeingusedtocomputeinflationrates,priceindexesarenecessaryforturningatimeseriesmeasuredinnominaldollars(orcurrentdollars)intorealdollars(orconstantdollars).Mosteconomicbehaviorisassumedtobeinfluencedbyreal,notnominal,variables.Forexample,classicallaboreconomicsassumesthatlaborsupplyisbasedontherealhourlywage,notthenominalwage.ObtainingtherealwagefromthenominalwageiseasyifwehaveapriceindexsuchastheCPI.WemustbealittlecarefultofirstdividetheCPIby100,sothatthevalueinthebaseyearisone.Then,ifwdenotestheaveragehourlywageinnominaldollarsandpCPI/100,therealwageissimplyw/p.ThiswageismeasuredindollarsforthebaseperiodoftheCPI.Forexample,inTableB-45inthe1997ERP,averagehourlyearningsarereportedinnom-inaltermsandin1982dollars(whichmeansthattheCPIusedincomputingtherealwagehadthebaseyear1982).Thistablereportsthatthenominalhourlywagein1960was$2.09,butmeasuredin1982dollars,thewagewas$6.79.Therealhourlywagehadpeakedin1973,at$8.55in1982dollars,andhadfallento$7.40by1995.Thus,therehasbeenanontrivialdeclineinrealwagesoverthepast20years.(Ifwecomparenom-inalwagesfrom1973and1995,wegetaverymisleadingpicture:$3.94in1973and$11.44in1995.Sincetherealwagehasactuallyfallen,theincreaseinthenominalwageisdueentirelytoinflation.)Standardmeasuresofeconomicoutputareinrealterms.Themostimportantoftheseisgrossdomesticproduct,orGDP.WhengrowthinGDPisreportedinthepop-ularpress,itisalwaysrealGDPgrowth.Inthe1997ERP,TableB-9,GDPisreportedinbillionsof1992dollars.Weusedasimilarmeasureofoutput,realgrossnationalproduct,inExample10.3.Interestingthingshappenwhenrealdollarvariablesareusedincombinationwithnaturallogarithms.Suppose,forexample,thataverageweeklyhoursworkedarerelatedtotherealwageaslog(hours)01log(w/p)u.Usingthefactthatlog(w/p)log(w)log(p),wecanwritethisaslog(hours)01log(w)2log(p)u,(10.21)butwiththerestrictionthat21.Therefore,theassumptionthatonlytherealwageinfluenceslaborsupplyimposesarestrictionontheparametersofmodel(10.21).328\nChapter10BasicRegressionAnalysiswithTimeSeriesDataIf21,thenthepricelevelhasaneffectonlaborsupply,somethingthatcanhap-penifworkersdonotfullyunderstandthedistinctionbetweenrealandnominalwages.Therearemanypracticalaspectstotheactualcomputationofindexnumbers,butitwouldtakeustoofarafieldtocoverthosehere.Detaileddiscussionsofpriceindexescanbefoundinmostintermediatemacroeconomictexts,suchasMankiw(1994,Chapter2).Forus,itisimportanttobeabletouseindexnumbersinregressionanaly-sis.Asmentionedearlier,sincethemagnitudesofindexnumbersarenotespeciallyinformative,theyoftenappearinlogarithmicform,sothatregressioncoefficientshavepercentagechangeinterpretations.Wenowgiveanexampleofaneventstudythatalsousesindexnumbers.EXAMPLE10.5(AntidumpingFilingsandChemicalImports)KruppandPollard(1996)analyzedtheeffectsofantidumpingfilingsbyU.S.chemicalindustriesonimportsofvariouschemicals.Wefocushereononeindustrialchemical,bar-iumchloride,acleaningagentusedinvariouschemicalprocessesandingasolineproduc-tion.Intheearly1980s,U.S.bariumchlorideproducersbelievedthatChinawasofferingitsU.S.importsatanunfairlylowprice(anactionknownasdumping),andthebariumchlo-rideindustryfiledacomplaintwiththeU.S.InternationalTradeCommission(ITC)inOctober1983.TheITCruledinfavoroftheU.S.bariumchlorideindustryinOctober1984.Thereareseveralquestionsofinterestinthiscase,butwewilltouchononlyafewofthem.First,areimportsunusuallyhighintheperiodimmediatelyprecedingtheinitialfiling?Second,doimportschangenoticeablyafteranantidumpingfiling?Finally,whatisthereductioninimportsafteradecisioninfavoroftheU.S.industry?Toanswerthesequestions,wefollowKruppandPollardbydefiningthreedummyvari-ables:befile6isequaltooneduringthesixmonthsbeforefiling,affile6indicatesthesixmonthsafterfiling,andafdec6denotesthesixmonthsafterthepositivedecision.ThedependentvariableisthevolumeofimportsofbariumchloridefromChina,chnimp,whichweuseinlogarithmicform.Weincludeasexplanatoryvariables,allinlogarithmicform,anindexofchemicalproduction,chempi(tocontrolforoveralldemandforbariumchloride),thevolumeofgasolineproduction,gas(anotherdemandvariable),andanexchangerateindex,rtwex,whichmeasuresthestrengthofthedollaragainstseveralothercurrencies.Thechemicalproductionindexwasdefinedtobe100inJune1977.Theanalysisheredif-ferssomewhatfromKruppandPollardinthatweusenaturallogarithmsofallvariables(exceptthedummyvariables,ofcourse),andweincludeallthreedummyvariablesinthesameregression.UsingmonthlydatafromFebruary1978throughDecember1988givesthefollowing:log(chˆnimp)17.80)(3.12)log(chempi)(.196)log(gas)og(chˆnimp)(21.05)(0.48)log(chempi)(.907)log(gas)(.983)log(rtwex)(.060)befile6(.032)affile6(.566)afdec6(10.22)(.400)log(rtwex)(.261)befile6(.264)affile6(.286)afdec622n131,R.305,R¯.271.329\nPart2RegressionAnalysiswithTimeSeriesDataTheequationshowsthatbefile6isstatisticallyinsignificant,sothereisnoevidencethatChineseimportswereunusuallyhighduringthesixmonthsbeforethesuitwasfiled.Further,althoughtheestimateonaffile6isnegative,thecoefficientissmall(indicatingabouta3.2%fallinChineseimports),anditisstatisticallyveryinsignificant.Thecoefficientonafdec6showsasubstantialfallinChineseimportsofbariumchlorideafterthedecisioninfavoroftheU.S.industry,whichisnotsurprising.Sincetheeffectissolarge,wecom-putetheexactpercentagechange:100[exp(.566)1]43.2%.Thecoefficientissta-tisticallysignificantatthe5%levelagainstatwo-sidedalternative.Thecoefficientsignsonthecontrolvariablesarewhatweexpect:anincreaseinover-allchemicalproductionincreasesthedemandforthecleaningagent.GasolineproductiondoesnotaffectChineseimportssignificantly.Thecoefficientonlog(rtwex)showsthatanincreaseinthevalueofthedollarrelativetoothercurrenciesincreasesthedemandforChineseimports,asispredictedbyeconomictheory.(Infact,theelasticityisnotstatisticallydifferentfromone.Why?)Interactionsamongqualitativeandquantitativevariablesarealsousedintimeseriesanalysis.Anexamplewithpracticalimportancefollows.EXAMPLE10.6(ElectionOutcomesandEconomicPerformance)Fair(1996)summarizeshisworkonexplainingpresidentialelectionoutcomesintermsofeconomicperformance.Heexplainstheproportionofthetwo-partyvotegoingtotheDemocraticcandidateusingdatafortheyears1916through1992(everyfouryears)foratotalof20observations.WeestimateasimplifiedversionofFair’smodel(usingvariablenamesthataremoredescriptivethanhis):demvote01partyWH2incum3partyWHgnews4partyWHinfu,wheredemvoteistheproportionofthetwo-partyvotegoingtotheDemocraticcandidate.TheexplanatoryvariablepartyWHissimilartoadummyvariable,butittakesonthevalueoneifaDemocratisintheWhiteHouseand1ifaRepublicanisintheWhiteHouse.FairusesthisvariabletoimposetherestrictionthattheeffectofaRepublicanbeingintheWhiteHousehasthesamemagnitudebutoppositesignasaDemocratbeingintheWhiteHouse.Thisisanaturalrestrictionsincethepartysharesmustsumtoone,bydefinition.Italsosavestwodegreesoffreedom,whichisimportantwithsofewobservations.Similarly,thevariableincumisdefinedtobeoneifaDemocraticincumbentisrunning,1ifaRepublicanincumbentisrunning,andzerootherwise.Thevariablegnewsisthenumberofquartersduringthecurrentadministration’sfirst15(outof16total),wherethequarterlygrowthinrealpercapitaoutputwasabove2.9%(atanannualrate),andinfistheaver-ageannualinflationrateoverthefirst15quartersoftheadministration.SeeFair(1996)forprecisedefinitions.EconomistsaremostinterestedintheinteractiontermspartyWHgnewsandpartyWHinf.SincepartyWHequalsonewhenaDemocratisintheWhiteHouse,3mea-surestheeffectofgoodeconomicnewsonthepartyinpower;weexpect30.Similarly,330\nChapter10BasicRegressionAnalysiswithTimeSeriesData4measurestheeffectthatinflationhasonthepartyinpower.Becauseinflationduringanadministrationisconsideredtobebadnews,weexpect40.TheestimatedequationusingthedatainFAIR.RAWisdemvˆote(.481)(.0435)partyWH(.0544)incumdemvˆote(.012)(.0405)partyWH(.0234)incum(.0108)partyWHgnews(.0077)partyWHinf(10.23)(.0041)partyWHgnews(.0033)partyWHinf22n20,R.663,R¯.573.Allcoefficients,exceptthatonpartyWH,arestatisticallysignificantatthe5%level.Incumbencyisworthabout5.4percentagepointsintheshareofthevote.(Remember,demvoteismeasuredasaproportion.)Further,theeconomicnewsvariablehasapositiveeffect:onemorequarterofgoodnewsisworthabout1.1percentagepoints.Inflation,asexpected,hasanegativeeffect:ifaverageannualinflationis,say,twopercentagepointshigher,thepartyinpowerlosesabout1.5percentagepointsofthetwo-partyvote.Wecouldhaveusedthisequationtopredicttheoutcomeofthe1996presidentialelec-tionbetweenBillClinton,theDemocrat,andBobDole,theRepublican.(Theindependentcandidate,RossPerot,isexcludedbecauseFair’sequationisforthetwo-partyvoteonly.)SinceClintonranasanincumbent,partyWH1andincum1.Topredicttheelectionoutcome,weneedthevariablesgnewsandinf.DuringClinton’sfirst15quartersinoffice,percapitarealGDPexceeded2.9%threetimes,sognews3.Further,usingtheGDPpricedeflatorreportedinTableB-4inthe1997ERP,theaverageannualinflationrate(computedusingFair’sformula)fromthefourthquarterin1991tothethirdquarterin1996was3.019.Pluggingtheseinto(10.23)givesdemvˆote.481.0435.0544.0108(3).0077(3.019).5011.Therefore,basedoninformationknownbeforetheelectioninNovember,Clintonwaspre-dictedtoreceiveaveryslightmajorityofthetwo-partyvote:about50.1%.Infact,Clintonwonmorehandily:hisshareofthetwo-partyvotewas54.65%.10.5TRENDSANDSEASONALITYCharacterizingTrendingTimeSeriesManyeconomictimeserieshaveacommontendencyofgrowingovertime.Wemustrecognizethatsomeseriescontainatimetrendinordertodrawcausalinferenceusingtimeseriesdata.Ignoringthefactthattwosequencesaretrendinginthesameoroppo-sitedirectionscanleadustofalselyconcludethatchangesinonevariableareactuallycausedbychangesinanothervariable.Inmanycases,twotimeseriesprocessesappeartobecorrelatedonlybecausetheyarebothtrendingovertimeforreasonsrelatedtootherunobservedfactors.Figure10.2containsaplotoflaborproductivity(outputperhourofwork)intheUnitedStatesfortheyears1947through1987.Thisseriesdisplaysaclearupwardtrend,whichreflectsthefactthatworkershavebecomemoreproductiveovertime.331\nPart2RegressionAnalysiswithTimeSeriesDataFigure10.2OutputperlaborhourintheUnitedStatesduringtheyears1947–1987;1977100.output110perhour8050194719671987yearOtherseries,atleastovercertaintimeperiods,havecleardownwardtrends.Becausepositivetrendsaremorecommon,wewillfocusonthoseduringourdiscussion.Whatkindofstatisticalmodelsadequatelycapturetrendingbehavior?Onepopularformulationistowritetheseries{yt}asyt01tet,t1,2,…,(10.24)where,inthesimplestcase,{et}isanindependent,identicallydistributed(i.i.d.)2sequencewithE(et)0,Var(et)e.Notehowtheparameter1multipliestime,t,resultinginalineartimetrend.Interpreting1in(10.24)issimple:holdingallotherfactors(thoseinet)fixed,1measuresthechangeinytfromoneperiodtothenextduetothepassageoftime:whenet0,ytytyt11.Anotherwaytothinkaboutasequencethathasalineartimetrendisthatitsaver-agevalueisalinearfunctionoftime:E(yt)01t.(10.25)If10,then,onaverage,ytisgrowingovertimeandthereforehasanupwardtrend.If10,thenythasadownwardtrend.Thevaluesofytdonotfallexactlyontheline332\nChapter10BasicRegressionAnalysiswithTimeSeriesDatain(10.25)duetorandomness,buttheQUESTION10.4expectedvaluesareontheline.UnliketheInExample10.4,weusedthegeneralfertilityrateasthedependentmean,thevarianceofytisconstantacrossvariableinafinitedistributedlagmodel.From1950throughthe2time:Var(yt)Var(et)e.mid-1980s,thegfrhasacleardownwardtrend.CanalineartrendIf{et}isani.i.d.sequence,then{yt}iswith10berealisticforallfuturetimeperiods?Explain.anindependent,thoughnotidentically,distributedsequence.Amorerealisticcharacterizationoftrendingtimeseriesallows{et}tobecorrelatedovertime,butthisdoesnotchangetheflavorofalineartimetrend.Infact,whatisimportantforregres-sionanalysisundertheclassicallinearmodelassumptionsisthatE(yt)islinearint.WhenwecoverlargesamplepropertiesofOLSinChapter11,wewillhavetodiscusshowmuchtemporalcorrelationin{et}isallowed.Manyeconomictimeseriesarebetterapproximatedbyanexponentialtrend,whichfollowswhenaserieshasthesameaveragegrowthratefromperiodtoperiod.Figure10.3plotsdataonannualnominalimportsfortheUnitedStatesduringtheyears1948through1995(ERP1997,TableB–101).Intheearlyyears,weseethatthechangeintheimportsovereachyearisrelativelysmall,whereasthechangeincreasesastimepasses.Thisisconsistentwithaconstantaveragegrowthrate:thepercentagechangeisroughlythesameineachperiod.Inpractice,anexponentialtrendinatimeseriesiscapturedbymodelingthenaturallogarithmoftheseriesasalineartrend(assumingthatyt0):Figure10.3NominalU.S.importsduringtheyears1948–1995(inbillionsofU.S.dollars).U.S.750imports4001007194819721995year333\nPart2RegressionAnalysiswithTimeSeriesDatalog(yt)01tet,t1,2,….(10.26)Exponentiatingshowsthatytitselfhasanexponentialtrend:ytexp(01tet).Becausewewillwanttouseexponentiallytrendingtimeseriesinlinearregressionmodels,(10.26)turnsouttobethemostconvenientwayforrepresentingsuchseries.Howdoweinterpret1in(10.26)?Rememberthat,forsmallchanges,log(yt)log(yt)log(yt1)isapproximatelytheproportionatechangeinyt:log(yt)(ytyt1)/yt1.(10.27)Theright-handsideof(10.27)isalsocalledthegrowthrateinyfromperiodt1toperiodt.Toturnthegrowthrateintoapercent,wesimplymultiplyby100.Ifytfollows(10.26),then,takingchangesandsettinget0,log(yt)1,forallt.(10.28)Inotherwords,1isapproximatelytheaverageperperiodgrowthrateinyt.Forexam-ple,iftdenotesyearand1.027,thenytgrowsabout2.7%peryearonaverage.Althoughlinearandexponentialtrendsarethemostcommon,timetrendscanbemorecomplicated.Forexample,insteadofthelineartrendmodelin(10.24),wemighthaveaquadratictimetrend:2yt01t2tet.(10.29)If1and2arepositive,thentheslopeofthetrendisincreasing,asiseasilyseenbycomputingtheapproximateslope(holdingetfixed):yt122t.(10.30)t[Ifyouarefamiliarwithcalculus,yourecognizetheright-handsideof(10.30)asthe2derivativeof01t2twithrespecttot.]If10,but20,thetrendhasahumpshape.Thismaynotbeaverygooddescriptionofcertaintrendingseriesbecauseitrequiresanincreasingtrendtobefollowed,eventually,byadecreasingtrend.Nevertheless,overagiventimespan,itcanbeaflexiblewayofmodelingtimeseriesthathavemorecomplicatedtrendsthaneither(10.24)or(10.26).UsingTrendingVariablesinRegressionAnalysisAccountingforexplainedorexplanatoryvariablesthataretrendingisfairlystraight-forwardinregressionanalysis.First,nothingabouttrendingvariablesnecessarilyvio-latestheclassicallinearmodelassumptions,TS.1throughTS.6.However,wemustbecarefultoallowforthefactthatunobserved,trendingfactorsthataffectytmightalsobecorrelatedwiththeexplanatoryvariables.Ifweignorethispossibility,wemayfindaspuriousrelationshipbetweenytandoneormoreexplanatoryvariables.Thephe-nomenonoffindingarelationshipbetweentwoormoretrendingvariablessimply334\nChapter10BasicRegressionAnalysiswithTimeSeriesDatabecauseeachisgrowingovertimeisanexampleofspuriousregression.Fortunately,addingatimetrendeliminatesthisproblem.Forconcreteness,consideramodelwheretwoobservedfactors,xt1andxt2,affectyt.Inaddition,thereareunobservedfactorsthataresystematicallygrowingorshrink-ingovertime.Amodelthatcapturesthisisyt01xt12xt23tut.(10.31)Thisfitsintothemultiplelinearregressionframeworkwithxt3t.Allowingforthetrendinthisequationexplicitlyrecognizesthatytmaybegrowing(30)orshrink-ing(30)overtimeforreasonsessentiallyunrelatedtoxt1andxt2.If(10.31)satis-fiesassumptionsTS.1,TS.2,andTS.3,thenomittingtfromtheregressionandregressingytonxt1,xt2willgenerallyyieldbiasedestimatorsof1and2:wehaveeffectivelyomittedanimportantvariable,t,fromtheregression.Thisisespeciallytrueifxt1andxt2arethemselvestrending,becausetheycanthenbehighlycorrelatedwitht.Thenextexampleshowshowomittingatimetrendcanresultinspuriousregression.EXAMPLE10.7(HousingInvestmentandPrices)ThedatainHSEINV.RAWareannualobservationsonhousinginvestmentandahousingpriceindexintheUnitedStatesfor1947through1988.Letinvpcdenoterealpercapitahousinginvestment(inthousandsofdollars)andletpricedenoteahousingpriceindex(equaltoonein1982).Asimpleregressioninconstantelasticityform,whichcanbethoughtofasasupplyequationforhousingstock,gives(log(invˆpc).550)(1.241)log(price)log(invˆpc)(.043)(0.382)log(price)(10.32)22n42,R.208,R¯.189.Theelasticityofpercapitainvestmentwithrespecttopriceisverylargeandstatisticallysig-nificant;itisnotstatisticallydifferentfromone.Wemustbecarefulhere.Bothinvpcandpricehaveupwardtrends.Inparticular,ifweregresslog(invpc)ont,weobtainacoefficientonthetrendequalto.0081(standarderror.0018);theregressionoflog(price)ontyieldsatrendcoefficientequalto.0044(standarderror.0004).Whilethestandarderrorsonthetrendcoefficientsarenotnecessarilyreliable—theseregressionstendtocontainsub-stantialserialcorrelation—thecoefficientestimatesdorevealupwardtrends.Toaccountforthetrendingbehaviorofthevariables,weaddatimetrend:log(invˆpc).913)(.381)log(price)(.0098)tlog(invˆpc)(.136)(.679)log(price)(.0035)t(10.33)22n42,R.341,R¯.307.Thestoryismuchdifferentnow:theestimatedpriceelasticityisnegativeandnotstatisti-callydifferentfromzero.Thetimetrendisstatisticallysignificant,anditscoefficientimplies335\nPart2RegressionAnalysiswithTimeSeriesDataanapproximate1%increaseininvpcperyear,onaverage.Fromthisanalysis,wecannotconcludethatrealpercapitahousinginvestmentisinfluencedatallbyprice.Thereareotherfactors,capturedinthetimetrend,thataffectinvpc,butwehavenotmodeledthese.Theresultsin(10.32)showaspuriousrelationshipbetweeninvpcandpriceduetothefactthatpriceisalsotrendingupwardovertime.Insomecases,addingatimetrendcanmakeakeyexplanatoryvariablemoresig-nificant.Thiscanhappenifthedependentandindependentvariableshavedifferentkindsoftrends(say,oneupwardandonedownward),butmovementintheindependentvariableaboutitstrendlinecausesmovementinthedependentvariableawayfromitstrendline.EXAMPLE10.8(FertilityEquation)Ifweaddalineartimetrendtothefertilityequation(10.18),weobtaingfˆrt(111.77)(.279)pet(35.59)ww2t0(.997)pillt(1.15)tgfˆrt00(3.36)(.040)pet0(6.30)ww2t(6.626)pillt(0.19)t(10.34)22n72,R.662,R¯.642.Thecoefficientonpeismorethantripletheestimatefrom(10.18),anditismuchmoresta-tisticallysignificant.Interestingly,pillisnotsignificantonceanallowanceismadeforalin-eartrend.Ascanbeseenbytheestimate,gfrwasfalling,onaverage,overthisperiod,otherfactorsbeingequal.Sincethegeneralfertilityrateexhibitedbothupwardanddownwardtrendsduringtheperiodfrom1913through1984,wecanseehowrobusttheestimatedeffectofpeiswhenweuseaquadratictrend:gfˆrt(124.09)(.348)pet(35.88)ww2t(10.12)pilltgfˆrt00(4.36)(.040)pet0(5.71)ww2t0(6.34)pillt2(2.53)t(.0196)t(10.35)2(0.39)t(.0050)t22n72,R.727,R¯.706.Thecoefficientonpeisevenlargerandmorestatisticallysignificant.Now,pillhastheexpectednegativeeffectandismarginallysignificant,andbothtrendtermsarestatisticallysignificant.Thequadratictrendisaflexiblewaytoaccountfortheunusualtrendingbehav-iorofgfr.YoumightbewonderinginExample10.8:Whystopataquadratictrend?Nothing3preventsusfromadding,say,tasanindependentvariable,and,infact,thismightbe336\nChapter10BasicRegressionAnalysiswithTimeSeriesDatawarranted(seeExercise10.12).Butwehavetobecarefulnottogetcarriedawaywhenincludingtrendtermsinamodel.Wewantrelativelysimpletrendsthatcapturebroadmovementsinthedependentvariablethatarenotexplainedbytheindependentvariablesinthemodel.Ifweincludeenoughpolynomialtermsint,thenwecantrackanyseriesprettywell.Butthisofferslittlehelpinfindingwhichexplanatoryvariablesaffectyt.ADetrendingInterpretationofRegressionswithaTimeTrendIncludingatimetrendinaregressionmodelcreatesaniceinterpretationintermsofdetrendingtheoriginaldataseriesbeforeusingtheminregressionanalysis.Forcon-creteness,wefocusonmodel(10.31),butourconclusionsaremuchmoregeneral.Whenweregressytonxt1,xt2andt,weobtainthefittedequationyˆtˆ0ˆ1xt1ˆ2xt2ˆ3t.(10.36)WecanextendtheresultsonthepartiallingoutinterpretationofOLSthatwecoveredinChapter3toshowthatˆ1andˆ2canbeobtainedasfollows.(i)Regresseachofyt,xt1andxt2onaconstantandthetimetrendtandsavetheresiduals,sayyt,xt1,xt2,t1,2,…,n.Forexample,ytytˆ0ˆ1t.Thus,wecanthinkofytasbeinglinearlydetrended.Indetrendingyt,wehaveesti-matedthemodelyt01tetbyOLS;theresidualsfromthisregression,eˆtyt,havethetimetrendremoved(atleastinthesample).Asimilarinterpretationholdsforxt1andxt2.(ii)Runtheregressionofytonxt1,xt2.(10.37)(Nointerceptisnecessary,butincludinganinterceptaffectsnothing:theinterceptwillbeestimatedtobezero.)Thisregressionexactlyyieldsˆ1andˆ2from(10.36).Thismeansthattheestimatesofprimaryinterest,ˆ1andˆ2,canbeinterpretedascomingfromaregressionwithoutatimetrend,butwherewefirstdetrendthedepen-dentvariableandallotherindependentvariables.Thesameconclusionholdswithanynumberofindependentvariablesandifthetrendisquadraticorofsomeotherpolyno-mialdegree.Iftisomittedfrom(10.36),thennodetrendingoccurs,andytmightseemtoberelatedtooneormoreofthextjsimplybecauseeachcontainsatrend;wesawthisinExample10.7.Ifthetrendtermisstatisticallysignificant,andtheresultschangeinimportantwayswhenatimetrendisaddedtoaregression,thentheinitialresultswith-outatrendshouldbetreatedwithsuspicion.Theinterpretationofˆ1andˆ2showsthatitisagoodideatoincludeatrendintheregressionifanyindependentvariableistrending,evenifytisnot.Ifythasnonotice-abletrend,but,say,xt1isgrowingovertime,thenexcludingatrendfromtheregression337\nPart2RegressionAnalysiswithTimeSeriesDatamaymakeitlookasifxt1hasnoeffectonyt,eventhoughmovementsofxt1aboutitstrendmayaffectyt.Thiswillbecapturediftisincludedintheregression.EXAMPLE10.9(PuertoRicanEmployment)Whenweaddalineartrendtoequation(10.17),theestimatesare(log(preˆpopt)8.70)(.169)log(mincovt)(1.06)log(usgnpt)log(preˆpopt)(1.30)(.044)log(mincovt)(0.18)log(usgnpt)(.032)t(.005)t(10.38)22n38,R.847,R¯.834.Thecoefficientonlog(usgnp)haschangeddramatically:from.012andinsignificantto1.06andverysignificant.Thecoefficientontheminimumwagehaschangedonlyslightly,althoughthestandarderrorisnotablysmaller,makinglog(mincov)moresignificantthanbefore.Thevariableprepoptdisplaysnoclearupwardordownwardtrend,butlog(usgnp)hasanupward,lineartrend.(Aregressionoflog(usgnp)ontgivesanestimateofabout.03,sothatusgnpisgrowingbyabout3%peryearovertheperiod.)Wecanthinkoftheestimate1.06asfollows:whenusgnpincreasesby1%aboveitslong-runtrend,prepopincreasesbyabout1.06%.ComputingR-squaredwhentheDependentVariableisTrendingR-squaredsintimeseriesregressionsareoftenveryhigh,especiallycomparedwithtyp-icalR-squaredsforcross-sectionaldata.Doesthismeanthatwelearnmoreaboutfac-torsaffectingyfromtimeseriesdata?Notnecessarily.Ononehand,timeseriesdataoftencomeinaggregateform(suchasaveragehourlywagesintheU.S.economy),andaggregatesareofteneasiertoexplainthanoutcomesonindividuals,families,orfirms,whichisoftenthenatureofcross-sectionaldata.ButtheusualandadjustedR-squaresfortimeseriesregressionscanbeartificiallyhighwhenthedependentvariableistrend-2ing.RememberthatRisameasureofhowlargetheerrorvarianceisrelativetothevarianceofy.TheformulafortheadjustedR-squaredshowsthisdirectly:222R¯1(ˆu/ˆy),22whereˆuistheunbiasedestimatoroftheerrorvariance,ˆySST/(n1),andn2SST(yty¯).Now,estimatingtheerrorvariancewhenytistrendingisnoprob-t1lem,providedatimetrendisincludedintheregression.However,whenE(yt)follows,say,alineartimetrend[see(10.24)],SST/(n1)isnolongeranunbiasedorconsis-tentestimatorofVar(yt).Infact,SST/(n1)cansubstantiallyoverestimatethevari-anceinyt,becauseitdoesnotaccountforthetrendinyt.338\nChapter10BasicRegressionAnalysiswithTimeSeriesDataWhenthedependentvariablesatisfieslinear,quadratic,oranyotherpolynomialtrends,itiseasytocomputeagoodness-of-fitmeasurethatfirstnetsouttheeffectofanytimetrendonyt.ThesimplestmethodistocomputetheusualR-squaredinaregres-sionwherethedependentvariablehasalreadybeendetrended.Forexample,ifthemodelis(10.31),thenwefirstregressytontandobtaintheresidualsyt.Then,weregressytonxt1,xt2,andt.(10.39)TheR-squaredfromthisregressionisSSR1n,(10.40)2ytt1nn2whereSSRisidenticaltothesumofsquaredresidualsfrom(10.36).Sinceytt1t12(yty¯)(andusuallytheinequalityisstrict),theR-squaredfrom(10.40)isnogreaterthan,andusuallylessthan,theR-squaredfrom(10.36).(Thesumofsquaredresidualsisidenticalinbothregressions.)Whenytcontainsastronglineartimetrend,(10.40)canbemuchlessthantheusualR-squared.TheR-squaredin(10.40)betterreflectshowwellxt1andxt2explainyt,becauseitnetsouttheeffectofthetimetrend.Afterall,wecanalwaysexplainatrendingvariablewithsomesortoftrend,butthisdoesnotmeanwehaveuncoveredanyfactorsthatcausemovementsinyt.AnadjustedR-squaredcanalsobecomputedbasedon(10.40):n2divideSSRby(n4)becausethisisthedfin(10.36)anddivideytby(n2),ast1therearetwotrendparametersestimatedindetrendingyt.Ingeneral,SSRisdividedbyn2thedfintheusualregression(thatincludesanytimetrends),andytisdividedbyt1(np),wherepisthenumberoftrendparametersestimatedindetrendingyt.SeeWooldridge(1991a)forfurtherdiscussiononcomputinggoodness-of-fitmeasureswithtrendingvariables.EXAMPLE10.10(HousingInvestment)InExample10.7,wesawthatincludingalineartimetrendalongwithlog(price)inthehousinginvestmentequationhadasubstantialeffectonthepriceelasticity.ButtheR-squaredfromregression(10.33),takenliterally,saysthatweare“explaining”34.1%ofthevariationinlog(invpc).Thisismisleading.Ifwefirstdetrendlog(invpc)andregressthedetrendedvariableonlog(price)andt,theR-squaredbecomes.008,andtheadjustedR-squaredisactuallynegative.Thus,movementsinlog(price)aboutitstrendhavevirtuallynoexplanatorypowerformovementsinlog(invpc)aboutitstrend.Thisisconsistentwiththefactthatthetstatisticonlog(price)inequation(10.33)isverysmall.339\nPart2RegressionAnalysiswithTimeSeriesDataBeforeleavingthissubsection,wemustmakeafinalpoint.IncomputingtheR-squaredformofanFstatisticfortestingmultiplehypotheses,wejustusetheusualR-squaredswithoutanydetrending.Remember,theR-squaredformoftheFstatisticisjustacomputationaldevice,andsotheusualformulaisalwaysappropriate.SeasonalityIfatimeseriesisobservedatmonthlyorquarterlyintervals(orevenweeklyordaily),itmayexhibitseasonality.Forexample,monthlyhousingstartsintheMidwestarestronglyinfluencedbyweather.Whileweatherpatternsaresomewhatrandom,wecanbesurethattheweatherduringJanuarywillusuallybemoreinclementthaninJune,andsohousingstartsaregenerallyhigherinJunethaninJanuary.Onewaytomodelthisphenomenonistoallowtheexpectedvalueoftheseries,yt,tobedifferentineachmonth.Asanotherexample,retailsalesinthefourthquarteraretypicallyhigherthaninthepreviousthreequartersbecauseoftheChristmasholiday.Again,thiscanbecap-turedbyallowingtheaverageretailsalestodifferoverthecourseofayear.Thisisinadditiontopossiblyallowingforatrendingmean.Forexample,retailsalesinthemostrecentfirstquarterwerehigherthanretailsalesinthefourthquarterfrom30yearsago,becauseretailsaleshavebeensteadilygrowing.Nevertheless,ifwecompareaveragesaleswithinatypicalyear,theseasonalholidayfactortendstomakesaleslargerinthefourthquarter.Eventhoughmanymonthlyandquarterlydataseriesdisplayseasonalpatterns,notallofthemdo.Forexample,thereisnonoticeableseasonalpatterninmonthlyinterestorinflationrates.Inaddition,seriesthatdodisplayseasonalpatternsareoftenseason-allyadjustedbeforetheyarereportedforpublicuse.Aseasonallyadjustedseriesisonethat,inprinciple,hashadtheseasonalfactorsremovedfromit.Seasonaladjustmentcanbedoneinavarietyofways,andacarefuldiscussionisbeyondthescopeofthistext.[SeeHarvey(1990)andHylleberg(1986)fordetailedtreatments.]Seasonaladjustmenthasbecomesocommonthatitisnotpossibletogetseasonallyunadjusteddatainmanycases.QuarterlyU.S.GDPisaleadingexample.IntheannualEconomicReportofthePresident,manymacroeconomicdatasetsreportedatmonthlyfrequencies(atleastforthemostrecentyears)andthosethatdisplayseasonalpatternsareallseasonallyadjusted.Themajorsourcesformacroeconomictimeseries,includ-ingCitibase,alsoseasonallyadjustmanyoftheseries.Thus,thescopeforusingourownseasonaladjustmentisoftenlimited.Sometimes,wedoworkwithseasonallyunadjusteddata,anditisusefultoknowthatsimplemethodsareavailablefordealingwithseasonalityinregressionmodels.Generally,wecanincludeasetofseasonaldummyvariablestoaccountforseasonal-ityinthedependentvariable,theindependentvariables,orboth.Theapproachissimple.Supposethatwehavemonthlydata,andwethinkthatsea-sonalpatternswithinayearareroughlyconstantacrosstime.Forexample,sinceChristmasalwayscomesatthesametimeofyear,wecanexpectretailsalestobe,onaverage,higherinmonthslateintheyearthaninearliermonths.Or,sinceweatherpat-ternsarebroadlysimilaracrossyears,housingstartsintheMidwestwillbehigheronaverageduringthesummermonthsthanthewintermonths.Ageneralmodelformonthlydatathatcapturesthesephenomenais340\nChapter10BasicRegressionAnalysiswithTimeSeriesDatayt01febt2mart3aprt…11dect(10.41)1xt1…kxtkut,wherefebt,mart,…,dectaredummyvariablesindicatingwhethertimeperiodtcorre-spondstotheappropriatemonth.Inthisformulation,Januaryisthebasemonth,QUESTION10.5and0istheinterceptforJanuary.IfthereInequation(10.41),whatistheinterceptforMarch?Explainwhyisnoseasonalityinyt,oncethextjhaveseasonaldummyvariablessatisfythestrictexogeneityassumption.beencontrolledfor,then1through11areallzero.ThisiseasilytestedviaanFtest.EXAMPLE10.11(EffectsofAntidumpingFilings)InExample10.5,weusedmonthlydatathathavenotbeenseasonallyadjusted.There-fore,weshouldaddseasonaldummyvariablestomakesurenoneoftheimportantconclu-sionschanges.Itcouldbethatthemonthsjustbeforethesuitwasfiledaremonthswhereimportsarehigherorlower,onaverage,thaninothermonths.Whenweaddthe11monthlydummyvariablesasin(10.41)andtesttheirjointsignificance,weobtainp-value.59,andsotheseasonaldummiesarejointlyinsignificant.Inaddition,nothingimportantchangesintheestimatesoncestatisticalsignificanceistakenintoaccount.KruppandPollard(1996)actuallyusedthreedummyvariablesfortheseasons(fall,spring,andsummer,withwinterasthebaseseason),ratherthanafullsetofmonthlydummies;theoutcomeisessentiallythesame.Ifthedataarequarterly,thenwewouldincludedummyvariablesforthreeofthefourquarters,withtheomittedcategorybeingthebasequarter.Sometimes,itisusefultointeractseasonaldummieswithsomeofthextjtoallowtheeffectofxtjonyttodif-feracrosstheyear.Justasincludingatimetrendinaregressionhastheinterpretationofinitiallydetrendingthedata,includingseasonaldummiesinaregressioncanbeinterpretedasdeseasonalizingthedata.Forconcreteness,considerequation(10.41)withk2.TheOLSslopecoefficientsˆ1andˆ2onx1andx2canbeobtainedasfollows:(i)Regresseachofyt,xt1andxt2onaconstantandthemonthlydummies,febt,mart,…,dect,andsavetheresiduals,sayyt,xt1andxt2,forallt1,2,…,n.Forexample,ytytˆ0ˆ1febtˆ2mart…ˆ11dect.Thisisonemethodofdeseasonalizingamonthlytimeseries.Asimilarinterpretationholdsforxt1andxt2.(ii)Runtheregression,withoutthemonthlydummies,ofytonxt1andxt2[justasin(10.37)].Thisgivesˆ1andˆ2.Insomecases,ifythaspronouncedseasonality,abettergoodness-of-fitmeasureisanR-squaredbasedonthedeseasonalizedyt.Thisnetsoutanyseasonaleffectsthatare341\nPart2RegressionAnalysiswithTimeSeriesDatanotexplainedbythextj.SpecificdegreesoffreedomajustmentsarediscussedinWooldridge(1991a).Timeseriesexhibitingseasonalpatternscanbetrendingaswell,inwhichcase,weshouldestimatearegressionmodelwithatimetrendandseasonaldummyvariables.Theregressionscanthenbeinterpretedasregressionsusingbothdetrendedanddesea-sonalizedseries.Goodness-of-fitstatisticsarediscussedinWooldridge(1991a):essen-tially,wedetrendanddeasonalizeytbyregressingonbothatimetrendandseasonaldummiesbeforecomputingR-squared.SUMMARYInthischapter,wehavecoveredbasicregressionanalysiswithtimeseriesdata.Underassumptionsthatparallelthoseforcross-sectionalanalysis,OLSisunbiased(underTS.1throughTS.3),OLSisBLUE(underTS.1throughTS.5),andtheusualOLSstan-darderrors,tstatistics,andFstatisticscanbeusedforstatisticalinference(underTS.1throughTS.6).Becauseofthetemporalcorrelationinmosttimeseriesdata,wemustexplicitlymakeassumptionsabouthowtheerrorsarerelatedtotheexplanatoryvari-ablesinalltimeperiodsandaboutthetemporalcorrelationintheerrorsthemselves.Theclassicallinearmodelassumptionscanbeprettyrestrictivefortimeseriesapplica-tions,buttheyareanaturalstartingpoint.Wehaveappliedthemtobothstaticregres-sionandfinitedistributedlagmodels.Logarithmsanddummyvariablesareusedregularlyintimeseriesapplicationsandineventstudies.Wealsodiscussedindexnumbersandtimeseriesmeasuredintermsofnominalandrealdollars.Trendsandseasonalitycanbeeasilyhandledinamultipleregressionframeworkbyincludingtimeandseasonaldummyvariablesinourregressionequations.WepresentedproblemswiththeusualR-squaredasagoodness-of-fitmeasureandsuggestedsomesimplealternativesbasedondetrendingordeseasonalizing.KEYTERMSAutocorrelationLong-RunElasticityBasePeriodLong-RunMultiplierBaseValueLong-RunPropensity(LRP)ContemporaneouslyExogenousSeasonalDummyVariablesDeseasonalizingSeasonalityDetrendingSeasonallyAdjustedEventStudySerialCorrelationExponentialTrendShort-RunElasticityFiniteDistributedLag(FDL)ModelSpuriousRegressionGrowthRateStaticModelImpactMultiplierStochasticProcessImpactPropensityStrictlyExogenousIndexNumberTimeSeriesProcessLagDistributionTimeTrendLinearTimeTrend342\nChapter10BasicRegressionAnalysiswithTimeSeriesDataPROBLEMS10.1Decideifyouagreeordisagreewitheachofthefollowingstatementsandgiveabriefexplanationofyourdecision:(i)Likecross-sectionalobservations,wecanassumethatmosttimeseriesobservationsareindependentlydistributed.(ii)TheOLSestimatorinatimeseriesregressionisunbiasedunderthefirstthreeGauss-Markovassumptions.(iii)Atrendingvariablecannotbeusedasthedependentvariableinmulti-pleregressionanalysis.(iv)Seasonalityisnotanissuewhenusingannualtimeseriesobservations.10.2LetgGDPtdenotetheannualpercentagechangeingrossdomesticproductandletinttdenoteashort-terminterestrate.SupposethatgGDPtisrelatedtointerestratesbygGDPt00intt1intt1ut,whereutisuncorrelatedwithintt,intt1,andallotherpastvaluesofinterestrates.SupposethattheFederalReservefollowsthepolicyrule:intt01(gGDPt13)vt,where10.(Whenlastyear’sGDPgrowthisabove3%,theFedincreasesinterestratestopreventan“overheated”economy.)Ifvtisuncorrelatedwithallpastvaluesofinttandut,arguethatinttmustbecorrelatedwithut1.(Hint:LagthefirstequationforonetimeperiodandsubstituteforgGDPt1inthesecondequation.)WhichGauss-Markovassumptiondoesthisviolate?10.3SupposeytfollowsasecondorderFDLmodel:yt00zt1zt12zt2ut.Letz*denotetheequilibriumvalueofztandlety*betheequilibriumvalueofyt,suchthaty*00z*1z*2z*.Showthatthechangeiny*,duetoachangeinz*,equalsthelong-runpropensitytimesthechangeinz*:y*LRPz*.ThisgivesanalternativewayofinterpretingtheLRP.10.4Whenthethreeeventindicatorsbefile6,affile6,andafdec6aredroppedfrom22equation(10.22),weobtainR.281andR¯.264.Aretheeventindicatorsjointlysignificantatthe10%level?10.5Supposeyouhavequarterlydataonnewhousingstarts,interestrates,andrealpercapitaincome.Specifyamodelforhousingstartsthataccountsforpossibletrendsandseasonalityinthevariables.10.6InExample10.4,wesawthatourestimatesoftheindividuallagcoefficientsinadistributedlagmodelwereveryimprecise.Onewaytoalleviatethemulticollinearity343\nPart2RegressionAnalysiswithTimeSeriesDataproblemistoassumethatthejfollowarelativelysimplepattern.Forconcreteness,consideramodelwithfourlags:yt00zt1zt12zt23zt34zt4ut.Now,letusassumethatthejfollowaquadraticinthelag,j:2j01j2j,forparameters0,1,and2.Thisisanexampleofapolynomialdistributedlag(PDL)model.(i)Plugtheformulaforeachjintothedistributedlagmodelandwritethemodelintermsoftheparametersh,forh0,1,2.(ii)Explaintheregressionyouwouldruntoestimatetheh.(iii)Thepolynomialdistributedlagmodelisarestrictedversionofthegen-eralmodel.Howmanyrestrictionsareimposed?Howwouldyoutestthese?(Hint:ThinkFtest.)COMPUTEREXERCISES10.7InOctober1979,theFederalReservechangeditspolicyoftargetingthemoneysupplyandinsteadbegantofocusdirectlyonshort-terminterestrates.UsingthedatainINTDEF.RAW,defineadummyvariableequaltooneforyearsafter1979.Includethisdummyinequation(10.15)toseeifthereisashiftintheinterestrateequationafter1979.Whatdoyouconclude?10.8UsethedatainBARIUM.RAWforthisexercise.(i)Addalineartimetrendtoequation(10.22).Areanyvariables,otherthanthetrend,statisticallysignificant?(ii)Intheequationestimatedinpart(i),testforjointsignificanceofallvariablesexceptthetimetrend.Whatdoyouconclude?(iii)Addmonthlydummyvariablestothisequationandtestforseasonality.Doesincludingthemonthlydummieschangeanyotherestimatesortheirstandarderrorsinimportantways?10.9Addthevariablelog(prgnp)totheminimumwageequationin(10.38).Isthisvariablesignificant?Interpretthecoefficient.Howdoesaddinglog(prgnp)affecttheestimatedminimumwageeffect?10.10UsethedatainFERTIL3.RAWtoverifythatthestandarderrorfortheLRPinequation(10.19)isabout.030.10.11UsethedatainEZANDERS.RAWforthisexercise.ThedataareonmonthlyunemploymentclaimsinAndersonTownshipinIndiana,fromJanuary1980throughNovember1988.In1984,anenterprisezone(EZ)waslocatedinAnderson(aswellasothercitiesinIndiana).[SeePapke(1994)fordetails.](i)Regresslog(uclms)onalineartimetrendand11monthlydummyvari-ables.Whatwastheoveralltrendinunemploymentclaimsoverthisperiod?(Interpretthecoefficientonthetimetrend.)Isthereevidenceofseasonalityinunemploymentclaims?344\nChapter10BasicRegressionAnalysiswithTimeSeriesData(ii)Addez,adummyvariableequaltooneinthemonthsAndersonhadanEZ,totheregressioninpart(i).Doeshavingtheenterprisezoneseemtodecreaseunemploymentclaims?Byhowmuch?[Youshouldusefor-mula(7.10)fromChapter7.](iii)Whatassumptionsdoyouneedtomaketoattributetheeffectinpart(ii)tothecreationofanEZ?10.12UsethedatainFERTIL3.RAWforthisexercise.2(i)Regressgfrtontandtandsavetheresiduals.Thisgivesadetrendedgfrt,saygfrt.2(ii)Regressgfrtonallofthevariablesinequation(10.35),includingtandt.ComparetheR-squaredwiththatfrom(10.35).Whatdoyouconclude?3(iii)Reestimateequation(10.35)butaddttotheequation.Isthisadditionaltermstatisticallysignificant?10.13UsethedatasetCONSUMP.RAWforthisexercise.(i)Estimateasimpleregressionmodelrelatingthegrowthinrealpercapitaconsumption(ofnondurablesandservices)tothegrowthinrealpercapitadisposableincome.Usethechangeinthelogarithmsinbothcases.Reporttheresultsintheusualform.Interprettheequationanddiscussstatisticalsignificance.(ii)Addalagofthegrowthinrealpercapitadisposableincometotheequationfrompart(i).Whatdoyouconcludeaboutadjustmentlagsinconsumptiongrowth?(iii)Addtherealinterestratetotheequationinpart(i).Doesitaffectcon-sumptiongrowth?10.14UsethedatainFERTIL3.RAWforthisexercise.(i)Addpet3andpet4toequation(10.19).Testforjointsignificanceoftheselags.(ii)Findtheestimatedlong-runpropensityanditsstandarderrorinthemodelfrompart(i).Comparethesewiththoseobtainedfromequation(10.19).(iii)EstimatethepolynomialdistributedlagmodelfromProblem10.6.FindtheestimatedLRPandcomparethiswithwhatisobtainedfromtheunrestrictedmodel.10.15UsethedatainVOLAT.RAWforthisexercise.Thevariablersp500isthemonthlyreturnontheStandard&Poors500stockmarketindex,atanannualrate.(Thisincludespricechangesaswellasdividends.)Thevariablei3isthereturnonthree-monthT-bills,andpcipisthepercentagechangeinindustrialproduction;thesearealsoatanannualrate.(i)Considertheequationrsp500t01pcipt2i3tut.Whatsignsdoyouthink1and2shouldhave?(ii)EstimatethepreviousequationbyOLS,reportingtheresultsinstan-dardform.Interpretthesignsandmagnitudesofthecoefficients.345\nPart2RegressionAnalysiswithTimeSeriesData(iii)Whichofthevariablesisstatisticallysignificant?(iv)Doesyourfindingfrompart(iii)implythatthereturnontheS&P500ispredictable?Explain.10.16Considerthemodelestimatedin(10.15);usethedatainINTDEF.RAW.(i)Findthecorrelationbetweeninfanddefoverthissampleperiodandcomment.(ii)Addasinglelagofinfanddeftotheequationandreporttheresultsintheusualform.(iii)ComparetheestimatedLRPfortheeffectofinflationfromthatinequa-tion(10.15).Aretheyvastlydifferent?(iv)Arethetwolagsinthemodeljointlysignificantatthe5%level?346\nd7/14/997:06PMPage347ChapterElevenFurtherIssuesinUsingOLSwithTimeSeriesDatanChapter10,wediscussedthefinitesamplepropertiesofOLSfortimeseriesdataIunderincreasinglystrongersetsofassumptions.Underthefullsetofclassicallin-earmodelassumptionsfortimeseries,TS.1throughTS.6,OLShasexactlythesamedesirablepropertiesthatwederivedforcross-sectionaldata.Likewise,statisticalinferenceiscarriedoutinthesamewayasitwasforcross-sectionalanalysis.Fromourcross-sectionalanalysisinChapter5,weknowthattherearegoodreasonsforstudyingthelargesamplepropertiesofOLS.Forexample,iftheerrortermsarenotdrawnfromanormaldistribution,thenwemustrelyonthecentrallimittheoremtojus-tifytheusualOLSteststatisticsandconfidenceintervals.Largesampleanalysisisevenmoreimportantintimeseriescontexts.(Thisissome-whatironicgiventhatlargetimeseriessamplescanbedifficulttocomeby;butweoftenhavenochoiceotherthantorelyonlargesampleapproximations.)InSection10.3,weexplainedhowthestrictexogeneityassumption(TS.2)mightbeviolatedinstaticanddistributedlagmodels.AswewillshowinSection11.2,modelswithlaggeddependentvariablesmustviolateAssumptionTS.2.Unfortunately,largesampleanalysisfortimeseriesproblemsisfraughtwithmanymoredifficultiesthanitwasforcross-sectionalanalysis.InChapter5,weobtainedthelargesamplepropertiesofOLSinthecontextofrandomsampling.Thingsaremorecomplicatedwhenweallowtheobservationstobecorrelatedacrosstime.Nevertheless,themajorlimittheoremsholdforcertain,althoughnotall,timeseriesprocesses.Thekeyiswhetherthecorrelationbetweenthevariablesatdifferenttimeperiodstendstozeroquicklyenough.Timeseriesthathavesubstantialtemporalcorrelationrequirespe-cialattentioninregressionanalysis.Thischapterwillalertyoutocertainissuesper-tainingtosuchseriesinregressionanalysis.11.1STATIONARYANDWEAKLYDEPENDENTTIMESERIESInthissection,wepresentthekeyconceptsthatareneededtoapplytheusuallargesam-pleapproximationsinregressionanalysiswithtimeseriesdata.Thedetailsarenotasimportantasageneralunderstandingoftheissues.347\nd7/14/997:06PMPage348Part2RegressionAnalysiswithTimeSeriesDataStationaryandNonstationaryTimeSeriesHistorically,thenotionofastationaryprocesshasplayedanimportantroleintheanalysisoftimeseries.Astationarytimeseriesprocessisonewhoseprobabilitydistri-butionsarestableovertimeinthefollowingsense:ifwetakeanycollectionofrandomvariablesinthesequenceandthenshiftthatsequenceaheadhtimeperiods,thejointprobabilitydistributionmustremainunchanged.Aformaldefinitionofstationarityfollows.STATIONARYSTOCHASTICPROCESS:Thestochasticprocess{xt:t1,2,…}issta-tionaryifforeverycollectionoftimeindices1t1t2…tm,thejointdistribu-tionof(xt1,xt2,…,xtm)isthesameasthejointdistributionof(xt1h,xt2h,…,xtmh)forallintegersh1.Thisdefinitionisalittleabstract,butitsmeaningisprettystraightforward.Oneimplication(bychoosingm1andt11)isthatxthasthesamedistributionasx1forallt2,3,….Inotherwords,thesequence{xt:t1,2,…}isidenticallydistributed.Stationarityrequiresevenmore.Forexample,thejointdistributionof(x1,x2)(thefirsttwotermsinthesequence)mustbethesameasthejointdistributionof(xt,xt1)foranyt1.Again,thisplacesnorestrictionsonhowxtandxt1arerelatedtooneanother;indeed,theymaybehighlycorrelated.Stationaritydoesrequirethatthenatureofanycorrelationbetweenadjacenttermsisthesameacrossalltimeperiods.Astochasticprocessthatisnotstationaryissaidtobeanonstationaryprocess.Sincestationarityisanaspectoftheunderlyingstochasticprocessandnotoftheavail-ablesinglerealization,itcanbeverydifficulttodeterminewhetherthedatawehavecollectedweregeneratedbyastationaryprocess.However,itiseasytospotcertainsequencesthatarenotstationary.AprocesswithatimetrendofthetypecoveredinSection10.5isclearlynonstationary:ataminimum,itsmeanchangesovertime.Sometimes,aweakerformofstationaritysuffices.If{xt:t1,2,…}hasafinite2secondmoment,thatis,E(xt)forallt,thenthefollowingdefinitionapplies.COVARIANCESTATIONARYPROCESS:Astochasticprocess{xt:t1,2,…}with2finitesecondmoment[E(xt)]iscovariancestationaryif(i)E(xt)isconstant;(ii)Var(xt)isconstant;(iii)foranyt,h1,Cov(xt,xth)dependsonlyonhandnotont.Covariancestationarityfocusesonlyonthefirsttwomomentsofastochasticprocess:themeanandvarianceoftheprocessareconstantacrosstime,andthecovari-ancebetweenxtandxthdependsonlyonthedistancebetweenthetwoterms,h,andQUESTION11.1notonthelocationoftheinitialtimeSupposethat{yt:t1,2,…}isgeneratedbyyt01tet,period,t.Itfollowsimmediatelythatthewhere10,and{et:t1,2,…}isani.i.d.sequencewithmeancorrelationbetweenxtandxthalsode-zeroandvariance2.(i)Is{y}covariancestationary?(ii)IsyE(y)etttpendsonlyonh.covariancestationary?Ifastationaryprocesshasafinitesec-ondmoment,thenitmustbecovariancestationary,buttheconverseiscertainlynottrue.Sometimes,toemphasizethatstation-arityisastrongerrequirementthancovariancestationarity,theformerisreferredtoasstrictstationarity.However,sincewewillnotbedelvingintotheintricaciesofcentral348\nd7/14/997:06PMPage349Chapter11FurtherIssuesinUsingOLSwithTimeSeriesDatalimittheoremsfortimeseriesprocesses,wewillnotbeworriedaboutthedistinctionbetweenstrictandcovariancestationarity:wewillcallaseriesstationaryifitsatisfieseitherdefinition.Howisstationarityusedintimeserieseconometrics?Onatechnicallevel,station-aritysimplifiesstatementsofthelawoflargenumbersandthecentrallimittheorem,althoughwewillnotworryaboutformalstatements.Onapracticallevel,ifwewanttounderstandtherelationshipbetweentwoormorevariablesusingregressionanalysis,weneedtoassumesomesortofstabilityovertime.Ifweallowtherelationshipbetweentwovariables(say,ytandxt)tochangearbitrarilyineachtimeperiod,thenwecannothopetolearnmuchabouthowachangeinonevariableaffectstheothervariableifweonlyhaveaccesstoasingletimeseriesrealization.Instatingamultipleregressionmodelfortimeseriesdata,weareassumingacer-tainformofstationarityinthatthejdonotchangeovertime.Further,AssumptionsTS.4andTS.5implythatthevarianceoftheerrorprocessisconstantovertimeandthatthecorrelationbetweenerrorsintwoadjacentperiodsisequaltozero,whichisclearlyconstantovertime.WeaklyDependentTimeSeriesStationarityhastodowiththejointdistributionsofaprocessasitmovesthroughtime.Averydifferentconceptisthatofweakdependence,whichplacesrestrictionsonhowstronglyrelatedtherandomvariablesxtandxthcanbeasthetimedistancebetweenthem,h,getslarge.Thenotionofweakdependenceismosteasilydiscussedforasta-tionarytimeseries:looselyspeaking,astationarytimeseriesprocess{xt:t1,2,…}issaidtobeweaklydependentifxtandxthare“almostindependent”ashincreaseswithoutbound.Asimilarstatementholdstrueifthesequenceisnonstationary,butthenwemustassumethattheconceptofbeingalmostindependentdoesnotdependonthestartingpoint,t.Thedescriptionofweakdependencegiveninthepreviousparagraphisnecessar-ilyvague.Wecannotformallydefineweakdependencebecausethereisnodefinitionthatcoversallcasesofinterest.Therearemanyspecificformsofweakdependencethatareformallydefined,butthesearewellbeyondthescopeofthistext.[SeeWhite(1984),Hamilton(1994),andWooldridge(1994b)foradvancedtreatmentsoftheseconcepts.]Forourpurposes,anintuitivenotionofthemeaningofweakdependenceissuffi-cient.Covariancestationarysequencescanbecharacterizedintermsofcorrelations:acovariancestationarytimeseriesisweaklydependentifthecorrelationbetweenxtandxthgoestozero“sufficientlyquickly”ash*.(Becauseofcovariancestationarity,thecorrelationdoesnotdependonthestartingpoint,t.)Inotherwords,asthevariablesgetfartherapartintime,thecorrelationbetweenthembecomessmallerandsmaller.CovariancestationarysequenceswhereCorr(xt,xth)*0ash*aresaidtobeasymptoticallyuncorrelated.Intuitively,thisishowwewillusuallycharacterizeweakdependence.Technically,weneedtoassumethatthecorrelationconvergestozerofastenough,butwewillglossoverthis.Whyisweakdependenceimportantforregressionanalysis?Essentially,itreplacestheassumptionofrandomsamplinginimplyingthatthelawoflargenumbers(LLN)349\nd7/14/997:06PMPage350Part2RegressionAnalysiswithTimeSeriesDataandthecentrallimittheorem(CLT)hold.Themostwell-knowncentrallimittheoremfortimeseriesdatarequiresstationarityandsomeformofweakdependence:thus,sta-tionary,weaklydependenttimeseriesareidealforuseinmultipleregressionanalysis.InSection11.2,wewillshowhowOLScanbejustifiedquitegenerallybyappealingtotheLLNandtheCLT.Timeseriesthatarenotweaklydependent—examplesofwhichwewillseeinSection11.3—donotgenerallysatisfytheCLT,whichiswhytheiruseinmultipleregressionanalysiscanbetricky.Thesimplestexampleofaweaklydependenttimeseriesisanindependent,identi-callydistributedsequence:asequencethatisindependentistriviallyweaklydependent.Amoreinterestingexampleofaweaklydependentsequenceisxtet1et1,t1,2,…,(11.1)2where{et:t0,1,…}isani.i.d.sequencewithzeromeanandvariancee.Theprocess{xt}iscalledamovingaverageprocessoforderone[MA(1)]:xtisaweightedaver-ageofetandet1;inthenextperiod,wedropet1,andthenxt1dependsonet1andet.Settingthecoefficientonettoonein(11.1)iswithoutlossofgenerality.WhyisanMA(1)processweaklydependent?Adjacenttermsinthesequenceare2correlated:becausext1et11et,Cov(xt,xt1)1Var(et)1e.Since222Var(xt)(11)e,Corr(xt,xt1)1/(11).Forexample,if1.5,thenCorr(xt,xt1).4.[Themaximumpositivecorrelationoccurswhen11;inwhichcase,Corr(xt,xt1).5.]However,oncewelookatvariablesinthesequencethataretwoormoretimeperiodsapart,thesevariablesareuncorrelatedbecausetheyareindependent.Forexample,xt2et21et1isindependentofxtbecause{et}isindependentacrosst.Duetotheidenticaldistributionassumptionontheet,{xt}in(11.1)isactuallystationary.Thus,anMA(1)isastationary,weaklydependentsequence,andthelawoflargenumbersandthecentrallimittheoremcanbeappliedto{xt}.Amorepopularexampleistheprocessyt1yt1et,t1,2,….(11.2)Thestartingpointinthesequenceisy0(att0),and{et:t1,2,…}isani.i.d.2sequencewithzeromeanandvariancee.Wealsoassumethattheetareindependentofy0andthatE(y0)0.Thisiscalledanautoregressiveprocessoforderone[AR(1)].ThecrucialassumptionforweakdependenceofanAR(1)processisthestabilitycondition11.Thenwesaythat{yt}isastableAR(1)process.ToseethatastableAR(1)processisasymptoticallyuncorrelated,itisusefultoassumethattheprocessiscovariancestationary.(Infact,itcangenerallybeshownthat{yt}isstrictlystationary,buttheproofissomewhattechnical.)Then,weknowthatE(yt)E(yt1),andfrom(11.2)with11,thiscanhappenonlyifE(yt)0.Takingthevarianceof(11.2)andusingthefactthatetandyt1areindependent(andtherefore2uncorrelated),Var(yt)1Var(yt1)Var(et),andso,undercovariancestationarity,22222wemusthavey1ye.Since11bythestabilitycondition,wecaneasily2solvefory:350\nd7/14/997:06PMPage351Chapter11FurtherIssuesinUsingOLSwithTimeSeriesData222ye/(11).(11.3)Nowwecanfindthecovariancebetweenytandythforh1.Usingrepeatedsub-stitution,yth1yth1eth1(1yth2eth1)eth21yth21eth1eth…hh11yt1et1…1eth1eth.SinceE(yt)0forallt,wecanmultiplythislastequationbyytandtakeexpecta-tionstoobtainCov(yt,yth).Usingthefactthatetjisuncorrelatedwithytforallj1givesh2h1Cov(yt,yth)E(ytyth)1E(yt)1E(ytet1)…E(yteth)h2h21E(yt)1y.Sinceyisthestandarddeviationofbothytandyth,wecaneasilyfindthecorrelationbetweenytandythforanyh1:hCorr(yt,yth)Cov(yt,yth)/(yy)1.(11.4)Inparticular,Corr(yt,yt1)1,so1isthecorrelationcoefficientbetweenanytwoadjacenttermsinthesequence.Equation(11.4)isimportantbecauseitshowsthat,whileytandytharecorrelatedhforanyh1,thiscorrelationgetsverysmallforlargeh:since11,1*0ash*.Evenwhen1islarge—say.9,whichimpliesaveryhigh,positivecorrelationbetweenadjacentterms—thecorrelationbetweenytandythtendstozerofairlyrapidly.Forexample,Corr(yt,yt5).591,Corr(yt,yt10).349,andCorr(yt,yt20).122.Iftindexesyear,thismeansthatthecorrelationbetweentheoutcomeoftwoythataretwentyyearsapartisabout.122.When1issmaller,thecorrelationdiesoutmuchmorequickly.(Youmighttry1.5toverifythis.)ThisanalysisheuristicallydemonstratesthatastableAR(1)processisweaklydependent.TheAR(1)modelisespeciallyimportantinmultipleregressionanalysiswithtimeseriesdata.WewillcoveradditionalapplicationsinChapter12andtheuseofitforforecastinginChapter18.Therearemanyothertypesofweaklydependenttimeseries,includinghybridsofautoregressiveandmovingaverageprocesses.Butthepreviousexamplesworkwellforourpurposes.Beforeendingthissection,wemustemphasizeonepointthatoftencausesconfu-sionintimeserieseconometrics.Atrendingseries,whilecertainlynonstationary,canbeweaklydependent.Infact,inthesimplelineartimetrendmodelinChapter10[seeequation(10.24)],theseries{yt}wasactuallyindependent.Aseriesthatisstationaryaboutitstimetrend,aswellasweaklydependent,isoftencalledatrend-stationaryprocess.(Noticethatthenameisnotcompletelydescriptivebecauseweassumeweakdependencealongwithstationarity.)SuchprocessescanbeusedinregressionanalysisjustasinChapter10,providedappropriatetimetrendsareincludedinthemodel.351\nd7/14/997:06PMPage352Part2RegressionAnalysiswithTimeSeriesData11.2ASYMPTOTICPROPERTIESOFOLSInChapter10,wesawsomecaseswheretheclassicallinearmodelassumptionsarenotsatisfiedforcertaintimeseriesproblems.Insuchcases,wemustappealtolargesamplepropertiesofOLS,justaswithcross-sectionalanalysis.Inthissection,westatetheassumptionsandmainresultsthatjustifyOLSmoregenerally.Theproofsofthetheoremsinthischapteraresomewhatdifficultandthereforeomitted.SeeWooldridge(1994b).ASSUMPTIONTS.1(LINEARITYANDWEAKDEPENDENCE)AssumptionTS.1isthesameasTS.1,exceptwemustalsoassumethat{(xt,yt):t1,2,…}isweaklydependent.Inotherwords,thelawoflargenumbersandthecentrallimittheo-remcanbeappliedtosampleaverages.Thelinearinparametersrequirementagainmeansthatwecanwritethemodelasyt01xt1…kxtkut,(11.5)wherethejaretheparameterstobeestimated.Thextjcancontainlaggeddependentandindependentvariables,providedtheweakdependenceassumptionismet.Wehavediscussedtheconceptofweakdependenceatlengthbecauseitisbynomeansaninnocuousassumption.Inthenextsection,wewillpresenttimeseriesprocessesthatclearlyviolatetheweakdependenceassumptionandalsodiscusstheuseofsuchprocessesinmultipleregressionmodels.ASSUMPTIONTS.2(ZEROCONDITIONALMEAN)Foreacht,E(utxt)0.Thisisthemostnaturalassumptionconcerningtherelationshipbetweenutandtheexplanatoryvariables.ItismuchweakerthanAssumptionTS.2becauseitputsnorestrictionsonhowutisrelatedtotheexplanatoryvariablesinothertimeperiods.WewillseeexamplesthatsatisfyTS.2shortly.Forcertainpurposes,itisusefultoknowthatthefollowingconsistencyresultonlyrequiresuttohavezerounconditionalmeanandtobeuncorrelatedwitheachxtj:E(ut)0,Cov(xtj,ut)0,j1,…,k.(11.6)Wewillworkmostlywiththezeroconditionalmeanassumptionbecauseitleadstothemoststraightforwardasymptoticanalysis.ASSUMPTIONTS.3(NOPERFECTCOLLINEARITY)SameasAssumptionTS.3.352\nd7/14/997:06PMPage353Chapter11FurtherIssuesinUsingOLSwithTimeSeriesDataTHEOREM11.1(CONSISTENCYOFOLS)UnderTS.1,TS.2,andTS.3,theOLSestimatorsareconsistent:plimˆjj,j0,1,…,k.TherearesomekeypracticaldifferencesbetweenTheorems10.1and11.1.First,inTheorem11.1,weconcludethattheOLSestimatorsareconsistent,butnotnecessarilyunbiased.Second,inTheorem11.1,wehaveweakenedthesenseinwhichtheexplana-toryvariablesmustbeexogenous,butweakdependenceisrequiredintheunderlyingtimeseries.Weakdependenceisalsocrucialinobtainingapproximatedistributionalresults,whichwecoverlater.EXAMPLE11.1(StaticModel)Considerastaticmodelwithtwoexplanatoryvariables:yt01zt12zt2ut.(11.7)Underweakdependence,theconditionsufficientforconsistencyofOLSisE(utzt1,zt2)0.(11.8)Thisrulesoutomittedvariablesthatareinutandarecorrelatedwitheitherzt1orzt2.Also,nofunctionofzt1orzt2canbecorrelatedwithut,andsoAssumptionTS.2rulesoutmis-specifiedfunctionalform,justasinthecross-sectionalcase.Otherproblems,suchasmea-surementerrorinthevariableszt1orzt2,cancause(11.8)tofail.Importantly,AssumptionTS.2doesnotruleoutcorrelationbetween,say,ut1andzt1.Thistypeofcorrelationcouldariseifzt1isrelatedtopastyt1,suchaszt101yt1vt.(11.9)Forexample,zt1mightbeapolicyvariable,suchasmonthlypercentagechangeinthemoneysupply,andthischangedependsonlastmonth’srateofinflation(yt1).Suchamechanismgenerallycauseszt1andut1tobecorrelated(ascanbeseenbyplugginginforyt1).ThiskindoffeedbackisallowedunderAssumptionTS.2.EXAMPLE11.2(FiniteDistributedLagModel)Inthefinitedistributedlagmodel,yt00zt1zt12zt2ut,(11.10)averynaturalassumptionisthattheexpectedvalueofut,givencurrentandallpastvaluesofz,iszero:353\nd7/14/997:06PMPage354Part2RegressionAnalysiswithTimeSeriesDataE(utzt,zt1,zt2,zt3,…)0.(11.11)Thismeansthat,oncezt,zt1,andzt2areincluded,nofurtherlagsofzaffectE(ytzt,zt1,zt2,zt3,…);ifthiswerenottrue,wewouldputfurtherlagsintotheequation.Forexample,ytcouldbetheannualpercentagechangeininvestmentandztameasureofinterestratesduringyeart.Whenwesetxt(zt,zt1,zt2),AssumptionTS.2isthensat-isfied:OLSwillbeconsistent.Asinthepreviousexample,TS.2doesnotruleoutfeedbackfromytofuturevaluesofz.Theprevioustwoexamplesdonotnecessarilyrequireasymptotictheorybecausetheexplanatoryvariablescouldbestrictlyexogenous.Thenextexampleclearlyviolatesthestrictexogeneityassumption,andthereforewecanonlyappealtolargesamplepropertiesofOLS.EXAMPLE11.3[AR(1)Model]ConsidertheAR(1)model,yt01yt1ut,(11.12)wheretheerroruthasazeroexpectedvalue,givenallpastvaluesofy:E(utyt1,yt2,…)0.(11.13)Combined,thesetwoequationsimplythatE(ytyt1,yt2,…)E(ytyt1)01yt1.(11.14)Thisresultisveryimportant.First,itmeansthat,onceylaggedoneperiodhasbeencon-trolledfor,nofurtherlagsofyaffecttheexpectedvalueofyt.(Thisiswherethename“firstorder”originates.)Second,therelationshipisassumedtobelinear.Sincextcontainsonlyyt1,equation(11.13)impliesthatAssumptionTS.2holds.Bycontrast,thestrictexogeneityassumptionneededforunbiasedness,AssumptionTS.2,doesnothold.Sincethesetofexplanatoryvariablesforalltimeperiodsincludesalloftheval-uesonyexceptthelast(y0,y1,…,yn1),AssumptionTS.2requiresthat,forallt,utisuncor-relatedwitheachofy0,y1,…,yn1.Thiscannotbetrue.Infact,becauseutisuncorrelatedwithyt1under(11.13),utandytmustbecorrelated.Therefore,amodelwithalaggeddependentvariablecannotsatisfythestrictexogeneityassumptionTS.2.Fortheweakdependenceconditiontohold,wemustassumethat11,aswedis-cussedinSection11.1.Ifthisconditionholds,thenTheorem11.1impliesthattheOLSesti-matorfromtheregressionofytonyt1producesconsistentestimatorsof0and1.Unfortunately,ˆ1isbiased,andthisbiascanbelargeifthesamplesizeissmallorif1is354\nd7/14/997:06PMPage355Chapter11FurtherIssuesinUsingOLSwithTimeSeriesDatanearone.(For1nearone,ˆ1canhaveaseveredownwardbias.)Inmoderatetolargesam-ples,ˆ1shouldbeagoodestimatorof1.Whenusingthestandardinferenceprocedures,weneedtoimposeversionsofthehomoskedasticityandnoserialcorrelationassumptions.ThesearelessrestrictivethantheirclassicallinearmodelcounterpartsfromChapter10.ASSUMPTIONTS.4(HOMOSKEDASTICITY)2Forallt,Var(utxt).ASSUMPTIONTS.5(NOSERIALCORRELATION)Forallts,E(utusxt,xs)0.InTS.4,notehowweconditiononlyontheexplanatoryvariablesattimet(comparetoTS.4).InTS.5,weconditiononlyontheexplanatoryvariablesinthetimeperiodscoincidingwithutandus.Asstated,thisassumptionisalittledifficulttointerpret,butitistherightconditionforstudyingthelargesamplepropertiesofOLSinavarietyoftimeseriesregressions.WhenconsideringTS.5,weoftenignoretheconditioningonxtandxs,andwethinkaboutwhetherutandusareuncorrelated,forallts.Serialcorrelationisoftenaprobleminstaticandfinitedistributedlagregressionmodels:nothingguaranteesthattheunobservablesutareuncorrelatedovertime.Importantly,AssumptionTS.5doesholdintheAR(1)modelstatedinequations(11.12)and(11.13).Sincetheexplanatoryvariableattimetisyt1,wemustshowthatE(utusyt1,ys1)0forallts.Toseethis,supposethatst.(Theothercasefol-lowsbysymmetry.)Then,sinceusys01ys1,usisafunctionofydatedbeforetimet.Butby(11.13),E(utus,yt1,ys1)0,andthenthelawofiteratedexpec-tations(seeAppendixB)impliesthatE(utusyt1,ys1)0.Thisisveryimportant:aslongasonlyonelagbelongsin(11.12),theerrorsmustbeseriallyuncorrelated.WewilldiscussthisfeatureofdynamicmodelsmoregenerallyinSection11.4.Wenowobtainanasymptoticresultthatispracticallyidenticaltothecross-sectionalcase.THEOREM11.2(ASYMPTOTICNORMALITYOFOLS)UnderTS.1throughTS.5,theOLSestimatorsareasymptoticallynormallydistributed.Further,theusualOLSstandarderrors,tstatistics,Fstatistics,andLMstatisticsareasymp-toticallyvalid.Thistheoremprovidesadditionaljustificationforatleastsomeoftheexamplesesti-matedinChapter10:eveniftheclassicallinearmodelassumptionsdonothold,OLSisstillconsistent,andtheusualinferenceproceduresarevalid.Ofcourse,thishingesonTS.1throughTS.5beingtrue.Inthenextsection,wediscusswaysinwhichtheweakdependenceassumptioncanfail.Theproblemsofserialcorrelationandhet-eroskedasticityaretreatedinChapter12.355\nd7/14/997:06PMPage356Part2RegressionAnalysiswithTimeSeriesDataEXAMPLE11.4(EfficientMarketsHypothesis)Wecanuseasymptoticanalysistotestaversionoftheefficientmarketshypothesis(EMH).Letytbetheweeklypercentagereturn(fromWednesdayclosetoWednesdayclose)ontheNewYorkStockExchangecompositeindex.Astrictformoftheefficientmarketshypothe-sisstatesthatinformationobservabletothemarketpriortoweektshouldnothelptopre-dictthereturnduringweekt.Ifweuseonlypastinformationony,theEMHisstatedasE(ytyt1,yt2,…)E(yt).(11.15)If(11.15)isfalse,thenwecoulduseinformationonpastweeklyreturnstopredictthecur-rentreturn.TheEMHpresumesthatsuchinvestmentopportunitieswillbenoticedandwilldisappearalmostinstantaneously.Onesimplewaytotest(11.15)istospecifytheAR(1)modelin(11.12)asthealterna-tivemodel.Then,thenullhypothesisiseasilystatedasH0:10.Underthenullhypoth-esis,AssumptionTS.2istrueby(11.15),and,aswediscussedearlier,serialcorrelationis2notanissue.ThehomoskedasticityassumptionisVar(ytyt1)Var(yt),whichwejustassumeistruefornow.Underthenullhypothesis,stockreturnsareseriallyuncorrelated,sowecansafelyassumethattheyareweaklydependent.Then,Theorem11.2sayswecanusetheusualOLStstatisticforˆ1totestH0:10againstH1:10.TheweeklyreturnsinNYSE.RAWarecomputedusingdatafromJanuary1976throughMarch1989.IntherarecasethatWednesdaywasaholiday,thecloseatthenexttradingdaywasused.Theaverageweeklyreturnoverthisperiodwas.196inpercentform,withthelargestweeklyreturnbeing8.45%andthesmallestbeing15.32%(duringthestockmarketcrashofOctober1987).EstimationoftheAR(1)modelgivesretuˆrnt(.180)(.059)returnt1retuˆrnt(.081)(.038)returnt1(11.16)22n689,R.0035,R¯.0020.Thetstatisticforthecoefficientonreturnt1isabout1.55,andsoH0:10cannotberejectedagainstthetwo-sidedalternative,evenatthe10%significancelevel.TheestimatedoessuggestaslightpositivecorrelationintheNYSEreturnfromoneweektothenext,butitisnotstrongenoughtowarrantrejectionoftheefficientmarketshypothesis.Inthepreviousexample,usinganAR(1)modeltotesttheEMHmightnotdetectcorrelationbetweenweeklyreturnsthataremorethanoneweekapart.Itiseasytoesti-matemodelswithmorethanonelag.Forexample,anautoregressivemodelofordertwo,orAR(2)model,isyt01yt12yt2ut(11.17)E(utyt1,yt2,…)0.356\nd7/14/997:06PMPage357Chapter11FurtherIssuesinUsingOLSwithTimeSeriesDataTherearestabilityconditionson1and2thatareneededtoensurethattheAR(2)processisweaklydependent,butthisisnotanissueherebecausethenullhypothesisstatesthattheEMHholds:H0:120.(11.18)2IfweaddthehomoskedasticityassumptionVar(utyt1,yt2),wecanuseastandardFstatistictotest(11.18).IfweestimateanAR(2)modelforreturnt,weobtainretuˆrnt(.186)(.060)returnt1(.038)returnt2retuˆrnt(.081)(.038)returnt1(.038)returnt222n688,R.0048,R¯.0019(whereweloseonemoreobservationbecauseoftheadditionallagintheequation).Thetwolagsareindividuallyinsignificantatthe10%level.Theyarealsojointlyinsignifi-2cant:usingR.0048,theFstatisticisapproximatelyF1.65;thep-valueforthisFstatistic(with2and685degreesoffreedom)isabout.193.Thus,wedonoreject(11.18)ateventhe15%significancelevel.EXAMPLE11.5(ExpectationsAugmentedPhillipsCurve)AlinearversionoftheexpectationsaugmentedPhillipscurvecanbewrittenaseinftinft1(unemt0)et,ewhere0isthenaturalrateofunemploymentandinftistheexpectedrateofinflationformedinyeart1.Thismodelassumesthatthenaturalrateisconstant,somethingthatmacroeconomistsquestion.Thedifferencebetweenactualunemploymentandthenaturalrateiscalledcyclicalunemployment,whilethedifferencebetweenactualandexpectedinflationiscalledunanticipatedinflation.Theerrorterm,et,iscalledasupplyshockbymacroeconomists.Ifthereisatradeoffbetweenunanticipatedinflationandcyclicalunem-ployment,then10.[ForadetaileddiscussionoftheexpectationsaugmentedPhillipscurve,seeMankiw(1994,Section11.2).]Tocompletethismodel,weneedtomakeanassumptionaboutinflationaryexpecta-tions.Underadaptiveexpectations,theexpectedvalueofcurrentinflationdependsonrecentlyobservedinflation.Aparticularlysimpleformulationisthatexpectedinflationthiseyearislastyear’sinflation:inftinft1.(SeeSection18.1foranalternativeformulationofadaptiveexpectations.)Underthisassumption,wecanwriteinftinft101unemtetorinft01unemtet,whereinftinftinft1and010.(0isexpectedtobepositive,since10and00.)Therefore,underadaptiveexpectations,theexpectationsaugmentedPhillipscurverelatesthechangeininflationtothelevelofunemploymentandasupplyshock,et.Ifetisuncorrelatedwithunemt,asistypicallyassumed,thenwecanconsistentlyestimate357\nd7/14/997:06PMPage358Part2RegressionAnalysiswithTimeSeriesData0and1byOLS.(Wedonothavetoassumethat,say,futureunemploymentratesareunaffectedbythecurrentsupplyshock.)WeassumethatTS.1throughTS.5hold.Theesti-matedequationisinˆft(3.03)(.543)unemtinˆft(1.38)(.230)unemt(11.19)22n48,R.108,R¯.088.Thetradeoffbetweencyclicalunemploymentandunanticipatedinflationispronouncedinequation(11.19):aone-pointincreaseinunemlowersunanticipatedinflationbyoverone-halfofapoint.Theeffectisstatisticallysignificant(two-sidedp-value.023).Wecancon-trastthiswiththestaticPhillipscurveinExample10.1,wherewefoundaslightlypositiverelationshipbetweeninflationandunemployment.Becausewecanwritethenaturalrateas00/(1),wecanuse(11.19)toobtainourownestimateofthenaturalrate:ˆ0ˆ0/(ˆ1)3.03/.5435.58.Thus,weesti-matethenaturalratetobeabout5.6,whichiswellwithintherangesuggestedbymacro-economists:historically,5to6%isacommonrangecitedforthenaturalrateofunemployment.Itispossibletoobtainanapproximatestandarderrorforthisestimate,butthemethodsarebeyondthescopeofthistext.[See,forexample,DavidsonandMacKinnon(1993).]UnderAssumptionsTS.1throughTS.5,wecanshowthattheOLSestimatorsareasymptoticallyefficientintheclassofestimatorsdescribedinTheorem5.3,butwereplacethecross-sectionalobservationindexiwiththetimeseriesindext.QUESTION11.2Finally,modelswithtrendingexplanatorySupposethatexpectationsareformedasinfe(1/2)infvariablescansatisfyAssumptionsTS.1tt1(1/2)inft2.Whatregressionwouldyouruntoestimatetheexpecta-throughTS.5,providedtheyaretrendsta-tionsaugmentedPhillipscurve?tionary.Aslongastimetrendsarein-cludedintheequationswhenneeded,theusualinferenceproceduresareasymptoticallyvalid.11.3USINGHIGHLYPERSISTENTTIMESERIESINREGRESSIONANALYSISTheprevioussectionshowsthat,providedthetimeseriesweuseareweaklydependent,usualOLSinferenceproceduresarevalidunderassumptionsweakerthantheclassicallinearmodelassumptions.Unfortunately,manyeconomictimeseriescannotbechar-acterizedbyweakdependence.Usingtimeserieswithstrongdependenceinregressionanalysisposesnoproblem,iftheCLMassumptionsinChapter10hold.Buttheusualinferenceproceduresareverysusceptibletoviolationoftheseassumptionswhenthedataarenotweaklydependent,becausethenwecannotappealtothelawoflargenum-bersandthecentrallimittheorem.Inthissection,weprovidesomeexamplesofhighly358\nd7/14/997:06PMPage359Chapter11FurtherIssuesinUsingOLSwithTimeSeriesDatapersistent(orstronglydependent)timeseriesandshowhowtheycanbetransformedforuseinregressionanalysis.HighlyPersistentTimeSeriesInthesimpleAR(1)model(11.2),theassumption11iscrucialfortheseriestobeweaklydependent.ItturnsoutthatmanyeconomictimeseriesarebettercharacterizedbytheAR(1)modelwith11.Inthiscase,wecanwriteytyt1et,t1,2,…,(11.20)whereweagainassumethat{et:t1,2,…}isindependentandidenticallydistributed2withmeanzeroandvariancee.Weassumethattheinitialvalue,y0,isindependentofetforallt1.Theprocessin(11.20)iscalledarandomwalk.Thenamecomesfromthefactthatyattimetisobtainedbystartingatthepreviousvalue,yt1,andaddingazeromeanrandomvariablethatisindependentofyt1.Sometimes,arandomwalkisdefineddif-ferentlybyassumingdifferentpropertiesoftheinnovations,et(suchaslackofcorrela-tionratherthanindependence),butthecurrentdefinitionsufficesforourpurposes.First,wefindtheexpectedvalueofyt.Thisismosteasilydonebyusingrepeatedsubstitutiontogetytetet1…e1y0.TakingtheexpectedvalueofbothsidesgivesE(yt)E(et)E(et1)…E(e1)E(y0)E(y0),forallt1.Therefore,theexpectedvalueofarandomwalkdoesnotdependont.Apopularassumptionisthaty00—theprocessbeginsatzeroattimezero—inwhichcase,E(yt)0forallt.Bycontrast,thevarianceofarandomwalkdoeschangewitht.Tocomputethevari-anceofarandomwalk,forsimplicityweassumethaty0isnonrandomsothatVar(y0)0;thisdoesnotaffectanyimportantconclusions.Then,bythei.i.d.assump-tionfor{et},2Var(yt)Var(et)Var(et1)…Var(e1)et.(11.21)Inotherwords,thevarianceofarandomwalkincreasesasalinearfunctionoftime.Thisshowsthattheprocesscannotbestationary.Evenmoreimportantly,arandomwalkdisplayshighlypersistentbehaviorinthesensethatthevalueofytodayissignificantfordeterminingthevalueofyintheverydistantfuture.Toseethis,writeforhperiodshence,ythetheth1…et1yt.Now,supposeattimet,wewanttocomputetheexpectedvalueofythgiventhecur-rentvalueyt.Sincetheexpectedvalueofetj,givenyt,iszeroforallj1,wehave359\nd7/14/997:06PMPage360Part2RegressionAnalysiswithTimeSeriesDataE(ythyt)yt,forallh1.(11.22)Thismeansthat,nomatterhowfarinthefuturewelook,ourbestpredictionofythistoday’svalue,yt.WecancontrastthiswiththestableAR(1)case,whereasimilarargu-mentcanbeusedtoshowthathE(ythyt)1yt,forallh1.Understability,11,andsoE(ythyt)approacheszeroash*:thevalueofytbecomeslessandlessimportant,andE(ythyt)getscloserandclosertotheuncondi-tionalexpectedvalue,E(yt)0.Whenh1,equation(11.22)isreminiscentoftheadaptiveexpectationsassump-tionweusedfortheinflationrateinExample11.5:ifinflationfollowsarandomwalk,thentheexpectedvalueofinft,givenpastvaluesofinflation,issimplyinft1.Thus,arandomwalkmodelforinflationjustifiestheuseofadaptiveexpectations.Wecanalsoseethatthecorrelationbetweenytandythisclosetooneforlargetwhen{yt}followsarandomwalk.IfVar(y0)0,itcanbeshownthatCorr(yt,yth)t/(th).Thus,thecorrelationdependsonthestartingpoint,t(sothat{yt}isnotcovariancesta-tionary).Further,forfixedt,thecorrelationtendstozeroash*0,butitdoesnotdosoveryquickly.Infact,thelargertis,themoreslowlythecorrelationtendstozeroashgetslarge.Ifwechoosehtobesomethinglarge—say,h100—wecanalwayschoosealargeenoughtsuchthatthecorrelationbetweenytandythisarbitrarilyclosetoone.(Ifh100andwewantthecorrelationtobegreaterthan.95,thent1,000doesthetrick.)Therefore,arandomwalkdoesnotsatisfytherequirementofanasymp-toticallyuncorrelatedsequence.Figure11.1plotstworealizationsofarandomwalkwithinitialvaluey00andet~Normal(0,1).Generally,itisnoteasytolookatatimeseriesplotandtodeterminewhetherornotitisarandomwalk.Next,wewilldiscussaninformalmethodformak-ingthedistinctionbetweenweaklyandhighlydependentsequences;wewillstudyfor-malstatisticaltestsinChapter18.Aseriesthatisgenerallythoughttobewell-characterizedbyarandomwalkisthethree-month,T-billrate.AnnualdataareplottedinFigure11.2fortheyears1948through1996.Arandomwalkisaspecialcaseofwhatisknownasaunitrootprocess.Thenamecomesfromthefactthat11intheAR(1)model.Amoregeneralclassofunitrootprocessesisgeneratedasin(11.20),but{et}isnowallowedtobeageneral,weaklydependentseries.[Forexample,{et}coulditselffollowanMA(1)orastableAR(1)process.]When{et}isnotani.i.d.sequence,thepropertiesoftherandomwalkwederivedearliernolongerhold.Butthekeyfeatureof{yt}ispreserved:thevalueofytodayishighlycorrelatedwithyeveninthedistantfuture.Fromapolicyperspective,itisoftenimportanttoknowwhetheraneconomictimeseriesishighlypersistentornot.ConsiderthecaseofgrossdomesticproductintheUnitedStates.IfGDPisasymptoticallyuncorrelated,thenthelevelofGDPinthecom-ingyearisatbestweaklyrelatedtowhatGDPwas,say,thirtyyearsago.ThismeansapolicythataffectedGDPlongagohasverylittlelastingimpact.Ontheotherhand,if360\nd7/14/997:06PMPage361Chapter11FurtherIssuesinUsingOLSwithTimeSeriesDataFigure11.1Tworealizationsoftherandomwalkytyt1et,withy00,etNormal(0,1),andn50.yt50–5–1002550tGDPisstronglydependent,thennextyear’sGDPcanbehighlycorrelatedwiththeGDPfrommanyyearsago.Then,weshouldrecognizethatapolicywhichcausesadis-cretechangeinGDPcanhavelong-lastingeffects.Itisextremelyimportantnottoconfusetrendingandhighlypersistentbehaviors.Aseriescanbetrendingbutnothighlypersistent,aswesawinChapter10.Further,fac-torssuchasinterestrates,inflationrates,andunemploymentratesarethoughtbymanytobehighlypersistent,buttheyhavenoobviousupwardordownwardtrend.However,itisoftenthecasethatahighlypersistentseriesalsocontainsacleartrend.Onemodelthatleadstothisbehavioristherandomwalkwithdrift:yt0yt1et,t1,2,…,(11.23)where{et:t1,2,…}andy0satisfythesamepropertiesasintherandomwalkmodel.Whatisnewistheparameter0,whichiscalledthedriftterm.Essentially,togenerateyt,theconstant0isaddedalongwiththerandomnoiseettothepreviousvalueyt1.Wecanshowthattheexpectedvalueofytfollowsalineartimetrendbyusingrepeatedsubstitution:yt0tetet1…e1y0.Therefore,ify00,E(yt)0t:theexpectedvalueofytisgrowingovertimeif00andshrinkingovertimeif00.Byreasoningaswedidinthepurerandomwalkcase,wecanshowthatE(ythyt)0hyt,andsothebestpredictionofythattimetisytplusthedrift0h.Thevarianceofytisthesameasitwasinthepurerandomwalkcase.361\nd7/14/997:06PMPage362Part2RegressionAnalysiswithTimeSeriesDataFigure11.2TheU.S.three-monthT-billrate,fortheyears1948–1996.interestrate1481194819721996yearFigure11.3containsarealizationofarandomwalkwithdrift,wheren50,y00,02,andtheetareNormal(0,9)randomvariables.Ascanbeseenfromthisgraph,yttendstogrowovertime,buttheseriesdoesnotregularlyreturntothetrendline.Arandomwalkwithdriftisanotherexampleofaunitrootprocess,becauseitisthespecialcase11inanAR(1)modelwithanintercept:yt01yt1et.When11and{et}isanyweaklydependentprocess,weobtainawholeclassofhighlypersistenttimeseriesprocessesthatalsohavelinearlytrendingmeans.TransformationsonHighlyPersistentTimeSeriesUsingtimeserieswithstrongpersistenceofthetypedisplayedbyaunitrootprocessinaregressionequationcanleadtoverymisleadingresultsiftheCLMassumptionsareviolated.WewillstudythespuriousregressionprobleminmoredetailinChapter18,butfornowwemustbeawareofpotentialproblems.Fortunately,simpletransforma-tionsareavailablethatrenderaunitrootprocessweaklydependent.Weaklydependentprocessesaresaidtobeintegratedoforderzero,[I(0)].Practically,thismeansthatnothingneedstobedonetosuchseriesbeforeusingtheminregressionanalysis:averagesofsuchsequencesalreadysatisfythestandardlimitthe-362\nd7/14/997:06PMPage363Chapter11FurtherIssuesinUsingOLSwithTimeSeriesDataFigure11.3Arealizationoftherandomwalkwithdrift,yt2yt1et,withy00,etNormal(0,9),andn50.Thedashedlineistheexpectedvalueofyt,E(yt)2t.yt10050002550torems.Unitrootprocesses,suchasarandomwalk(withorwithoutdrift),aresaidtobeintegratedoforderzero,orI(0).Thismeansthatthefirstdifferenceoftheprocessisweaklydependent(andoftenstationary).Thisissimpletoseeforarandomwalk.With{yt}generatedasin(11.20)fort1,2,…,ytytyt1et,t2,3,…;(11.24)therefore,thefirst-differencedseries{yt:t2,3,…}isactuallyani.i.d.sequence.Moregenerally,if{yt}isgeneratedby(11.24)where{et}isanyweaklydependentprocess,then{yt}isweaklydependent.Thus,whenwesuspectprocessesareinte-gratedoforderone,weoftenfirstdifferenceinordertousetheminregressionanaly-sis;wewillseesomeexampleslater.Manytimeseriesytthatarestrictlypositivearesuchthatlog(yt)isintegratedoforderone.Inthiscase,wecanusethefirstdifferenceinthelogs,log(yt)log(yt)log(yt1),inregressionanalysis.Alternatively,sincelog(yt)(ytyt1)/yt1,(11.25)363\nd7/14/997:06PMPage364Part2RegressionAnalysiswithTimeSeriesDatawecanusetheproportionateorpercentagechangeinytdirectly;thisiswhatwedidinExample11.4where,ratherthanstatingtheefficientmarketshypothesisintermsofthestockprice,pt,weusedtheweeklypercentagechange,returnt100[(ptpt1)/pt1].Differencingtimeseriesbeforeusingtheminregressionanalysishasanotherben-efit:itremovesanylineartimetrend.Thisiseasilyseenbywritingalinearlytrendingvariableasyt01tvt,wherevthasazeromean.Thenyt1vt,andsoE(yt)1E(vt)1.Inotherwords,E(yt)isconstant.Thesameargumentworksforlog(yt)whenlog(yt)followsalineartimetrend.Therefore,ratherthanincludingatimetrendinaregression,wecaninsteaddifferencethosevariablesthatshowobvioustrends.DecidingWhetheraTimeSeriesIsI(1)DeterminingwhetheraparticulartimeseriesrealizationistheoutcomeofanI(1)ver-susanI(0)processcanbequitedifficult.Statisticaltestscanbeusedforthispurpose,butthesearemoreadvanced;weprovideanintroductorytreatmentinChapter18.Thereareinformalmethodsthatprovideusefulguidanceaboutwhetheratimeseriesprocessisroughlycharacterizedbyweakdependence.Averysimpletoolismoti-vatedbytheAR(1)model:if11,thentheprocessisI(0),butitisI(1)if11.Earlier,weshowedthat,whentheAR(1)processisstable,1Corr(yt,yt1).There-fore,wecanestimate1fromthesamplecorrelationbetweenytandyt1.Thissamplecorrelationcoefficientiscalledthefirstorderautocorrelationof{yt};wedenotethisbyˆ1.Byapplyingthelawoflargenumbers,ˆ1canbeshowntobeconsistentfor1pro-vided11.(However,ˆ1isnotanunbiasedestimatorof1.)Wecanusethevalueofˆ1tohelpdecidewhethertheprocessisI(1)orI(0).Unfortunately,becauseˆ1isanestimate,wecanneverknowforsurewhether11.Ideally,wecouldcomputeaconfidenceintervalfor1toseeifitexcludesthevalue11,butthisturnsouttoberatherdifficult:thesamplingdistributionsoftheestima-torofˆ1areextremelydifferentwhen1isclosetooneandwhen1ismuchlessthanone.(Infact,when1isclosetoone,ˆ1canhaveaseveredownwardbias.)InChapter18,wewillshowhowtotestH0:11againstH0:11.Fornow,wecanonlyuseˆ1asaroughguidefordeterminingwhetheraseriesneedstobediffer-enced.Nohardandfastruleexistsformakingthischoice.Mosteconomiststhinkthatdifferencingiswarrantedifˆ1.9;somewoulddifferencewhenˆ1.8.EXAMPLE11.6(FertilityEquation)InExample10.4,weexplainedthegeneralfertilityrate,gfr,intermsofthevalueofthepersonalexemption,pe.Thefirstorderautocorrelationsfortheseseriesareverylarge:ˆ1.977forgfrandˆ1.964forpe.Thesearesuggestiveofunitrootbehavior,andtheyraisequestionsabouttheuseoftheusualOLStstatisticsinChapter10.Wenowesti-matetheequationsusingthefirstdifferences(anddroppingthedummyvariablesforsim-plicity):364\nd7/14/997:06PMPage365Chapter11FurtherIssuesinUsingOLSwithTimeSeriesData(gfˆr.785)(.043)pegfˆr(.502)(.028)pe(11.26)22n71,R.032,R¯.018.Now,anincreaseinpeisestimatedtolowergfrcontemporaneously,althoughtheestimateisnotstatisticallydifferentfromzeroatthe5%level.Thisgivesverydifferentresultsthanwhenweestimatedthemodelinlevels,anditcastsdoubtonourearlieranalysis.Ifweaddtwolagsofpe,thingsimprove:(gfˆr.964)(.036)pe(.014)pe1(.110)pe2gfˆr(.468)(.027)pe(.028)pe1(.027)pe2(11.27)22n69,R.233,R¯.197.Eventhoughpeandpe1havenegativecoefficients,theircoefficientsaresmallandjointlyinsignificant(p-value.28).Thesecondlagisverysignificantandindicatesaposi-tiverelationshipbetweenchangesinpeandsubsequentchangesingfrtwoyearshence.Thismakesmoresensethanhavingacontemporaneouseffect.SeeExercise11.12forfur-theranalysisoftheequationinfirstdifferences.Whentheseriesinquestionhasanobviousupwardordownwardtrend,itmakesmoresensetoobtainthefirstorderautocorrelationafterdetrending.Ifthedataarenotdetrended,theautoregressivecorrelationtendstobeoverestimated,whichbiasestowardfindingaunitrootinatrendingprocess.EXAMPLE11.7(WagesandProductivity)ThevariablehrwageisaveragehourlywageintheU.S.economy,andoutphrisoutputperhour.Onewaytoestimatetheelasticityofhourlywagewithrespecttooutputperhouristoestimatetheequation,log(hrwaget)01log(outphrt)2tut,wherethetimetrendisincludedbecauselog(hrwage)andlog(outphrt)bothdisplayclear,upward,lineartrends.UsingthedatainEARNS.RAWfortheyears1947through1987,weobtain(log(hrˆwaget)5.33)(1.64)log(outphrt)(.018)tlog(hrˆwaget)(0.37)(0.09)log(outphrt)(.002)t(11.28)22n41,R.971,R¯.970.(Wehavereportedtheusualgoodness-of-fitmeasureshere;itwouldbebettertoreportthosebasedonthedetrendeddependentvariable,asinSection10.5.)Theestimatedelas-ticityseemstoolarge:a1%increaseinproductivityincreasesrealwagesbyabout1.64%.365\nd7/14/997:06PMPage366Part2RegressionAnalysiswithTimeSeriesDataBecausethestandarderrorissosmall,the95%confidenceintervaleasilyexcludesaunitelasticity.U.S.workerswouldprobablyhavetroublebelievingthattheirwagesincreasebymorethan1.5%forevery1%increaseinproductivity.Theregressionresultsin(11.28)mustbeviewedwithcaution.Evenafterlinearlyde-trendinglog(hrwage),thefirstorderautocorrelationis.967,andfordetrendedlog(outphr),ˆ1.945.Thesesuggestthatbothserieshaveunitroots,sowereestimatetheequationinfirstdifferences(andwenolongerneedatimetrend):(log(hrˆwaget).0036)(.809)log(outphr)log(hrˆwaget)(.0042)(.173)log(outphr)(11.29)22n40,R.364,R¯.348.Now,a1%increaseinproductivityisestimatedtoincreaserealwagesbyabout.81%,andtheestimateisnotstatisticallydifferentfromone.TheadjustedR-squaredshowsthatthegrowthinoutputexplainsabout35%ofthegrowthinrealwages.SeeExercise11.9forasimpledistributedlagversionofthemodelinfirstdifferences.Intheprevioustwoexamples,boththedependentandindependentvariablesappeartohaveunitroots.Inothercases,wemighthaveamixtureofprocesseswithunitrootsandthosethatareweaklydependent(thoughpossiblytrending).AnexampleisgiveninExercise11.8.11.4DYNAMICALLYCOMPLETEMODELSANDTHEABSENCEOFSERIALCORRELATIONIntheAR(1)model(11.12),weshowedthat,underassumption(11.13),theerrors{ut}mustbeseriallyuncorrelatedinthesensethatAssumptionTS.5issatisfied:assum-ingthatnoserialcorrelationexistsispracticallythesamethingasassumingthatonlyonelagofyappearsinE(ytyt1,yt2,…).Canwemakeasimilarstatementforotherregressionmodels?Theanswerisyes.Considerthesimplestaticregressionmodelyt01ztut,(11.30)whereytandztarecontemporaneouslydated.ForconsistencyofOLS,weonlyneedE(utzt)0.Generally,the{ut}willbeseriallycorrelated.However,ifweassumethatE(utzt,yt1,zt1,…)0,(11.31)then(aswewillshowgenerallylater)AssumptionTS.5holds.Inparticular,the{ut}areseriallyuncorrelated.Togaininsightintothemeaningof(11.31),wecanwrite(11.30)and(11.31)equiv-alentlyas366\nd7/14/997:06PMPage367Chapter11FurtherIssuesinUsingOLSwithTimeSeriesDataE(ytzt,yt1,zt1,…)E(ytzt)01zt,(11.32)wherethefirstequalityistheoneofcurrentinterest.Itsaysthat,oncezthasbeencon-trolledfor,nolagsofeitheryorzhelptoexplaincurrenty.Thisisastrongrequirement;ifitisfalse,thenwecanexpecttheerrorstobeseriallycorrelated.Next,considerafinitedistributedlagmodelwithtwolags:yt01zt2zt13zt2ut.(11.33)Sincewearehopingtocapturethelaggedeffectsthatzhasony,wewouldnaturallyassumethat(11.33)capturesthedistributedlagdynamics:E(ytzt,zt1,zt2,zt3,…)E(ytzt,zt1,zt2);(11.34)thatis,atmosttwolagsofzmatter.If(11.31)holds,wecanmakefurtherstatements:oncewehavecontrolledforzanditstwolags,nolagsofyoradditionallagsofzaffectcurrenty:E(ytzt,yt1,zt1,…)E(ytzt,zt1,zt2).(11.35)Equation(11.35)ismorelikelythan(11.32),butitstillrulesoutlaggedyaffectingcur-renty.Next,consideramodelwithonelagofbothyandz:yt01zt2yt13zt1ut.Sincethismodelincludesalaggeddependentvariable,(11.31)isanaturalassumption,asitimpliesthatE(ytzt,yt1,zt1,yt2…)E(ytzt,yt1,zt1);inotherwords,oncezt,yt1,andzt1havebeencontrolledfor,nofurtherlagsofeitheryorzaffectcurrenty.Inthegeneralmodelyt01xt1…kxtkut,(11.36)wheretheexplanatoryvariablesxt(xt1,…,xtk)mayormaynotcontainlagsofyorz,(11.31)becomesE(utxt,yt1,xt1,…)0.(11.37)Writtenintermsofyt,E(ytxt,yt1,xt1,…)E(ytxt).(11.38)Inwords,whateverisinxt,enoughlagshavebeenincludedsothatfurtherlagsofyandtheexplanatoryvariablesdonotmatterforexplainingyt.Whenthisconditionholds,we367\nd7/14/997:06PMPage368Part2RegressionAnalysiswithTimeSeriesDatahaveadynamicallycompletemodel.Aswesawearlier,dynamiccompletenesscanbeaverystrongassumptionforstaticandfinitedistributedlagmodels.Oncewestartputtinglaggedyasexplanatoryvariables,weoftenthinkthatthemodelshouldbedynamicallycomplete.Wewilltouchonsomeexceptionstothisprac-ticeinChapter18.Since(11.37)isequivalenttoE(utxt,ut1,xt1,ut2,…)0,(11.39)wecanshowthatadynamicallycompletemodelmustsatisfyAssumptionTS.5.(Thisderivationisnotcrucialandcanbeskippedwithoutlossofcontinuity.)Forconcrete-ness,takest.Then,bythelawofiteratedexpectations(seeAppendixB),E(utusxt,xs)E[E(utusxt,xs,us)xt,xs]E[usE(utxt,xs,us)xt,xs],wherethesecondequalityfollowsfromE(utusxt,xs,us)usE(utxt,xs,us).Now,sincest,(xt,xs,us)isasubsetoftheconditioningsetin(11.39).Therefore,(11.39)impliesthatE(utxt,xs,us)0,andsoE(utusxt,xs)E(us0xt,xs)0,whichsaysthatAssumptionTS.5holds.Sincespecifyingadynamicallycompletemodelmeansthatthereisnoserialcorre-lation,doesitfollowthatallmodelsshouldbedynamicallycomplete?AswewillseeinChapter18,forforecastingpurposes,theanswerisyes.SomethinkthatallmodelsshouldbedynamicallycompleteandthatserialcorrelationintheerrorsofamodelisQUESTION11.3asignofmisspecification.ThisstanceisIf(11.33)holdswhereutet1et1andwhere{et}isani.i.d.toorigid.Sometimes,wereallyareinter-2sequencewithmeanzeroandvariancee,canequation(11.33)bedynamicallycomplete?estedinastaticmodel(suchasaPhillipscurve)orafinitedistributedlagmodel(suchasmeasuringthelong-runpercentagechangeinwagesgivena1%increaseinproductivity).Inthenextchapter,wewillshowhowtodetectandcorrectforserialcor-relationinsuchmodels.EXAMPLE11.8(FertilityEquation)Inequation(11.27),weestimatedadistributedlagmodelforgfronpe,allowingfortwolagsofpe.Forthismodeltobedynamicallycompleteinthesenseof(11.38),neitherlagsofgfrnorfurtherlagsofpeshouldappearintheequation.Wecaneasilyseethatthisisfalsebyaddinggfr1:thecoefficientestimateis.300,anditststatisticis2.84.Thus,themodelisnotdynamicallycompleteinthesenseof(11.38).Whatshouldwemakeofthis?WewillpostponeaninterpretationofgeneralmodelswithlaggeddependentvariablesuntilChapter18.Butthefactthat(11.27)isnotdynami-callycompletesuggeststhattheremaybeserialcorrelationintheerrors.WewillseehowtotestandcorrectforthisinChapter12.368\nd7/14/997:06PMPage369Chapter11FurtherIssuesinUsingOLSwithTimeSeriesData11.5THEHOMOSKEDASTICITYASSUMPTIONFORTIMESERIESMODELSThehomoskedasticityassumptionfortimeseriesregressions,particularlyTS.4,looksverysimilartothatforcross-sectionalregressions.However,sincextcancontainlaggedyaswellaslaggedexplanatoryvariables,webrieflydiscussthemeaningofthehomo-skedasticityassumptionfordifferenttimeseriesregressions.Inthesimplestaticmodel,sayyt01ztut,(11.37)AssumptionTS.4requiresthat2Var(utzt).Therefore,eventhoughE(ytzt)isalinearfunctionofzt,Var(ytzt)mustbeconstant.Thisisprettystraightforward.InExample11.4,wesawthat,fortheAR(1)model(11.12),thehomoskedasticityassumptionis2Var(utyt1)Var(ytyt1);eventhoughE(ytyt1)dependsonyt1,Var(ytyt1)doesnot.Thus,thevariationinthedistributionofytcannotdependonyt1.Hopefully,thepatternisclearnow.Ifwehavethemodelyt01zt2yt13zt1ut,thehomoskedasticityassumptionis2Var(utzt,yt1,zt1)Var(ytzt,yt1,zt1),sothatthevarianceofutcannotdependonzt,yt1,orzt1(orsomeotherfunctionoftime).Generally,whateverexplanatoryvariablesappearinthemodel,wemustassumethatthevarianceofytgiventheseexplanatoryvariablesisconstant.Ifthemodelcon-tainslaggedyorlaggedexplanatoryvariables,thenweareexplicitlyrulingoutdynamicformsofheteroskedasticity(somethingwestudyinChapter12).But,inastaticmodel,weareonlyconcernedwithVar(ytzt).Inequation(11.37),nodirectrestrictionsareplacedon,say,Var(ytyt1).SUMMARYInthischapter,wehavearguedthatOLScanbejustifiedusingasymptoticanalysis,pro-videdcertainconditionsaremet.Ideally,thetimeseriesprocessesarestationaryandweaklydependent,althoughstationarityisnotcrucial.Weakdependenceisnecessaryforapplyingthestandardlargesampleresults,particularlythecentrallimittheorem.Processeswithdeterministictrendsthatareweaklydependentcanbeuseddirectlyinregressionanalysis,providedtimetrendsareincludedinthemodel(asinSection10.5).Asimilarstatementholdsforprocesseswithseasonality.369\nd7/14/997:06PMPage370Part2RegressionAnalysiswithTimeSeriesDataWhenthetimeseriesarehighlypersistent(theyhaveunitroots),wemustexerciseextremecautioninusingthemdirectlyinregressionmodels(unlessweareconvincedtheCLMassumptionsfromChapter10hold).Analternativetousingthelevelsistousethefirstdifferencesofthevariables.Formosthighlypersistenteconomictimeseries,thefirstdifferenceisweaklydependent.Usingfirstdifferenceschangesthenatureofthemodel,butthismethodisoftenasinformativeasamodelinlevels.Whendataarehighlypersistent,weusuallyhavemorefaithinfirst-differenceresults.InChapter18,wewillcoversomerecent,moreadvancedmethodsforusingI(1)variablesinmultipleregressionanalysis.Whenmodelshavecompletedynamicsinthesensethatnofurtherlagsofanyvari-ableareneededintheequation,wehaveseenthattheerrorswillbeseriallyuncorre-lated.Thisisusefulbecausecertainmodels,suchasautoregressivemodels,areassumedtohavecompletedynamics.Instaticanddistributedlagmodels,thedynami-callycompleteassumptionisoftenfalse,whichgenerallymeanstheerrorswillbeseri-allycorrelated.WewillseehowtoaddressthisprobleminChapter12.KEYTERMSAsymptoticallyUncorrelatedNonstationaryProcessAutoregressiveProcessofOrderOneRandomWalk[AR(1)]RandomWalkwithDriftCovarianceStationarySeriallyUncorrelatedDynamicallyCompleteModelStableAR(1)ProcessFirstDifferenceStationaryProcessHighlyPersistentStronglyDependentIntegratedofOrderOne[I(1)]Trend-StationaryProcessIntegratedofOrderZero[I(0)]UnitRootProcessMovingAverageProcessofOrderOneWeaklyDependent[MA(1)]PROBLEMS11.1Let{xt:t1,2,…}beacovariancestationaryprocessanddefinehCov(xt,xth)forh0.[Therefore,0Var(xt).]ShowthatCorr(xt,xth)h/0.11.2Let{et:t1,0,1,…}beasequenceofindependent,identicallydistributedran-domvariableswithmeanzeroandvarianceone.Defineastochasticprocessbyxtet(1/2)et1(1/2)et2,t1,2,….(i)FindE(xt)andVar(xt).Doeitherofthesedependont?(ii)ShowthatCorr(xt,xt1)1/2andCorr(xt,xt2)1/3.(Hint:ItiseasiesttousetheformulainProblem11.1.)(iii)WhatisCorr(xt,xth)forh2?(iv)Is{xt}anasymptoticallyuncorrelatedprocess?11.3Supposethatatimeseriesprocess{yt}isgeneratedbyytzet,forall2t1,2,…,where{et}isani.i.d.sequencewithmeanzeroandvariancee.Theran-370\nd7/14/997:06PMPage371Chapter11FurtherIssuesinUsingOLSwithTimeSeriesData2domvariablezdoesnotchangeovertime;ithasmeanzeroandvariancez.Assumethateachetisuncorrelatedwithz.(i)Findtheexpectedvalueandvarianceofyt.Doyouranswersdependont?(ii)FindCov(yt,yth)foranytandh.Is{yt}covariancestationary?222(iii)Useparts(i)and(ii)toshowthatCorr(yt,yth)z/(ze)foralltandh.(iv)Doesytsatisfytheintuitiverequirementforbeingasymptoticallyuncor-related?Explain.11.4Let{yt:t1,2,…}followarandomwalk,asin(11.20),withy00.ShowthatCorr(yt,yth)t/(th)fort1,h0.11.5FortheU.S.economy,letgpricedenotethemonthlygrowthintheoverallpricelevelandletgwagebethemonthlygrowthinhourlywages.[Thesearebothobtainedasdifferencesoflogarithms:gpricelog(price)andgwagelog(wage).]UsingthemonthlydatainWAGEPRC.RAW,weestimatethefollowingdistributedlagmodel:(gpriˆce.00093)(.119)gwage(.097)gwage1(.040)gwage2gpriˆce(.00057)(.052)gwage(.039)gwage1(.039)gwage2(.038)gwage3(.081)gwage4(.107)gwage5(.095)gwage6(.039)gwage3(.039)gwage4(.039)gwage5(.039)gwage60(.104)gwage7(.103)gwage8(.159)gwage9(.110)gwage100(.039)gwage7(.039)gwage8(.039)gwage9(.039)gwage10(.103)gwage11(.016)gwage12(.039)gwage11(.052)gwage1222n273,R.317,R¯.283.(i)Sketchtheestimatedlagdistribution.Atwhatlagistheeffectofgwageongpricelargest?Whichlaghasthesmallestcoefficient?(ii)Forwhichlagsarethetstatisticslessthantwo?(iii)Whatistheestimatedlong-runpropensity?Isitmuchdifferentthanone?ExplainwhattheLRPtellsusinthisexample.(iv)WhatregressionwouldyouruntoobtainthestandarderroroftheLRPdirectly?(v)Howwouldyoutestthejointsignificanceofsixmorelagsofgwage?WhatwouldbethedfsintheFdistribution?(Becarefulhere;youlosesixmoreobservations.)11.6Lethy6tdenotethethree-monthholdingyield(inpercent)frombuyingasix-monthT-billattime(t1)andsellingitattimet(threemonthshence)asathree-monthT-bill.Lethy3t1bethethree-monthholdingyieldfrombuyingathree-monthT-billattime(t1).Attime(t1),hy3t1isknown,whereashy6tisunknownbecausep3t(thepriceofthree-monthT-bills)isunknownattime(t1).Theexpecta-tionshypothesis(EH)saysthatthesetwodifferentthree-monthinvestmentsshouldbethesame,onaverage.Mathematically,wecanwritethisasaconditionalexpectation:E(hy6tIt1)hy3t1,371\nd7/14/997:06PMPage372Part2RegressionAnalysiswithTimeSeriesDatawhereIt1denotesallobservableinformationupthroughtimet1.Thissuggestsesti-matingthemodelhy6t01hy3t1ut,andtestingH0:11.(WecanalsotestH0:00,butweoftenallowforatermpre-miumforbuyingassetswithdifferentmaturities,sothat00.)(i)EstimatingthepreviousequationbyOLSusingthedatainINTQRT.RAW(spacedeverythreemonths)gives(hyˆ6t.058)(1.104)hy3t1hyˆ6t(.070)(0.039)hy3t12n123,R.866.DoyourejectH0:11againstH0:11atthe1%significancelevel?Doestheestimateseempracticallydifferentfromone?(ii)AnotherimplicationoftheEHisthatnoothervariablesdatedas(t1)orearliershouldhelpexplainhy6t,oncehy3t1hasbeencontrolledfor.Includingonelagofthespreadbetweensix-monthandthree-month,T-billratesgives(hyˆ6t.123)(1.053)hy3t1(.480)(r6t1r3t1)hyˆ6t(.067)(0.039)hy3t1(.109)(r6t1r3t1)2n123,R.885.Nowisthecoefficientonhy3t1statisticallydifferentfromone?Isthelaggedspreadtermsignificant?Accordingtothisequation,if,attime(t1),r6isabover3,shouldyouinvestinsix-monthorthree-month,T-bills?(iii)Thesamplecorrelationbetweenhy3tandhy3t1is.914.Whymightthisraisesomeconcernswiththepreviousanalysis?(iv)Howwouldyoutestforseasonalityintheequationestimatedinpart(ii)?11.7Apartialadjustmentmodelisy*t01xtetytyt1(y*tyt1)at,wherey*tisthedesiredoroptimallevelofy,andytistheactual(observed)level.Forexample,y*tisthedesiredgrowthinfirminventories,andxtisgrowthinfirmsales.Theparameter1measurestheeffectofxtony*t.Thesecondequationdescribeshowtheactualyadjustsdependingontherelationshipbetweenthedesiredyintimetandtheactualyintime(t1).Theparametermeasuresthespeedofadjustmentandsatis-fies01.(i)Plugthefirstequationfory*tintothesecondequationandshowthatwecanwriteyt01yt12xtut.372\nd7/14/997:06PMPage373Chapter11FurtherIssuesinUsingOLSwithTimeSeriesDataInparticular,findthejintermsofthejandandfindutintermsofetandat.Therefore,thepartialadjustmentmodelleadstoamodelwithalaggeddependentvariableandacontemporaneousx.(ii)IfE(etxt,yt1,xt1,…)E(atxt,yt1,xt1,…)0andallseriesareweaklydependent,howwouldyouestimatethej?(iii)Ifˆ1.7andˆ2.2,whataretheestimatesof1and?COMPUTEREXERCISES11.8UsethedatainHSEINV.RAWforthisexercise.(i)Findthefirstorderautocorrelationinlog(invpc).Nowfindtheautocor-relationafterlinearlydetrendinglog(invpc).Dothesameforlog(price).Whichofthetwoseriesmayhaveaunitroot?(ii)Basedonyourfindingsinpart(i),estimatetheequationlog(invpct)01log(pricet)2tutandreporttheresultsinstandardform.Interpretthecoefficientˆ1anddeterminewhetheritisstatisticallysignificant.(iii)Linearlydetrendlog(invpct)andusethedetrendedversionasthedepen-dentvariableintheregressionfrompart(ii)(seeSection10.5).What2happenstoR?(iv)Nowuselog(invpct)asthedependentvariable.Howdoyourresultschangefrompart(ii)?Isthetimetrendstillsignificant?Whyorwhynot?11.9InExample11.7,definethegrowthinhourlywageandoutputperhourasthechangeinthenaturallog:ghrwagelog(hrwage)andgoutphrlog(outphr).Considerasimpleextensionofthemodelestimatedin(11.29):ghrwaget01goutphrt2goutphrt1ut.Thisallowsanincreaseinproductivitygrowthtohavebothacurrentandlaggedeffectonwagegrowth.(i)EstimatetheequationusingthedatainEARNS.RAWandreporttheresultsinstandardform.Isthelaggedvalueofgoutphrstatisticallysig-nificant?(ii)If121,apermanentincreaseinproductivitygrowthisfullypassedoninhigherwagegrowthafteroneyear.TestH0:121againstthetwo-sidedalternative.Remember,theeasiestwaytodothisistowritetheequationsothat12appearsdirectlyinthemodel,asinExample10.4fromChapter10.(iii)Doesgoutphrt2needtobeinthemodel?Explain.11.10(i)InExample11.4,itmaybethattheexpectedvalueofthereturnattimet,givenpastreturns,isaquadraticfunctionofreturnt1.Tocheckthispossibility,usethedatainNYSE.RAWtoestimate2returnt01returnt12returnt1ut;reporttheresultsinstandardform.373\nd7/14/997:06PMPage374Part2RegressionAnalysiswithTimeSeriesData(ii)StateandtestthenullhypothesisthatE(returntreturnt1)doesnotdependonreturnt1.(Hint:Therearetworestrictionstotesthere.)Whatdoyouconclude?2(iii)Dropreturnt1fromthemodel,butaddtheinteractiontermreturnt1returnt2.Now,testtheefficientmarketshypothesis.(iv)Whatdoyouconcludeaboutpredictingweeklystockreturnsbasedonpaststockreturns?11.11UsethedatainPHILLIPS.RAWforthisexercise.(i)InExample11.5,weassumedthatthenaturalrateofunemploymentisconstant.AnalternativeformoftheexpectationsaugmentedPhillipscurveallowsthenaturalrateofunemploymenttodependonpastlevelsofunemployment.Inthesimplestcase,thenaturalrateattimetequalsunemt1.Ifweassumeadaptiveexpectations,weobtainaPhillipscurvewhereinflationandunemploymentareinfirstdifferences:inf01unemu.Estimatethismodel,reporttheresultsintheusualform,anddiscussthesign,size,andstatisticalsignificanceofˆ1.(ii)Whichmodelfitsthedatabetter,(11.19)orthemodelfrompart(i)?Explain.11.12(i)Addalineartimetrendtoequation(11.27).Isatimetrendnecessaryinthefirst-differenceequation?(ii)Dropthetimetrendandaddthevariablesww2andpillto(11.27)(donotdifferencethesedummyvariables).Arethesevariablesjointlysig-nificantatthe5%level?(iii)Usingthemodelfrompart(ii),estimatetheLRPandobtainitsstandarderror.Comparethisto(10.19),wheregfrandpeappearedinlevelsratherthaninfirstdifferences.11.13LetinventbetherealvalueinventoriesintheUnitedStatesduringyeart,letGDPtdenoterealgrossdomesticproduct,andletr3tdenotethe(expost)realinterestrateonthree-monthT-bills.Theexpostrealinterestrateis(approximately)r3ti3tinft,wherei3tistherateonthree-monthT-billsandinftistheannualinflationrate[seeMankiw(1994,Section6.4)].Thechangeininventories,invent,istheinventoryinvestmentfortheyear.Theacceleratormodelofinventoryinvestmentisinvent01GDPtut,where10.[See,forexample,Mankiw(1994),Chapter17.](i)UsethedatainINVEN.RAWtoestimatetheacceleratormodel.Reporttheresultsintheusualformandinterprettheequation.Isˆ1statisticallygreaterthanzero?(ii)Iftherealinterestraterises,thentheopportunitycostofholdinginven-toriesrises,andsoanincreaseintherealinterestrateshoulddecreaseinventories.Addtherealinterestratetotheacceleratormodelanddis-cusstheresults.Doestheleveloftherealinterestrateworkbetterthanthefirstdifference,r3t?374\nd7/14/997:06PMPage375Chapter11FurtherIssuesinUsingOLSwithTimeSeriesData11.14UseCONSUMP.RAWforthisexercise.Oneversionofthepermanentincomehypothesis(PIH)ofconsumptionisthatthegrowthinconsumptionisunpredictable.[Anotherversionisthatthechangeinconsumptionitselfisunpredictable;seeMankiw(1994,Chapter15)fordiscussionofthePIH.]Letgctlog(ct)log(ct1)bethegrowthinrealpercapitaconsumption(ofnondurablesandservices).ThenthePIHimpliesthatE(gctIt1)E(gct),whereIt1denotesinformationknownattime(t1);inthiscase,tdenotesayear.(i)TestthePIHbyestimatinggct01gct1ut.Clearlystatethenullandalternativehypotheses.Whatdoyouconclude?(ii)Totheregressioninpart(i),addgyt1andi3t1,wheregytisthegrowthinrealpercapitadisposableincomeandi3tistheinterestrateonthree-monthT-bills;notethateachmustbelaggedintheregression.Arethesetwoadditionalvariablesjointlysignificant?11.15UsethedatainPHILLIPS.RAWforthisexercise.(i)EstimateanAR(1)modelfortheunemploymentrate.Usethisequationtopredicttheunemploymentratefor1997.Comparethiswiththeactualunemploymentratefor1997.(YoucanfindthisinformationinarecentEconomicReportofthePresident.)(ii)AddalagofinflationtotheAR(1)modelfrompart(i).Isinft1statis-ticallysignificant?(iii)Usetheequationfrompart(ii)topredicttheunemploymentratefor1997.Istheresultbetterorworsethaninthemodelfrompart(i)?(iv)UsethemethodfromSection6.4toconstructa95%predictionintervalforthe1997unemploymentrate.Isthe1997unemploymentrateintheinterval?375\n\nd7/14/997:19PMPage376ChapterTwelveSerialCorrelationandHeteroskedasticityinTimeSeriesRegressionsnthischapter,wediscussthecriticalproblemofserialcorrelationintheerrortermsIofamultipleregressionmodel.WesawinChapter11thatwhen,inanappropriatesense,thedynamicsofamodelhavebeencompletelyspecified,theerrorswillnotbeseriallycorrelated.Thus,testingforserialcorrelationcanbeusedtodetectdynamicmisspecification.Furthermore,staticandfinitedistributedlagmodelsoftenhaveseri-allycorrelatederrorsevenifthereisnounderlyingmisspecificationofthemodel.Therefore,itisimportanttoknowtheconsequencesandremediesforserialcorrelationfortheseusefulclassesofmodels.InSection12.1,wepresentthepropertiesofOLSwhentheerrorscontainserialcor-relation.InSection12.2,wedemonstratehowtotestforserialcorrelation.Wecoverteststhatapplytomodelswithstrictlyexogenousregressorsandteststhatareasymp-toticallyvalidwithgeneralregressors,includinglaggeddependentvariables.Section12.3explainshowtocorrectforserialcorrelationundertheassumptionofstrictlyexogenousexplanatoryvariables,whileSection12.4showshowusingdifferenceddataofteneliminatesserialcorrelationintheerrors.Section12.5coversmorerecentadvancesonhowtoadjusttheusualOLSstandarderrorsandteststatisticsinthepres-enceofverygeneralserialcorrelation.InChapter8,wediscussedtestingandcorrectingforheteroskedasticityincross-sectionalapplications.InSection12.6,weshowhowthemethodsusedinthecross-sectionalcasecanbeextendedtothetimeseriescase.Themechanicsareessentiallythesame,butthereareafewsubtletiesassociatedwiththetemporalcorrelationintimeseriesobservationsthatmustbeaddressed.Inaddition,webrieflytouchontheconse-quencesofdynamicformsofheteroskedasticity.12.1PROPERTIESOFOLSWITHSERIALLYCORRELATEDERRORSUnbiasednessandConsistencyInChapter10,weprovedunbiasednessoftheOLSestimatorunderthefirstthreeGauss-Markovassumptionsfortimeseriesregressions(TS.1throughTS.3).Inpartic-ular,Theorem10.1assumednothingaboutserialcorrelationintheerrors.Itfollows376\nd7/14/997:19PMPage377Chapter12SerialCorrelationandHeteroskedasticityinTimeSeriesRegressionsthat,aslongastheexplanatoryvariablesarestrictlyexogenous,theˆjareunbiased,regardlessofthedegreeofserialcorrelationintheerrors.Thisisanalogoustotheobservationthatheteroskedasticityintheerrorsdoesnotcausebiasintheˆj.InChapter11,werelaxedthestrictexogeneityassumptiontoE(utxt)0andshowedthat,whenthedataareweaklydependent,theˆjarestillconsistent(althoughnotnecessarilyunbiased).Thisresultdidnothingeonanyassumptionaboutserialcor-relationintheerrors.EfficiencyandInferenceSincetheGauss-Markovtheorem(Theorem10.4)requiresbothhomoskedasticityandseriallyuncorrelatederrors,OLSisnolongerBLUEinthepresenceofserialcorrela-tion.Evenmoreimportantly,theusualOLSstandarderrorsandteststatisticsarenotvalid,evenasymptotically.WecanseethisbycomputingthevarianceoftheOLSesti-matorunderthefirstfourGauss-MarkovassumptionsandtheAR(1)modelfortheerrorterms.Moreprecisely,weassumethatutut1et,t1,2,…,n(12.1)1,(12.2)2wheretheetareuncorrelatedrandomvariableswithmeanzeroandvariancee;recallfromChapter11thatassumption(12.2)isthestabilitycondition.WeconsiderthevarianceoftheOLSslopeestimatorinthesimpleregressionmodelyt01xtut,and,justtosimplifytheformula,weassumethatthesampleaverageofthextiszero(x¯0).ThentheOLSestimatorˆ1of1canbewrittenasn1ˆ11SSTxxtut,(12.3)t1n2whereSSTxxt.Now,incomputingthevarianceofˆ1(conditionalonX),wemustt1accountfortheserialcorrelationintheut:nn222Var(ˆ1)SSTxVarxtutSSTxxtVar(ut)t1t1n1nt2xtxtjE(ututj)(12.4)t1j1n1nt222j/SSTx2(/SSTx)xtxtj,t1j12j2whereVar(ut)andwehaveusedthefactthatE(ututj)Cov(ut,utj)[see2equation(11.4)].Thefirstterminequation(12.4),/SSTx,isthevarianceofˆ1when0,whichisthefamiliarOLSvarianceundertheGauss-Markovassumptions.Ifwe377\nd7/14/997:19PMPage378Part2RegressionAnalysiswithTimeSeriesDataignoretheserialcorrelationandestimatethevarianceintheusualway,thevarianceestimatorwillusuallybebiasedwhen0becauseitignoresthesecondtermin(12.4).Aswewillseethroughlaterexamples,0ismostcommon,inwhichcase,j0forallj.Further,theindependentvariablesinregressionmodelsareoftenposi-tivelycorrelatedovertime,sothatxtxtjispositiveformostpairstandtj.Therefore,n1ntjinmosteconomicapplications,thetermxtxtjispositive,andsotheusualOLSt1j12varianceformula/SSTxunderestimatesthetruevarianceoftheOLSestimator.Ifislargeorxthasahighdegreeofpositiveserialcorrelation—acommoncase—thebiasintheusualOLSvarianceestimatorcanbesubstantial.WewilltendtothinktheOLSslopeestimatorismoreprecisethanitactuallyis.jWhen0,isnegativewhenjisoddandpositivewhenjiseven,andsoitisn1ntjdifficulttodeterminethesignofxtxtj.Infact,itispossiblethattheusualOLSt1j1varianceformulaactuallyoverstatesthetruevarianceofˆ1.Ineithercase,theusualvarianceestimatorwillbebiasedforVar(ˆ1)inthepresenceofserialcorrelation.Becausethestandarderrorofˆ1isanestimateofthestandarddeviationofˆ1,QUESTION12.1usingtheusualOLSstandarderrorintheSupposethat,ratherthantheAR(1)model,utfollowstheMA(1)presenceofserialcorrelationisinvalid.modelutetet1.FindVar(ˆ1)andshowthatitisdifferentTherefore,tstatisticsarenolongervalidfromtheusualformulaif0.fortestingsinglehypotheses.Sinceasmallerstandarderrormeansalargertsta-tistic,theusualtstatisticswilloftenbetoolargewhen0.TheusualFandLMsta-tisticsfortestingmultiplehypothesesarealsoinvalid.SerialCorrelationinthePresenceofLaggedDependentVariablesBeginnersineconometricsareoftenwarnedofthedangersofseriallycorrelatederrorsinthepresenceoflaggeddependentvariables.Almosteverytextbookoneconometricscontainssomeformofthestatement“OLSisinconsistentinthepresenceoflaggeddependentvariablesandseriallycorrelatederrors.”Unfortunately,asageneralasser-tion,thisstatementisfalse.Thereisaversionofthestatementthatiscorrect,butitisimportanttobeveryprecise.Toillustrate,supposethattheexpectedvalueofyt,givenyt1,islinear:E(ytyt1)01yt1,(12.5)whereweassumestability,11.Weknowwecanalwayswritethiswithanerrortermasyt01yt1ut,(12.6)E(utyt1)0.(12.7)Byconstruction,thismodelsatisfiesthekeyAssumptionTS.3forconsistencyofOLS,andthereforetheOLSestimatorsˆ0andˆ1areconsistent.Itisimportanttoseethat,378\nd7/14/997:19PMPage379Chapter12SerialCorrelationandHeteroskedasticityinTimeSeriesRegressionswithoutfurtherassumptions,theerrors{ut}canbeseriallycorrelated.Condition(12.7)ensuresthatutisuncorrelatedwithyt1,bututandyt2couldbecorrelated.Then,sinceut1yt101yt2,thecovariancebetweenutandut1is1Cov(ut,yt2),whichisnotnecessarilyzero.Thus,theerrorsexhibitserialcorrelationandthemodelcontainsalaggeddependentvariable,butOLSconsistentlyestimates0and1becausethesearetheparametersintheconditionalexpectation(12.5).TheserialcorrelationintheerrorswillcausetheusualOLSstatisticstobeinvalidfortestingpurposes,butitwillnotaffectconsistency.SowhenisOLSinconsistentiftheerrorsareseriallycorrelatedandtheregressorscontainalaggeddependentvariable?Thishappenswhenwewritethemodelinerrorform,exactlyasin(12.6),butthenweassumethat{ut}followsastableAR(1)modelasin(12.1)and(12.2),whereE(etut1,ut2,…)E(etyt1,yt2,…)0.(12.8)Sinceetisuncorrelatedwithyt1byassumption,Cov(yt1,ut)Cov(yt1,ut1),whichisnotzerounless0.ThiscausestheOLSestimatorsof0and1fromtheregressionofytonyt1tobeinconsistent.WenowseethatOLSestimationof(12.6),whentheerrorsutalsofollowanAR(1)model,leadstoinconsistentestimators.However,thecorrectnessofthisstatementmakesitnolesswrongheaded.Wehavetoask:Whatwouldbethepointinestimatingtheparametersin(12.6)whentheerrorsfollowanAR(1)model?Itisdifficulttothinkofcaseswherethiswouldbeinteresting.Atleastin(12.5)theparameterstellustheexpectedvalueofytgivenyt1.Whenwecombine(12.6)and(12.1),weseethatytreallyfollowsasecondorderautoregressivemodel,orAR(2)model.Toseethis,writeut1yt101yt2andplugthisintoutut1et.Then,(12.6)canberewrittenasyt01yt1(yt101yt2)et0(1)(1)yt11yt2et01yt12yt2et,where00(1),11,and21.Given(12.8),itfollowsthatE(ytyt1,yt2,…)E(ytyt1,yt2)01yt12yt2.(12.9)Thismeansthattheexpectedvalueofyt,givenallpasty,dependsontwolagsofy.Itisequation(12.9)thatwewouldbeinterestedinusingforanypracticalpurpose,includ-ingforecasting,aswewillseeinChapter18.Weareespeciallyinterestedintheparam-etersj.UndertheappropriatestabilityconditionsforanAR(2)model—wewillcovertheseinSection12.3—OLSestimationof(12.9)producesconsistentandasymptoti-callynormalestimatorsofthej.Thebottomlineisthatyouneedagoodreasonforhavingbothalaggeddependentvariableinamodelandaparticularmodelofserialcorrelationintheerrors.Oftense-rialcorrelationintheerrorsofadynamicmodelsimplyindicatesthatthedynamicregressionfunctionhasnotbeencompletelyspecified:inthepreviousexample,weshouldaddyt2totheequation.379\nd7/14/997:19PMPage380Part2RegressionAnalysiswithTimeSeriesDataInChapter18,wewillseeexamplesofmodelswithlaggeddependentvariableswheretheerrorsareseriallycorrelatedandarealsocorrelatedwithyt1.Buteveninthesecases,theerrorsdonotfollowanautoregressiveprocess.12.2TESTINGFORSERIALCORRELATIONInthissection,wediscussseveralmethodsoftestingforserialcorrelationintheerrortermsinthemultiplelinearregressionmodelyt01xt1…kxtkut.Wefirstconsiderthecasewhentheregressorsarestrictlyexogenous.Recallthatthisrequirestheerror,ut,tobeuncorrelatedwiththeregressorsinalltimeperiods(seeSection10.3),andso,amongotherthings,itrulesoutmodelswithlaggeddependentvariables.AttestforAR(1)SerialCorrelationwithStrictlyExogenousRegressorsWhiletherearenumerouswaysinwhichtheerrortermsinamultipleregressionmodelcanbeseriallycorrelated,themostpopularmodel—andthesimplesttoworkwith—istheAR(1)modelinequations(12.1)and(12.2).Intheprevioussection,weexplainedtheimplicationsofperformingOLSwhentheerrorsareseriallycorrelatedingeneral,andwederivedthevarianceoftheOLSslopeestimatorinasimpleregressionmodelwithAR(1)errors.WenowshowhowtotestforthepresenceofAR(1)serialcorrela-tion.Thenullhypothesisisthatthereisnoserialcorrelation.Therefore,justaswithtestsforheteroskedasticity,weassumethebestandrequirethedatatoprovidereason-ablystrongevidencethattheidealassumptionofnoserialcorrelationisviolated.Wefirstderivealargesampletest,undertheassumptionthattheexplanatoryvari-ablesarestrictlyexogenous:theexpectedvalueofut,giventheentirehistoryofinde-pendentvariables,iszero.Inaddition,in(12.1),wemustassumethatE(etut1,ut2,…)0(12.10)and2Var(etut1)Var(et)e.(12.11)ThesearestandardassumptionsintheAR(1)model(whichfollowwhen{et}isani.i.d.sequence),andtheyallowustoapplythelargesampleresultsfromChapter11fordynamicregression.Aswithtestingforheteroskedasticity,thenullhypothesisisthattheappropriateGauss-Markovassumptionistrue.IntheAR(1)model,thenullhypothesisthattheerrorsareseriallyuncorrelatedisH0:0.(12.12)Howcanwetestthishypothesis?Iftheutwereobserved,then,under(12.10)and(12.11),wecouldimmediatelyapplytheasymptoticnormalityresultsfromTheorem11.2tothedynamicregressionmodel380\nd7/14/997:19PMPage381Chapter12SerialCorrelationandHeteroskedasticityinTimeSeriesRegressionsutut1et,t2,…,n.(12.13)(Underthenullhypothesis0,{ut}isclearlyweaklydependent.)Inotherwords,wecouldestimatefromtheregressionofutonut1,forallt2,…,n,withoutaninter-cept,andusetheusualtstatisticforˆ.Thisdoesnotworkbecausetheerrorsutarenotobserved.Nevertheless,justaswithtestingforheteroskedasticity,wecanreplaceutwiththecorrespondingOLSresidual,uˆt.SinceuˆtdependsontheOLSestimatorsˆ0,ˆ1,…,ˆk,itisnotobviousthatusinguˆtforutintheregressionhasnoeffectonthedis-tributionofthetstatistic.Fortunately,itturnsoutthat,becauseofthestrictexogeneityassumption,thelargesampledistributionofthetstatisticisnotaffectedbyusingtheOLSresidualsinplaceoftheerrors.Aproofiswell-beyondthescopeofthistext,butitfollowsfromtheworkofWooldridge(1991b).WecansummarizetheasymptotictestforAR(1)serialcorrelationverysimply:TESTINGFORAR(1)SERIALCORRELATIONWITHSTRICTLYEXOGENOUSREGRESSORS:(i)RuntheOLSregressionofytonxt1,…,xtkandobtaintheOLSresiduals,uˆt,forallt1,2,…,n.(ii)Runtheregressionofuˆtonuˆt1,forallt2,…,n,(12.14)obtainingthecoefficientˆonuˆt1anditststatistic,tˆ.(Thisregressionmayormaynotcontainanintercept;thetstatisticforˆwillbeslightlyaffected,butitisasymptoticallyvalideitherway.)(iii)UsetˆtotestH0:0againstH1:0intheusualway.(Actually,since0isoftenexpectedapriori,thealternativecanbeH0:0.)Typically,wecon-cludethatserialcorrelationisaproblemtobedealtwithonlyifH0isrejectedatthe5%level.Asalways,itisbesttoreportthep-valueforthetest.Indecidingwhetherserialcorrelationneedstobeaddressed,weshouldrememberthedifferencebetweenpracticalandstatisticalsignificance.Withalargesamplesize,itispossibletofindserialcorrelationeventhoughˆispracticallysmall;whenˆisclosetozero,theusualOLSinferenceprocedureswillnotbefaroff[seeequation(12.4)].Suchoutcomesaresomewhatrareintimeseriesapplicationsbecausetimeseriesdatasetsareusuallysmall.EXAMPLE12.1[TestingforAR(1)SerialCorrelationinthePhillipsCurve]InChapter10,weestimatedastaticPhillipscurvethatexplainedtheinflation-unemploymenttradeoffintheUnitedStates(seeExample10.1).InChapter11,westudiedaparticularexpectationsaugmentedPhillipscurve,whereweassumedadaptiveexpecta-tions(seeExample11.5).Wenowtesttheerrortermineachequationforserialcorrelation.Sincetheexpectationsaugmentedcurveusesinftinftinft1asthedependentvari-able,wehaveonefewerobservation.381\nd7/14/997:19PMPage382Part2RegressionAnalysiswithTimeSeriesDataForthestaticPhillipscurve,theregressionin(12.14)yieldsˆ.573,t4.93,andp-value.000(with48observations).Thisisverystrongevidenceofpositive,firstorderserialcorrelation.OneconsequenceofthisisthatthestandarderrorsandtstatisticsfromChapter10arenotvalid.Bycontrast,thetestforAR(1)serialcorrelationintheexpecta-tionsaugmentedcurvegivesˆ.036,t.297,andp-value.775(with47obser-vations):thereisnoevidenceofAR(1)serialcorrelationintheexpectationsaugmentedPhillipscurve.Althoughthetestfrom(12.14)isderivedfromtheAR(1)model,thetestcandetectotherkindsofserialcorrelation.Remember,ˆisaconsistentestimatorofthecorrela-tionbetweenutandut1.Anyserialcorrelationthatcausesadjacenterrorstobecorre-latedcanbepickedupbythistest.Ontheotherhand,itdoesnotdetectserialcorrelationwhereadjacenterrorsareuncorrelated,Corr(ut,ut1)0.(Forexample,utandut2couldbecorrelated.)Inusingtheusualtstatisticfrom(12.14),wemustassumethattheerrorsin(12.13)satisfytheappropriatehomoskedasticityassumption,(12.11).Infact,itiseasytomakethetestrobusttoheteroskedasticityinet:wesimplyusetheusual,heteroskedasticity-QUESTION12.2robusttstatisticfromChapter8.FortheHowwouldyouuseregression(12.14)toconstructanapproximatestaticPhillipscurveinExample12.1,the95%confidenceintervalfor?heteroskedasticity-robusttstatisticis4.03,whichissmallerthanthenonrobusttsta-tisticbutstillverysignificant.InSection12.6,wefurtherdiscussheteroskedasticityintimeseriesregressions,includingitsdynamicforms.TheDurbin-WatsonTestUnderClassicalAssumptionsAnothertestforAR(1)serialcorrelationistheDurbin-Watsontest.TheDurbin-Watson(DW)statisticisalsobasedontheOLSresiduals:n2(uˆtuˆt1)t2DW.(12.15)n2uˆtt1SimplealgebrashowsthatDWandˆfrom(12.14)arecloselylinked:DW2(1ˆ).(12.16)n2Onereasonthisrelationshipisnotexactisthatˆhasuˆt1initsdenominator,whilet2theDWstatistichasthesumofsquaresofallOLSresidualsinitsdenominator.Evenwithmoderatesamplesizes,theapproximationin(12.16)isoftenprettyclose.Therefore,testsbasedonDWandthettestbasedonˆareconceptuallythesame.382\nd7/14/997:19PMPage383Chapter12SerialCorrelationandHeteroskedasticityinTimeSeriesRegressionsDurbinandWatson(1950)derivethedistributionofDW(conditionalonX),some-thingthatrequiresthefullsetofclassicallinearmodelassumptions,includingnormal-ityoftheerrorterms.Unfortunately,thisdistributiondependsonthevaluesoftheindependentvariables.(Italsodependsonthesamplesize,thenumberofregressors,andwhethertheregressioncontainsanintercept.)Whilesomeeconometricspackagestabulatecriticalvaluesandp-valuesforDW,manydonot.Inanycase,theydependonthefullsetofCLMassumptions.Severaleconometricstextsreportupperandlowerboundsforthecriticalvaluesthatdependonthedesiredsignificancelevel,thealternativehypothesis,thenumberofobservations,andthenumberofregressors.(Weassumethataninterceptisincludedinthemodel.)Usually,theDWtestiscomputedforthealternativeH1:0.(12.17)Fromtheapproximationin(12.16),ˆ0impliesthatDW2,andˆ0impliesthatDW2.Thus,torejectthenullhypothesis(12.12)infavorof(12.17),wearelookingforavalueofDWthatissignificantlylessthantwo.Unfortunately,becauseoftheprob-lemsinobtainingthenulldistributionofDW,wemustcompareDWwithtwosetsofcriticalvalues.TheseareusuallylabelledasdU(forupper)anddL(forlower).IfDWdL,thenwerejectH0infavorof(12.17);ifDWdU,wefailtorejectH0.IfdLDWdU,thetestisinconclusive.Asanexample,ifwechoosea5%significancelevelwithn45andk4,dU1.720anddL1.336[seeSavinandWhite(1977)].IfDW1.336,werejectthenullofnoserialcorrelationatthe5%level;ifDW1.72,wefailtorejectH0;if1.336DW1.72,thetestisinconclusive.InExample12.1,forthestaticPhillipscurve,DWiscomputedtobeDW.80.Wecanobtainthelower1%criticalvaluefromSavinandWhite(1977)fork1andn50:dL1.32.Therefore,werejectthenullofnoserialcorrelationagainstthealterna-tiveofpositiveserialcorrelationatthe1%level.(Usingthepreviousttest,wecancon-cludethatthep-valueequalszerotothreedecimalplaces.)FortheexpectationsaugmentedPhillipscurve,DW1.77,whichiswellwithinthefail-to-rejectregionateventhe5%level(dU1.59).ThefactthatanexactsamplingdistributionforDWcanbetabulatedistheonlyadvantagethatDWhasoverthettestfrom(12.14).Giventhatthetabulatedcriticalval-uesareexactlyvalidonlyunderthefullsetofCLMassumptionsandthattheycanleadtoawideinconclusiveregion,thepracticaldisadvantagesoftheDWaresubstantial.Thetstatisticfrom(12.14)issimpletocomputeandasymptoticallyvalidwithoutnor-mallydistributederrors.Thetstatisticisalsovalidinthepresenceofheteroskedastic-itythatdependsonthextj;anditiseasytomakeitrobusttoanyformofhet-eroskedasticity.TestingforAR(1)SerialCorrelationwithoutStrictlyExogenousRegressorsWhentheexplanatoryvariablesarenotstrictlyexogenous,sothatoneormorextjiscor-relatedwithut1,neitherthettestfromregression(12.14)northeDurbin-Watson383\nd7/14/997:19PMPage384Part2RegressionAnalysiswithTimeSeriesDatastatisticarevalid,eveninlargesamples.Theleadingcaseofnonstrictlyexogenousregressorsoccurswhenthemodelcontainsalaggeddependentvariable:yt1andut1areobviouslycorrelated.Durbin(1970)suggestedtwoalternativestotheDWstatisticwhenthemodelcontainsalaggeddependentvariableandtheotherregressorsarenon-random(or,moregenerally,strictlyexogenous).ThefirstiscalledDurbin’shstatistic.Thisstatistichasapracticaldrawbackinthatitcannotalwaysbecomputed,andsowedonotcoverithere.Durbin’salternativestatisticissimpletocomputeandisvalidwhenthereareanynumberofnon-strictlyexogenousexplanatoryvariables.Thetestalsoworksiftheexplanatoryvariableshappentobestrictlyexogenous.TESTINGFORSERIALCORRELATIONWITHGENERALREGRESSORS:(i)RuntheOLSregressionofytonxt1,…,xtkandobtaintheOLSresiduals,uˆt,forallt1,2,…,n.(ii)Runtheregressionofutonxt1,xt2,…,xtk,uˆt1,forallt2,…,n.(12.18)toobtainthecoefficientˆonuˆt1anditststatistic,tˆ.(iii)UsetˆtotestH0:0againstH1:0intheusualway(oruseaone-sidedalternative).Inequation(12.18),weregresstheOLSresidualsonallindependentvariables,includ-inganintercept,andthelaggedresidual.Thetstatisticonthelaggedresidualisavalid2testof(12.12)intheAR(1)model(12.13)(whenweaddVar(utxt,ut1)underH0).Anynumberoflaggeddependentvariablesmayappearamongthextj,andothernon-strictlyexogenousexplanatoryvariablesareallowedaswell.Theinclusionofxt1,…,xtkexplicitlyallowsforeachxtjtobecorrelatedwithut1,andthisensuresthattˆhasanapproximatetdistributioninlargesamples.Thetstatis-ticfrom(12.14)ignorespossiblecorrelationbetweenxtjandut1,soitisnotvalidwith-outstrictlyexogenousregressors.Incidentally,becauseuˆtytˆ0ˆ1xt1…ˆkxtk,itcanbeshownthatthetstatisticonuˆt1isthesameifytisusedinplaceofuˆtasthedependentvariablein(12.18).Thetstatisticfrom(12.18)iseasilymaderobusttoheteroskedasticityofunknownform(inparticular,whenVar(utxt,ut1)isnotconstant):justusetheheteroskedasticity-robusttstatisticonuˆt1.EXAMPLE12.2[TestingforAR(1)SerialCorrelationintheMinimumWageEquation]InChapter10(seeExample10.9),weestimatedtheeffectoftheminimumwageonthePuertoRicanemploymentrate.Wenowcheckwhethertheerrorsappeartocontainserialcorrelation,usingthetestthatdoesnotassumestrictexogeneityoftheminimumwageorGNPvariables.[WeaddthelogofPuertoRicanrealGNPtoequation(10.38),asinProblem384\nd7/14/997:19PMPage385Chapter12SerialCorrelationandHeteroskedasticityinTimeSeriesRegressions10.9].Weareassumingthattheunderlyingstochasticprocessesareweaklydependent,butweallowthemtocontainalineartimetrend(byincludingtintheregression).LettinguˆtdenotetheOLSresiduals,weruntheregressionofutonlog(mincovt),log(prgnpt),log(usgnpt),t,anduˆt1,usingthe37availableobservations.Theestimatedcoefficientonuˆt1isˆ.481witht2.89(two-sidedp-value.007).Therefore,thereisstrongevidenceofAR(1)serialcorre-lationintheerrors,whichmeansthetstatisticsfortheˆjthatweobtainedbeforearenotvalidforinference.Remember,though,theˆjarestillconsistentifutiscontemporaneouslyuncorrelatedwitheachexplanatoryvariable.Incidentally,ifweuseregression(12.14)instead,weobtainˆ.417andt2.63,sotheoutcomeofthetestissimilarinthiscase.TestingforHigherOrderSerialCorrelationThetestfrom(12.18)iseasilyextendedtohigherordersofserialcorrelation.Forexample,supposethatwewishtotestH0:10,20(12.19)intheAR(2)model,ut1ut12ut2et.Thisalternativemodelofserialcorrelationallowsustotestforsecondorderserialcor-relation.Asalways,weestimatethemodelbyOLSandobtaintheOLSresiduals,uˆt.Then,wecanruntheregressionofuˆtonxt1,xt2,…,xtk,uˆt1,anduˆt2,forallt3,…,n,toobtaintheFtestforjointsignificanceofuˆt1anduˆt2.Ifthesetwolagsarejointlysignificantatasmallenoughlevel,say5%,thenwereject(12.19)andconcludethattheerrorsareseriallycorrelated.Moregenerally,wecantestforserialcorrelationintheautoregressivemodeloforderq:ut1ut12ut2…qutqet.(12.20)ThenullhypothesisisH0:10,20,…,q0.(12.21)TESTINGFORAR(q)SERIALCORRELATION:(i)RuntheOLSregressionofytonxt1,…,xtkandobtaintheOLSresiduals,uˆt,forallt1,2,…,n.(ii)Runtheregressionof385\nd7/14/997:19PMPage386Part2RegressionAnalysiswithTimeSeriesDatauˆtonxt1,xt2,…,xtk,uˆt1,uˆt2,…,uˆtq,forallt(q1),…,n.(12.22)(iii)ComputetheFtestforjointsignificanceofuˆt1,uˆt2,…,uˆtqin(12.22).[TheFstatisticwithytasthedependentvariablein(12.22)canalsobeused,asitgivesanidenticalanswer.]Ifthextjareassumedtobestrictlyexogenous,sothateachxtjisuncorrelatedwithut1,ut2,…,utq,thenthextjcanbeomittedfrom(12.22).Includingthextjintheregres-sionmakesthetestvalidwithorwithoutthestrictexogeneityassumption.Thetestrequiresthehomoskedasticityassumption2Var(utxt,ut1,…,utq).(12.23)Aheteroskedasticity-robustversioncanbecomputedasdescribedinChapter8.AnalternativetocomputingtheFtestistousetheLagrangemultiplier(LM)formofthestatistic.(WecoveredtheLMstatisticfortestingexclusionrestrictionsinChapter5forcross-sectionalanalysis.)TheLMstatisticfortesting(12.21)issimply2LM(nq)Ruˆ,(12.24)2whereRuˆisjusttheusualR-squaredfromregression(12.22).Underthenullhypothe-2sis,LM~ªq.ThisisusuallycalledtheBreusch-GodfreytestforAR(q)serialcorrela-tion.TheLMstatisticalsorequires(12.23),butitcanbemaderobusttohet-eroskedasticity.[Fordetails,seeWooldridge(1991b).EXAMPLE12.3[TestingforAR(3)SerialCorrelation]Intheeventstudyofthebariumchlorideindustry(seeExample10.5),weusedmonthlydata,sowemaywishtotestforhigherordersofserialcorrelation.Forillustrationpurposes,wetestforAR(3)serialcorrelationintheerrorsunderlyingequation(10.22).Usingregres-sion(12.22),theFstatisticforjointsignificanceofuˆt1,uˆt2,anduˆt3isF5.12.Originally,wehadn131,andwelosethreeobservationsintheauxiliaryregression(12.22).Becauseweestimate10parametersin(12.22)forthisexample,thedfintheFstatisticare3and118.Thep-valueoftheFstatisticis.0023,sothereisstrongevidenceofAR(3)serialcor-relation.Withquarterlyormonthlydatathathavenotbeenseasonallyadjusted,wesome-timeswishtotestforseasonalformsofserialcorrelation.Forexample,withquarterlydata,wemightpostulatetheautoregressivemodelut4ut4et.(12.25)FromtheAR(1)serialcorrelationtests,itisprettyclearhowtoproceed.Whentheregressorsarestrictlyexogenous,wecanuseattestonuˆt4intheregressionof386\nd7/14/997:19PMPage387Chapter12SerialCorrelationandHeteroskedasticityinTimeSeriesRegressionsuˆtonuˆt4,forallt5,…,n.AmodificationoftheDurbin-Watsonstatisticisalsoavailable[seeWallis(1972)].Whenthextjarenotstrictlyexogenous,wecanusetheregressionin(12.18),withuˆt4replacinguˆt1.InExample12.3,thedataaremonthlyandarenotseasonallyadjusted.Therefore,itmakessensetotestforcorrelationbetweenutandut12.Aregressionofuˆtonuˆt12yieldsˆ12.187andp-value.028,sothereisevidenceofnegativeseasonalauto-correlation.(IncludingtheregressorsQUESTION12.3changesthingsonlymodestly:ˆ12Supposeyouhavequarterlydataandyouwanttotestforthepres-.170andp-value.052.)Thisissome-enceoffirstorderorfourthorderserialcorrelation.Withstrictlywhatunusualanddoesnothaveanobviousexogenousregressors,howwouldyouproceed?explanation.12.3CORRECTINGFORSERIALCORRELATIONWITHSTRICTLYEXOGENOUSREGRESSORSIfwedetectserialcorrelationafterapplyingoneofthetestsinSection12.2,wehavetodosomethingaboutit.Ifourgoalistoestimateamodelwithcompletedynamics,weneedtorespecifythemodel.Inapplicationswhereourgoalisnottoestimateafullydynamicmodel,weneedtofindawaytocarryoutstatisticalinference:aswesawinSection12.1,theusualOLSteststatisticsarenolongervalid.Inthissection,webeginwiththeimportantcaseofAR(1)serialcorrelation.Thetraditionalapproachtothisproblemassumesfixedregressors.Whatareactuallyneededarestrictlyexogenousregressors.Therefore,ataminimum,weshouldnotusethesecorrectionswhentheexplanatoryvariablesincludelaggeddependentvariables.ObtainingtheBestLinearUnbiasedEstimatorintheAR(1)ModelWeassumetheGauss-MarkovAssumptionsTS.1throughTS.4,butwerelaxAssump-tionTS.5.Inparticular,weassumethattheerrorsfollowtheAR(1)modelutut1et,forallt1,2,….(12.26)RememberthatAssumptionTS.2impliesthatuthasazeromeanconditionalonX.Inthefollowinganalysis,welettheconditioningonXbeimpliedinordertosimplifythenotation.Thus,wewritethevarianceofutas22Var(ut)e/(1).(12.27)Forsimplicity,considerthecasewithasingleexplanatoryvariable:yt01xtut,forallt1,2,…,n.Sincetheprobleminthisequationisserialcorrelationintheut,itmakessensetotrans-formtheequationtoeliminatetheserialcorrelation.Fort2,wewrite387\nd7/14/997:19PMPage388Part2RegressionAnalysiswithTimeSeriesDatayt101xt1ut1yt01xtut.Now,ifwemultiplythisfirstequationbyandsubtractitfromthesecondequation,wegetytyt1(1)01(xtxt1)et,t2,wherewehaveusedthefactthatetutut1.Wecanwritethisasy˜t(1)01x˜tet,t2,(12.28)wherey˜tytyt1,x˜txtxt1(12.29)arecalledthequasi-differenceddata.(If1,thesearedifferenceddata,butremem-berweareassuming1.)Theerrortermsin(12.28)areseriallyuncorrelated;infact,thisequationsatisfiesalloftheGauss-Markovassumptions.Thismeansthat,ifweknew,wecouldestimate0and1byregressingy˜tonx˜t,providedwedividetheesti-matedinterceptby(1).TheOLSestimatorsfrom(12.28)arenotquiteBLUEbecausetheydonotusethefirsttimeperiod.Thisiseasilyfixedbywritingtheequationfort1asy101x1u1.(12.30)Sinceeachetisuncorrelatedwithu1,wecanadd(12.30)to(12.28)andstillhaveseri-222allyuncorrelatederrors.However,using(12.27),Var(u1)e/(1)eVar(et).[Equation(12.27)clearlydoesnotholdwhen1,whichiswhyweassumethesta-21/2bilitycondition.]Thus,wemustmultiply(12.30)by(1)togeterrorswiththesamevariance:21/221/221/221/2(1)y1(1)01(1)x1(1)u1or21/2y˜1(1)01x˜1u˜1,(12.31)21/221/2whereu˜1(1)u1,y˜1(1)y1,andsoon.Theerrorin(12.31)hasvari-22anceVar(u˜1)(1)Var(u1)e,sowecanuse(12.31)alongwith(12.28)inanOLSregression.ThisgivestheBLUEestimatorsof0and1underAssumptionsTS.1throughTS.4andtheAR(1)modelforut.Thisisanotherexampleofageneralizedleastsquares(orGLS)estimator.WesawotherGLSestimatorsinthecontextofhet-eroskedasticityinChapter8.Addingmoreregressorschangesverylittle.Fort2,weusetheequationy˜t(1)01x˜t1…kx˜tket,(12.32)388\nd7/14/997:19PMPage389Chapter12SerialCorrelationandHeteroskedasticityinTimeSeriesRegressions21/221/2wherex˜tjxtjxt1,j.Fort1,wehavey˜1(1)y1,x˜1j(1)x1j,21/2andtheinterceptis(1)0.Forgiven,itisfairlyeasytotransformthedataandtocarryoutOLS.Unless0,theGLSestimator,thatis,OLSonthetransformeddata,willgenerallybedifferentfromtheoriginalOLSestimator.TheGLSestimatorturnsouttobeBLUE,and,sincetheerrorsinthetransformedequationareseriallyuncorrelatedandhomoskedastic,tandFstatisticsfromthetransformedequationarevalid(atleastasymptotically,andexactlyiftheerrorsetarenormallydistributed).FeasibleGLSEstimationwithAR(1)ErrorsTheproblemwiththeGLSestimatoristhatisrarelyknowninpractice.However,wealreadyknowhowtogetaconsistentestimatorof:wesimplyregresstheOLSresid-ualsontheirlaggedcounterparts,exactlyasinequation(12.14).Next,weusethisesti-mate,ˆ,inplaceoftoobtainthequasi-differencedvariables.WethenuseOLSontheequationy˜t0x˜t01x˜t1…kx˜tkerrort,(12.33)21/2wherex˜t0(1ˆ)fort2,andx˜10(1ˆ).ThisresultsinthefeasibleGLS(FGLS)estimatorofthej.Theerrortermin(12.33)containsetandalsothetermsinvolvingtheestimationerrorinˆ.Fortunately,theestimationerrorinˆdoesnotaffecttheasymptoticdistributionoftheFGLSestimators.FEASIBLEGLSESTIMATIONOFTHEAR(1)MODEL:(i)RuntheOLSregressionofytonxt1,…,xtkandobtaintheOLSresiduals,uˆt,t1,2,…,n.(ii)Runtheregressioninequation(12.14)andobtainˆ.(iii)ApplyOLStoequation(12.33)toestimate0,1,…,k.Theusualstandarderrors,tstatistics,andFstatisticsareasymptoticallyvalid.ThecostofusingˆinplaceofisthatthefeasibleGLSestimatorhasnotractablefinitesampleproperties.Inparticular,itisnotunbiased,althoughitisconsistentwhenthedataareweaklydependent.Further,evenifetin(12.32)isnormallydistributed,thetandFstatisticsareonlyapproximatelytandFdistributedbecauseoftheestimationerrorinˆ.Thisisfineformostpurposes,althoughwemustbecarefulwithsmallsam-plesizes.SincetheFGLSestimatorisnotunbiased,wecertainlycannotsayitisBLUE.Nevertheless,itisasymptoticallymoreefficientthantheOLSestimatorwhentheAR(1)modelforserialcorrelationholds(andtheexplanatoryvariablesarestrictlyexogenous).Again,thisstatementassumesthatthetimeseriesareweaklydependent.ThereareseveralnamesforFGLSestimationoftheAR(1)modelthatcomefromdifferentmethodsofestimatinganddifferenttreatmentofthefirstobservation.Cochrane-Orcutt(CO)estimationomitsthefirstobservationandusesˆfrom(12.14),whereasPrais-Winsten(PW)estimationusesthefirstobservationinthepre-viouslysuggestedway.Asymptotically,itmakesnodifferencewhetherornotthefirstobservationisused,butmanytimeseriessamplesaresmall,sothedifferencescanbenotableinapplications.389\nd7/14/997:19PMPage390Part2RegressionAnalysiswithTimeSeriesDataInpractice,boththeCochrane-OrcuttandPrais-Winstenmethodsareusedinaniterativescheme.OncetheFGLSestimatorisfoundusingˆfrom(12.14),wecancom-puteanewsetofresiduals,obtainanewestimatoroffrom(12.14),transformthedatausingthenewestimateof,andestimate(12.33)byOLS.Wecanrepeatthewholeprocessmanytimes,untiltheestimateofchangesbyverylittlefromthepreviousiter-ation.Manyregressionpackagesimplementaniterativeprocedureautomatically,sothereisnoadditionalworkforus.Itisdifficulttosaywhethermorethanoneiterationhelps.Itseemstobehelpfulinsomecases,but,theoretically,thelargesampleproper-tiesoftheiteratedestimatorarethesameastheestimatorthatusesonlythefirstitera-tion.Fordetailsontheseandothermethods,seeDavidsonandMacKinnon(1993,Chapter10).EXAMPLE12.4(Cochrane-OrcuttEstimationintheEventStudy)WeestimatetheequationinExample10.5usingiteratedCochrane-Orcuttestimation.Forcomparison,wealsopresenttheOLSresultsinTable12.1.ThecoefficientsthatarestatisticallysignificantintheCochrane-OrcuttestimationdonotdifferbymuchfromtheOLSestimates[inparticular,thecoefficientsonlog(chempi),log(rtwex),andafdec6].Itisnotsurprisingforstatisticallyinsignificantcoefficientstochange,perhapsmarkedly,acrossdifferentestimationmethods.Noticehowthestandarderrorsinthesecondcolumnareuniformlyhigherthanthestandarderrorsincolumn(1).Thisiscommon.TheCochrane-Orcuttstandarderrorsaccountforserialcorrelation;theOLSstandarderrorsdonot.AswesawinSection12.1,theOLSstandarderrorsusuallyunderstatetheactualsamplingvariationintheOLSesti-matesandshouldnotberelieduponwhensignificantserialcorrelationispresent.Therefore,theeffectonChineseimportsaftertheInternationalTradeCommissionsdeci-sionisnowlessstatisticallysignificantthanwethought(tafdec61.68).TheCochrane-Orcutt(CO)methodreportsonefewerobservationthanOLS;thisreflectsthefactthatthefirsttransformedobservationisnotusedintheCOmethod.Thisslightlyaffectsthedegreesoffreedomthatareusedinhypothesistests.Finally,anR-squaredisreportedfortheCOestimation,whichiswell-belowtheR-squaredfortheOLSestimationinthiscase.However,theseR-squaredsshouldnotbecompared.ForOLS,theR-squared,asusual,isbasedontheregressionwiththeuntrans-formeddependentandindependentvariables.ForCO,theR-squaredcomesfromthefinalregressionofthetransformeddependentvariableonthetransformedindependentvari-2ables.ItisnotclearwhatthisRisactuallymeasuring,nevertheless,itistraditionallyreported.ComparingOLSandFGLSInsomeapplicationsoftheCochrane-OrcuttorPrais-Winstenmethods,theFGLSesti-matesdifferinpracticallyimportantwaysfromtheOLSestimates.(Thiswasnotthe390\nd7/14/997:19PMPage391Chapter12SerialCorrelationandHeteroskedasticityinTimeSeriesRegressionsTable12.1DependentVariable:log(chnimp)CoefficientOLSCochrane-Orcuttlog(chempi)3.122.95(0.48)(0.65)log(gas).1961.05(.907)(0.99)log(rtwex).9831.14(.400)(0.51)befile6.060.016(.261)(.321)affile6.032.033(.264)(.323)afdec6.565.577(.286)(.343)intercept17.7037.31(20.05)(23.22)ˆ———.293(.084)Observations.131.130R-Squared.305.193caseinExample12.4.)Typically,thishasbeeninterpretedasaverificationoffeasibleGLS’ssuperiorityoverOLS.Unfortunately,thingsarenotsosimple.Toseewhy,con-sidertheregressionmodelyt01xtut,wherethetimeseriesprocessesarestationary.Now,assumingthatthelawoflargenumbersholds,consistencyofOLSfor1holdsifCov(xt,ut)0.(12.34)Earlier,weassertedthatFGLSwasconsistentunderthestrictexogeneityassumption,whichismorerestrictivethan(12.34).Infact,itcanbeshownthattheweakestassump-391\nd7/14/997:19PMPage392Part2RegressionAnalysiswithTimeSeriesDatationthatmustholdforFGLStobeconsistent,inadditionto(12.34),isthatthesumofxt1andxt1isuncorrelatedwithut:Cov({xt1xt1},ut)0.(12.35)Practicallyspeaking,consistencyofFGLSrequiresuttobeuncorrelatedwithxt1,xt,andxt1.ThismeansthatOLSandFGLSmightgivesignificantlydifferentestimatesbecause(12.35)fails.Inthiscase,OLS—whichisstillconsistentunder(12.34)—ispreferredtoFGLS(whichisinconsistent).Ifxhasalaggedeffectony,orxt1reactstochangesinut,FGLScanproducemisleadingresults.SinceOLSandFGLSaredifferentestimationprocedures,weneverexpectthemtogivethesameestimates.Iftheyprovidesimilarestimatesofthej,thenFGLSispre-ferredifthereisevidenceofserialcorrelation,becausetheestimatorismoreefficientandtheFGLSteststatisticsareatleastasymptoticallyvalid.AmoredifficultproblemariseswhentherearepracticaldifferencesintheOLSandFGLSestimates:itishardtodeterminewhethersuchdifferencesarestatisticallysignificant.ThegeneralmethodproposedbyHausman(1978)canbeused,butthisisbeyondthescopeofthistext.ConsistencyandasymptoticnormalityofOLSandFGLSrelyheavilyonthetimeseriesprocessesytandthextjbeingweaklydependent.StrangethingscanhappenifweapplyeitherOLSorFGLSwhensomeprocesseshaveunitroots.Wediscussthisfur-therinChapter18.EXAMPLE12.5(StaticPhillipsCurve)Table12.2presentsOLSanditeratedCochrane-OrcuttestimatesofthestaticPhillipscurvefromExample10.1.Table12.2DependentVariable:infCoefficientOLSCochrane-Orcuttunem.468.665(.289)(.320)intercept1.4247.580(1.719)(2.379)ˆ———.774(.091)Observations.49.48R-Squared.053.086392\nd7/14/997:19PMPage393Chapter12SerialCorrelationandHeteroskedasticityinTimeSeriesRegressionsThecoefficientofinterestisonunem,anditdiffersmarkedlybetweenCOandOLS.SincetheCOestimateisconsistentwiththeinflation-unemploymenttradeoff,ourtendencyistofocusontheCOestimates.Infact,theseestimatesarefairlyclosetowhatisobtainedbyfirstdifferencingbothinfandunem(seeProblem11.11),whichmakessensebecausethequasi-differencingusedinCOwithˆ.774issimilartofirstdifferencing.Itmayjustbethatinfandunemarenotrelatedinlevels,buttheyhaveanegativerelationshipinfirstdif-ferences.CorrectingforHigherOrderSerialCorrelationItisalsopossibletocorrectforhigherordersofserialcorrelation.AgeneraltreatmentisgiveninHarvey(1990).Here,weillustratetheapproachforAR(2)serialcorrelation:ut1ut12ut2et,where{et}satisfiestheassumptionsstatedfortheAR(1)model.Thestabilityconditionismorecomplicatednow.Theycanbeshowntobe[seeHarvey(1990)]21,211,and121.Forexample,themodelisstableif1.8and2.3;themodelisunstableif1.7and2.4.Assumingthestabilityconditionshold,wecanobtainthetransformationthatelim-inatestheserialcorrelation.Inthesimpleregressionmodel,thisiseasywhent2:yt1yt12yt20(112)1(xt1xt12xt2)etory˜t0(112)1x˜tet,t3,4,…,n.(12.36)Ifweknow1and2,wecaneasilyestimatethisequationbyOLSafterobtainingthetransformedvariables.Sincewerarelyknow1and2,wehavetoestimatethem.Asusual,wecanusetheOLSresiduals,uˆt:obtainˆ1andˆ2fromtheregressionofuˆtonuˆt1,uˆt2,t3,…,n.[ThisisthesameregressionusedtotestforAR(2)serialcorrelationwithstrictlyexoge-nousregressors.]Then,weuseˆ1andˆ2inplaceof1and2toobtainthetransformedvariables.ThisgivesoneversionofthefeasibleGLSestimator.Ifwehavemultipleexplanatoryvariables,theneachoneistransformedbyx˜tjxtjˆ1xt1,jˆ2xt2,j,whent2.Thetreatmentofthefirsttwoobservationsisalittletricky.Itcanbeshownthatthedependentvariableandeachindependentvariable(includingtheintercept)shouldbetransformedby221/2z˜1{(12)[(12)1]/(12)}z121/221/2z˜2(12)z2{1(11)/(12)}z1,393\nd7/14/997:19PMPage394Part2RegressionAnalysiswithTimeSeriesDatawherez1andz2denoteeitherthedependentoranindependentvariableatt1andt2,respectively.Wewillnotderivethesetransformations.Briefly,theyeliminatethese-rialcorrelationbetweenthefirsttwoobservationsandmaketheirerrorvariancesequal2toe.Fortunately,econometricspackagesgearedtowardtimeseriesanalysiseasilyesti-matemodelswithgeneralAR(q)errors;werarelyneedtodirectlycomputethetrans-formedvariablesourselves.12.4DIFFERENCINGANDSERIALCORRELATIONInChapter11,wepresenteddifferencingasatransformationformakinganintegratedprocessweaklydependent.Thereisanotherwaytoseethemeritsofdifferencingwhendealingwithhighlypersistentdata.Supposethatwestartwiththesimpleregressionmodel:yt01xtut,t1,2,…,(12.37)whereutfollowstheAR(1)process(12.26).AswementionedinSection11.3,andaswewilldiscussmorefullyinChapter18,theusualOLSinferenceprocedurescanbeverymisleadingwhenthevariablesytandxtareintegratedoforderone,orI(1).Intheextremecasewheretheerrors{ut}in(12.37)followarandomwalk,theequationmakesnosensebecause,amongotherthings,thevarianceofutgrowswitht.Itismorelogi-caltodifferencetheequation:yt1xt ut,t2,…,n.(12.38)Ifutfollowsarandomwalk,thenetuthaszeromean,aconstantvariance,andisseriallyuncorrelated.Thus,assumingthatetandxtareuncorrelated,wecanestimate(12.38)byOLS,wherewelosethefirstobservation.Evenifutdoesnotfollowarandomwalk,butispositiveandlarge,firstdiffer-encingisoftenagoodidea:itwilleliminatemostoftheserialcorrelation.Ofcourse,(12.38)isdifferentfrom(12.37),butatleastwecanhavemorefaithintheOLSstan-darderrorsandtstatisticsin(12.38).Allowingformultipleexplanatoryvariablesdoesnotchangeanything.EXAMPLE12.6(DifferencingtheInterestRateEquation)InExample10.2,weestimatedanequationrelatingthethree-month,T-billratetoinflationandthefederaldeficit[seeequation(10.15)].Ifweregresstheresidualsfromthisequationonasinglelag,weobtainˆ.530(.123),whichisstatisticallygreaterthanzero.Ifwedif-ferencei3,inf,anddefandthenchecktheresidualsforAR(1)serialcorrelation,weobtainˆ.068(.145),andsothereisnoevidenceofserialcorrelation.Thedifferencinghasappar-entlyeliminatedanyserialcorrelation.[Inaddition,thereisevidencethati3containsaunitroot,andinfmayaswell,sodifferencingmightbeneededtoproduceI(0)variablesanyway.]394\nd7/14/997:19PMPage395Chapter12SerialCorrelationandHeteroskedasticityinTimeSeriesRegressionsAsweexplainedinChapter11,theQUESTION12.4decisionofwhetherornottodifferenceisSupposeafterestimatingamodelbyOLSthatyouestimatefromatoughone.Butthisdiscussionpointsoutregression(12.14)andyouobtainˆ.92.Whatwouldyoudoanotherbenefitofdifferencing,whichisaboutthis?thatitremovesserialcorrelation.WewillcomebacktothisissueinChapter18.12.5SERIALCORRELATION-ROBUSTINFERENCEAFTEROLSInrecentyears,ithasbecomemorepopulartoestimatemodelsbyOLSbuttocorrectthestandarderrorsforfairlyarbitraryformsofserialcorrelation(andheteroskedastic-ity).EventhoughweknowOLSwillbeinefficient,therearesomegoodreasonsfortak-ingthisapproach.First,theexplanatoryvariablesmaynotbestrictlyexogenous.Inthiscase,FGLSisnotevenconsistent,letaloneefficient.Second,inmostapplicationsofFGLS,theerrorsareassumedtofollowanAR(1)model.ItmaybebettertocomputestandarderrorsfortheOLSestimatesthatarerobusttomoregeneralformsofserialcorrelation.Togettheidea,considerequation(12.4),whichisthevarianceoftheOLSslopeestimatorinasimpleregressionmodelwithAR(1)errors.Wecanestimatethisvariance2verysimplybyplugginginourstandardestimatorsofand.TheonlyproblemwiththisisthatitassumestheAR(1)modelholdsandalsohomoskedasticity.Itispossibletorelaxbothoftheseassumptions.Ageneraltreatmentofstandarderrorsthatarebothheteroskedasticityandserialcorrelation-robustisgiveninDavidsonandMacKinnon(1993).Rightnow,weprovideasimplemethodtocomputetherobuststandarderrorofanyOLScoefficient.OurtreatmentherefollowsWooldridge(1989).Considerthestandardmultiplelin-earregressionmodelyt01xt1…kxtkut,t1,2,…,n,(12.39)whichwehaveestimatedbyOLS.Forconcreteness,weareinterestedinobtainingaserialcorrelation-robuststandarderrorforˆ1.Thisturnsouttobefairlyeasy.Writext1asalinearfunctionoftheremainingindependentvariablesandanerrorterm,xt102xt2…kxtkrt,(12.40)wheretheerrorrthaszeromeanandisuncorrelatedwithxt2,xt3,…,xtk.Then,itcanbeshownthattheasymptoticvarianceoftheOLSestimatorˆ1isn2n2Avar(ˆ1)E(rt)Varrtut.t1t1UnderthenoserialcorrelationAssumptionTS.5,{atrtut}isseriallyuncorrelated,andsoeithertheusualOLSstandarderrors(underhomoskedasticity)ortheheteroskedasticity-robuststandarderrorswillbevalid.ButifTS.5fails,ourexpressionforAvar(ˆ1)mustaccountforthecorrelationbetweenatandas,whents.Inprac-395\nd7/14/997:19PMPage396Part2RegressionAnalysiswithTimeSeriesDatatice,itiscommontoassumethat,oncethetermsarefartherapartthanafewperiods,thecorrelationisessentiallyzero.Rememberthatunderweakdependence,thecorrela-tionmustbeapproachingzero,sothisisareasonableapproach.FollowingthegeneralframeworkofNeweyandWest(1987),Wooldridge(1989)showsthatAvar(ˆ1)canbeestimatedasfollows.Let“se(ˆ1)”denotetheusual(butincorrect)OLSstandarderrorandletˆbetheusualstandarderroroftheregression(orrootmeansquarederror)fromestimating(12.39)byOLS.Letrˆtdenotetheresidualsfromtheauxiliaryregressionofxt1onxt2,xt3,…,xtk(12.41)(includingaconstant,asusual).Forachosenintegerg0,definengn2vˆaˆt2[1h/(g1)]aˆtaˆth,(12.42)t1h1th1whereaˆtrˆtuˆt,t1,2,…,n.Thislookssomewhatcomplicated,butinpracticeitiseasytoobtain.Theintegergin(12.42)controlshowmuchserialcorrelationweareallowingincomputingthestandarderror.Oncewehavevˆ,theserialcorrelation-robuststandarderrorofˆ1issimply2se(ˆ1)[“se(ˆ1)”/ˆ]vˆ.(12.43)Inotherwords,wetaketheusualOLSstandarderrorofˆ1,divideitbyˆ,squaretheresult,andthenmultiplybythesquarerootofvˆ.Thiscanbeusedtoconstructconfi-denceintervalsandtstatisticsforˆ1.Itisusefultoseewhatvˆlookslikeinsomesimplecases.Wheng1,nn2vˆaˆtaˆtaˆt1,(12.44)t1t2andwheng2,nnn2vˆaˆt(4/3)aˆtaˆt1(2/3)aˆtaˆt2.(12.45)t1t2t3Thelargerthatgis,themoretermsareincludedtocorrectforserialcorrelation.Thepurposeofthefactor[1h/(g1)]in(12.42)istoensurethatvˆisinfactnonnega-tive[NeweyandWest(1987)verifythis].Weclearlyneedvˆ0,sincevˆisestimatingavarianceandthesquarerootofvˆappearsin(12.43).Thestandarderrorin(12.43)alsoturnsouttoberobusttoarbitraryheteroskedas-ticity.Infact,ifwedropthesecondtermin(12.42),then(12.43)becomestheusual396\nd7/14/997:19PMPage397Chapter12SerialCorrelationandHeteroskedasticityinTimeSeriesRegressionsheteroskedasticity-robuststandarderrorthatwediscussedinChapter8(withoutthedegreesoffreedomadjustment).Thetheoryunderlyingthestandarderrorin(12.43)istechnicalandsomewhatsub-tle.Remember,westartedoffbyclaimingwedonotknowtheformofserialcorrela-tion.Ifthisisthecase,howcanweselecttheintegerg?Theorystatesthat(12.43)worksforfairlyarbitraryformsofserialcorrelation,providedggrowswithsamplesizen.Theideaisthat,withlargersamplesizes,wecanbemoreflexibleabouttheamountofcor-relationin(12.42).Therehasbeenmuchrecentworkontherelationshipbetweengandn,butwewillnotgointothathere.Forannualdata,choosingasmallg,suchasg1org2,islikelytoaccountformostoftheserialcorrelation.Forquarterlyormonthlydata,gshouldprobablybelarger(suchasg4or8forquarterly,g12or24formonthly),assumingthatwehaveenoughdata.NeweyandWest(1987)recommendtak-2/91/4inggtobetheintegerpartof4(n/100);othershavesuggestedtheintegerpartofn.®TheNewey-WestsuggestionisimplementedbytheeconometricsprogramEviews.For,say,n50(whichisreasonableforannual,postwardatafromWorldWarII),1/4g3.(Theintegerpartofngivesg2.)Wesummarizehowtoobtainaserialcorrelation-robuststandarderrorforˆ1.Ofcourse,sincewecanlistanyindependentvariablefirst,thefollowingprocedureworksforcomputingastandarderrorforanyslopecoefficient.SERIALCORRELATION-ROBUSTSTANDARDERRORFORˆ1:(i)Estimate(12.39)byOLS,whichyields“se(ˆ1)”,ˆ,andtheOLSresiduals{uˆt:t1,…,n}.(ii)Computetheresiduals{rˆt:t1,…,n}fromtheauxiliaryregression(12.41).Thenformaˆtrˆtuˆt(foreacht).(iii)Foryourchoiceofg,computevˆasin(12.42).(iv)Computese(ˆ1)from(12.43).Empirically,theserialcorrelation-robuststandarderrorsaretypicallylargerthantheusualOLSstandarderrorswhenthereisserialcorrelation.Thisisbecause,inmostcases,theerrorsarepositivelyseriallycorrelated.However,itispossibletohavesub-stantialserialcorrelationin{ut}buttoalsohavesimilaritiesintheusualandSC-robuststandarderrorsofsomecoefficients:itisthesampleautocorrelationsofaˆtrˆtuˆtthatdeterminetherobuststandarderrorforˆ1.TheuseofSC-robuststandarderrorshaslaggedbehindtheuseofstandarderrorsrobustonlytoheteroskedasticityforseveralreasons.First,largecrosssections,wheretheheteroskedasticity-robuststandarderrorswillhavegoodproperties,aremorecom-monthanlargetimeseries.TheSC-robuststandarderrorscanbepoorlybehavedwhenthereissubstantialserialcorrelationandthesamplesizeissmall.(Wheresmallcanevenbeaslargeas,say,100.)Second,sincewemustchoosetheintegerginequation(12.42),computationoftheSC-robuststandarderrorsisnotautomatic.Asmentionedearlier,someeconometricspackageshaveautomatedtheselection,butyoustillhavetoabidebythechoice.AnotherimportantreasonthatSC-robuststandarderrorsarenotyetroutinelycom-putedisthat,inthepresenceofsevereserialcorrelation,OLScanbeveryinefficient,especiallyinsmallsamplesizes.AfterperformingOLSandcorrectingthestandard397\nd7/14/997:19PMPage398Part2RegressionAnalysiswithTimeSeriesDataerrorsforserialcorrelation,thecoefficientsareofteninsignificant,oratleastlesssig-nificantthantheywerewiththeusualOLSstandarderrors.TheSC-robuststandarderrorsafterOLSestimationaremostusefulwhenwehavedoubtsaboutsomeoftheexplanatoryvariablesbeingstrictlyexogenous,sothatmeth-odssuchasCochrane-Orcuttarenotevenconsistent.ItisalsovalidtousetheSC-robuststandarderrorsinmodelswithlaggeddependentvariablesassuming,ofcourse,thatthereisgoodreasonforallowingserialcorrelationinsuchmodels.EXAMPLE12.7(ThePuertoRicanMinimumWage)WeobtainanSC-robuststandarderrorfortheminimumwageeffectinthePuertoRicanemploymentequation.InExample12.2,wefoundprettystrongevidenceofAR(1)serialcorrelation.Asinthatexample,weuseasadditionalcontrolslog(usgnp),log(prgnp),andalineartimetrend.TheOLSestimateoftheelasticityoftheemploymentratewithrespecttotheminimumwageisˆ1.2123,andtheusualOLSstandarderroris“se(ˆ1)”.0402.Thestandarderroroftheregressionisˆ.0328.Further,usingthepreviousprocedurewithg2[see(12.45)],weobtainvˆ.000805.ThisgivestheSC/heteroskedasticity-robuststandarderror2asse(ˆ1)[(.0402/.0328)].000805.0426.Interestingly,therobuststandarderrorisonlyslightlygreaterthantheusualOLSstandarderror.Therobusttstatisticisabout4.98,andsotheestimatedelasticityisstillverystatisticallysignificant.Forcomparison,theiteratedCOestimateof1is.1111,withastandarderrorof.0446.Thus,theFGLSestimateismuchclosertozerothantheOLSestimate,andwemightsuspectviolationofthestrictexogeneityassumption.Or,thedifferenceintheOLSandFGLSestimatesmightbeexplainablebysamplingerror.Itisverydifficulttotell.Beforeleavingthissection,wenotethatitispossibletoconstructserialcorrelation-robust,F-typestatisticsfortestingmultiplehypotheses,butthesearetooadvancedtocoverhere.[SeeWooldridge(1991b,1995)andDavidsonandMacKinnon(1993)fortreatments.]12.6HETEROSKEDASTICITYINTIMESERIESREGRESSIONSWediscussedtestingandcorrectingforheteroskedasticityforcross-sectionalapplica-tionsinChapter8.Heteroskedasticitycanalsooccurintimeseriesregressionmodels,andthepresenceofheteroskedasticity,whilenotcausingbiasorinconsistencyintheˆj,doesinvalidatetheusualstandarderrors,tstatistics,andFstatistics.Thisisjustasinthecross-sectionalcase.Intimeseriesregressionapplications,heteroskedasticityoftenreceiveslittle,ifany,attention:theproblemofseriallycorrelatederrorsisusuallymorepressing.Never-theless,itisusefultobrieflycoversomeoftheissuesthatariseinapplyingtestsandcorrectionsforheteroskedasticityintimeseriesregressions.398\nd7/14/997:19PMPage399Chapter12SerialCorrelationandHeteroskedasticityinTimeSeriesRegressionsSincetheusualOLSstatisticsareasymptoticallyvalidunderAssumptionsTS.1throughTS.5,weareinterestedinwhathappenswhenthehomoskedasticityassump-tion,TS.4,doesnothold.AssumptionTS.2rulesoutmisspecificationssuchasomit-tedvariablesandcertainkindsofmeasurementerror,whileTS.5rulesoutserialcorrelationintheerrors.Itisimportanttorememberthatseriallycorrelatederrorscauseproblemswhichtestsandadjustmentsforheteroskedasticityarenotabletoaddress.Heteroskedasticity-RobustStatisticsInstudyingheteroskedasticityforcross-sectionalregressions,wenotedhowithasnobearingontheunbiasednessorconsistencyoftheOLSestimators.Exactlythesameconclusionsholdinthetimeseriescase,aswecanseebyreviewingtheassumptionsneededforunbiasedness(Theorem10.1)andconsistency(Theorem11.1).InSection8.2,wediscussedhowtheusualOLSstandarderrors,tstatistics,andFstatisticscanbeadjustedtoallowforthepresenceofheteroskedasticityofunknownform.ThesesameadjustmentsworkfortimeseriesregressionsunderAssumptionsTS.1,TS.2,TS.3,andTS.5.Thus,providedtheonlyassumptionviolatedisthehomoskedasticityassumption,validinferenceiseasilyobtainedinmosteconometricpackages.TestingforHeteroskedasticitySometimes,wewishtotestforheteroskedasticityintimeseriesregressions,especiallyifweareconcernedabouttheperformanceofheteroskedasticity-robuststatisticsinrel-ativelysmallsamplesizes.ThetestswecoveredinChapter8canbeapplieddirectly,butwithafewcaveats.First,theerrorsutshouldnotbeseriallycorrelated;anyserialcorrelationwillgenerallyinvalidateatestforheteroskedasticity.Thus,itmakessensetotestforserialcorrelationfirst,usingaheteroskedasticity-robusttestifheteroskedas-ticityissuspected.Then,aftersomethinghasbeendonetocorrectforserialcorrelation,wecantestforheteroskedasticity.Second,considertheequationusedtomotivatetheBreusch-Pagantestforhet-eroskedasticity:2ut01xt1…kxtkvt,(12.46)2wherethenullhypothesisisH0:12…k0.FortheFstatistic—withuˆt2replacingutasthedependentvariable—tobevalid,wemustassumethattheerrors{vt}arethemselveshomoskedastic(asinthecross-sectionalcase)andseriallyuncorrelated.Theseareimplicitlyassumedincomputingallstandardtestsforheteroskedasticity,includingtheversionoftheWhitetestwecoveredinSection8.3.Assumingthatthe{vt}areseriallyuncorrelatedrulesoutcertainformsofdynamicheteroskedasticity,somethingwewilltreatinthenextsubsection.Ifheteroskedasticityisfoundintheut(andtheutarenotseriallycorrelated),thentheheteroskedasticity-robustteststatisticscanbeused.Analternativeistouseweightedleastsquares,asinSection8.4.Themechanicsofweightedleastsquaresforthetimeseriescaseareidenticaltothoseforthecross-sectionalcase.399\nd7/14/997:19PMPage400Part2RegressionAnalysiswithTimeSeriesDataEXAMPLE12.8(HeteroskedasticityandtheEfficientMarketsHypothesis)InExample11.4,weestimatedthesimplemodelreturnt01returnt1ut.(12.47)TheEMHstatesthat10.WhenwetestedthishypothesisusingthedatainNYSE.RAW,weobtainedt1.55withn689.With1suchalargesample,thisisnotmuchevi-QUESTION12.5denceagainsttheEMH.WhiletheEMHHowwouldyoucomputetheWhitetestforheteroskedasticityinstatesthattheexpectedreturngivenpastequation(12.47)?observableinformationshouldbeconstant,itsaysnothingabouttheconditionalvari-ance.Infact,theBreusch-Pagantestforheteroskedasticityentailsregressingthesquared2OLSresidualsuˆtonreturnt1:2uˆt(4.66)(1.104)returnt1residualt2uˆt(0.43)(0.201)returnt1residualt(12.48)2n689,R.042.Thetstatisticonreturnt1isabout5.5,indicatingstrongevidenceofheteroskedasticity.Becausethecoefficientonreturnt1isnegative,wehavetheinterestingfindingthatvolatil-ityinstockreturnsislowerwhenthepreviousreturnwashigh,andviceversa.Therefore,wehavefoundwhatiscommoninmanyfinancialstudies:theexpectedvalueofstockreturnsdoesnotdependonpastreturns,butthevarianceofreturnsdoes.AutoregressiveConditionalHeteroskedasticityInrecentyears,economistshavebecomeinterestedindynamicformsofheteroskedas-ticity.Ofcourse,ifxtcontainsalaggeddependentvariable,thenheteroskedasticityasin(12.46)isdynamic.Butdynamicformsofheteroskedasticitycanappeareveninmodelswithnodynamicsintheregressionequation.Toseethis,considerasimplestaticregressionmodel:yt01ztut,andassumethattheGauss-Markovassumptionshold.ThismeansthattheOLSesti-matorsareBLUE.ThehomoskedasticityassumptionsaysthatVar(utZ)isconstant,whereZdenotesallnoutcomesofzt.EvenifthevarianceofutgivenZisconstant,thereareotherwaysthatheteroskedasticitycanarise.Engle(1982)suggestedlookingattheconditionalvarianceofutgivenpasterrors(wheretheconditioningonZisleftimplicit).Englesuggestedwhatisknownastheautoregressiveconditionalhet-eroskedasticity(ARCH)model.ThefirstorderARCHmodelis400\nd7/14/997:19PMPage401Chapter12SerialCorrelationandHeteroskedasticityinTimeSeriesRegressions222E(utut1,ut2,…)E(utut1)01ut1,(12.49)whereweleavetheconditioningonZimplicit.Thisequationrepresentstheconditionalvarianceofutgivenpastut,onlyifE(utut1,ut2,…)0,whichmeansthattheerrorsareseriallyuncorrelated.Sinceconditionalvariancesmustbepositive,thismodelonlymakessenseif00and10;if10,therearenodynamicsinthevarianceequation.Itisinstructivetowrite(12.49)as22ut01ut1vt,(12.50)wheretheexpectedvalueofvt(givenut1,ut2,…)iszerobydefinition.(Thevtare2notindependentofpastutbecauseoftheconstraintvt01ut1.)Equation2(12.50)lookslikeanautoregressivemodelinut(hencethenameARCH).Thestabilityconditionforthisequationis11,justasintheusualAR(1)model.When10,thesquarederrorscontain(positive)serialcorrelationeventhoughtheutthemselvesdonot.Whatimplicationsdoes(12.50)haveforOLS?SincewebeganbyassumingtheGauss-Markovassumptionshold,OLSisBLUE.Further,evenifutisnotnormallydis-tributed,weknowthattheusualOLSteststatisticsareasymptoticallyvalidunderAssumptionsTS.1throughTS.5,whicharesatisfiedbystaticanddistributedlagmod-elswithARCHerrors.IfOLSstillhasdesirablepropertiesunderARCH,whyshouldwecareaboutARCHformsofheteroskedasticityinstaticanddistributedlagmodels?Weshouldbecon-cernedfortworeasons.First,itispossibletogetconsistent(butnotunbiased)estima-torsofthejthatareasymptoticallymoreefficientthantheOLSestimators.Aweightedleastsquaresprocedure,basedonestimating(12.50),willdothetrick.Amaximumlikelihoodprocedurealsoworksundertheassumptionthattheerrorsuthaveacondi-tionalnormaldistribution.Second,economistsinvariousfieldshavebecomeinterestedindynamicsintheconditionalvariance.Engle’sorginalapplicationwastothevarianceofUnitedKingdominflation,wherehefoundthatalargermagnitudeoftheerrorinthe2previoustimeperiod(largerut1)wasassociatedwithalargererrorvarianceinthecur-rentperiod.Sincevarianceisoftenusedtomeasurevolatility,andvolatilityisakeyele-mentinassetpricingtheories,ARCHmodelshavebecomeimportantinempiricalfinance.ARCHmodelsalsoapplywhentherearedynamicsintheconditionalmean.Supposewehavethedependentvariable,yt,acontemporaneousexogenousvariable,zt,andE(ytzt,yt1,zt1,yt2,…)01zt2yt13zt1,sothatatmostonelagofyandzappearsinthedynamicregression.ThetypicalapproachistoassumethatVar(ytzt,yt1,zt1,yt2,…)isconstant,aswediscussedinChapter11.ButthisvariancecouldfollowanARCHmodel:Var(ytzt,yt1,zt1,yt2,…)Var(utzt,yt1,zt1,yt2,…)201ut1,401\nd7/14/997:19PMPage402Part2RegressionAnalysiswithTimeSeriesDatawhereutytE(ytzt,yt1,zt1,yt2,…).AsweknowfromChapter11,thepresenceofARCHdoesnotaffectconsistencyofOLS,andtheusualheteroskedasticity-robuststandarderrorsandteststatisticsarevalid.(Remember,thesearevalidforanyformofheteroskedasticity,andARCHisjustoneparticularformofheteroskedasticity.)IfyouareinterestedintheARCHmodelanditsextensions,seeBollerslev,Chou,andKroner(1992)andBollerslev,Engle,andNelson(1994)forrecentsurveys.EXAMPLE12.9(ARCHinStockReturns)InExample12.8,wesawthattherewasheteroskedasticityinweeklystockreturns.Thishet-eroskedasticityisactuallybettercharacterizedbytheARCHmodelin(12.50).Ifwecom-putetheOLSresidualsfrom(12.47),squarethese,andregressthemonthelaggedsquaredresidual,weobtain22uˆt(2.95)(.337)uˆt1residualt22uˆt(0.44)(.036)uˆt1residualt(12.51)2n688,R.114.2Thetstatisticonuˆt1isovernine,indicatingstrongARCH.Aswediscussedearlier,alargererrorattimet1impliesalargervarianceinstockreturnstoday.Itisimportanttoseethat,whilethesquaredOLSresidualsareautocorrelated,theOLSresidualsthemselvesarenot(asisconsistentwiththeEMH).Regressinguˆtonuˆt1givesˆ.0014withtˆ.038.HeteroskedasticityandSerialCorrelationinRegressionModelsNothingrulesoutthepossibilityofbothheteroskedasticityandserialcorrelationbeingpresentinaregressionmodel.Ifweareunsure,wecanalwaysuseOLSandcomputefullyrobuststandarderrors,asdescribedinSection12.5.Muchofthetimeserialcorrelationisviewedasthemostimportantproblem,becauseitusuallyhasalargerimpactonstandarderrorsandtheefficiencyofestimatorsthandoesheteroskedasticity.AsweconcludedinSection12.2,obtainingtestsforserialcor-relationthatarerobusttoarbitraryheteroskedasticityisfairlystraightforward.Ifwedetectserialcorrelationusingsuchatest,wecanemploytheCochrane-Orcutttransfor-mation[seeequation(12.32)]and,inthetransformedequation,useheteroskedasticity-robuststandarderrorsandteststatistics.Or,wecaneventestforheteroskedasticityin(12.32)usingtheBreusch-PaganorWhitetests.Alternatively,wecanmodelheteroskedasticityandserialcorrelation,andcorrectforboththroughacombinedweightedleastsquaresAR(1)procedure.Specifically,con-siderthemodel402\nd7/14/997:19PMPage403Chapter12SerialCorrelationandHeteroskedasticityinTimeSeriesRegressionsyt01xt1…kxtkututhtvt(12.52)vtvt1et,1,wheretheexplanatoryvariablesXareindependentofetforallt,andhtisafunctionof2thextj.Theprocess{et}haszeromean,constantvariancee,andisseriallyuncorre-lated.Therefore,{vt}satisfiesastableAR(1)process.Suppressingtheconditioningontheexplanatoryvariables,wehave2Var(ut)vht,222whereve/(1).Butvtut/htishomoskedasticandfollowsastableAR(1)model.Therefore,thetransformedequationyt/ht0(1/ht)1(xt1/ht)…k(xtk/ht)vt(12.53)hasAR(1)errors.Now,ifwehaveaparticularkindofheteroskedasticityinmind—thatis,weknowht—wecanestimate(12.52)usingstandardCOorPWmethods.Inmostcases,wehavetoestimatehtfirst.ThefollowingmethodcombinestheweightedleastsquaresmethodfromSection8.4withtheAR(1)serialcorrelationcor-rectionfromSection12.3.FEASIBLEGLSWITHHETEROSKEDASTICITYANDAR(1)SERIALCORRELATION:(i)Estimate(12.52)byOLSandsavetheresiduals,uˆt.22(ii)Regresslog(uˆt)onxt1,…,xtk(oronyˆt,yˆt)andobtainthefittedvalues,saygˆt.(iii)Obtaintheestimatesofht:hˆtexp(gˆt).(iv)Estimatethetransformedequation1/21/21/21/2hˆtythˆt01hˆtxt1…khˆtxtkerrort(12.54)bystandardCochrane-OrcuttorPrais-Winstenmethods.ThesefeasibleGLSestimatorsareasymptoticallyefficient.Moreimportantly,allstandarderrorsandteststatisticsfromtheCOorPWmethodsareasymptoticallyvalid.SUMMARYWehavecoveredtheimportantproblemofserialcorrelationintheerrorsofmultipleregressionmodels.Positivecorrelationbetweenadjacenterrorsiscommon,especiallyinstaticandfinitedistributedlagmodels.ThiscausestheusualOLSstandarderrorsandstatisticstobemisleading(althoughtheˆjcanstillbeunbiased,oratleastconsistent).Typically,theOLSstandarderrorsunderestimatethetrueuncertaintyintheparameterestimates.ThemostpopularmodelofserialcorrelationistheAR(1)model.Usingthisasthestartingpoint,itiseasytotestforthepresenceofAR(1)serialcorrelationusingthe403\nd7/14/997:19PMPage404Part2RegressionAnalysiswithTimeSeriesDataOLSresiduals.AnasymptoticallyvalidtstatisticisobtainedbyregressingtheOLSresidualsonthelaggedresiduals,assumingtheregressorsarestrictlyexogenousandahomoskedasticityassumptionholds.Makingthetestrobusttoheteroskedasticityissim-ple.TheDurbin-Watsonstatisticisavailableundertheclassicallinearmodelassump-tions,butitcanleadtoaninconclusiveoutcome,andithaslittletoofferoverthettest.Formodelswithalaggeddependentvariable,orothernonstrictlyexogenousregres-sors,thestandardttestonuˆt1isstillvalid,providedallindependentvariablesareincludedasregressorsalongwithuˆt1.WecanuseanForanLMstatistictotestforhigherorderserialcorrelation.Inmodelswithstrictlyexogenousregressors,wecanuseafeasibleGLSproce-dure—Cochrane-OrcuttorPrais-Winsten—tocorrectforAR(1)serialcorrelation.ThisgivesestimatesthataredifferentfromtheOLSestimates:theFGLSestimatesareobtainedfromOLSonquasi-differencedvariables.Alloftheusualteststatisticsfromthetransformedequationareasymptoticallyvalid.Almostallregressionpackageshavebuilt-infeaturesforestimatingmodelswithAR(1)errors.Anotherwaytodealwithserialcorrelation,especiallywhenthestrictexogeneityassumptionmightfail,istouseOLSbuttocomputeserialcorrelation-robuststandarderrors(thatarealsorobusttoheteroskedasticity).ManyregressionpackagesfollowamethodsuggestedbyNeweyandWest(1987);itisalsopossibletousestandardregres-sionpackagestoobtainonestandarderroratatime.Finally,wediscussedsomespecialfeaturesofheteroskedasticityintimeseriesmodels.Asinthecross-sectionalcase,themostimportantkindofheteroskedasticityisthatwhichdependsontheexplanatoryvariables;thisiswhatdetermineswhethertheusualOLSstatisticsarevalid.TheBreusch-PaganandWhitetestscoveredinChapter8canbeapplieddirectly,withthecaveatthattheerrorsshouldnotbeseriallycorre-lated.Inrecentyears,economists—especiallythosewhostudythefinancialmarkets—havebecomeinterestedindynamicformsofheteroskedasticity.TheARCHmodelistheleadingexample.KEYTERMSAutoregressiveConditionalFeasibleGLS(FGLS)Heteroskedasticity(ARCH)Prais-Winsten(PW)EstimationBreusch-GodfreyTestQuasi-DifferencedDataCochrane-Orcutt(CO)EstimationSerialCorrelation-RobustStandardErrorDurbin-Watson(DW)StatisticWeightedLeastSquaresPROBLEMS12.1WhentheerrorsinaregressionmodelhaveAR(1)serialcorrelation,whydotheOLSstandarderrorstendtounderestimatethesamplingvariationintheˆj?IsitalwaystruethattheOLSstandarderrorsaretoosmall?12.2Explainwhatiswrongwiththefollowingstatement:“TheCochrane-OrcuttandPrais-WinstenmethodsarebothusedtoobtainvalidstandarderrorsfortheOLSesti-mates.”404\nd7/14/997:19PMPage405Chapter12SerialCorrelationandHeteroskedasticityinTimeSeriesRegressions12.3InExample10.6,weestimatedavariantonFair’smodelforpredictingpresiden-tialelectionoutcomesintheUnitedStates.(i)Whatargumentcanbemadefortheerrorterminthisequationbeingseriallyuncorrelated.(Hint:Howoftendopresidentialelectionstakeplace?)(ii)WhentheOLSresidualsfrom(10.23)areregressedonthelaggedresid-uals,weobtainˆ.068andse(ˆ).240.Whatdoyouconcludeaboutserialcorrelationintheut?(iii)Doesthesmallsamplesizeinthisapplicationworryyouintestingforserialcorrelation?12.4TrueorFalse:“IftheerrorsinaregressionmodelcontainARCH,theymustbeseriallycorrelated.”12.5(i)IntheenterprisezoneeventstudyinProblem10.11,aregressionoftheOLSresidualsonthelaggedresidualsproducesˆ.841andse(ˆ).053.WhatimplicationsdoesthishaveforOLS?(ii)IfyouwanttouseOLSbutalsowanttoobtainavalidstandarderrorfortheEZcoefficient,whatwouldyoudo?12.6InExample12.8,wefoundevidenceofheteroskedasticityinutinequation(12.47).Thus,wecomputetheheteroskedasticity-robuststandarderrors(in[])alongwiththeusualstandarderrors:retuˆrnt(.180)(.059)returnt1retuˆrnt(.081)(.038)returnt1retuˆrnt[.085][.069]returnt122n689,R.0035,R¯.0020.Whatdoesusingtheheteroskedasticity-robusttstatisticdotothesignificanceofreturnt1?COMPUTEREXERCISES12.7InExample11.6,weestimatedafiniteDLmodelinfirstdifferences:gfrt00pet1pet12pet2ut.UsethedatainFERTIL3.RAWtotestwhetherthereisAR(1)serialcorrelationintheerrors.12.8(i)UsingthedatainWAGEPRC.RAW,estimatethedistributedlagmodelfromProblem11.5.Useregression(12.14)totestforAR(1)serialcorrelation.(ii)ReestimatethemodelusingiteratedCochrane-Orcuttestimation.Whatisyournewestimateofthelong-runpropensity?(iii)UsingiteratedCO,findthestandarderrorfortheLRP.(Thisrequiresyoutoestimateamodifiedequation.)DeterminewhethertheestimatedLRPisstatisticallydifferentfromoneatthe5%level.405\nd7/14/997:19PMPage406Part2RegressionAnalysiswithTimeSeriesData12.9(i)Inpart(i)ofProblem11.13,youwereaskedtoestimatetheacceleratormodelforinventoryinvestment.TestthisequationforAR(1)serialcorrelation.(ii)Ifyoufindevidenceofserialcorrelation,reestimatetheequationbyCochrane-Orcuttandcomparetheresults.12.10(i)UseNYSE.RAWtoestimateequation(12.48).Lethˆtbethefittedvaluesfromthisequation(theestimatesoftheconditionalvariance).Howmanyhˆtarenegative?2(ii)Addreturnt1to(12.48)andagaincomputethefittedvalues,hˆt.Areanyhˆtnegative?(iii)Usethehˆtfrompart(ii)toestimate(12.47)byweightedleastsquares(asinSection8.4).Compareyourestimateof1withthatinequation(11.16).TestH0:10andcomparetheoutcomewhenOLSisused.(iv)Now,estimate(12.47)byWLS,usingtheestimatedARCHmodelin(12.51)toobtainthehˆt.Doesthischangeyourfindingsfrompart(iii)?12.11ConsidertheversionofFair’smodelinExample10.6.Now,ratherthanpredict-ingtheproportionofthetwo-partyvotereceivedbytheDemocrat,estimatealinearprobabilitymodelforwhetherornottheDemocratwins.(i)Usethebinaryvariabledemwinsinplaceofdemvotein(10.23)andreporttheresultsinstandardform.Whichfactorsaffecttheprobabilityofwinning?Usethedataonlythrough1992.(ii)Howmanyfittedvaluesarelessthanzero?Howmanyaregreaterthanone?(iii)Usethefollowingpredictionrule:ifdemwˆins.5,youpredicttheDemocratwins;otherwise,theRepublicanwins.Usingthisrule,deter-minehowmanyofthe20electionsarecorrectlypredictedbythemodel.(iv)Pluginthevaluesoftheexplanatoryvariablesfor1996.WhatisthepredictedprobabilitythatClintonwouldwintheelection?Clintondidwin;didyougetthecorrectprediction?(v)Useaheteroskedasticity-robustttestforAR(1)serialcorrelationintheerrors.Whatdoyoufind?(vi)Obtaintheheteroskedasticity-robuststandarderrorsfortheestimatesinpart(i).Aretherenotablechangesinanytstatistics?12.12(i)InProblem10.13,youestimatedasimplerelationshipbetweenconsumptiongrowthandgrowthindisposableincome.TesttheequationforAR(1)serialcorrelation(usingCONSUMP.RAW).(ii)InProblem11.14,youtestedthepermanentincomehypothesisbyregressingthegrowthinconsumptionononelag.Afterrunningthisregression,testforheteroskedasticitybyregressingthesquaredresidu-2alsongct1andgct1.Whatdoyouconclude?406\n\nd7/14/997:25PMPage408ChapterThirteenPoolingCrossSectionsAcrossTime.SimplePanelDataMethodspuntilnow,wehavecoveredmultipleregressionanalysisusingpurecross-Usectionalorpuretimeseriesdata.Whilethesetwocasesariseofteninapplica-tions,datasetsthathavebothcross-sectionalandtimeseriesdimensionsarebeingusedmoreandmoreofteninempiricalresearch.Multipleregressionmethodscanstillbeusedonsuchdatasets.Infact,datawithcross-sectionalandtimeseriesaspectscanoftenshedlightonimportantpolicyquestions.Wewillseeseveralexamplesinthischapter.Wewillanalyzetwokindsofdatasetsinthischapter.Anindependentlypooledcrosssectionisobtainedbysamplingrandomlyfromalargepopulationatdifferentpointsintime(usually,butnotnecessarily,differentyears).Forinstance,ineachyear,wecandrawarandomsampleonhourlywages,education,experience,andsoon,fromthepopulationofworkingpeopleintheUnitedStates.Or,ineveryotheryear,wedrawarandomsampleonthesellingprice,squarefootage,numberofbathrooms,andsoon,ofhousessoldinaparticularmetropolitanarea.Fromastatisticalstandpoint,thesedatasetshaveanimportantfeature:theyconsistofindependentlysampledobservations.Thiswasalsoakeyaspectinouranalysisofcross-sectionaldata:amongotherthings,itrulesoutcorrelationintheerrortermsfordifferentobservations.Anindependentlypooledcrosssectiondiffersfromasinglerandomsampleinthatsamplingfromthepopulationatdifferentpointsintimelikelyleadstoobservationsthatarenotidenticallydistributed.Forexample,distributionsofwagesandeducationhavechangedovertimeinmostcountries.Aswewillsee,thisiseasytodealwithinpracticebyallowingtheinterceptinamultipleregressionmodel,andinsomecasestheslopes,tochangeovertime.WecoversuchmodelsinSection13.1.InSection13.2,wediscusshowpoolingcrosssectionsovertimecanbeusedtoevaluatepolicychanges.Apaneldataset,whilehavingbothacross-sectionalandatimeseriesdimension,differsinsomeimportantrespectsfromanindependentlypooledcrosssection.Tocol-lectpaneldata—sometimescalledlongitudinaldata—wefollow(orattempttofol-low)thesameindividuals,families,firms,cities,states,orwhatever,acrosstime.Forexample,apaneldatasetonindividualwages,hours,education,andotherfactorsiscollectedbyrandomlyselectingpeoplefromapopulationatagivenpointintime.Then,thesesamepeoplearereinterviewedatseveralsubsequentpointsintime.This408\nd7/14/997:25PMPage409Chapter13PoolingCrossSectionsAcrossTime.SimplePanelDataMethodsgivesusdataonwages,hours,education,andsoon,forthesamegroupofpeopleindifferentyears.Paneldatasetsarefairlyeasytocollectforschooldistricts,cities,counties,states,andcountries,andpolicyanalysisisgreatlyenhancedbyusingpaneldatasets;wewillseesomeexamplesinthefollowingdiscussion.Fortheeconometricanalysisofpaneldata,wecannotassumethattheobservationsareindependentlydistributedacrosstime.Forexample,unobservedfactors(suchasability)thataffectsomeone’swagein1990willalsoaffectthatperson’swagein1991;unobservedfactorsthataffectacity’scrimeratein1985willalsoaffectthatcity’scrimeratein1990.Forthisreason,specialmod-elsandmethodshavebeendevelopedtoanalyzepaneldata.InSections13.3,13.4,and13.5,wedescribethestraightforwardmethodofdifferencingtoremovetime-constant,unobservedattributesoftheunitsbeingstudied.Becausepaneldatamethodsaresome-whatmoreadvanced,wewillrelymostlyonintuitionindescribingthestatisticalprop-ertiesoftheestimationprocedures,leavingdetailstothechapterappendix.WefollowthesamestrategyinChapter14,whichcoversmorecomplicatedpaneldatamethods.13.1POOLINGINDEPENDENTCROSSSECTIONSACROSSTIMEManysurveysofindividuals,families,andfirmsarerepeatedatregularintervals,ofteneachyear.AnexampleistheCurrentPopulationSurvey(orCPS),whichrandomlysampleshouseholdseachyear.(See,forexample,CPS78_85.RAW,whichcontainsdatafromthe1978and1985CPS.)Ifarandomsampleisdrawnateachtimeperiod,pool-ingtheresultingrandomsamplesgivesusanindependentlypooledcrosssection.Onereasonforusingindependentlypooledcrosssectionsistoincreasethesamplesize.Bypoolingrandomsamplesdrawnfromthesamepopulation,butatdifferentpointsintime,wecangetmorepreciseestimatorsandteststatisticswithmorepower.Poolingishelpfulinthisregardonlyinsofarastherelationshipbetweenthedependentvariableandatleastsomeoftheindependentvariablesremainsconstantovertime.Asmentionedintheintroduction,usingpooledcrosssectionsraisesonlyminorsta-tisticalcomplications.Typically,toreflectthefactthatthepopulationmayhavediffer-entdistributionsindifferenttimeperiods,weallowtheintercepttodifferacrossperiods,usuallyyears.Thisiseasilyaccomplishedbyincludingdummyvariablesforallbutoneyear,wheretheearliestyearinthesampleisusuallychosenasthebaseyear.Itisalsopossiblethattheerrorvariancechangesovertime,somethingwediscusslater.Sometimes,thepatternofcoefficientsontheyeardummyvariablesisitselfofinter-est.Forexample,ademographermaybeinterestedinthefollowingquestion:Aftercon-trollingforeducation,hasthepatternoffertilityamongwomenoverage35changedbetween1972and1984?Thefollowingexampleillustrateshowthisquestionissimplyansweredbyusingmultipleregressionanalysiswithyeardummyvariables.EXAMPLE13.1(Women’sFertilityOverTime)ThedatasetinFERTIL1.RAW,whichissimilartothatusedbySander(1994),comesfromtheNationalOpinionResearchCenter’sGeneralSocialSurveyfortheevenyearsfrom1972409\nd7/14/997:25PMPage410Part3AdvancedTopicsto1984,inclusively.Weusethesedatatoestimateamodelexplainingthetotalnumberofkidsborntoawoman(kids).Onequestionofinterestis:Aftercontrollingforotherobservablefactors,whathashap-penedtofertilityratesovertime?Thefactorswecontrolforareyearsofeducation,age,race,regionofthecountrywherelivingatage16,andlivingenvironmentatage16.TheestimatesaregiveninTable13.1.Thebaseyearis1972.Thecoefficientsontheyeardummyvariablesshowasharpdropinfertilityintheearly1980s.Forexample,thecoefficientony82impliesthat,holdingedu-cation,age,andotherfactorsfixed,awomanhadonaverage.52lesschildren,oraboutone-halfachild,in1982thanin1972.Thisisaverylargedrop:holdingeduc,age,andtheotherfactorsfixed,100womenin1982arepredictedtohaveabout52fewerchildrenthan100comparablewomenin1972.Sincewearecontrollingforeducation,thisdropisseparatefromthedeclineinfertilitythatisduetotheincreaseinaverageeducationlevels.(Theaver-ageyearsofeducationare12.2for1972and13.3for1984.)Thecoefficientsony82andy84representdropsinfertilityforreasonsthatarenotcapturedintheexplanatoryvariables.Giventhatthe1982and1984yeardummiesareindividuallyquitesignificant,itisnotsurprisingthatasagrouptheyeardummiesarejointlyverysignificant:theR-squaredfortheregressionwithouttheyeardummiesis.1019,andthisleadstoF6,11115.87andp-value0.Womenwithmoreeducationhavefewerchildren,andtheestimateisverystatisticallysignificant.Otherthingsbeingequal,100womenwithacollegeeducationwillhaveabout51fewerchildrenonaveragethan100womenwithonlyahighschooleducation:.128(4).512.Agehasadiminishingeffectonfertility.(Theturningpointinthequadraticisataboutage46,bywhichtimemostwomenhavefinishedhavingchildren.)ThemodelestimatedinTable13.1assumesthattheeffectofeachexplanatoryvariable,particularlyeducation,hasremainedconstant.Thismayormaynotbetrue;youwillbeaskedtoexplorethisissueinProblem13.7.Finally,theremaybeheteroskedasticityintheerrortermunderlyingtheestimatedequa-tion.ThiscanbedealtwithusingthemethodsinChapter8.Thereisoneinterestingdif-ferencehere:now,theerrorvariancemaychangeovertimeevenifitdoesnotchangewiththevaluesofeduc,age,black,andsoon.Theheteroskedasticity-robuststandarderrorsandteststatisticsareneverthelessvalid.TheBreusch-Pagantestwouldbeobtainedbyregress-ingthesquaredOLSresidualsonalloftheindependentvariablesinTable13.1,includingtheyeardummies.(ForthespecialcaseoftheWhitestatistic,thefittedvalueskiˆdsandthesquaredfittedvaluesareusedastheindependentvariables,asalways.)Aweightedleastsquaresprocedureshouldaccountforvariancesthatpossiblychangeovertime.Inthepro-cedurediscussedinSection8.4,yeardummieswouldbeincludedinequation(8.32).WecanalsointeractayeardummyvariablewithkeyexplanatoryvariablestoQUESTION13.1seeiftheeffectofthatvariablehasInreadingTable13.1,someoneclaimsthat,ifeverythingelseischangedoveracertaintimeperiod.Theequalinthetable,ablackwomanisexpectedtohaveonemorenextexampleexamineshowthereturntochildthananonblackwoman.Doyouagreewiththisclaim?educationandthegendergaphavechangedfrom1978to1985.410\nd7/14/997:25PMPage411Chapter13PoolingCrossSectionsAcrossTime.SimplePanelDataMethodsTable13.1DeterminantsofWomen’sFertilityDependentVariable:kidsIndependentVariablesCoefficientsStandardErrorseduc.128.018age.532.1382age.0058.0016black1.076.174east.217.133northcen.363.121west.198.167farm.053.147othrural.163.175town.084.124smcity.212.160y74.268.173y76.097.179y78.069.182y80.071.183y82.522.172y84.545.175constant7.7423.052n1,1292R.12952R¯.1162411\nd7/14/997:25PMPage412Part3AdvancedTopicsEXAMPLE13.2(ChangesintheReturntoEducationandtheGenderWageGap)Alog(wage)equation(wherewageishourlywage)pooledacrosstheyears1978(thebaseyear)and1985islog(wage)00y851educ1y85educ2exper2(13.1)3exper4union5female5y85femaleu,wheremostexplanatoryvariablesshouldbynowbefamiliar.Thevariableunionisadummyvariableequaltooneifthepersonbelongstoaunion,andzerootherwise.Thevariabley85isadummyvariableequaltooneiftheobservationcomesfrom1985andzeroifitcomesfrom1978.Thereare550peopleinthesamplein1978andadifferentsetof534peoplein1985.Theinterceptfor1978is0,andtheinterceptfor1985is00.Thereturntoedu-cationin1978is1,andthereturntoeducationin1985is11.Therefore,1measureshowthereturntoanotheryearofeducationhaschangedovertheseven-yearperiod.Finally,in1978,thelog(wage)differentialbetweenwomenandmenis5;thedifferentialin1985is55.Thus,wecantestthenullhypothesisthatnothinghashappenedtothegenderdifferentialoverthisseven-yearperiodbytestingH0:50.ThealternativethatthegenderdifferentialhasbeenreducedisH1:50.Forsimplicity,wehaveassumedthatexperienceandunionmembershiphavethesameeffectonwagesinbothtimeperiods.Beforewepresenttheestimates,thereisoneotherissueweneedtoaddress;namely,hourlywagehereisinnominal(orcurrent)dollars.Sincenominalwagesgrowsimplyduetoinflation,wearereallyinterestedintheeffectofeachexplanatoryvariableonrealwages.Supposethatwesettleonmeasuringwagesin1978dollars.Thisrequiresdeflating1985wagesto1978dollars.(Usingtheconsumerpriceindexforthe1997EconomicReportofthePresident,thedeflationfactoris107.6/65.21.65.)Whilewecaneasilydivideeach1985wageby1.65,itturnsoutthatthisisnotnecessary,provideda1985yeardummyisincludedintheregressionandlog(wage)(asopposedtowage)isusedasthedependentvariable.Usingrealornominalwageinalogarithmicfunctionalformonlyaffectsthecoef-ficientontheyeardummy,y85.Toseethis,letP85denotethedeflationfactorfor1985wages(1.65,ifweusetheCPI).Then,thelogoftherealwageforeachpersoniinthe1985sampleislog(wagei/P85)log(wagei)log(P85).Now,whilewageidiffersacrosspeople,P85doesnot.Therefore,log(P85)willbeabsorbedintotheinterceptfor1985.(Thisconclusionwouldchangeif,forexample,weusedadif-ferentpriceindexforpeopleinvariouspartsofthecountry.)Thebottomlineisthat,forstudyinghowthereturntoeducationorthegendergaphaschanged,wedonotneedtoturnnominalwagesintorealwagesinequation(13.1).Problem13.8asksyoutoverifythisforthecurrentexample.Ifweforgettoallowdifferentinterceptsin1978and1985,theuseofnominalwagescanproduceseriouslymisleadingresults.Ifweusewageratherthanlog(wage)asthedependentvariable,itisimportanttousetherealwageandtoincludeayeardummy.412\nd7/14/997:25PMPage413Chapter13PoolingCrossSectionsAcrossTime.SimplePanelDataMethodsThepreviousdiscussiongenerallyholdswhenusingdollarvaluesforeitherthedepen-dentorindependentvariables.Providedthedollaramountsappearinlogarithmicformanddummyvariablesareusedforalltimeperiods(except,ofcourse,thebaseperiod),theuseofaggregatepricedeflatorswillonlyaffecttheintercepts;noneoftheslopeestimateswillchange.Now,weusethedatainCPS78_85.RAWtoestimatetheequation:log(wˆage)(.459)(.118)y85(.0747)educ(.0185)y85educlog(wˆage)(.093)(.123)y85(.0067)educ(.0094)y85educ2(.0296)exper(.00040)exper(.202)union2(.0036)exper(.00008)exper(.030)union(13.2)(.317)female(.085)y85female(.037)female(.051)y85female22n1,084,R.426,R¯.422.Thereturntoeducationin1978isestimatedtobeabout7.5%;thereturntoeducationin1985isabout1.85percentagepointshigher,orabout9.35%.Becausethetstatisticontheinteractiontermis.0185/.00941.97,thedifferenceinthereturntoeducationissta-tisticallysignificantatthe5%levelagainstatwo-sidedalternative.Whataboutthegendergap?In1978,otherthingsbeingequal,awomanearnedabout31.7%lessthanaman(27.2%isthemoreaccurateestimate).In1985,thegapinlog(wage)is.317.085.232.Therefore,thegendergapappearstohavefallenfrom1978to1985byabout8.5percentagepoints.Thetstatisticontheinteractiontermisabout1.67,whichmeansitissignificantatthe5%levelagainstthepositiveone-sidedalternative.Whathappensifweinteractallindependentvariableswithy85inequation(13.2)?Thisisidenticaltoestimatingtwoseparateequations,onefor1978andonefor1985.Sometimesthisisdesirable.Forexample,inChapter7,wediscussedastudybyKrueger(1993),whereheestimatedthereturntousingacomputeronthejob.Kruegerestimatestwoseparateequations,oneusingthe1984CPSandtheotherusingthe1989CPS.Bycomparinghowthereturntoeducationchangesacrosstimeandwhetherornotcomputerusageiscontrolledfor,heestimatesthatone-thirdtoone-halfoftheobservedincreaseinthereturntoeducationoverthefive-yearperiodcanbeattributedtoin-creasedcomputerusage.[SeeTablesVIIIandIXinKrueger(1993).]TheChowTestforStructuralChangeAcrossTimeInChapter7,wediscussedhowtheChowtest—whichissimplyanFtest—canbeusedtodeterminewhetheramultipleregressionfunctiondiffersacrosstwogroups.Wecanapplythattesttotwodifferenttimeperiodsaswell.OneformofthetestobtainsthesumofsquaredresidualsfromthepooledestimationastherestrictedSSR.Theunre-strictedSSRisthesumoftheSSRsforthetwoseparatelyestimatedtimeperiods.The413\nd7/14/997:25PMPage414Part3AdvancedTopicsmechanicsofcomputingthestatisticareexactlyastheywereinSection7.4.Aheteroskedasticity-robustversionisalsoavailable(seeSection8.2).Example13.2suggestsanotherwaytocomputetheChowtestfortwotimeperiodsbyinteractingeachvariablewithayeardummyforoneofthetwoyearsandtestingforjointsignificanceoftheyeardummyandalloftheinteractionterms.Sincetheinter-ceptinaregressionmodeloftenchangesovertime(dueto,say,inflationinthehous-ingpriceexample),thisfull-blownChowtestcandetectsuchchanges.Itisusuallymoreinterestingtoallowforaninterceptdifferenceandthentotestwhethercertainslopecoefficientschangeovertime(aswedidinExample13.2).AChowtestcanbecomputedformorethantwotimeperiods,butthecalculationscanbetedious.Usually,afteranallowanceforinterceptdifference,certainslopecoef-ficientsaretestedforconstancybyinteractingthevariableofinterestwithyeardum-mies.(SeeProblems13.7and13.8forexamples.)13.2POLICYANALYSISWITHPOOLEDCROSSSECTIONSPooledcrosssectionscanbeveryusefulforevaluatingtheimpactofacertaineventorpolicy.Thefollowingexampleofaneventstudyshowshowtwocross-sectionaldatasets,collectedbeforeandaftertheoccurrenceofanevent,canbeusedtodeterminetheeffectoneconomicoutcomes.EXAMPLE13.3(EffectofaGarbageIncinerator’sLocationonHousingPrices)KielandMcClain(1995)studiedtheeffectthatanewgarbageincineratorhadonhousingvaluesinNorthAndover,Massachusetts.Theyusedmanyyearsofdataandafairlycompli-catedeconometricanalysis.Wewillusetwoyearsofdataandsomesimplifiedmodels,butouranalysisissimilar.TherumorsthatanewincineratorwouldbebuiltinNorthAndoverbeganafter1978,andconstructionbeganin1981.Theincineratorwasexpectedtobeinoperationsoonafterthestartofconstruction;theincineratoractuallybeganoperatingin1985.Wewillusedataonpricesofhousesthatsoldin1978andanothersampleonthosethatsoldin1981.Thehypothesisisthatthepriceofhouseslocatedneartheincineratorwouldfallbelowthepriceofmoredistanthouses.Forillustration,wedefineahousetobeneartheincineratorifitiswithinthreemiles.[Intheproblems,youareinsteadaskedtousetheactualdistancefromthehousetotheincinerator,asinKielandMcClain(1995).]Wewillstartbylookingatthedollareffectonhousingprices.Thisrequiresustomeasurepriceinconstantdollars.Wemeasureallhous-ingpricesin1978dollars,usingtheBostonhousingpriceindex.Letrpricedenotethehousepriceinrealterms.Anaiveanalystwoulduseonlythe1981dataandestimateaverysimplemodel:rprice01nearincu,(13.3)414\nd7/14/997:25PMPage415Chapter13PoolingCrossSectionsAcrossTime.SimplePanelDataMethodswherenearincisabinaryvariableequaltooneifthehouseisneartheincinerator,andzerootherwise.EstimatingthisequationusingthedatainKIELMC.RAWgivesrpriˆce(101,307.5)(30,688.27)nearincrpriˆce00(3,093.0)0(5,827.71)nearinc(13.4)2n142,R.165.Sincethisisasimpleregressiononasingledummyvariable,theinterceptistheaveragesellingpriceforhomesnotneartheincinerator,andthecoefficientonnearincisthedif-ferenceintheaveragesellingpricebetweenhomesneartheincineratorandthosethatarenot.Theestimateshowsthattheaveragesellingpricefortheformergroupwas$30,688.27lessthanforthelattergroup.Thetstatisticisgreaterthanfiveinabsolutevalue,sowecanstronglyrejectthehypothesisthattheaveragevalueforhomesneartoandfarfromtheincineratorarenotthesame.Unfortunately,equation(13.4)doesnotimplythatthesitingoftheincineratoriscaus-ingthelowerhousingvalues.Infact,ifwerunthesameregressionfor1978(beforetheincineratorwasevenrumored),weobtainrpriˆce(82,517.23)(18,824.37)nearincrpriˆce0(2,653.79)0(5,827.71)nearinc(13.5)2n179,R.082.Therefore,evenbeforetherewasanytalkofanincinerator,theaveragevalueofahomenearthesitewas$18,824.37lessthantheaveragevalueofahomenotnearthesite($82,517.23);thedifferenceisstatisticallysignificant,aswell.Thisisconsistentwiththeviewthattheincineratorwasbuiltinanareawithlowerhousingvalues.How,then,canwetellwhetherbuildinganewincineratordepresseshousingvalues?Thekeyistolookathowthecoefficientonnearincchangedbetween1978and1981.Thedifferenceinaveragehousingvaluewasmuchlargerin1981thanin1978($30,688.27ver-sus$18,824.37),evenasapercentageoftheaveragevalueofhomesnotneartheincin-eratorsite.Thedifferenceinthetwocoefficientsonnearincwasˆ130,688.27(18,824.37)11,863.9.Thisisourestimateoftheeffectoftheincineratoronvaluesofhomesneartheincineratorsite.Inempiricaleconomics,ˆ1hasbecomeknownasthedifference-in-differencesesti-matorbecauseitcanbeexpressedasˆ1(rprice81,nrrprice81,fr)(rprice78,nrrprice78,fr),(13.6)where“nr”standsfor“neartheincineratorsite”and“fr”standsfor“fartherawayfromthesite.”Inotherwords,ˆ1isthedifferenceovertimeintheaveragedifferenceofhous-ingpricesinthetwolocations.Totestwhetherˆ1isstatisticallydifferentfromzero,weneedtofinditsstandarderrorbyusingaregressionanalysis.Infact,ˆ1canbeobtainedbyestimatingrprice00y811nearinc1y81nearincu,(13.7)415\nd7/14/997:25PMPage416Part3AdvancedTopicsusingthedatapooledoverbothyears.Theintercept,0,istheaveragepriceofahomenotneartheincineratorin1978.Theparameter,0captureschangesinallhousingvaluesinNorthAndoverfrom1978to1981.[Acomparisonofequations(13.4)and(13.5)showedthathousingvaluesinNorthAndover,relativetotheBostonhousingpriceindex,increasedsharplyoverthisperiod.]Thecoefficientonnearinc,1,measuresthelocationeffectthatisnotduetothepresenceoftheincinerator:aswesawinequation(13.5),evenin1978,homesneartheincineratorsitesoldforlessthanhomesfartherawayfromthesite.Theparameterofinterestisontheinteractiontermy81nearinc:1measuresthedeclineinhousingvaluesduetothenewincinerator,providedweassumethathousesbothnearandfarfromthesitedidnotappreciateatdifferentratesforotherreasons.Theestimatesofequation(13.7)aregivenincolumn(1)ofTable13.2.Table13.2DependentVariable:rpriceIndependentVariable(1)(2)(3)constant82,517.2389,116.5413,807.67(2,726.91)(2,406.05)(11,166.59)y8118,790.2921,321.0413,928.48(4,050.07)(3,443.63)(2,798.75)nearinc18,824.379,397.943,780.34(4,875.32)(4,812.22)(4,453.42)y81nearinc11,863.9021,920.2714,177.93(7,456.65)(6,359.75)(4,987.27)2OtherControlsNoage,ageFullSetObservations.321.321.321R-Squared.174.414.660Theonlynumberwecouldnotobtainfromequations(13.4)and(13.5)isthestandarderrorofˆ1.Thetstatisticonˆ1isabout1.59,whichismarginallysignificantagainstaone-sidedalternative(p-value.057).KielandMcClain(1995)includedvarioushousingcharacteristicsintheiranalysisoftheincineratorsiting.Therearetwogoodreasonsfordoingthis.First,thekindsofhousessell-ingin1981mighthavebeensystematicallydifferentthanthosesellingin1978;ifso,itisimportanttocontrolforcharacteristicsthatmighthavebeendifferent.Butjustasimpor-tant,eveniftheaveragehousingcharacteristicsarethesameforbothyears,includingthemcangreatlyreducetheerrorvariance,whichcanthenshrinkthestandarderrorofˆ1.(SeeSection6.3fordiscussion.)Incolumn(2),wecontrolfortheageofthehouses,usingaqua-416\nd7/14/997:25PMPage417Chapter13PoolingCrossSectionsAcrossTime.SimplePanelDataMethodsdratic.ThissubstantiallyincreasestheR-squared(byreducingtheresidualvariance).Thecoefficientony81nearincisnowmuchlargerinmagnitude,anditsstandarderrorislower.Inadditiontotheagevariablesincolumn(2),column(3)controlsfordistancetotheinterstateinfeet(intst),landareainfeet(land),houseareainfeet(area),numberofrooms(rooms),andnumberofbaths(baths).Thisproducesanestimateony81nearincclosertothatwithoutanycontrols,butityieldsamuchsmallerstandarderror:thetstatisticforˆ1isabout2.84.Therefore,wefindamuchmoresignificanteffectincolumn(3)thanincol-umn(1).Thecolumn(3)estimatesarepreferredbecausetheycontrolforthemostfactorsandhavethesmalleststandarderrors(exceptintheconstant,whichisnotimportanthere).Thefactthatnearinchasamuchsmallercoefficientandisinsignificantincolumn(3)indi-catesthatthecharacteristicsincludedincolumn(3)largelycapturethehousingcharacter-isticsthataremostimportantfordetermininghousingprices.Forthepurposeofintroducingthemethod,weusedthelevelofrealhousingpricesinTable13.2.Itmakesmoresensetouselog(price)[orlog(rprice)]intheanalysisinordertogetanapproximatepercentageeffect.Thebasicmodelbecomeslog(price)00y811nearinc1y81nearincu.(13.8)Now,1001istheapproximatepercentagereductioninhousingvalueduetotheinciner-ator.[JustasinExample13.2,usinglog(price)versuslog(rprice)onlyaffectsthecoefficientony81.]Usingthesame321pooledobservationsgiveslog(priceˆ)(11.29)(.457)y81(.340)nearinc(.063)y81nearinclog(priceˆ)0(0.31)(.045)y81(.055)nearinc(.083)y81nearinc(13.9)2n321,R.409.Thecoefficientontheinteractiontermimpliesthat,becauseofthenewincinerator,housesneartheincineratorlostabout6.3%invalue.However,thisestimateisnotstatisticallydif-ferentfromzero.Butwhenweuseafullsetofcontrols,asincolumn(3)ofTable13.2(butwithintst,land,andareaappearinginlogarithmicform),thecoefficientony81nearincbecomes.132withatstatisticofabout2.53.Again,controllingforotherfactorsturnsouttobeimportant.Usingthelogarithmicform,weestimatethathousesneartheincin-eratorweredevaluedbyabout13.2%.Themethodologyappliedtothepreviousexamplehasnumerousapplications,espe-ciallywhenthedataarisefromanaturalexperiment(oraquasi-experiment).Anat-uralexperimentoccurswhensomeexogenousevent—oftenachangeingovernmentpolicy—changestheenvironmentinwhichindividuals,families,firms,orcitiesoper-ate.Anaturalexperimentalwayshasacontrolgroup,whichisnotaffectedbythepol-icychange,andatreatmentgroup,whichisthoughttobeaffectedbythepolicychange.Unlikewithatrueexperiment,wheretreatmentandcontrolgroupsarerandomlyandexplicitlychosen,thecontrolandtreatmentgroupsinnaturalexperimentsarisefromtheparticularpolicychange.Inordertocontrolforsystematicdifferencesbetweenthecontrolandtreatmentgroups,weneedtwoyearsofdata,onebeforethepolicychange417\nd7/14/997:25PMPage418Part3AdvancedTopicsandoneafterthechange.Thus,oursampleisusefullybrokendownintofourgroups:thecontrolgroupbeforethechange,thecontrolgroupafterthechange,thetreatmentgroupbeforethechange,andthetreatmentgroupafterthechange.CallAthecontrolgroupandBthetreatmentgroup,lettingdBequalunityforthoseinthetreatmentgroupB,andzerootherwise.Then,lettingd2denoteadummyvariableforthesecond(postpolicychange)timeperiod,theequationofinterestisy00d21dB1d2dBotherfactors,(13.10)whereyistheoutcomevariableofinterest.AsinExample13.3,1measurestheeffectofthepolicy.Withoutotherfactorsintheregression,ˆ1willbethedifference-in-differencesestimator:ˆ1(y¯2,By¯2,A)(y¯1,By¯1,A),(13.11)wherethebardenotesaverage,thefirstsubscriptdenotestheyear,andthesecondsub-scriptdenotesthegroup.Whenexplanatoryvariablesareaddedtoequation(13.10)(tocontrolforthefactthatthepopulationssampledmaydiffersystematicallyoverthetwoperiods),theOLSestimateof1nolongerhasthesimpleformof(13.11),butitsinter-pretationissimilar.EXAMPLE13.4(EffectofWorkerCompensationLawsonDuration)Meyer,Viscusi,andDurbin(1995)(hereafter,MVD)studiedthelengthoftime(inweeks)thataninjuredworkerreceivesworkers’compensation.OnJuly15,1980,Kentuckyraisedthecaponweeklyearningsthatwerecoveredbyworkers’compensation.Anincreaseinthecaphasnoeffectonthebenefitforlow-incomeworkers,butitmakesitlesscostlyforahigh-incomeworkertostayonworkers’compensation.Therefore,thecontrolgroupislow-incomeworkers,andthetreatmentgroupishigh-incomeworkers;high-incomework-ersaredefinedasthosewhoaresubjecttotheprepolicychangecap.Usingrandomsam-plesbothbeforeandafterthepolicychange,MVDwereabletotestwhethermoregenerousworkers’compensationcausespeopletostayoutofworklonger(everythingelsefixed).Theystartedwithadifference-in-differencesanalysis,usinglog(durat)asthedepen-dentvariable.Letafchngebethedummyvariableforobservationsafterthepolicychangeandhighearnthedummyvariableforhighearners.Theestimatedequation,withstandarderrorsinparentheses,islog(dˆurat)(1.126)(.0077)afchnge(.256)highearnlog(dˆurat)(0.031)(.0447)afchnge(.047)highearn(.191)afchngehighearn(13.12)(.069)afchngehighearn2n5,626,R.021.Therefore,ˆ1.191(t2.77),whichimpliesthattheaveragelengthoftimeonworkers’compensationincreasedbyabout19%duetothehigherearningscap.Thecoefficienton418\nd7/14/997:25PMPage419Chapter13PoolingCrossSectionsAcrossTime.SimplePanelDataMethodsafchngeissmallandstatisticallyinsignificant:asisexpected,theincreaseintheearningscaphasnoeffectondurationforlow-incomeworkers.Thisisagoodexampleofhowwecangetafairlypreciseestimateoftheeffectofapolicychange,eventhoughwecannotexplainmuchofthevariationinthedependentvari-able.Thedummyvariablesin(13.12)explainonly2.1%ofthevariationinlog(durat).Thismakessense:thereareclearlymanyfactors,includingseverityoftheinjury,thataffecthowlongsomeoneisonworkers’compensation.Fortunately,wehaveaverylargesamplesize,andthisallowsustogetasignificanttstatistic.MVDalsoaddedavarietyofcontrolsforgender,maritalstatus,age,industry,andtypeofinjury.Thisallowsforthefactthatthekindsofpeopleandtypesofinjuriesdiffersys-tematicallyinthetwoyears.Controllingforthesefactorsturnsouttohavelittleeffectontheestimateof1.(SeeProblem13.10.)Sometimes,thetwogroupsconsistofpeoplelivingintwoneighboringstatesintheUnitedStates.Forexample,toassesstheimpactofchangingcigarettetaxesoncigaretteconsumption,wecanobtainrandomsam-plesfromtwostatesfortwoyears.InStateQUESTION13.2A,thecontrolgroup,therewasnochangeWhatdoyoumakeofthecoefficientandtstatisticonhighearnininthecigarettetax.InStateB,thetaxequation(13.12)?increased(ordecreased)betweenthetwoyears.Theoutcomevariablewouldbeameasureofcigaretteconsumption,andequation(13.10)canbeestimatedtodeterminetheeffectofthetaxoncigaretteconsumption.Foraninterestingsurveyonnaturalexperimentmethodologyandseveraladditionalexamples,seeMeyer(1995).13.3TWO-PERIODPANELDATAANALYSISWenowturntotheanalysisofthesimplestkindofpaneldata:foracrosssectionofindividuals,schools,firms,cities,orwhatever,wehavetwoyearsofdata;calltheset1andt2.Theseyearsneednotbeadjacent,butt1correspondstotheearlieryear.Forexample,thefileCRIME2.RAWcontainsdataon(amongotherthings)crimeandunemploymentratesfor46citiesfor1982and1987.Therefore,t1correspondsto1982,andt2correspondsto1987.Whathappensifweusethe1987crosssectionandrunasimpleregressionofcrmrteonunem?Weobtaincrmˆrte(128.38)(4.16)unemcrmˆrte0(20.76)(3.42)unem2n46,R.033.Ifweinterprettheestimatedequationcausally,itimpliesthatanincreaseintheunem-ploymentratelowersthecrimerate.Thisiscertainlynotwhatweexpect.Thecoeffi-cientonunemisnotstatisticallysignificantatstandardsignificancelevels:atbest,wehavefoundnolinkbetweencrimeandunemploymentrates.419\nd7/14/997:25PMPage420Part3AdvancedTopicsAswehaveemphasizedthroughoutthistext,thissimpleregressionequationlikelysuffersfromomittedvariableproblems.Onepossibilesolutionistotrytocontrolformorefactors,suchasagedistribution,genderdistribution,educationlevels,lawenforcementefforts,andsoon,inamultipleregressionanalysis.Butmanyfactorsmightbehardtocontrolfor.InChapter9,weshowedhowincludingthecrmrtefromapreviousyear—inthiscase,1982—canhelptocontrolforthefactthatdifferentcitieshavehistoricallydifferentcrimerates.Thisisonewaytousetwoyearsofdataforesti-matingacausaleffect.Analternativewaytousepaneldataistoviewtheunobservedfactorsaffectingthedependentvariableasconsistingoftwotypes:thosethatareconstantandthosethatvaryovertime.Lettingidenotethecross-sectionalunitandtthetimeperiod,wecanwriteamodelwithasingleobservedexplanatoryvariableasyit00d2t1xitaiuit,t1,2.(13.13)Inthenotationyit,idenotestheperson,firm,city,andsoon,andtdenotesthetimeperiod.Thevariabled2tisadummyvariablethatequalszerowhent1andonewhent2;itdoesnotchangeacrossi,whichiswhyithasnoisubscript.Therefore,theinterceptfort1is0,andtheinterceptfort2is00.Justasinusinginde-pendentlypooledcrosssections,allowingtheintercepttochangeovertimeisimportantinmostapplications.Inthecrimeexample,seculartrendsintheUnitedStateswillcausecrimeratesinallU.S.citiestochange,perhapsmarkedly,overafive-yearperiod.Thevariableaicapturesallunobserved,time-constantfactorsthataffectyit.(Thefactthataihasnotsubscripttellsusthatitdoesnotchangeovertime.)Generically,aiiscalledanunobservedeffect.Itisalsocommoninappliedworktofindaireferredtoasafixedeffect,whichhelpsustorememberthataiisfixedovertime.Themodelin(13.13)iscalledanunobservedeffectsmodelorafixedeffectsmodel.Inapplications,youmightseeaireferredtoasunobservedheterogeneityaswell(orindividualhet-erogeneity,firmheterogeneity,cityheterogeneity,andsoon).Theerroruitisoftencalledtheidiosyncraticerrorortime-varyingerror,becauseitrepresentsunobservedfactorsthatchangeovertimeandaffectyit.Theseareverymuchliketheerrorsinastraighttimeseriesregressionequation.Asimpleunobservedeffectsmodelforcitycrimeratesfor1982and1987iscrmrteit00d87t1unemitaiuit,(13.14)whered87isadummyvariablefor1987.Sinceidenotesdifferentcities,wecallaianunobservedcityeffectoracityfixedeffect:itrepresentsallfactorsaffectingcitycrimeratesthatdonotchangeovertime.Geographicalfeatures,suchasthecity’slocationintheUnitedStates,areincludedinai.Manyotherfactorsmaynotbeexactlyconstant,buttheymightberoughlyconstantoverafive-yearperiod.Thesemightincludecertaindemographicfeaturesofthepopulation(age,race,andeducation).Differentcitiesmayhavetheirownmethodsforreportingcrimes,andthepeoplelivinginthecitiesmighthavedifferentattitudestowardcrime;thesearetypicallyslowtochange.Forhistoricalreasons,citiescanhaveverydifferentcrimerates,whichareatleastpartiallycapturedbytheunobservedeffectai.420\nd7/14/997:25PMPage421Chapter13PoolingCrossSectionsAcrossTime.SimplePanelDataMethodsHowshouldweestimatetheparameterofinterest,1,giventwoyearsofpaneldata?OnepossibilityistojustpoolthetwoyearsanduseOLS,essentiallyasinSection13.1.Thismethodhastwodrawbacks.Themostimportantoftheseisthat,inorderforpooledOLStoproduceaconsistentestimatorof1,wewouldhavetoassumethattheunob-servedeffect,ai,isuncorrelatedwithxit.Wecaneasilyseethisbywriting(13.13)asyit00d2t1xitvit,t1,2,(13.15)wherevitaiuitisoftencalledthecompositeerror.FromwhatweknowaboutOLS,wemustassumethatvitisuncorrelatedwithxit,wheret1or2,forOLStoconsistentlyestimate1(andtheotherparameters).Thisistruewhetherweuseasinglecrosssectionorpoolthetwocrosssections.Therefore,evenifweassumethattheidio-QUESTION13.3syncraticerroruitisuncorrelatedwithxit,Supposethatai,ui1,andui2havezeromeansandarepairwisepooledOLSisbiasedandinconsistentifaiuncorrelated.ShowthatCov(vi1,vi2)Var(ai),sothatthecompositeandxarecorrelated.Theresultingbiasinerrorsarepositivelyseriallycorrelatedacrosstime,unlessa0.itiWhatdoesthisimplyabouttheusualOLSstandarderrorsfrompooledOLSissometimescalledhetero-pooledOLSestimation?geneitybias,butitisreallyjustbiascausedfromomittingatime-constantvariable.Toillustratewhathappens,weusethedatainCRIME2.RAWtoestimate(13.14)bypooledOLS.Sincethereare46citiesandtwoyearsforeachcity,thereare92totalobservations:crmˆrte(93.42)(7.94)d87(0.427)unemcrmˆrte(12.74)(7.98)d87(1.188)unem(13.16)2n92,R.012.(Whenreportingtheestimatedequation,weusuallydroptheiandtsubscripts.)Thecoefficientonunem,thoughpositivein(13.16),hasaverysmalltstatistic.Thus,usingpooledOLSonthetwoyearshasnotsubstantiallychangedanythingfromusingasin-glecrosssection.ThisisnotsurprisingsinceusingpooledOLSdoesnotsolvetheomit-tedvariablesproblem.(Thestandarderrorsinthisequationareincorrectbecauseoftheserialcorrelationnotedearlier,butweignorethissincepooledOLSisnotthefocushere.)Inmostapplications,themainreasonforcollectingpaneldataistoallowfortheunobservedeffect,ai,tobecorrelatedwiththeexplanatoryvariables.Forexample,inthecrimeequation,wewanttoallowtheunmeasuredcityfactorsinaithataffectthecrimeratetoalsobecorrelatedwiththeunemploymentrate.Itturnsoutthatthisissim-pletoallow:becauseaiisconstantovertime,wecandifferencethedataacrossthetwoyears.Moreprecisely,foracross-sectionalobservationi,writethetwoyearsasyi2(00)1xi2aiui2(t2)yi101xi1aiui1(t1).Ifwesubtractthesecondequationfromthefirst,weobtain(yi2yi1)01(xi2xi1)(ui2ui1),421\nd7/14/997:25PMPage422Part3AdvancedTopicsoryi01xiui,(13.17)where“”denotesthechangefromt1tot2.Theunobservedeffect,ai,doesnotappearin(13.17):ithasbeen“differencedaway.”Also,theinterceptin(13.17)isactu-allythechangeintheinterceptfromt1tot2.Equation(13.17),whichwecallthefirst-differencedequation,isverysimple.Itisjustasinglecross-sectionalequation,buteachvariableisdifferencedovertime.Wecananalyze(13.17)usingthemethodswedevelopedinPart1,providedthekeyassumptionsaresatisfied.Themostimportantoftheseisthatuiisuncorrelatedwithxi.Thisassumptionholdsiftheidiosyncraticerrorateachtimet,uit,isuncorrelatedwiththeexplanatoryvariableinbothtimeperiods.ThisisanotherversionofthestrictexogeneityassumptionthatweencounteredinChapter10fortimeseriesmodels.Inparticular,thisassumptionrulesoutthecasewherexitisthelaggeddependentvariable,yi,t1.UnlikeinChapter10,weallowxittobecorrelatedwithunobservablesthatareconstantovertime.WhenweobtaintheOLSestimatorof1from(13.17)wecalltheresultingestimatorthefirst-differencedestimator.Inthecrimeexample,assumingthatuiandunemiareuncorrelatedmayberea-sonable,butitcanalsofail.Forexample,supposethatlawenforcementeffort(whichisintheidiosyncraticerror)increasesmoreincitieswheretheunemploymentratedecreases.Thiscancausenegativecorrelationbetweenuiandunemi,whichwouldthenleadtobiasintheOLSestimator.Naturally,thisproblemcanbeovercometosomeextentbyincludingmorefactorsintheequation,somethingwewillcoverlater.Asusual,itisalwayspossiblethatwehavenotaccountedforenoughtime-varyingfactors.Anothercrucialconditionisthatximusthavesomevariationacrossi.Thisquali-ficationfailsiftheexplanatoryvariabledoesnotchangeovertimeforanycross-sectionalobservation,orifitchangesbythesameamountforeveryobservation.Thisisnotanissueinthecrimerateexamplebecausetheunemploymentratechangesacrosstimeforalmostallcities.But,ifidenotesanindividualandxitisadummyvariableforgender,xi0foralli;weclearlycannotestimate(13.17)byOLSinthiscase.Thisactuallymakesperfectlygoodsense:sinceweallowaitobecorrelatedwithxit,wecan-nothopetoseparatetheeffectofaionyitfromtheeffectofanyvariablethatdoesnotchangeovertime.TheonlyotherassumptionweneedtoapplytotheusualOLSstatisticsisthat(13.17)satisfiesthehomoskedasticityassumption.Thisisreasonableinmanycases,and,ifitdoesnothold,weknowhowtotestandcorrectforheteroskedasticityusingthemethodsinChapter8.Itissometimesfairtoassumethat(13.17)fulfillsalloftheclassicallinearmodelassumptions.TheOLSestimatorsareunbiasedandallstatisticalinferenceisexactinsuchcases.Whenweestimate(13.17)forthecrimerateexample,wegetcrmˆrte(15.40)(2.22)unemcrmˆrte0(4.70)(0.88)unem(13.18)2n46,R.127,422\nd7/14/997:25PMPage423Chapter13PoolingCrossSectionsAcrossTime.SimplePanelDataMethodswhichnowgivesapositive,statisticallysignificantrelationshipbetweenthecrimeandunemploymentrates.Thus,differencingtoeliminatetime-constanteffectsmakesabigdifferenceinthisexample.Theinterceptin(13.18)alsorevealssomethinginteresting.Evenifunem0,wepredictanincreaseinthecrimerate(crimesper1,000people)of15.40.ThisreflectsasecularincreaseincrimeratesthroughouttheUnitedStatesfrom1982to1987.Evenifwedonotbeginwiththeunobservedeffectsmodel(13.13),usingdiffer-encesacrosstimemakesintuitivesense.Ratherthanestimatingastandardcross-sectionalrelationship—whichmaysufferfromomittedvariables,therebymakingce-terisparibusconclusionsdifficult—equation(13.17)explicitlyconsidershowchangesintheexplanatoryvariableovertimeaffectthechangeinyoverthesametimeperiod.Nevertheless,itisstillveryusefultohave(13.13)inmind:itexplicitlyshowsthatwecanestimatetheeffectofxitonyit,holdingaifixed.Whiledifferencingtwoyearsofpaneldataisapowerfulwaytocontrolforunob-servedeffects,itisnotwithoutcost.First,paneldatasetsarehardertocollectthanasinglecrosssection,especiallyforindividuals.Wemustuseasurveyandkeeptrackoftheindividualforafollow-upsurvey.Itisoftendifficulttolocatesomepeopleforasecondsurvey.Forunitssuchasfirms,somefirmswillgobankruptormergewithotherfirms.Paneldataaremucheasiertoobtainforschools,cities,counties,states,andcountries.Evenifwehavecollectedapaneldataset,thedifferencingusedtoeliminateaicangreatlyreducethevariationintheexplanatoryvariables.Whilexitfrequentlyhassub-stantialvariationinthecrosssectionforeacht,ximaynothavemuchvariation.WeknowfromChapter3thatlittlevariationinxicanleadtolargeOLSstandarderrors.Wecancombatthisbyusingalargecrosssection,butthisisnotalwayspossi-ble.Also,usinglongerdifferencesovertimeissometimesbetterthanusingyear-to-yearchanges.Asanexample,considertheproblemofestimatingthereturntoeducation,nowusingpaneldataonindividualsfortwoyears.Themodelforpersoniislog(wageit)00d2t1educitaiuit,t1,2,whereaicontainsunobservedability—whichisprobablycorrelatedwitheducit.Again,weallowdifferentinterceptsacrosstimetoaccountforaggregateproductivitygains(andinflation,ifwageitisinnominalterms).Since,bydefinition,innateabilitydoesnotchangeovertime,paneldatamethodsseemideallysuitedtoestimatethereturntoeducation.Theequationinfirstdifferencesislog(wagei)01educiui,(13.19)andwecanestimatethisbyOLS.Theproblemisthatweareinterestedinworkingadults,andformostemployedindividuals,educationdoesnotchangeovertime.Ifonlyasmallfractionofoursamplehaseducidifferentfromzero,itwillbedifficulttogetapreciseestimatorof1from(13.19),unlesswehavearatherlargesamplesize.Inthe-ory,usingafirstdifferencedequationtoestimatethereturntoeducationisagoodidea,butitdoesnotworkverywellwithmostcurrentlyavailablepaneldatasets.423\nd7/14/997:25PMPage424Part3AdvancedTopicsAddingseveralexplanatoryvariablescausesnodifficulties.Webeginwiththeunobservedeffectsmodelyit00d2t1xit12xit2…kxitkaiuit,(13.20)fort1and2.Thisequationlooksmorecomplicatedthanitisbecauseeachexplana-toryvariablehasthreesubscripts.Thefirstdenotesthecross-sectionalobservationnumber,theseconddenotesthetimeperiod,andthethirdisjustavariablelabel.EXAMPLE13.5(SleepingVersusWorking)WeusethetwoyearsofpaneldatainSLP75_81.RAW,fromBiddleandHamermesh(1990),toestimatethetradeoffbetweensleepingandworking.InProblem3.3,weusedjustthe1975crosssection.Thepaneldatasetfor1975and1981has239people,whichismuchsmallerthanthe1975crosssectionthatincludesover700people.Anunobservedeffectsmodelfortotalminutesofsleepingperweekisslpnapit00d81t1totwrkit2educit3marrit4yngkidit5gdhlthitaiuit,t1,2.Theunobservedeffect,ai,wouldbecalledanunobservedindividualeffectoranindividualfixedeffect.Itispotentiallyimportanttoallowaitobecorrelatedwithtotwrkit:thesamefactors(somebiological)thatcausepeopletosleepmoreorless(capturedinai)arelikelycorrelatedwiththeamountoftimespentworking.Somepeoplejusthavemoreenergy,andthiscausesthemtosleeplessandworkmore.Thevariableeducisyearsofeducation,marrisamarriagedummyvariable,yngkidisadummyvariableindicatingthepresenceofasmallchild,andgdhlthisa“goodhealth”dummyvariable.Noticethatwedonotincludegenderorrace(aswedidinthecross-sectionalanalysis),sincethesedonotchangeovertime;theyarepartofai.Ourprimaryinterestisin1.Differencingacrossthetwoyearsgivestheestimableequationslpnapi01totwrki2educi3marri4yngkidi5gdhlthiui.Assumingthatthechangeintheidiosyncraticerror,ui,isuncorrelatedwiththechangesinallexplanatoryvariables,wecangetconsistentestimatorsusingOLS.Thisgives(slpˆnap92.63)(.227)totwrk(00.024)educslpˆnap(45.87)(.036)totwrk(48.759)educ(104.21)marr(94.67)yngkid(87.58)gdhlth(13.21)0(92.86)marr(87.65)yngkid(76.60)gdhlth2n239,R.150.Thecoefficientontotwrkindicatesatradeoffbetweensleepingandworking:holdingotherfactorsfixed,onemorehourofworkisassociatedwith.227(60)13.62lessmin-utesofsleeping.Thetstatistic(6.31)isverysignificant.Nootherestimates,exceptthe424\nd7/14/997:25PMPage425Chapter13PoolingCrossSectionsAcrossTime.SimplePanelDataMethodsintercept,arestatisticallydifferentfromzero.TheFtestforjointsignificanceofallvariablesexcepttotwrkgivesp-value.49,whichmeanstheyarejointlyinsignificantatanyrea-sonablesignificancelevelandcouldbedroppedfromtheequation.Thestandarderroroneducisespeciallylargerelativetotheestimate.Thisisthephe-nomenondescribedearlierforthewageequation.Inthesampleof239people,183(76.6%)havenochangeineducationoverthesix-yearperiod;90%ofthepeoplehaveachangeineducationofatmostoneyear.Asreflectedbytheextremelylargestandarderrorofˆ2,thereisnotnearlyenoughvariationineducationtoestimate2withanyprecision.Anyway,ˆ2ispracticallyverysmall.Paneldatacanalsobeusedtoestimatefinitedistributedlagmodels.Evenifwespecifytheequationforonlytwoyears,weneedtocollectmoreyearsofdatatoobtainthelaggedexplanatoryvariables.Thefollowingisasimpleexample.EXAMPLE13.6(DistributedLagofCrimeRateonClear-upRate)Eide(1994)usespaneldatafrompolicedistrictsinNorwaytoestimateadistributedlagmodelforcrimerates.Thesingleexplanatoryvariableisthe“clear-uppercentage”(clrprc)—thepercentageofcrimesthatledtoaconviction.Thecrimeratedataarefromtheyears1972and1978.FollowingEide,welagclrprcforoneandtwoyears:itislikelythatpastclear-uprateshaveadeterrenteffectoncurrentcrime.Thisleadstothefollowingunob-servedeffectsmodelforthetwoyears:log(crimeit)00d78t1clrprci,t12clrprci,t2aiuit.WhenwedifferencetheequationandestimateitusingthedatainCRIME3.RAW,wegetlog(crimeˆ)(.086)(.0040)clrprc1(.0132)clrprc2log(crimeˆ)(.064)(.0047)clrprc1(.0052)clrprc2(13.22)22n53,R.193,R¯.161.Thesecondlagisnegativeandstatisticallysignificant,whichimpliesthatahigherclear-uppercentagetwoyearsagowoulddetercrimethisyear.Inparticular,a10percentagepointincreaseinclrprctwoyearsagowouldleadtoanestimated13.2%dropinthecrimeratethisyear.Thissuggeststhatusingmoreresourcesforsolvingcrimesandobtainingconvic-tionscanreducecrimeinthefuture.OrganizingPanelDataInusingpaneldatainaneconometricstudy,itisimportanttoknowhowthedatashouldbestored.Wemustbecarefultoarrangethedatasothatthedifferenttimeperiodsforthesamecross-sectionalunit(person,firm,city,andsoon)areeasilylinked.Forcon-creteness,supposethatthedatasetisoncitiesfortwodifferentyears.Formostpur-425\nd7/14/997:25PMPage426Part3AdvancedTopicsposes,thebestwaytoenterthedataistohavetworecordsforeachcity,oneforeachyear:thefirstrecordforeachcitycorrespondstotheearlyyear,andthesecondrecordisforthelateryear.Thesetworecordsshouldbeadjacent.Therefore,adatasetfor100citiesandtwoyearswillcontain200records.Thefirsttworecordsareforthefirstcityinthesample,thenexttworecordsareforthesecondcity,andsoon.(SeeTable1.5inChapter1foranexample.)Thismakesiteasytoconstructthedifferencestostoretheseinthesecondrecordforeachcity,andtodoapooledcross-sectionalanalysis,whichcanbecomparedwiththedifferencingestimation.Mostofthetwo-periodpaneldatasetsaccompanyingthistextarestoredinthisway(forexample,CRIME2.RAW,CRIME3.RAW,GPA3.RAW,LOWBRTH.RAW,andRENTAL.RAW).Weuseadirectextensionofthisschemeforpaneldatasetswithmorethantwotimeperiods.Asecondwayoforganizingtwoperiodsofpaneldataistohaveonlyonerecordpercross-sectionalunit.Thisrequirestwoentriesforeachvariable,oneforeachtimeperiod.ThepaneldatainSLP75_81.RAWareorganizedinthisway.Eachindividualhasdataonthevariablesslpnap75,slpnap81,totwrk75,totwrk81,andsoon.Creatingthedifferencesfrom1975to1981iseasy.OtherpaneldatasetswiththisstructureareTRAFFIC1.RAWandVOTE2.RAW.AdrawbacktoputtingthedatainonerecordisthatitdoesnotallowapooledOLSanalysisusingthetwotimeperiodsontheoriginaldata.Also,thisorganizationalmethoddoesnotworkforpaneldatasetswithmorethantwotimeperiods,acasewewillconsiderinSection13.5.13.4POLICYANALYSISWITHTWO-PERIODPANELDATAPaneldatasetsareveryusefulforpolicyanalysisand,inparticular,progamevaluation.Inthesimplestprogramevaluationsetup,asampleofindividuals,firms,orcities,andsoon,isobtainedinthefirsttimeperiod.Someoftheseunitsthentakepartinapar-ticularprograminalatertimeperiod;theonesthatdonotarethecontrolgroup.Thisissimilartothenaturalexperimentliteraturediscussedearlier,withoneimportantdif-ference:thesamecross-sectionalunitsappearineachtimeperiod.Asanexample,supposewewishtoevaluatetheeffectofaMichiganjobtrainingprogramonworkerproductivityofmanufacturingfirms(seealsoProblem9.8).Letscrapitdenotethescraprateoffirmiduringyeart(thenumberofitems,per100,thatmustbescrappedduetodefects).Letgrantitbeabinaryindicatorequaltooneiffirmiinyeartreceivedajobtraininggrant.Fortheyears1987and1988,themodelisscrapit00y88t1grantitaiuit,t1,2,(13.23)wherey88tisadummyvariablefor1988andaiistheunobservedfirmeffectorthefirmfixedeffect.Theunobservedeffectcontainsthingssuchasaverageemployeeability,capital,andmanagerialskill;theseareroughlyconstantoveratwo-yearperiod.Weareconcernedaboutaibeingsystematicallyrelatedtowhetherafirmreceivesagrant.Forexample,administratorsoftheprogrammightgiveprioritytofirmswhoseworkershavelowerskills.Or,theoppositeproblemcouldoccur:inordertomakethejobtrain-426\nd7/14/997:25PMPage427Chapter13PoolingCrossSectionsAcrossTime.SimplePanelDataMethodsingprogramappeareffective,administratorsmaygivethegrantstoemployerswithmoreproductiveworkers.Actually,inthisparticularprogram,grantswereawardedonafirst-come,first-servebasis.Butwhetherafirmappliedearlyforagrantcouldbecor-relatedwithworkerproductivity.Inanycase,ananalysisusingasinglecrosssectionorjustapoolingofthecrosssectionswillproducebiasedandinconsistentestimators.Differencingtoremoveaigivesscrapi01grantiui.(13.24)Therefore,wesimplyregressthechangeinthescraprateonthechangeinthegrantindicator.Becausenofirmsreceivedgrantsin1987,granti10foralli,andsograntigranti2granti1granti2,whichsimplyindicateswhetherthefirmreceivedagrantin1988.However,itisgenerallyimportanttodifferenceallvariables(dummyvariablesincluded)becausethisisnecessaryforremovingaiintheunobservedeffectsmodel(13.23).Estimatingthefirst-differencedequationusingthedatainJTRAIN.RAWgives(scrˆap.564)(.739)grantscrˆap(.405)(.683)grant2n54,R.022.Therefore,weestimatethathavingajobtraininggrantloweredthescraprateonaver-ageby.739.Buttheestimateisnotstatisticallydifferentfromzero.Wegetstrongerresultsbyusinglog(scrap)andestimatingthepercentageeffect:(log(scrˆap).057)(.317)grantlog(scrˆap)(.097)(.164)grant2n54,R.067.Havingajobtraininggrantisestimatedtolowerthescrapratebyabout27.2%[becauseexp(.317)1.272].Thetstatisticisabout1.93,whichismargin-allysignificant.Bycontrast,usingpooledOLSoflog(scrap)ony88andgrantgivesˆ1.057(standarderror.431).Thus,wefindnosignificantrelationshipbetweenthescraprateandthejobtraininggrant.Sincethisdifferssomuchfromthefirst-differenceestimates,itsuggeststhatfirmsthathavelowerabilityworkersaremorelikelytoreceiveagrant.Itisusefultostudytheprogramevaluationmodelmoregenerally.Letyitdenoteanoutcomevariableandletprogitbeaprogramparticipationdummyvariable.Thesim-plestunobservedeffectsmodelisyit00d2t1progitaiuit.(13.25)Ifprogramparticipationonlyoccurredinthesecondperiod,thentheOLSestimatorof1inthedifferencedequationhasaverysimplerepresentation:ˆ1ytreatycontrol.(13.26)427\nd7/14/997:25PMPage428Part3AdvancedTopicsThatis,wecomputetheaveragechangeinyoverthetwotimeperiodsforthetreatmentandcontrolgroups.Then,ˆ1isthedifferenceofthese.Thisisthepaneldataversionofthedifference-in-differencesestimatorinequation(13.11)fortwopooledcrosssec-tions.Withpaneldata,wehaveapotentiallyimportantadvantage:wecandifferenceyacrosstimeforthesamecross-sectionalunits.Thisallowsustocontrolforperson,firm,orcityspecificeffects,asthemodelin(13.25)makesclear.Ifprogramparticipationtakesplaceinbothperiods,ˆ1cannotbewrittenasin(13.26),butweinterpretitinthesameway:itisthechangeintheaveragevalueofyduetoprogramparticipation.Controllingfortime-varyingfactorsdoesnotchangeanythingofsignificance.Wesimplydifferencethosevariablesandincludethemalongwithprog.Thisallowsustocontrolfortime-varyingvariablesthatmightbecorrelatedwithprogramdesignation.Thesamedifferencingmethodworksforanalyzingtheeffectsofanypolicythatvariesacrosscityorstate.Thefollowingisasimpleexample.EXAMPLE13.7(EffectofDrunkDrivingLawsonTrafficFatalities)ManystatesintheUnitedStateshaveadopteddifferentpoliciesinanattempttocurbdrunkdriving.Twotypesoflawsthatwewillstudyhereareopencontainerlaws—whichmakeitillegalforpassengerstohaveopencontainersofalcoholicbeverages—andadmin-istrativeperselaws—whichallowcourtstosuspendlicensesafteradriverisarrestedfordrunkdrivingbutbeforethedriverisconvicted.Onepossibleanalysisistouseasinglecrosssectionofstatestoregressdrivingfatalities(orthoserelatedtodrunkdriving)ondummyvariableindicatorsforwhethereachlawispresent.Thisisunlikelytoworkwellbecausestatesdecide,throughlegislativeprocesses,whethertheyneedsuchlaws.Therefore,thepresenceoflawsislikelytoberelatedtotheaveragedrunkdrivingfatalitiesinrecentyears.Amoreconvincinganalysisusespaneldataoveratimeperiodwheresomestatesadoptednewlaws(andsomestatesmayhaverepealedexistinglaws).ThefileTRAFFIC1.RAWcon-tainsdatafor1985and1990forall50statesandtheDistrictofColumbia.Thedependentvariableisthenumberoftrafficdeathsper100millionmilesdriven(dthrte).In1985,19stateshadopencontainerlaws,while22stateshadsuchlawsin1990.In1985,21stateshadperselaws;thenumberhadgrownto29by1990.UsingOLSafterfirstdifferencinggives(dthˆrte.497)(.420)open(.151)admndthˆrte(.052)(.206)open(.117)admn(13.27)2n51,R.119.Theestimatessuggestthatadoptinganopencontainerlawloweredthetrafficfatalityrateby.42,anontrivialeffectgiventhattheaveragedeathratein1985was2.7withastan-darddeviationofabout.6.Theestimateisstatisticallysignificantatthe5%levelagainstatwo-sidedalternative.Theadministrativeperselawhasasmallereffect,anditststatisticisonly1.29;buttheestimateisthesignweexpect.Theinterceptinthisequationshowsthattrafficfatalitiesfellsubstantiallyforallstatesoverthefive-yearperiod,whetherornot428\nd7/14/997:25PMPage429Chapter13PoolingCrossSectionsAcrossTime.SimplePanelDataMethodstherewereanylawchanges.Thestatesthatadoptedanopencontainerlawoverthisperiodsawafurtherdrop,onaverage,infatalityrates.Otherlawsmightalsoaffecttrafficfatal-QUESTION13.4ities,suchasseatbeltlaws,motorcyclehel-metlaws,andmaximumspeedlimits.InInExample13.7,admn1forthestateofWashington.Explainaddition,wemightwanttocontrolforagewhatthismeans.andgenderdistributions,aswellasmea-suresofhowinfluentialanorganizationsuchasMothersAgainstDrunkDrivingisineachstate.13.5DIFFERENCINGWITHMORETHANTWOTIMEPERIODSWecanalsousedifferencingwithmorethantwotimeperiods.Forillustration,supposewehaveNindividualsandT3timeperiodsforeachindividual.Ageneralfixedeffectsmodelisyit12d2t3d3t1xit1…kxitkaiuit,(13.28)fort1,2,and3.(Thetotalnumberofobservationsistherefore3N.)Noticethatwenowincludetwotimeperioddummiesinadditiontotheintercept.Itisagoodideatoallowaseparateinterceptforeachtimeperiod,especiallywhenwehaveasmallnum-berofthem.Thebaseperiod,asalways,ist1.Theinterceptforthesecondtimeperiodis12,andsoon.Weareprimarilyinterestedin1,2,…,k.Iftheunob-servedeffectaiiscorrelatedwithanyoftheexplanatoryvariables,thenusingpooledOLSonthethreeyearsofdataresultsinbiasedandinconsistentestimates.Thekeyassumptionisthattheidiosyncraticerrorsareuncorrelatedwiththeexplanatoryvariableineachtimeperiod:Cov(xitj,uis)0,forallt,s,andj.(13.29)Thatis,theexplanatoryvariablesarestrictlyexogenousafterwetakeouttheunob-servedeffect,ai.(Thestrictexogeneityassumptionstatedintermsofazeroconditionalexpectationisgiveninthechapterappendix.)Assumption(13.29)rulesoutcaseswherefutureexplanatoryvariablesreacttocurrentchangesintheidiosyncraticerrors,asmustbethecaseifxitjisalaggeddependentvariable.Ifwehaveomittedanimportanttime-varyingvariable,then(13.29)isgenerallyviolated.Measurementerrorinoneormoreexplanatoryvariablescancause(13.29)tobefalse,justasinChapter9.InChapters15and16,wewilldiscusswhatcanbedoneinsuchcases.Ifaiiscorrelatedwithxitj,thenxitjwillbecorrelatedwiththecompositeerror,vitaiuit,under(13.29).Wecaneliminateaibydifferencingadjacentperiods.IntheT3case,wesubtracttimeperiodonefromtimeperiodtwoandtimeperiodtwofromtimeperiodthree.Thisgivesyit2d2t3d3t1xit1…kxitkuit,(13.30)429\nd7/14/997:25PMPage430Part3AdvancedTopicsfort2and3.Wedonothaveadifferencedequationfort1becausethereisnoth-ingtosubtractfromthet1equation.Now,(13.30)representstwotimeperiodsforeachindividualinthesample.Ifthisequationsatisfiestheclassicallinearmodelassumptions,thenpooledOLSgivesunbiasedestimators,andtheusualtandFstatis-ticsarevalidforhypothesis.Wecanalsoappealtoasymptoticresults.TheimportantrequirementforOLStobeconsistentisthatuitisuncorrelatedwithxitjforalljandt2and3.Thisisthenaturalextensionfromthetwotimeperiodcase.Noticehow(13.30)containsthedifferencesintheyeardummies,d2tandd3t.Fort2,d2t1andd3t0;fort3,d2t1andd3t1.Therefore,(13.30)doesnotcontainanintercept.Thisisinconvenientforcertainpurposes,includingthecomputationofR-squared.Unlessthetimeinterceptsintheoriginalmodel(13.28)areofdirectinterest—theyrarelyare—itisbettertoestimatethefirst-differencedequationwithaninterceptandasingletimeperioddummy,usuallyforthethirdperiod.Inotherwords,theequationbecomesyit03d3t1xit1…kxitkuit,fort2and3.Theestimatesofthejareidenticalineitherformulation.Withmorethanthreetimeperiods,thingsaresimilar.IfwehavethesameTtimeperiodsforeachofNcross-sectionalunits,wesaythatthedatasetisabalancedpanel:wehavethesametimeperiodsforallindividuals,firms,cities,andsoon.WhenTissmallrelativetoN,weshouldincludeadummyvariableforeachtimeperiodtoaccountforsecularchangesthatarenotbeingmodeled.Therefore,afterfirstdifferencing,theequationlookslikeyit03d3t4d4t…TdTt1xit1…(13.31)kxitkuit,t2,3,…,T,wherewehaveT1timeperiodsoneachunitiforthefirst-differencedequation.ThetotalnumberofobservationsisN(T1).Itissimpletoestimate(13.31)bypooledOLS,providedtheobservationshavebeenproperlyorganizedandthedifferencingcarefullydone.Tofacilitatefirstdifferencing,thedatafileshouldconsistofNTrecords.ThefirstTrecordsareforthefirstcross-sectionalobservation,arrangedchronologically;thesecondTrecordsareforthesec-ondcross-sectionalobservations,arrangedchronologically;andsoon.Then,wecom-putethedifferences,withthechangefromt1totstoredinthetimetrecord.Therefore,thedifferencesfort1shouldbemissingvaluesforallNcross-sectionalobservations.Withoutdoingthis,youruntheriskofusingbogusobservationsintheregressionanalysis.Aninvalidobservationiscreatedwhenthelastobservationfor,say,personi1issubstractedfromthefirstobservationforpersoni.Ifyoudotheregres-siononthedifferenceddata,andNTorNT1observationsarereported,thenyoufor-gottosetthet1observationsasmissing.Whenusingmorethantwotimeperiods,wemustassumethatuitisuncorrelatedovertimefortheusualstandarderrorsandteststatisticstobevalid.Thisassumptionissometimesreasonable,butitdoesnotfollowifweassumethattheoriginalidiosyncraticerrors,uit,areuncorrelatedovertime(anassumptionwewilluseinChapter14).Infact,ifweassumetheuitareseriallyuncorrelatedwithconstantvariance,thenthecorrela-430\nd7/14/997:25PMPage431Chapter13PoolingCrossSectionsAcrossTime.SimplePanelDataMethodstionbetweenuitandui,t1canbeshowntobe.5.IfuitfollowsastableAR(1)model,thenuitwillbeseriallycorrelated.Onlywhenuitfollowsarandomwalkwilluitbeseriallyuncorrelated.Itiseasytotestforserialcorrelationinthefirst-differencedequation.Letrituitdenotethefirstdifferenceoftheoriginalerror.IfritfollowstheAR(1)modelritri,t1eit,thenwecaneasilytestH0:0.First,weestimate(13.31)bypooledOLSandobtaintheresiduals,rˆit.Then,weruntheregressionagainwithrˆi,t1asanadditionalexplanatoryvariable.Thecoefficientonrˆi,t1isanestimateof,andsowecanusetheusualtstatisticonrˆi,t1totestH0:0.BecauseweareusingthelaggedOLSresidual,weloseanothertimeperiod.Forexample,ifweoriginallyhadT3,thedifferencedequationhasT2.Thetestforserialcorrelationisjustacross-sectionalregressiononfirstdifferences,usingthethirdtimeperiod,withthelaggedOLSresid-ualincluded.ThisissimilartothetestwecoveredinSection12.2forpuretimeseriesmodels.Wegiveanexamplelater.WecancorrectforthepresenceofAR(1)serialcorrelationbyquasi-differencingequation(13.31).[WecanalsousethePrais-Winstentransformationforthefirsttimeperiodin(13.31).]Unfortunately,standardpackagesthatperformAR(1)correctionsfortimeseriesregressionswillnotwork.StandardCochrane-OrcuttorPrais-WinstenmethodswilltreattheobservationsasiftheyfollowedanAR(1)processacrossiandt;thismakesnosense,asweareassumingtheobservationsareindependentacrossi.CorrectionstotheOLSstandarderrorsthatallowarbitraryformsofserialcorrelation(andheteroskedasticity)canbecomputedwhenNislarge(andNshouldbenotablylargerthanT).Adetailedtreatmentofthesetopicsisbeyondthescopeofthistext[seeWooldridge(1999,Chapter10)],buttheyareeasytocomputeincertainregressionQUESTION13.5packages.Doesserialcorrelationinuitcausethefirst-differencedestimatortoIfthereisnoserialcorrelationinthebebiasedandinconsistent?Whyisserialcorrelationaconcern?errors,theusualmethodsfordealingwithheteroskedasticityarevalid.WecanusetheBreusch-PaganandWhitetestsforheteroskedasticityfromChapter8,andwecanalsocomputerobuststandarderrors.Differencingmorethantwoyearsofpaneldataisveryusefulforpolicyanalysis,asshownbythefollowingexample.EXAMPLE13.8(EffectofEnterpriseZonesonUnemploymentClaims)Papke(1994)studiedtheeffectoftheIndianaenterprisezone(EZ)programonunemploy-mentclaims.Sheanalyzed22citiesinIndianaovertheperiodfrom1980to1988.Sixenter-prisezonesweredesignatedin1984,andfourmorewereassignedin1985.Twelveofthecitiesinthesampledidnotreceiveanenterprisezoneoverthisperiod;theyservedasthecontrolgroup.Asimplepolicyevaluationmodelislog(uclmsit)t1ezitaiuit,431\nd7/14/997:25PMPage432Part3AdvancedTopicswhereuclmsitisthenumberofunemploymentclaimsfiledduringyeartincityi.Thepara-metertjustdenotesadifferentinterceptforeachtimeperiod.Generally,unemploymentclaimswerefallingstatewideoverthisperiod,andthisshouldbereflectedinthedifferentyearintercepts.Thebinaryvariableezitisequaltooneifcityiattimetwasanenterprisezone;weareinterestedin1.Theunobservedeffectairepresentsfixedfactorsthataffecttheeconomicclimateincityi.Becauseenterprisezonedesignationwasnotdeterminedrandomly—enterprisezonesareusuallyeconomicallydepressedareas—itislikelythatezitandaiarepositivelycorrelated(highaimeanshigherunemploymentclaims,whichleadtoahigherchanceofbeinggivenanEZ).Thus,weshoulddifferencetheequationtoelimi-nateai:log(uclmsit)01d82t…7d88t1ezituit.(13.32)Thedependentvariableinthisequation,thechangeinlog(uclmsit),istheapproximateannualgrowthrateinunemploymentclaimsfromyeart1tot.Wecanestimatethisequationfortheyears1981to1988usingthedatainEZUNEM.RAW;thetotalsamplesizeis228176.Theestimateof1isˆ1.182(standarderror.078).Therefore,itappearsthatthepresenceofanEZcausesabouta16.6%[exp(.182)1.166]fallinunemploymentclaims.Thisisaneconomicallylargeandstatisticallysignificanteffect.Thereisnoevidenceofheteroskedasticityintheequation:theBreusch-PaganFtestyieldsF.85,p-value.557.However,whenweaddthelaggedOLSresidualstothedifferencedequation(andlosetheyear1981),wegetˆ.197(t2.44),sothereisevidenceofminimalnegativeserialcorrelationinthefirst-differencederrors.Unlikewithpositiveserialcorrelation,theusualOLSstandarderrorsmaynotgreatlyunderstatethecorrectstandarderrorswhentheerrorsarenegativelycorrelated(seeSection12.1).Thus,thesignificanceoftheenterprisezonedummyvariablewillprobablynotbeaffected.EXAMPLE13.9(CountyCrimeRatesinNorthCarolina)CornwellandTrumbull(1994)useddataon90countiesinNorthCarolina,fortheyears1981through1987,toestimateanunobservedeffectsmodelofcrime;thedataarecon-tainedinCRIME4.RAW.Here,weestimateasimplerversionoftheirmodel,andwediffer-encetheequationovertimetoeliminateai,theunobservedeffect.(CornwellandTrumbulluseadifferenttransformation,whichwewillcoverinChapter14.)Variousfactorsinclud-inggeographicallocation,attitudestowardcrime,historicalrecords,andreportingconven-tionsmightbecontainedinai.Thecrimerateisnumberofcrimesperperson,prbarristheestimatedprobabilityofarrest,prbconvistheestimatedprobabilityofconviction(givenanarrest),prbprisistheprobabilityofservingtimeinprison(givenaconviction),avgsenistheaveragesentencelengthserved,andpolpcisthenumberofpoliceofficerspercapita.Asisstandardincriminometricstudies,weusethelogsofallvariablesinordertoestimateelas-ticities.Wealsoincludeafullsetofyeardummiestocontrolforstatetrendsincrimerates.Wecanusetheyears1982through1987toestimatethedifferencedequation.Thequan-432\nd7/14/997:25PMPage433Chapter13PoolingCrossSectionsAcrossTime.SimplePanelDataMethodstitiesinparenthesesaretheusualOLSstandarderrors;thequantitiesinbracketsarestan-darderrorsrobusttobothserialcorrelationandheteroskedasticity:log(crmrteˆ)(.008)(.100)d83(.048)d84(.005)d85log(crmrteˆ)(.017)(.024)d83(.024)d84(.023)d85log(crmrteˆ)[.014][.022]d83[.020]d84[.025]d85(.028)d86(.041)d87(.327)log(prbarr)(.024)d86(.024)d87(.030)log(prbarr)[.021]d86[.024]d87[.056]log(prbarr)(.238)log(prbconv)(.165)log(prbpris)(13.33)(.018)log(prbconv)(.026)log(prbpris)[.039]log(prbconv)[.045]log(prbpris)(.022)log(avgsen)(.398)log(polpc)(.022)log(avgsen)(.027)log(polpc)[.025]log(avgsen)[.101]log(polpc)22n540,R.433,R¯.422.Thethreeprobabilityvariables—ofarrest,conviction,andservingprisontime—allhavetheexpectedsign,andallarestatisticallysignificant.Forexample,a1%increaseintheproba-bilityofarrestispredictedtolowerthecrimeratebyabout.33%.Theaveragesentencevariableshowsamodestdeterrenteffect,butitisnotstatisticallysignificant.Thecoefficientonthepolicepercapitavariableissomewhatsurprisingandisafeatureofmoststudiesthatseektoexplaincrimerates.Interpretedcausally,itsaysthata1%increaseinpolicepercapitaincreasescrimeratesbyabout.4%.(Theusualtstatisticisverylarge,almost15.)Itishardtobelievethathavingmorepoliceofficerscausesmorecrime.Whatisgoingonhere?Thereareatleasttwopossibilities.First,thecrimeratevariableiscalculatedfromreportedcrimes.Itmightbethat,whenthereareadditionalpolice,morecrimesarereported.Thepolicevariablemightbeendogenousintheequationforotherrea-sons:countiesmayenlargethepoliceforcewhentheyexpectcrimeratestoincrease.Inthiscase,(13.33)cannotbeinterpretedinacausalfashion.InChapters15and16,wewillcovermodelsandestimationmethodsthatcanaccountforthisadditionalformofendogeneity.ThespecialcaseoftheWhitetestforheteroskedasticityinSection8.3givesF75.48andp-value.0000,sothereisstrongevidenceofheteroskedasticity.(Technically,thistestisnotvalidifthereisalsoserialcorrelation,butitisstronglysuggestive.)TestingforAR(1)serialcorrelationyieldsˆ.233,t4.77,sonegativeserialcorrelationexists.Thestan-darderrorsinbracketsadjustforserialcorrelationandheteroskedasticity.[Wewillnotgivethedetailsofthis;thecalculationsaresimilartothosedescribedinSection12.5andarecarriedoutbymanyeconometricpackages.SeeWooldridge(1999,Chapter10)formorediscussion.]Novariableslosestatisticalsignificance,butthetstatisticsonthesignificantdeterrentvariablesgetnotablysmaller.Forexample,thetstatisticontheprobabilityofcon-victionvariablegoesfrom13.22usingtheusualOLSstandarderrorto6.10usingthefullyrobuststandarderror.Equivalently,theconfidenceintervalsconstructedusingtherobuststandarderrorswill,appropriately,bemuchwiderthanthosebasedontheusualOLSstandarderrors.433\nd7/14/997:25PMPage434Part3AdvancedTopicsSUMMARYWehavestudiedmethodsforanalyzingindependentlypooledcross-sectionalandpaneldatasets.Independentcrosssectionsarisewhendifferentrandomsamplesareobtainedindifferenttimeperiods(usuallyyears).OLSusingpooleddataistheleadingmethodofestimation,andtheusualinferenceproceduresareavailable,includingcorrectionsforheteroskedasticity.(Serialcorrelationisnotanissuebecausethesamplesareinde-pendentacrosstime.)Becauseofthetimeseriesdimension,weoftenallowdifferenttimeintercepts.Wemightalsointeracttimedummieswithcertainkeyvariablestoseehowtheyhavechangedovertime.Thisisespeciallyimportantinthepolicyevaluationliteraturefornaturalexperiments.Paneldatasetsarebeingusedmoreandmoreinappliedwork,especiallyforpolicyanalysis.Thesearedatasetswherethesamecross-sectionalunitsarefollowedovertime.Paneldatasetsaremostusefulwhencontrollingfortime-constantunobservedfeatures—ofpeople,firms,cities,andsoon—whichwethinkmightbecorrelatedwiththeexplanatoryvariablesinourmodel.Onewaytoremovetheunobservedeffectistodifferencethedatainadjacenttimeperiods.Then,astandardOLSanalysisonthedif-ferencescanbeused.Usingtwoperiodsofdataresultsinacross-sectionalregressionofthedifferenceddata.Theusualinferenceproceduresareasymptoticallyvalidunderhomoskedasticity;exactinferenceisavailableundernormality.Formorethantwotimeperiods,wecanusepooledOLSonthedifferenceddata;welosethefirsttimeperiodbecauseofthedifferencing.Inadditiontohomoskedasticity,wemustassumethatthedifferencederrorsareseriallyuncorrelatedinordertoapplytheusualtandFstatistics.(Thechapterappendixcontainsacarefullistingoftheassump-tions.)Naturally,anyvariablethatisconstantovertimedropsoutoftheanalysis.KEYTERMSBalancedPanelLongitudinalDataCompositeErrorNaturalExperimentDifference-in-DifferencesEstimatorPanelDataFirstDifferencedEquationQuasi-ExperimentFirst-DifferencedEstimatorStrictExogeneityFixedEffectUnobservedEffectFixedEffectsModelUnobservedEffectsModelHeterogeneityBiasUnobservedHeterogeneityIdiosyncraticErrorYearDummyVariablesIndependentlyPooledCrossSectionPROBLEMS13.1InExample13.1,assumethattheaverageofallfactorsotherthaneduchaveremainedconstantovertimeandthattheaveragelevelofeducationis12.2forthe1972sampleand13.3inthe1984sample.UsingtheestimatesinTable13.1,findtheesti-matedchangeinaveragefertilitybetween1972and1984.(Besuretoaccountfortheinterceptchangeandthechangeinaverageeducation.)434\nd7/14/997:25PMPage435Chapter13PoolingCrossSectionsAcrossTime.SimplePanelDataMethods13.2UsingthedatainKIELMC.RAW,thefollowingequationswereestimatedusingtheyears1978and1981:log(priceˆ)(11.49)(.547)nearinc(.394)y81nearinclog(priceˆ)0(0.26)(.058)nearinc(.080)y81nearinc2n321,R.220andlog(priceˆ)(11.18)(.563)y81(.403)y81nearinclog(priceˆ)0(0.27)(.044)y81(.067)y81nearinc2n321,R.337.Comparetheestimatesontheinteractiontermy81nearincwiththosefromequation(13.9).Whyaretheestimatessodifferent?13.3Whycanwenotusefirstdifferenceswhenwehaveindependentcrosssectionsintwoyears(asopposedtopaneldata)?13.4Ifwethinkthat1ispositivein(13.14)andthatuiandunemiarenegativelycorrelated,whatisthebiasintheOLSestimatorof1inthefirst-differencedequation?(Hint:ReviewTable3.2.)13.5SupposethatwewanttoestimatetheeffectofseveralvariablesonannualsavingandthatwehaveapaneldatasetonindividualscollectedonJanuary31,1990andJanuary31,1992.Ifweincludeayeardummyfor1992andusefirstdifferencing,canwealsoincludeageintheoriginalmodel?Explain.13.6In1985,neitherFloridanorGeorgiahadlawsbanningopenalcoholcontainersinvehiclepassengercompartments.By1990,Floridahadpassedsuchalaw,butGeorgiahadnot.(i)Supposeyoucancollectrandomsamplesofthedriving-agepopulationinbothstates,for1985and1990.Letarrestbeabinaryvariableequaltounityifapersonwasarrestedfordrunkdrivingduringtheyear.Withoutcontrollingforanyotherfactors,writedownalinearprobabil-itymodelthatallowsyoutotestwhethertheopencontainerlawreducedtheprobabilityofbeingarrestedfordrunkdriving.Whichcoef-ficientinyourmodelmeasurestheeffectofthelaw?(ii)Whymightyouwanttocontrolforotherfactorsinthemodel?Whatmightsomeofthesefactorsbe?COMPUTEREXERCISES13.7UsethedatainFERTIL1.RAWforthisexercise.(i)IntheequationestimatedinExample13.1,testwhetherlivingenviron-mentatage16hasaneffectonfertility.(Thebasegroupislargecity.)ReportthevalueoftheFstatisticandthep-value.(ii)Testwhetherregionofthecountryatage16(southisthebasegroup)hasaneffectonfertility.435\nd7/14/997:25PMPage436Part3AdvancedTopics(iii)Letubetheerrorterminthepopulationequation.Supposeyouthinkthatthevarianceofuchangesovertime(butnotwitheduc,age,andsoon).Amodelthatcapturesthisis2u01y742y76…6y84v.Usingthismodel,testforheteroskedasticityinu.[Hint:YourFtestshouldhave6and1122degreesoffreedom.](iv)Addtheinteractiontermsy74educ,y76educ,…,y84eductothemodelestimatedinTable13.1.Explainwhatthesetermsrepresent.Aretheyjointlysignificant?13.8UsethedatainCPS78_85.RAWforthisexercise.(i)Howdoyouinterpretthecoefficientony85inequation(13.2)?Doesithaveaninterestinginterpretation?(Becarefulhere;youmustaccountfortheinteractiontermsy85educandy85female.)(ii)Holdingotherfactorsfixed,whatistheestimatedpercentincreaseinnominalwageforamalewithtwelveyearsofeducation?Proposearegressiontoobtainaconfidenceintervalforthisestimate.[Hint:Togettheconfidenceinterval,replacey85educwithy85(educ12);refertoExample6.3.](iii)Reestimateequation(13.2)butletallwagesbemeasuredin1978dol-lars.Inparticular,definetherealwageasrwagewagefor1978andasrwagewage/1.65for1985.Nowuselog(rwage)inplaceoflog(wage)inestimating(13.2).Whichcoefficientsdifferfromthoseinequation(13.2)?(iv)ExplainwhytheR-squaredfromyourregressioninpart(iii)isnotthesameasinequation(13.2).(Hint:Theresiduals,andthereforethesumofsquaredresiduals,fromthetworegressionsareidentical.)(v)Describehowunionparticipationhaschangedfrom1978to1985.(vi)Startingwithequation(13.2),testwhethertheunionwagedifferentialhaschangedovertime.(Thisshouldbeasimplettest.)(vii)Doyourfindingsinparts(v)and(vi)conflict?Explain.13.9UsethedatainKIELMC.RAWforthisexercise.(i)Thevariabledististhedistancefromeachhometotheincineratorsite,infeet.Considerthemodellog(price)00y811log(dist)1y81log(dist)u.Ifbuildingtheincineratorreducesthevalueofhomesclosertothesite,whatisthesignof1?Whatdoesitmeanif10?(ii)Estimatethemodelfrompart(i)andreporttheresultsintheusualform.Interpretthecoefficientony81log(dist).Whatdoyouconclude?2(iii)Addage,age,rooms,baths,log(intst),log(land),andlog(area)totheequation.Nowwhatdoyouconcludeabouttheeffectoftheincineratoronhousingvalues?13.10UsethedatainINJURY.RAWforthisexercise.436\nd7/14/997:25PMPage437Chapter13PoolingCrossSectionsAcrossTime.SimplePanelDataMethods(i)UsingthedataforKentucky,reestimateequation(13.12)addingasex-planatoryvariablesmale,married,andafullsetofindustryandinjurytypedummyvariables.Howdoestheestimateonafchngehighearnchangewhentheseotherfactorsarecontrolledfor?Istheestimatestillstatisticallysignificant?(ii)WhatdoyoumakeofthesmallR-squaredfrompart(i)?Doesthismeantheequationisuseless?(iii)Estimateequation(13.12)usingthedataforMichigan.ComparetheestimatesontheinteractiontermforMichiganandKentucky.IstheMichiganestimatestatisticallysignificant?Whatdoyoumakeofthis?13.11UsethedatainRENTAL.RAWforthisexercise.Thedatafortheyears1980and1990includerentalpricesandothervariablesforcollegetowns.Theideaistoseewhetherastrongerpresenceofstudentsaffectsrentalrates.Theunobservedeffectsmodelislog(rentit)00y90t1log(popit)2log(avgincit)3pctstuitaiuit,wherepopiscitypopulation,avgincisaverageincome,andpctstuisstudentpopula-tionasapercentageofcitypopulation(duringtheschoolyear).(i)EstimatetheequationbypooledOLSandreporttheresultsinstandardform.Whatdoyoumakeoftheestimateonthe1990dummyvariable?Whatdoyougetforˆpctstu?(ii)Arethestandarderrorsyoureportinpart(i)valid?Explain.(iii)NowdifferencetheequationandestimatebyOLS.Compareyouresti-mateofpctstuwiththatfrompart(ii).Doestherelativesizeofthestu-dentpopulationappeartoaffectrentalprices?(iv)Obtaintheheteroskedasticity-robuststandarderrorsforthefirst-differencedequationinpart(iii).Doesthischangeyourconclusions?13.12UseCRIME3.RAWforthisexercise.(i)InthemodelofExample13.6,testthehypothesisH0:12.(Hint:Define112andwrite1intermsof1and2.Substitutethisintotheequationandthenrearrange.Doatteston1.)(ii)If12,showthatthedifferencedequationcanbewrittenaslog(crimei)01avgclriui,where121andavgclri(clrprci,1clrprci,2)/2istheaverageclear-uppercentageovertheprevioustwoyears.(iii)Estimatetheequationfrompart(ii).ComparetheadjustedR-squaredwiththatin(13.22).Whichmodelwouldyoufinallyuse?13.13UseGPA3.RAWforthisexercise.Thedatasetisfor366studentathletesfromalargeuniversityforfallandspringsemesters.(AsimilaranalysisisinMaloneyandMcCormick(1993),buthereweuseatruepaneldataset).Becauseyouhavetwotermsofdataforeachstudent,anunobservedeffectsmodelisappropriate.Theprimaryques-tionofinterestisthis:Doathletesperformmorepoorlyinschoolduringthesemestertheirsportisinseason?437\nd7/14/997:25PMPage438Part3AdvancedTopics(i)UsepooledOLStoestimateamodelwithtermGPA(trmgpa)asthedependentvariable.Theexplanatoryvariablesarespring,sat,hsperc,female,black,white,frstsem,tothrs,crsgpa,andseason.Interpretthecoefficientonseason.Isitstatisticallysignificant?(ii)Mostoftheathleteswhoplaytheirsportonlyinthefallarefootballplayers.Supposetheabilitylevelsoffootballplayersdiffersystemati-callyfromthoseofotherathletes.IfabilityisnotadequatelycapturedbySATscoreandhighschoolpercentile,explainwhythepooledOLSestimatorswillbebiased.(iii)Nowusethedatadifferencedacrossthetwoterms.Whichvariablesdropout?Nowtestforanin-seasoneffect.(iv)Canyouthinkofoneormorepotentiallyimportant,time-varyingvari-ablesthathavebeenomittedfromtheanalysis?13.14VOTE2.RAWincludespaneldataonHouseofRepresentativeelectionsin1988and1990.Onlywinnersfrom1988whoarealsorunningin1990appearinthesample;thesearetheincumbents.Anunobservedeffectsmodelexplainingtheshareoftheincumbent’svoteintermsofexpendituresbybothcandidatesisvoteit00d90t1log(inexpit)2log(chexpit)3incshritaiuit,whereincshritistheincumbent’sshareoftotalcampaignspending(inpercentform).Theunobservedeffectaicontainscharacteristicsoftheincumbent—suchas“qual-ity”—aswellasthingsaboutthedistrictthatareconstant.Theincumbent’sgenderandpartyareconstantovertime,sothesearesubsumedinai.Weareinterestedintheeffectofcampaignexpendituresonelectionoutcomes.(i)Differencethegivenequationacrossthetwoyearsandestimatethedif-ferencedequationbyOLS.Whichvariablesareindividuallysignificantatthe5%levelagainstatwo-sidedalternative?(ii)Intheequationfrompart(i),testforjointsignificanceoflog(inexp)andlog(chexp).Reportthep-value.(iii)Reestimatetheequationfrompart(i)usingincshrastheonlyinde-pendentvariable.Interpretthecoefficientonincshr.Forexample,iftheincumenbent’sshareofspendingincreasesby10percentagepoints,howisthispredictedtoaffecttheincumbent’sshareofthevote?(iv)Redopart(iii),butnowuseonlythepairsthathaverepeatchallengers.[Thisallowsustocontrolforcharacteristicsofthechallengersaswell,whichwouldbeinai.Levitt(1995)conductsamuchmoreextensiveanalysis.]13.15UseCRIME4.RAWforthisexercise.(i)Addthelogsofeachwagevariableinthedatasetandestimatethemodelbyfirstdifferencing.HowdoesincludingthesevariablesaffectthecoefficientsonthecriminaljusticevariablesinExample13.9?(ii)Dothewagevariablesin(ii)allhavetheexpectedsign?Aretheyjointlysignificant?Explain.13.16Forthisexercise,weuseJTRAIN.RAWtodeterminetheeffectofthejobtrain-inggrantonhoursofjobtrainingperemployee.Thebasicmodelforthethreeyearsis438\nd7/14/997:25PMPage439Chapter13PoolingCrossSectionsAcrossTime.SimplePanelDataMethodshrsempit01d88t2d89t1grantit2granti,t13log(employit)aiuit.(i)Estimatetheequationusingfirstdifferencing.Howmanyfirmsareusedintheestimation?Howmanytotalobservationswouldbeusedifeachfirmhaddataonallvariables(inparticular,hrsemp)forallthreetimeperiods?(ii)Interpretthecoefficientongrantandcommentonitssignificance.(iii)Isitsurprisingthatgrant1isinsignificant?Explain.(iv)Dolargerfirmstraintheiremployeesmoreorless,onaverage?Howbigarethedifferencesintraining?APPENDIX13AAssumptionsforPooledOLSUsingFirstDifferencesInthisappendix,weprovidecarefulstatementsoftheassumptionsforthefirst-differencingestimator.Verificationoftheseclaimsissomewhatinvolved,butitcanbefoundinWooldridge(1999,Chapter10).ASSUMPTIONFD.1Foreachi,themodelisyit1xit1…kxitkaiuit,t1,…,T,wherethejaretheparameterstoestimateandaiistheunobservedeffect.ASSUMPTIONFD.2Wehavearandomsamplefromthecrosssection.Forthenextassumption,itisusefultoletXidenotetheexplanatoryvariablesforalltimeperiodsforcross-sectionalobservationi;thus,Xicontainsxitj,t1,…,T,j1,…,k.ASSUMPTIONFD.3Foreacht,theexpectedvalueoftheidiosyncraticerrorgiventheexplanatoryvariablesinalltimeperiodsandtheunobservedeffectiszero:E(uitXi,ai)0.WhenAssumptionFD.3holds,wesometimessaythatthexitjarestrictlyexogenousconditionalontheunobservedeffect.Theideaisthat,oncewecontrolforai,thereisnocorrelationbetweenthexisjandtheremainingerror,uit,forallsandt.AnimportantimplicationofFD.3isthatE(uitXi)0,t2,...,T.439\nd7/14/997:25PMPage440Part3AdvancedTopicsASSUMPTIONFD.4Eachexplanatoryvariablechangesovertime(foratleastsomei),andnoperfectlinearrela-tionshipsexistamongtheexplanatoryvariables.Underthesefirstfourassumptions,thefirst-differenceestimatorsareunbiased.ThekeyassumptionisFD.3,whichisstrictexogeneityoftheexplanatoryvariables.Underthesesameassumptions,wecanalsoshowthattheFDestimatorisconsistentwithafixedTandasN*(andperhapsmoregenerally).ASSUMPTIONFD.5Thevarianceofthedifferencederrors,conditionalonallexplanatoryvariables,isconstant:2Var(uitXi),t2,…,T.ASSUMPTIONFD.6Forallts,thedifferencesintheidiosyncraticerrorsareuncorrelated(conditionalonallexplanatoryvariables):Cov(uit,uisXi)0,ts.AssumptionFD.5ensuresthatthedifferencederrors,uit,arehomoskedastic.AssumptionFD.6statesthatthedifferencederrorsareseriallyuncorrelated,whichmeansthattheuitfollowarandomwalkacrosstime(seeChapter11).UnderAssumptionsFD.1throughFD.6,theFDestimatorofthejisthebestlinearunbiasedestimator(conditionalontheexplanatoryvariables).ASSUMPTIONFD.7ConditionalonXi,theuitareindependentandidenticallydistributednormalrandomvari-ables.WhenweaddAssumptionFD.7,theFDestimatorsarenormallydistributedandthetandFstatisticsfrompooledOLSonthedifferenceshaveexacttandFdistributions.WithoutFD.7,wecanrelyontheusualasymptoticapproximations.440\n\nChapterFourteenAdvancedPanelDataMethodsnthischapter,wecovertwomethodsforestimatingunobservedeffectspaneldataImodelsthatareatleastascommonasfirstdifferencing.Althoughthesemethodsaresomewhathardertodescribeandimplement,severaleconometricspackagessup-portthem.InSection14.1,wediscussthefixedeffectsestimator,which,likefirstdifferencing,usesatransformationtoremovetheunobservedeffectaipriortoestimation.Anytime-constantexplanatoryvariablesareremovedalongwithai.Therandomeffectsestimatorisattractivewhenwethinktheunobservedeffectisuncorrelatedwithalltheexplanatoryvariables.Ifwehavegoodcontrolsinourequa-tion,wemightbelievethatanyleftoverneglectedheterogeneityonlyinducesserialcor-relationinthecompositeerrorterm,butitdoesnotcausecorrelationbetweenthecompositeerrorsandtheexplanatoryvariables.Estimationofrandomeffectsmodelsbygeneralizedleastsquaresisfairlyeasyandisroutinelydonebymanyeconometricspackages.InSection14.3,weshowhowpaneldatamethodscanbeappliedtootherdatastruc-tures,includingmatchedpairsandclustersamples.14.1FIXEDEFFECTSESTIMATIONFirstdifferencingisjustoneofthemanywaystoeliminatethefixedeffect,ai.Analter-nativemethod,whichworksbetterundercertainassumptions,iscalledthefixedeffectstransformation.Toseewhatthismethodinvolves,consideramodelwithasingleexplanatoryvariable:foreachi,yit1xitaiuit,t1,2,…,T.(14.1)Now,foreachi,averagethisequationovertime.Wegety¯i1x¯iaiu¯i,(14.2)T1wherey¯iTyit,andsoon.Becauseaiisfixedovertime,itappearsinboth(14.1)t1and(14.2).Ifwesubstract(14.2)from(14.1)foreacht,wewindupwith441\nPart3AdvancedTopicsyity¯i1(xitx¯i)uitu¯i,t1,2,…,T,oryit1xituit,t1,2,…,T,(14.3)whereyityity¯iisthetime-demeaneddataony,andsimilarlyforxitanduit.Thefixedeffectstransformationisalsocalledthewithintransformation.Theimportantthingaboutequation(14.3)isthattheunobservedeffect,ai,hasdisappeared.Thissug-geststhatweestimate(14.3)bypooledOLS.ApooledOLSestimatorthatisbasedonthetime-demeanedvariablesiscalledthefixedeffectsestimatororthewithinesti-mator.ThelatternamecomesfromthefactthatOLSon(14.3)usesthetimevariationinyandxwithineachcross-sectionalobservation.ThebetweenestimatorisobtainedastheOLSestimatoronthecross-sectionalequation(14.2)(whereweincludeanintercept,0):weusethetime-averagesforbothyandxandthenrunacross-sectionalregression.Wewillnotstudythebetweenesti-matorindetailbecauseitisbiasedwhenaiiscorrelatedwithxi(seeProblem14.2).Ifwethinkaiisuncorrelatedwithxit,itisbettertousetherandomeffectsestimator,whichwecoverinSection14.2.Thebetweenestimatorignoresimportantinformationonhowthevariableschangeovertime.Addingmoreexplanatoryvariablestotheequationcausesfewchanges.Theorigi-nalmodelisyit1xit12xit2…kxitkaiuit,t1,2,…,T.(14.4)Wesimplyusethetime-demeaningoneachexplanatoryvariable—includingthingsliketimeperioddummies—andthendoapooledOLSregressionusingalltime-demeanedvariables.Thegeneraltime-demeanedequationforeachiisyit1xit12xit2…kxitkuit,t1,2,…,T,(14.5)whichweestimatebypooledOLS.Underastrictexogeneityassumptionontheexplanatoryvariables,thefixedeffectsestimatorisunbiased:roughly,theidiosyncraticerroruitshouldbeuncorrelatedwitheachexplanatoryvariableacrossalltimeperiods.(Seethechapterappendixforprecisestatementsoftheassumptions.)Thefixedeffectsestimatorallowsforarbitrarycorrela-tionbetweenaiandtheexplanatoryvariablesinanytimeperiod,justaswithfirstdif-ferencing.Becauseofthis,anyexplanatoryvariablethatisconstantovertimeforalligetssweptawaybythefixedeffectstransformation:xit0foralliandt,ifxitiscon-stantacrosst.Therefore,wecannotincludevariablessuchasgenderorwhetheracityislocatednearariver.QUESTION14.1TheotherassumptionsneededforaSupposethatinafamilysavingsequation,fortheyears1990,1991,straightOLSanalysistobevalidarethatand1992,weletkidsitdenotethenumberofchildreninfamilyiforyeart.Ifthenumberofkidsisconstantoverthisthree-yearperiodtheerrorsuitarehomoskedasticandseri-formostfamiliesinthesample,whatproblemsmightthiscauseforallyuncorrelated(acrosst);seetheappen-estimatingtheeffectthatthenumberofkidshasonsavings?dixtothischapter.442\nChapter14AdvancedPanelDataMethodsThereisonesubtlepointindeterminingthedegreesoffreedomforthefixedeffectsestimator.Whenweestimatethetime-demeanedequation(14.5)bypooledOLS,wehaveNTtotalobservationsandkindependentvariables.[Noticethatthereisnointerceptin(14.5);itiseliminatedbythefixedeffectstransformation.]Therefore,weshouldappar-entlyhaveNTkdegreesoffreedom.Thiscalculationisincorrect.Foreachcross-sectionalobservationi,weloseonedfbecauseofthetime-demeaning.Inotherwords,foreachi,thedemeanederrorsuitadduptozerowhensummedacrosst,soweloseonedegreeoffreedom.(Thereisnosuchconstraintontheoriginalidiosyncraticerrorsuit.)Therefore,theappropriatedegreesoffreedomisdfNTNkN(T1)k.Fortunately,modernregressionpackagesthathaveafixedeffectsestimationfeatureprop-erlycomputethedf.Butifwehavetodothetime-demeaningandtheestimationbypooledOLSourselves,weneedtocorrectthestandarderrorsandteststatistics.EXAMPLE14.1(EffectofJobTrainingonFirmScrapRates)Weusethedataforthethreeyears,1987,1988,and1989onthe54firmsthatreportedscrapratesineachyear.Nofirmsreceivedgrantspriorto1988;in1988,19firmsreceivedgrants;in1989,10differentfirmsreceivedgrants.Therefore,wemustalsoallowforthepossibilitythattheadditionaljobtrainingin1988madeworkersmoreproductivein1989.Thisiseasilydonebyincludingalaggedvalueofthegrantindicator.Wealsoincludeyeardummiesfor1988and1989.TheresultsaregiveninTable14.1:Table14.1FixedEffectsEstimationoftheScrapRateEquationDependentVariable:log(scrap)IndependentVariablesd88.080(.109)d89.247(.133)grant.252(.151)grant1.422(.210)Observations.162DegreesofFreedom.104R-Squared.201443\nPart3AdvancedTopicsWehavereportedtheresultsinawaythatemphasizestheneedtointerprettheesti-matesinlightoftheunobservedeffectsmodel,(14.4).Weareexplicitlycontrollingfortheunobserved,time-constanteffectsinai.Thetime-demeaningallowsustoestimatethej,but(14.5)isnotthebestequationforinterpretingtheestimates.Interestingly,theestimatedlaggedeffectofthetraininggrantissubstantiallylargerthanthecontemporaneouseffect:jobtraininghasaneffectatleastoneyearlater.Becausethedependentvariableisinlogarithmicform,obtainingagrantin1988ispredictedtolowerthefirmscrapratein1989byabout34.4%[exp(.422)1.344];thecoeffi-cientongrant1issignificantatthe5%levelagainstatwo-sidedalternative.Thecoeffi-cientongrantissignificantatthe10%level,andthesizeofthecoefficientishardlytrivial.NoticethedfisobtainedasN(T1)k54(31)4104.Thecoefficientond89indicatesthatthescrapratewassubstantiallylowerin1989thaninthebaseyear,1987,evenintheabsenceofjobtraininggrants.Thus,itisimpor-tanttoallowfortheseaggregateeffects.Ifweomittedtheyeardummies,thesecularincreaseinworkerproductivitywouldbeattributedtothejobtraininggrants.TableQUESTION14.214.1showsthat,evenaftercontrollingforUndertheMichiganprogram,ifafirmreceivedagrantinoneyear,aggregatetrendsinproductivity,thejobitwasnoteligibleforagrantthefollowingyear.Whatdoesthistraininggrantshadalargeestimatedeffect.implyaboutthecorrelationbetweengrantandgrant1?Finally,itiscrucialtoallowforthelaggedeffectinthemodel.Ifweomitgrant1,thenweareassumingthattheeffectofjobtrainingdoesnotlastintothenextyear.Theesti-mateongrantwhenwedropgrant1is.082(t.65);thisismuchsmallerandstatis-ticallyinsignificant.Whenestimatinganunobservedeffectsmodelbyfixedeffects,itisnotclearhowweshouldcomputeagoodness-of-fitmeasure.TheR-squaredgiveninTable14.1isbasedonthewithintransformation:itistheR-squaredobtainedfromestimating(14.5).Thus,itisinterpretedastheamountoftimevariationintheyitthatisexplainedbythetimevariationintheexplanatoryvariables.OtherwaysofcomputingR-squaredarepossible,oneofwhichwediscusslater.Althoughtime-constantvariablescannotbeincludedbythemselvesinafixedeffectsmodel,theycanbeinteractedwithvariablesthatchangeovertimeand,inpar-ticular,withyeardummyvariables.Forexample,inawageequationwhereeducationisconstantovertimeforeachindividualinoursample,wecaninteracteducationwitheachyeardummytoseehowthereturntoeducationhaschangedovertime.Butwecannotusefixedeffectstoestimatethereturntoeducationinthebaseperiod—whichmeanswecannotestimatethereturntoeducationinanyperiod—wecanonlyseehowthereturntoeducationineachyeardiffersfromthatinthebaseperiod.Whenweincludeafullsetofyeardummies—thatis,yeardummiesforallyearsbutthefirst—wecannotestimatetheeffectofanyvariablewhosechangeacrosstimeisconstant.Anexampleisyearsofexperienceinapaneldatasetwhereeachpersonworksineveryyear,sothatexperiencealwaysincreasesbyoneineachyear,foreverypersoninthesample.Thepresenceofaiaccountsfordifferencesacrosspeopleintheir444\nChapter14AdvancedPanelDataMethodsyearsofexperienceintheinitialtimeperiod.Butthentheeffectofaone-yearincreaseinexperiencecannotbedistinguishedfromtheaggregatetimeeffects(becauseexperi-enceincreasesbythesameamountforeveryone).Thiswouldalsobetrueif,inplaceofseparateyeardummies,weusedalineartimetrend:foreachperson,experiencecan-notbedistinguishedfromalineartrend.EXAMPLE14.2(HastheReturntoEducationChangedOverTime?)ThedatainWAGEPAN.RAWarefromVellaandVerbeek(1998).Eachofthe545meninthesampleworkedineveryyearfrom1980through1987.Somevariablesinthedatasetchangeovertime:experience,maritalstatus,andunionstatusarethethreeimportantones.Othervariablesdonotchange:raceandeducationarethekeyexamples.Ifweusefixedeffects(orfirstdifferencing),wecannotincluderace,education,orexperienceintheequation.However,wecanincludeinteractionsofeducwithyeardummiesfor1981through1987totestwhetherthereturntoeducationwasconstantoverthistimeperiod.Weuselog(wage)asthedependentvariable,aquadraticinexperience,dummyvariablesformaritalandunionstatus,afullsetofyeardummies,andtheinteractiontermsd81educ,d82educ,…,d87educ.Theestimatesontheseinteractiontermsareallpositive,andtheygenerallygetlargerformorerecentyears.Thelargestcoefficientof.030isond87educ,witht2.48.Inotherwords,thereturntoeducationisestimatedtobeabout3percentagepointslargerin1987thaninthebaseyear,1980.(Wedonothaveanestimateofthereturntoeducationinthebaseyearforthereasonsgivenearlier.)Theothersignificantinteractiontermisd86educ(coefficient.027,t2.23).Theestimatesontheearlieryearsaresmallerandinsignifi-cantatthe5%levelagainstatwo-sidedalternative.IfwedoajointFtestforsignificanceofallseveninteractionterms,wegetp-value.28:thisgivesanexamplewhereasetofvariablesisjointlyinsignificanteventhoughsomevariablesareindividuallysignificant.[ThedffortheFtestare7and3,799;thesecondofthesecomesfromN(T1)k545(81)163,799.]Generally,theresultsareconsistentwithanincreaseinthereturntoeducationoverthisperiod.TheDummyVariableRegressionAtraditionalviewofthefixedeffectsmodelistoassumethattheunobservedeffect,ai,isaparametertobeestimatedforeachi.Thus,inequation(14.4),aiistheinterceptforpersoni(orfirmi,cityi,andsoon)thatistobeestimatedalongwiththej.(Clearlywecannotdothiswithasinglecrosssection:therewouldbeNkparameterstoesti-matewithonlyNobservations.Weneedatleasttwotimeperiods.)Thewayweesti-mateaninterceptforeachiistoputinadummyvariableforeachcross-sectionalobservation,alongwiththeexplanatoryvariables(andprobablydummyvariablesforeachtimeperiod).Thismethodisusuallycalledthedummyvariableregression.EvenwhenNisnotverylarge(say,N54asinExample14.1),thisresultsinmanyexplana-toryvariables—inmostcases,toomanytoexplicitlycarryouttheregression.Thus,the445\nPart3AdvancedTopicsdummyvariablemethodisnotverypracticalforpaneldatasetswithmanycross-sectionalobservations.Nevertheless,thedummyvariableregressionhassomeinterestingfeatures.Mostimportantly,itgivesusexactlythesameestimatesofthejthatwewouldobtainfromtheregressionontime-demeaneddata,andthestandarderrorsandothermajorstatis-ticsareidentical.Therefore,thefixedeffectsestimatorcanbeobtainedbythedummyvariableregression.Onebenefitofthedummyvariableregressionisthatitproperlycomputesthedegreesoffreedomdirectly.Thisisaminoradvantagenowthatmanyeconometricspackageshaveprogrammedfixedeffectsoptions.TheR-squaredfromthedummyvariableregressionisusuallyratherhigh.Thisisbecauseweareincludingadummyvariableforeachcross-sectionalunit,whichexplainsmuchofthevariationinthedata.Forexample,ifweestimatetheunobservedeffectsmodelinExample13.8byfixedeffectsusingthedummyvariableregression2(whichispossiblewithN22),thenR.933.WeshouldnotgettooexcitedaboutthislargeR-squared:itisnotsurprisingthatwecanexplainmuchofthevariationinunemploymentclaimsusingbothyearandcitydummies.JustasinExample13.8,the2estimateontheEZdummyvariableismoreimportantthanR.TheR-squaredfromthedummyvariableregressioncanbeusedtocomputeFtestsintheusualway,assumingofcoursethattheclassicallinearmodelassumptionshold(seethechapterappendix).Inparticular,wecantestthejointsignificanceofallofthecross-sectionaldummies(N1,sinceoneunitischosenasthebasegroup).Theunre-strictedR-squaredisobtainedfromtheregressionwithallofthecross-sectionaldum-mies;therestrictedR-squaredomitsthese.Inthevastmajorityofapplications,thedummyvariableswillbejointlysignificant.Occasionally,theestimatedintercepts,sayaˆi,areofinterest.Thisisthecaseifwewanttostudythedistributionoftheaˆiacrossi,orifwewanttopickaparticularfirmorcitytoseewhetheritsaˆiisaboveorbelowtheaveragevalueinthesample.Theseestimatesaredirectlyavailablefromthedummyvariableregression,buttheyarerarelyreportedbypackagesthathavefixedeffectsroutines(forthepracticalreasonthattherearesomanyaˆi).AfterfixedeffectsestimationwithNofanysize,theaˆiareprettyeasytocompute:aˆiy¯iˆ1x¯i1…ˆkx¯ik,i1,…,N,(14.6)wheretheoverbarreferstothetimeaveragesandtheˆjarethefixedeffectsestimates.Forexample,ifwehaveestimatedamodelofcrimewhilecontrollingforvarioustime-varyingfactors,wecanobtainaˆiforacitytoseewhethertheunobservedfixedeffectsthatcontributetocrimeareaboveorbelowaverage.Inmoststudies,theˆjareofinterest,andsothetime-demeanedequationsareusedtoobtaintheseestimates.Further,itisusuallybesttoviewtheaiasomittedvariablesthatwecontrolforthroughthewithintransformation.Thesenseinwhichtheaicanbeestimatedisgenerallyweak.Infact,eventhoughaˆiisunbiased(underassumptionsFE.1throughFE.4inthechapterappendix),itisnotconsistentwithafixedTasN*.Thereasonisthat,asweaddeachadditionalcross-sectionalobservation,weaddanewai.NoinformationaccumulatesoneachaiwhenTisfixed.WithlargerT,wecangetbet-terestimatesoftheai,butmostpaneldatasetsareofthelargeNandsmallTvariety.446\nChapter14AdvancedPanelDataMethodsFixedEffectsorFirstDifferencing?Sofar,wehaveseentwomethodsforestimatingunobservedeffectsmodels.Oneinvolvesdifferencingthedata,andtheotherinvolvestime-demeaning.Howdoweknowwhichonetouse?Wecaneliminateonecaseimmediately:whenT2,theFEandFDestimatesandallteststatisticsareidentical,andsoitdoesnotmatterwhichweuse.Firstdifferenc-inghastheadvantageofbeingstraightforwardinvirtuallyanyeconometricspackage,anditiseasytocomputeheteroskedasticity-robuststatisticsintheFDregression.WhenT3,theFEandFDestimatorsarenotthesame.SincebothareunbiasedunderAssumptionsFE.1throughFE.4,wecannotuseunbiasednessasacriterion.Further,bothareconsistent(withTfixedasN*)underFE.1throughFE.4.ForlargeNandsmallT,thechoicebetweenFEandFDhingesontherelativeefficiencyoftheestimators,andthisisdeterminedbytheserialcorrelationintheidiosyncraticerrors,uit.(Wewillassumehomoskedasticityoftheuit,sinceefficiencycomparisonsrequirehomoskedasticerrors.)Whentheuitareseriallyuncorrelated,fixedeffectsismoreefficientthanfirstdif-ferencing(andthestandarderrorsreportedfromfixedeffectsarevalid).Sincethefixedeffectsmodelisalmostalwaysstatedwithseriallyuncorrelatedidiosyncraticerrors,theFEestimatorisusedmoreoften.Butweshouldrememberthatthisassumptioncanbefalse.Inmanyapplications,wecanexpecttheunobservedfactorsthatchangeovertimetobeseriallycorrelated.Ifuitfollowsarandomwalk—whichmeansthatthereisverysubstantial,positiveserialcorrelation—thenthedifferenceuitisseriallyuncorrelated,andfirstdifferencingisbetter.Inmanycases,theuitexhibitsomepositiveserialcorre-lation,butperhapsnotasmuchasarandomwalk.Then,wecannoteasilycomparetheefficiencyoftheFEandFDestimators.ItisdifficulttotestwhethertheuitareseriallyuncorrelatedafterFEestimation:wecanestimatethetime-demeanederrors,uit,butnottheuit.However,inSection13.3,weshowedhowtotestwhetherthedifferencederrors,uit,areseriallyuncorrelated.Ifthisseemstobethecase,FDcanbeused.Ifthereissubstantialnegativeserialcorrelationintheuit,FEisprobablybetter.Itisoftenagoodideatotryboth:iftheresultsarenotsensitive,somuchthebetter.WhenTislarge,andespeciallywhenNisnotverylarge(forexample,N20andT30),wemustexercisecautioninusingthefixedeffectsestimator.Whileexactdis-tributionalresultsholdforanyNandTundertheclassicalfixedeffectsassumptions,theyareextremelysensitivetoviolationsoftheassumptionswhenNissmallandTislarge.Inparticular,ifweareusingunitrootprocesses—seeChapter11—thespuriousregressionproblemcanarise.AswesawinChapter11,differencinganintegratedprocessresultsinaweaklydependentprocess,andwemustappealtothecentrallimitapproximations.Inthiscase,usingdifferencesisfavorable.Ontheotherhand,fixedeffectsturnsouttobelesssensitivetoviolationofthestrictexogeneityassumption,especiallywithlargeT.Someauthorsevenrecommendesti-matingfixedeffectsmodelswithlaggeddependentvariables(whichclearlyviolatesAssumptionFE.3inthechapterappendix).WhentheprocessesareweaklydependentovertimeandTislarge,thebiasinthefixedeffectsestimatorcanbesmall[see,forexample,Wooldridge(1999,Chapter11)].447\nPart3AdvancedTopicsItisdifficulttochoosebetweenFEandFDwhentheygivesubstantivelydifferentresults.Itmakessensetoreportbothsetsofresultsandtotrytodeterminewhytheydiffer.FixedEffectswithUnbalancedPanelsSomepaneldatasets,especiallyonindividualsorfirms,havemissingyearsforatleastsomecross-sectionalunitsinthesample.Inthiscase,wecallthedatasetanunbal-ancedpanel.Themechanicsoffixedeffectsestimationwithanunbalancedpanelarenotmuchmoredifficultthanwithabalancedpanel.IfTiisthenumberoftimeperiodsforcross-sectionaluniti,wesimplyusetheseTiobservationsindoingthetime-demeaning.ThetotalnumberofobservationsisthenT1T2…TN.Asinthebal-ancedcase,onedegreeoffreedomislostforeverycross-sectionalobservationduetothetime-demeaning.Anyregressionpackagethatdoesfixedeffectsmakestheappro-priateadjustmentforthisloss.Thedummyvariableregressionalsogoesthroughinexactlythesamewayaswithabalancedpanel,andthedfisappropriatelyobtained.Itiseasytoseethatunitsforwhichwehaveonlyasingletimeperiodplaynoroleinafixedeffectsanalysis.Thetime-demeaningforsuchobservationsyieldsallzeros,whicharenotusedintheestimation.(IfTiisatmosttwoforalli,wecanusefirstdif-ferencing:ifTi1foranyi,wedonothavetwoperiodstodifference.)Themoredifficultissuewithanunbalancedpanelisdeterminingwhythepanelisunbalanced.Withcitiesandstates,forexample,dataonkeyvariablesaresometimesmissingforcertainyears.Providedthereasonwehavemissingdataforsomeiisnotcorrelatedwiththeidiosyncraticerrors,uit,theunbalancedpanelcausesnoproblems.Whenwehavedataonindividuals,families,orfirms,thingsaretrickier.Imagine,forexample,thatweobtainarandomsampleofmanufacturingfirmsin1990,andweareinterestedintestinghowunionizationaffectsfirmprofitability.Ideally,wecanuseapaneldataanalysistocontrolforunobservedworkerandmanagementcharacteristicsthataffectprofitabilityandmightalsobecorrelatedwiththefractionofthefirm’sworkforcethatisunionized.Ifwecollectdataagaininsubsequentyears,somefirmsmaybelostbecausetheyhavegoneoutofbusinessorhavemergedwithothercompanies.Ifso,weprobablyhaveanonrandomsampleinsubsequenttimeperiods.Thequestionis:Ifweapplyfixedeffectstotheunbalancedpanel,whenwilltheestimatorsbeunbiased(oratleastconsistent)?Ifthereasonafirmleavesthesample(calledattrition)iscorrelatedwiththeidio-syncraticerror—thoseunobservedfactorsthatchangeovertimeandaffectprofits—thentheresultingsamplesectionproblem(seeChapter9)cancausebiasedestimators.Thisisaseriousconsiderationinthisexample.Nevertheless,oneusefulthingaboutafixedeffectsanalysisisthatitdoesallowattritiontobecorrelatedwithai,theunob-servedeffect.Theideaisthat,withtheinitialsampling,someunitsaremorelikelytodropoutofthesurvey,andthisiscapturedbyai.EXAMPLE14.3(EffectofJobTrainingonFirmScrapRates)WeaddtwovariablestotheanalysisinTable14.1:log(salesit)andlog(employit),wheresalesisannualfirmsalesandemployisnumberofemployees.Threeofthe54firmsdropoutof448\nChapter14AdvancedPanelDataMethodstheanalysisentirelybecausetheydonothavesalesoremploymentdata.Fiveadditionalobservationsarelostduetomissingdataononeorbothofthesevariablesforsomeyears,leavinguswithn148.Usingfixedeffectsontheunbalancedpaneldoesnotchangethebasicstory,althoughtheestimatedgranteffectgetslarger:ˆgrant.297,tgrant1.89;ˆgrant1.536,tgrant12.389.Solvingattritionproblemsinpaneldataiscomplicatedandbeyondthescopeofthistext.[See,forexample,Wooldridge(1999,Chapter17).]14.2RANDOMEFFECTSMODELSWebeginwiththesameunobservedeffectsmodelasbefore,yit01xit1…kxitkaiuit,(14.7)whereweexplicitlyincludeaninterceptsothatwecanmaketheassumptionthattheunobservedeffect,ai,haszeromean(withoutlossofgenerality).Wewouldusuallyallowfortimedummiesamongtheexplanatoryvariablesaswell.Inusingfixedeffectsorfirstdifferencing,thegoalistoeliminateaibecauseitisthoughttobecorrelatedwithoneormoreofthexitj.Butsupposewethinkaiisuncorrelatedwitheachexplanatoryvariableinalltimeperiods?Then,usingatransformationtoeliminateairesultsininef-ficientestimators.Equation(14.7)becomesarandomeffectsmodelwhenweassumethattheunob-servedeffectaiisuncorrelatedwitheachexplanatoryvariable:Cov(xitj,ai)0,t1,2,…,T;j1,2,…,k.(14.8)Infact,theidealrandomeffectsassumptionsincludeallofthefixedeffectsassumptionsplustheadditionalrequirementthataiisindependentofallexplanatoryvariablesinalltimeperiods.(Seethechapterappendixfortheactualassumptionsused.)Ifwethinktheunobservedeffectaiiscorrelatedwithanyexplanatoryvariables,weshouldusefirstdifferencingforfixedeffects.Under(14.8)andalongwiththerandomeffectsassumptions,howshouldweesti-matethej?Itisimportanttoseethat,ifwebelievethataiisuncorrelatedwiththeexplanatoryvariables,thejcanbeconsistentlyestimatedbyusingasinglecrosssec-tion:thereisnoneedforpaneldataatall.Butusingasinglecrosssectiondisregardsmuchusefulinformationintheothertimeperiods.WecanusethisinformationinapooledOLSprocedure:justrunOLSofyitontheexplanatoryvariablesandprobablythetimedummies.This,too,producesconsistentestimatorsofthejundertherandomeffectsassumption.Butitignoresakeyfeatureofthemodel.Ifwedefinethecompos-iteerrortermasvitaiuit,then(14.7)canbewrittenasyit01xit1…kxitkvit.(14.9)449\nPart3AdvancedTopicsBecauseaiisinthecompositeerrorineachtimeperiod,thevitareseriallycorrelatedacrosstime.Infact,undertherandomeffectsassumptions,222Corr(vit,vis)a/(au),ts,22whereaVar(ai)anduVar(uit).This(necessarily)positiveserialcorrelationintheerrortermcanbesubstantial:becausetheusualpooledOLSstandarderrorsignorethiscorrelation,theywillbeincorrect,aswilltheusualteststatistics.InChapter12,weshowedhowgeneralizedleastsquarescanbeusedtoestimatemodelswithautoregres-siveserialcorrelation.WecanalsouseGLStosolvetheserialcorrelationproblemhere.Inorderfortheproceduretohavegoodproperties,itmusthavelargeNandrelativelysmallT.Weassumethatwehaveabalancedpanel,althoughthemethodcanbeextendedtounbalancedpanels.DerivingtheGLStransformationthateliminatesserialcorrelationintheerrorsrequiressophisticatedmatrixalgebra[see,forexample,Wooldridge(1999)Chapter10].Butthetransformationitselfissimple.Define2221/21[u/(uTa)],(14.10)whichisbetweenzeroandone.Then,thetransformedequationturnsouttobeyity¯i0(1)1(xit1x¯i1)…(14.11)k(xitkx¯ik)(vitv¯i),wheretheoverbaragaindenotesthetimeaverages.Thisisaveryinterestingequation,asitinvolvesaquasi-demeaneddataoneachvariable.Thefixedeffectsestimatorsub-tractsthetimeaveragesfromthecorrespondingvariable.Therandomeffectstransfor-22mationsubtractsafractionofthattimeaverage,wherethefractiondependsonu,a,andthenumberoftimeperiods,T.TheGLSestimatorissimplythepooledOLSesti-matorofequation(14.11).Itishardlyobviousthattheerrorsin(14.11)areseriallyuncorrelated,buttheyare.Thetransformationin(14.11)allowsforexplanatoryvariablesthatareconstantovertime,andthisisoneadvantageofrandomeffects(RE)overeitherfixedeffectsorfirstdifferencing.ThisispossiblebecauseREassumesthattheunobservedeffectisuncorrelatedwithallexplanatoryvariables,whethertheyarefixedovertimeornot.Thus,inawageequation,wecanincludeavariablesuchaseducationevenifitdoesnotchangeovertime.Butweareassumingthateducationisuncorrelatedwithai,whichcontainsabilityandfamilybackground.Inmanyapplications,thewholereasonforusingpaneldataistoallowtheunobservedeffecttobecorrelatedwiththeexplanatoryvariables.Theparameterisneverknowninpractice,butitcanalwaysbeestimated.Therearedifferentwaystodothis,whichmaybebasedonpooledOLSorfixedeffects,for221/22example.Generally,ˆtakestheformˆ1{1/[1T(ˆa/ˆu)]},whereˆaisa222consistentestimatorofaandˆuisaconsistentestimatorofu.TheseestimatorscanbebasedonthepooledOLSorfixedeffectsresiduals.OnepossibilityisthatNT1T21ˆa[NT(T1)/2k]vˆitvˆis,wherethevˆitaretheresidualsfromesti-i1t1st1450\nChapter14AdvancedPanelDataMethods2222mating(14.9)bypooledOLS.Giventhis,wecanestimateubyusingˆuˆvˆa,2whereˆvisthesquareoftheusualstandarderroroftheregressionfrompooledOLS.[SeeWooldridge(1999,Chapter10)foradditionaldiscussionoftheseestimators.]Manyeconometricspackagessupportestimationofrandomeffectsmodelsandautomaticallycomputesomeversionofˆ.ThefeasibleGLSestimatorthatusesˆinplaceofiscalledtherandomeffectsestimator.Undertherandomeffectsassump-tionsinthechapterappendix,theestimatorisconsistent(notunbiased)andasymptoti-callynormallydistributedasNgetslargewithfixedT.ThepropertiesoftheREestimatorwithsmallNandlargeTarelargelyunknown,althoughithascertainlybeenusedinsuchsituations.Equation(14.11)allowsustorelatetheREestimatortobothpooledOLSandfixedeffects.PooledOLSisobtainedwhen0,andFEisobtainedwhen1.Inprac-tice,theestimateˆisneverzeroorone.Butifˆisclosetozero,theREestimateswillbeclosetothepooledOLSestimates.Thisisthecasewhentheunobservedeffect,ai,2isrelativelyunimportant(sinceithassmallvariancerelativetou).Itismorecommon22foratobelargerelativetou,inwhichcaseˆwillbeclosertounity.AsTgetslarge,ˆtendstoone,andthismakestheREandFEestimatesverysimilar.EXAMPLE14.4(AWageEquationUsingPanelData)WeagainusethedatainWAGEPAN.RAWtoestimateawageequationformen.Weusethreemethods:pooledOLS,randomeffects,andfixedeffects.Inthefirsttwomethods,wecanincludeeducandracedummies(blackandhispan),butthesedropoutofthefixed2effectsanalysis.Thetime-varyingvariablesareexper,exper,union,andmarried.Aswedis-2cussedinSection14.1,experisdroppedintheFEanalysis(butexperremains).Eachregres-sionalsocontainsafullsetofyeardummies.TheestimationresultsareinTable14.2.Thecoefficientsoneduc,black,andhispanaresimilarforthepooledOLSandrandomeffectsestimations.ThepooledOLSstandarderrorsaretheusualOLSstandarderrors,andtheseunderestimatethetruestandarderrorsbecausetheyignorethepositiveserialcorre-lation;wereportthemhereforcomparisononly.Theexperienceprofileissomewhatdif-ferent,andboththemarriageandunionpremiumsfallnotablyintherandomeffectsestimation.Whenweeliminatetheunobservedeffectentirelybyusingfixedeffects,themarriagepremiumfallstoabout4.7%,althoughitisstillstatisticallysignificant.Thedropinthemarriagepremiumisconsistentwiththeideathatmenwhoaremoreable—asQUESTION14.3capturedbyahigherunobservedeffect,ai—Theunionpremiumestimatedbyfixedeffectsisabout10percent-aremorelikelytobemarried.Therefore,inagepointslowerthantheOLSestimate.WhatdoesthisstronglythepooledOLSestimation,alargepartofsuggestaboutthecorrelationbetweenunionandtheunobservedthemarriagepremiumreflectsthefactthateffect?menwhoaremarriedwouldearnmoreeveniftheywerenotmarried.Theremaining4.7%hasatleasttwopossibleexplanations:(1)marriagereallymakesmenmoreproduc-tiveor(2)employerspaymarriedmenapremiumbecausemarriageisasignalofstability.Wecannotdistinguishbetweenthesetwohypotheses.451\nPart3AdvancedTopicsTable14.2ThreeDifferentEstimatorsofaWageEquationDependentVariable:log(wage)IndependentPooledRandomFixedVariablesOLSEffectsEffectseduc.091.092—(.005)(.011)black.139.139—(.024)(.048)hispan.016.022—(.021)(.043)exper.067.106—(.014)(.015)2exper.0024.0047.0052(.0008)(.0007)(.0007)married.108.064.047(.016)(.017)(.018)union.182.106.080(.017)(.018)(.019)Theestimateoffortherandomeffectsestimationisˆ.643,whichexplainswhy,onthetime-varyingvariables,theREestimateslieclosertotheFEestimatesthantothepooledOLSestimates.RandomEffectsorFixedEffects?Inreadingempiricalwork,youmayfindthatauthorsdecidebetweenfixedandrandomeffectsbasedonwhethertheai(orwhatevernotationtheauthorsuse)arebestviewedasparameterstobeestimatedorasoutcomesofarandomvariable.Whenwecannotconsidertheobservationstoberandomdrawsfromalargepopulation—forexample,ifwehavedataonstatesorprovinces—itoftenmakessensetothinkoftheaiasparame-terstoestimate,inwhichcaseweusefixedeffectsmethods.Rememberthatusingfixedeffectsisthesameasallowingadifferentinterceptforeachobservation,andwecanestimatetheseinterceptsbyincludingdummyvariablesorby(14.6).452\nChapter14AdvancedPanelDataMethodsEvenifwedecidetotreattheaiasrandomvariables,wemustdecidewhethertheaiareuncorrelatedwiththeexplanatoryvariables.Peoplesometimesmistakenlybelievethatassumingaiisrandomautomaticallymeansthatrandomeffectsistheappropriateestimationstrategy.Ifwecanassumetheaiareuncorrelatedwithallxit,thentherandomeffectsmethodisappropriate.Butiftheaiarecorrelatedwithsomeexplanatoryvariables,thefixedeffectsmethod(orfirstdifferencing)isneeded;ifREisused,thentheestimatorsaregenerallyinconsistent.ComparingtheFEandREestimatescanbeatestforwhetherthereiscorrelationbetweentheaiandthexitj,assumingthattheidiosyncraticerrorsandexplanatoryvari-ablesareuncorrelatedacrossalltimeperiods.Hausman(1978)firstsuggestedthistest.Someeconometricspackagesroutinelycomputethetestundertheidealrandomeffectsassumptionslistedinthechapterappendix.DetailsonthisstatisticcanbefoundinWooldridge(1999,Chapter10).14.3APPLYINGPANELDATAMETHODSTOOTHERDATASTRUCTURESDifferencing,fixedeffects,andrandomeffectsmethodscanbeappliedtodatastruc-turesthatdonotinvolvetime.Forexample,indemography,itiscommontousesiblings(sometimestwins)tocontrolforunobservedfamilyandbackgroundcharacter-istics.Differencingacrosssiblingsor,moregenerally,usingthewithintransformationwithinafamily,removesfamilyeffectsthatmaybecorrelatedwiththeexplanatoryvariables.Asanexample,GeronimusandKorenman(1992)usepairsofsisterstostudytheeffectsofteenchildbearingonfutureeconomicoutcomes.Whentheoutcomeisincomerelativetoneeds—somethingthatdependsonthenumberofchildren—themodelislog(incneedsfs)00sister2s1teenbrthfs(14.12)2agefsotherfactorsafufs,wherefindexesfamilyandsindexesasisterwithinthefamily.Theinterceptforthefirstsisteris0,andtheinterceptforthesecondsisteris00.Thevariableofinterestisteenbrthfs,whichisabinaryvariableequaltooneifsistersinfamilyfhadachildwhileateenager.Thevariableagefsisthecurrentageofsistersinfamilyf;GeronimusandKorenmanalsousesomeothercontrols.Theunobservedvariableaf,whichchangesonlyacrossfamily,isanunobservedfamilyeffectorafamilyfixedeffect.Themaincon-cernintheanalysisisthatteenbrthiscorrelatedwiththefamilyeffect.Ifso,anOLSanalysisthatpoolsacrossfamiliesandsistersgivesabiasedestimatoroftheeffectofteenagemotherhoodoneconomicoutcomes.Solvingthisproblemissimple:withineachfamily,difference(14.12)acrosssisterstogetlog(incneeds)01teenbrth2age…u;(14.13)thisremovesthefamilyeffect,af,andtheresultingequationcanbeestimatedbyOLS.Noticethatthereisnotimeelementhere:thedifferencingisacrosssisterswithinafamily.453\nPart3AdvancedTopicsUsing129sisterpairsfromthe1982NationalLongitudinalSurveyofYoungWomen,GeronimusandKorenmanfirstestimate1bypooledOLStoobtain.33or.26,wherethesecondestimatecomesfromcontrollingforfamilybackgroundvari-ables(suchasparents’education);bothestimatesareverystatisticallysignificant[seeTable3inGeronimusandKorenman(1992)].Therefore,teenagemotherhoodQUESTION14.4hasaratherlargeimpactonfuturefamilyWhenusingthedifferencingmethod,doesitmakesensetoincludeincome.However,whenthedifferenceddummyvariablesforthemotherandfather’sracein(14.12)?equationisestimated,thecoefficientonExplain.teenbrthis.08,whichissmallandstatis-ticallyinsignificant.Thissuggeststhatitislargelyawoman’sfamilybackgroundthataffectsherfutureincome,ratherthanteenagechildbearing.GeronimusandKorenmanlookatseveralotheroutcomesandtwootherdatasets;insomecases,thewithinfamilyestimatesareeconomicallylargeandstatisticallysig-nificant.Theyalsoshowhowtheeffectsdisappearentirelywhenthesisters’educationlevelsarecontrolledfor.AshenfelterandKrueger(1994)usedthedifferencingmethodologytoestimatethereturntoeducation.Theyobtainedasampleof149identicaltwinsandcollectedinfor-mationonearnings,education,andothervariables.Thereasonforusingidenticaltwinsisthattheyshouldhavethesameunderlyingability.Thiscanbedifferencedawaybyusingtwindifferences,ratherthanOLSonthepooleddata.Becauseidenticaltwinsarethesameinage,gender,andrace,thesefactorsalldropoutofthedifferencedequation.Therefore,AshenfelterandKruegerregressedthedifferenceinlog(earnings)onthedif-ferenceineducationandestimatedthereturntoeducationtobeabout9.2%(t3.83).Interestingly,thisisactuallylargerthanthepooledOLSestimateof8.4%(whichcon-trolsforgender,age,andrace).AshenfelterandKruegeralsoestimatedtheequationbyrandomeffectsandobtained8.7%asthereturntoeducation.(SeeTable5intheirpaper.)Therandomeffectsanalysisismechanicallythesameasthepaneldatacasewithtwotimeperiods.ThesamplesusedbyGeronimusandKorenman(1992)andAshenfelterandKrueger(1994)areexamplesofmatchedpairsamples.Generally,fixedandrandomeffectsmethodscanbeappliedtoaclustersample.Thesearecross-sectionaldatasets,buteachobservationbelongstoawell-definedcluster.Inthepreviousexamples,eachfamilyisacluster.Asanotherexample,supposewehaveparticipationdataonvariouspensionplans,wherefirmsoffermorethanoneplan.Wecanthenvieweachfirmasacluster,anditisprettyclearthatunobservedfirmeffectswouldbeanimportantfactorindeterminingparticipationratesinpensionplanswithinthefirm.Educationaldataonstudentssampledfrommanyschoolsformaclustersample,whereeachschoolisacluster.Sincetheoutcomeswithinaclusterarelikelytobecor-related,allowingforanunobservedclustereffectistypicallyimportant.Fixedeffectsestimationispreferredwhenwethinktheunobservedclustereffect—anexampleofwhichisafin(14.12)—iscorrelatedwithoneormoreoftheexplanatoryvariables.Then,wecanonlyincludeexplanatoryvariablesthatvary,atleastsomewhat,withinclusters.Theclustersizesarerarelythesame,sofixedeffectsmethodsforunbalancedpanelsareusuallyrequired.454\nChapter14AdvancedPanelDataMethodsRandomeffectsmethodscanalsobeusedwithunbalancedclusters,providedtheclustereffectisuncorrelatedwithalltheexplanatoryvariables.WecanalsousepooledOLSinthiscase,buttheusualstandarderrorsareincorrectunlessthereisnocorrela-tionwithinclusters.Someregressionpackageshavesimplecommandstocorrectstan-darderrorsandtheusualteststatisticsforgeneralwithinclustercorrelation(aswellasheteroskedasticity).ThesearethesamecorrectionsthatworkforpooledOLSonpaneldatasets,whichwereportedinExample13.9.Asanexample,Papke(1999)estimateslinearprobabilitymodelsforthecontinuationofdefinedbenefitpensionplansbasedonwhetherfirmsadopteddefinedcontributionplans.Becausethereislikelytobeafirmeffectthatinducescorrelationacrossdifferentplanswithinthesamefirm,Papkecor-rectstheusualOLSstandarderrorsforclustersampling,aswellasforheteroskedas-ticityinthelinearprobabilitymodel.SUMMARYWehavestudiedtwocommonmethodsforestimatingpaneldatamodelswithunob-servedeffects.Comparedwithfirstdifferencing,thefixedeffectsestimatorisefficientwhentheidiosyncraticerrorsareseriallyuncorrelated(aswellashomoskedastic),andwemakenoassumptionsaboutcorrelationbetweentheunobservedeffectaiandtheexplanatoryvariables.Aswithfirstdifferencing,anytime-constantexplanatoryvari-ablesdropoutoftheanalysis.Fixedeffectsmethodsapplyimmediatelytounbalancedpanels,butwemustassumethatthereasonssometimeperiodsaremissingarenotsys-tematicallyrelatedtotheidiosyncraticerrors.Therandomeffectsestimatorisappropriatewhentheunobservedeffectisthoughttobeuncorrelatedwithalltheexplanatoryvariables.Then,aicanbeleftintheerrorterm,andtheresultingserialcorrelationovertimecanbehandledbygeneralizedleastsquaresestimation.Conveniently,feasibleGLScanbeobtainedbyapooledregressiononquasi-demeaneddata.Thevalueoftheestimatedtransformationparameter,ˆ,indi-cateswhethertheestimatesarelikelytobeclosertothepooledOLSorthefixedeffectsestimates.Ifthefullsetofrandomeffectsassumptionshold,therandomeffectsesti-matorisasymptotically—asNgetslargewithTfixed—moreefficientthanpooledOLS,firstdifferencing,orfixedeffects(whichareallunbiased,consistent,andasymp-toticallynormal).Finally,thepaneldatamethodsstudiedinChapters13and14canbeusedwhenworkingwithmatchedpairsorclustersamples.Differencingorthewithintransforma-tioneliminatestheclustereffect.Iftheclustereffectisuncorrelatedwiththeexplana-toryvariables,pooledOLScanbeused,butthestandarderrorsandteststatisticsshouldbeadjustedforclustercorrelation.Randomeffectsestimationisalsoapossibility.KEYTERMSClusterEffectFixedEffectsTransformationClusterSampleMatchedPairSamplesCompositeErrorTermQuasi-DemeanedDataDummyVariableRegressionRandomEffectsEstimatorFixedEffectsEstimatorRandomEffectsModel455\nPart3AdvancedTopicsTime-DemeanedDataWithinEstimatorUnbalancedPanelWithinTransformationPROBLEMS14.1Supposethattheidiosyncraticerrorsin(14.4),{uit:t1,2,…,T},areserially2uncorrelatedwithconstantvariance,u.Showthatthecorrelationbetweenadjacentdifferences,uitandui,t1,is.5.Therefore,undertheidealFEassumptions,firstdifferencinginducesnegativeserialcorrelationofaknownvalue.14.2Withasingleexplanatoryvariable,theequationusedtoobtainthebetweenesti-matorisy¯i01x¯iaiu¯i,wheretheoverbarrepresentstheaverageovertime.WecanassumethatE(ai)0becausewehaveincludedaninterceptintheequation.Supposethatu¯iisuncorrelatedwithx¯i,butCov(xit,ai)xaforallt(andibecauseofrandomsamplinginthecrosssection).(i)Letting˜1bethebetweenestimator,thatis,theOLSestimatorusingthetimeaverages,showthatplim˜11xa/Var(x¯i),wheretheprobabilitylimitisdefinedasN*.[Hint:Seeequations(5.5)and(5.6).](ii)Assumefurtherthatthexit,forallt1,2,…,T,areuncorrelatedwith22constantvariancex.Showthatplim˜11T(xa/x).(iii)Iftheexplanatoryvariablesarenotveryhighlycorrelatedacrosstime,whatdoespart(ii)suggestaboutwhethertheinconsistencyinthebetweenestimatorissmallerwhentherearemoretimeperiods?14.3Inarandomeffectsmodel,definethecompositeerrorvitaiuit,whereaiis2uncorrelatedwithuitandtheuithaveconstantvarianceuandareseriallyuncorrelated.Defineeitvitv¯i,whereisgivenin(14.10).Showthattheeithavemeanzero,constantvariance,andareseriallyuncorrelated.14.4Inordertodeterminetheeffectsofcollegiateathleticperformanceonapplicants,youcollectdataonapplicationsforasampleofDivisionIcollegesfor1985,1990,and1995.(i)Whatmeasuresofathleticsuccesswouldyouincludeinanequation?Whataresomeofthetimingissues?(ii)Whatotherfactorsmightyoucontrolforintheequation?(iii)Writeanequationthatallowsyoutoestimatetheeffectsofathleticsuc-cessonthepercentagechangeinapplications.Howwouldyouestimatethisequation?Whywouldyouchoosethismethod?14.5Supposethat,foronesemester,youcancollectthefollowingdataonarandomsampleofcollegejuniorsandseniorsforeachclasstaken:astandardizedfinalexam456\nChapter14AdvancedPanelDataMethodsscore,percentageoflecturesattended,adummyvariableindicatingwhethertheclassiswithinthestudent’smajor,cumulativegradepointaveragepriortothestartofthesemester,andSATscore.(i)Whywouldyouclassifythisdatasetasaclustersample?Roughlyhowmanyobservationswouldyouexpectforthetypicalstudent?(ii)Writeamodel,similartoequation(14.12),thatexplainsfinalexamper-formanceintermsofattendanceandtheothercharacteristics.Usestosubscriptstudentandctosubscriptclass.Whichvariablesdonotchangewithinastudent?(iii)IfyoupoolallofthedatatogetheranduseOLS,whatareyouassum-ingaboutunobservedstudentcharacteristicsthataffectperformanceandattendancerate?WhatrolesdoSATscoreandpriorGPAplayinthisregard?(iv)IfyouthinkSATscoreandpriorGPAdonotadequatelycapturestudentability,howwouldyouestimatetheeffectofattendanceonfinalexamperformance?COMPUTEREXERCISES14.6UsethedatainRENTAL.RAWforthisexercise.Thedataonrentalpricesandothervariablesforcollegetownsarefortheyears1980and1990.Theideaistoseewhetherastrongerpresenceofstudentsaffectsrentalrates.Theunobservedeffectsmodelislog(rentit)00y90t1log(popit)2log(avgincit)3pctstuitaiuit,wherepopiscitypopulation,avgincisaverageincome,andpctstuisstudentpopula-tionasapercentageofcitypopulation(duringtheschoolyear).(i)EstimatetheequationbypooledOLSandreporttheresultsinstandardform.Whatdoyoumakeoftheestimateonthe1990dummyvariable?Whatdoyougetforˆpctstu?(ii)Arethestandarderrorsyoureportinpart(i)valid?Explain.(iii)Now,differencetheequationandestimatebyOLS.Compareyouresti-mateofpctstuwiththatfrompart(i).Doestherelativesizeofthestu-dentpopulationappeartoaffectrentalprices?(iv)Estimatethemodelbyfixedeffectstoverifythatyougetidenticalesti-matesandstandarderrorstothoseinpart(iii).14.7UseCRIME4.RAWforthisexercise.(i)ReestimatetheunobservedeffectsmodelforcrimeinExample13.9butusefixedeffectsratherthandifferencing.Arethereanynotablesignormagnitudechangesinthecoefficients?Whataboutstatisticalsignifi-cance?(ii)Addthelogsofeachwagevariableinthedatasetandestimatethemodelbyfixedeffects.Howdoesincludingthesevariablesaffectthecoefficientsonthecriminaljusticevariablesinpart(i)?457\nPart3AdvancedTopics(iii)Dothewagevariablesinpart(ii)allhavetheexpectedsign?Explain.Aretheyjointlysignificant?14.8Forthisexercise,weuseJTRAIN.RAWtodeterminetheeffectofthejobtrain-inggrantonhoursofjobtrainingperemployee.Thebasicmodelforthethreeyearsishrsempit01d88t2d89t1grantit2granti,t13log(employit)aiuit.(i)Estimatetheequationusingfixedeffects.HowmanyfirmsareusedintheFEestimation?Howmanytotalobservationswouldbeusedifeachfirmhaddataonallvariables(inparticular,hrsemp)forallthreeyears?(ii)Interpretthecoefficientongrantandcommentonitssignificance.(iii)Isitsurprisingthatgrant1isinsignificant?Explain.(iv)Dolargerfirmsprovidetheiremployeeswithmoreorlesstraining,onaverage?Howbigarethedifferences?(Forexample,ifafirmhas10%moreemployees,whatisthechangeinaveragehoursoftraining?)14.9InExample13.8,weusedtheunemploymentclaimsdatafromPapke(1994)toestimatetheeffectofenterprisezonesonunemploymentclaims.Papkealsousesamodelthatallowseachcitytohaveitsowntimetrend:log(uclmsit)aicit1ezituit,whereaiandciarebothunobservedeffects.Thisallowsformoreheterogeneityacrosscities.(i)Showthat,whenthepreviousequationisfirstdifferenced,weobtainlog(uclmsit)ci1ezituit,t2,…,T.Noticethatthedifferencedequationcontainsafixedeffect,ci.(ii)Estimatethedifferencedequationbyfixedeffects.Whatistheestimateof1?IsitverydifferentfromtheestimateobtainedinExample13.8?Istheeffectofenterprisezonesstillstatisticallysignificant?(iii)Addafullsetofyeardummiestotheestimationinpart(ii).Whathap-penstotheestimateof1?14.10(i)InthewageequationinExample14.4,explainwhydummyvariablesforoccupationmightbeimportantomittedvariablesforestimatingtheunionwagepremium.(ii)UsingthedatainWAGEPAN.RAW,includeeightoftheoccupationdummyvariablesintheequationandestimatetheequationusingfixedeffects.Doesthecoefficientonunionchangebymuch?Whataboutitsstatisticalsignificance?14.11AddtheinteractiontermunionitttotheequationestimatedinTable14.2toseeifwagegrowthdependsonunionstatus.Estimatetheequationbyrandomandfixedeffectsandcomparetheresults.458\nChapter14AdvancedPanelDataMethodsAPPENDIX14AAssumptionsforFixedandRandomEffectsInthisappendix,weprovidestatementsoftheassumptionsforfixedandrandomeffectsestimation.Wealsoprovideadiscussionofthepropertiesoftheestimatorsunderdif-ferentsetsofassumptions.Verificationoftheseclaimsissomewhatinvolved,butitcanbefoundinWooldridge(1999,Chapter10).ASSUMPTIONFE.1Foreachi,themodelisyit1xit1…kxitkaiuit,t1,…,T,wherethejaretheparameterstoestimate.ASSUMPTIONFE.2Wehavearandomsampleinthecross-sectionaldimension.ASSUMPTIONFE.3Foreacht,theexpectedvalueoftheidiosyncraticerrorgiventheexplanatoryvariablesinalltimeperiodsandtheunobservedeffectiszero:E(uitXi,ai)0.ASSUMPTIONFE.4Eachexplanatoryvariablechangesovertime(foratleastsomei),andtherearenoperfectlinearrelationshipsamongtheexplanatoryvariables.Underthesefirstfourassumptions—whichareidenticaltotheassumptionsforthefirst-differencingestimator—thefixedeffectsestimatorisunbiased.Again,thekeyisthestrictexogeneityassumption,FE.3.Underthesesameassumptions,theFEestima-torisconsistentwithafixedTasN*.ASSUMPTIONFE.52Var(uitXi,ai)Var(uit)u,forallt1,…,T.ASSUMPTIONFE.6Forallts,theidiosyncraticerrorsareuncorrelated(conditionalonallexplanatoryvari-ablesandai):Cov(uit,uisXi,ai)0.UnderAssumptionsFE.1throughFE.6,thefixedeffectsestimatorofthejisthebestlinearunbiasedestimator.SincetheFDestimatorislinearandunbiased,itisnec-essarilyworsethantheFEestimator.TheassumptionthatmakesFEbetterthanFDisFE.6,whichimpliesthattheidiosyncraticerrorsareseriallyuncorrelated.459\nPart3AdvancedTopicsASSUMPTIONFE.7ConditionalonXiandai,theuitareindependentandidenticallydistributedas2Normal(0,u).AssumptionFE.7impliesFE.3,FE.5,andFE.6,butitisstrongerbecauseitassumesanormaldistributionfortheidiosyncraticerrors.IfweaddFE.7,theFEestimatorisnor-mallydistributed,andtandFstatisticshaveexacttandFdistributions.WithoutFE.7,wecanrelyonasymptoticapproximations.But,withoutmakingspecialassumptions,theseapproximationsrequirelargeNandsmallT.TheidealrandomeffectsassumptionsincludeFE.1,FE.2,FE.3,FE.5,andFE.6.Wecannowallowfortime-constantvariables.(FE.7couldbeadded,butitgainsuslittleinpractice.)However,weneedtoaddassumptionsabouthowaiisrelatedtotheexplana-toryvariables.Thus,thethirdassumptionisstrengthenedasfollows.ASSUMPTIONRE.3InadditiontoFE.3,theexpectedvalueofaigivenallexplanatoryvariablesiszero:E(aiXi)0.Thisistheassumptionthatrulesoutcorrelationbetweentheunobservedeffectandtheexplanatoryvariables.BecausetheREtransformationdoesnotcompletelyremovethetimeaverage,wecanallowexplanatoryvariablesthatareconstantacrosstimeforalli.ASSUMPTIONRE.4Therearenoperfectlinearrelationshipsamongtheexplanatoryvariables.Wealsoneedtoimposehomoskedasticityonaiasfollows:ASSUMPTIONRE.5InadditiontoFE.5,thevarianceofaigivenallexplanatoryvariablesisconstant:2Var(aiXi)a.Underthesixrandomeffectsassumptions(FE.1,FE.2,RE.3,RE.4,RE.5,andFE.6),therandomeffectsestimatorisconsistentasNgetslargeforfixedT.(Actually,onlythefirstfourassumptionsareneededforconsistency.)TheREestimatorisnotunbiasedunlessweknow,whichkeepsupfromhavingtoestimateit.TheREesti-matorisalsoapproximatelynormallydistributedwithlargeN,andtheusualstandarderrors,tstatistics,andFstatisticsobtainedfromthequasi-demeanedregressionarevalidwithlargeN.[Formoreinformation,seeWooldridge(1999,Chapter10).]460\nd7/14/997:43PMPage461ChapterFifteenInstrumentalVariablesEstimationandTwoStageLeastSquaresnthischapter,wefurtherstudytheproblemofendogenousexplanatoryvariablesIinmultipleregressionmodels.InChapter3,wederivedthebiasintheOLSesti-matorswhenanimportantvariableisomitted;inChapter5,weshowedthatOLSisgenerallyinconsistentunderomittedvariables.Chapter9demonstratedthatomittedvariablesbiascanbeeliminated(oratleastmitigated)whenasuitableproxyvariableisgivenforanunobservedexplanatoryvariable.Unfortunately,suitableproxyvariablesarenotalwaysavailable.Intheprevioustwochapters,weexplainedhowfixedeffectsestimationorfirstdif-ferencingcanbeusedwithpaneldatatoestimatetheeffectsoftime-varyingindepen-dentvariablesinthepresenceoftime-constantomittedvariables.Whilesuchmethodsareveryuseful,wedonotalwayshaveaccesstopaneldata.Evenifwecanobtainpaneldata,itdoesuslittlegoodifweareinterestedintheeffectofavariablethatdoesnotchangeovertime:firstdifferencingorfixedeffectsestimationeliminatestime-constantvariables.Inaddition,thepaneldatamethodswhichwehavestudiedsofardonotsolvetheproblemoftime-varyingomittedvariablesthatarecorrelatedwiththeexplanatoryvariables.Inthischapter,wetakeadifferentapproachtotheendogeneityproblem.Youwillseehowthemethodofinstrumentalvariables(IV)canbeusedtosolvetheproblemofendogeneityofoneormoreexplanatoryvariables.Themethodoftwostageleastsquares(2SLSorTSLS)issecondinpopularityonlytoordinaryleastsquaresforesti-matinglinearequationsinappliedeconometrics.WebeginbyshowinghowIVmethodscanbeusedtoobtainconsistentestima-torsinthepresenceofomittedvariables.IVcanalsobeusedtosolvetheerrors-in-variablesproblem,atleastundercertainassumptions.Thenextchapterwilldemon-stratehowtoestimatesimultaneousequationsmodelsusingIVmethods.OurtreatmentofinstrumentalvariablesestimationcloselyfollowsourdevelopmentofordinaryleastsquaresinPart1,whereweassumedthatwehadarandomsamplefromanunderlyingpopulation.Thisisadesirablestartingpointbecause,inadditiontosimplifyingthenotation,itemphasizesthattheimportantassumptionsforIVestima-tionarestatedintermsoftheunderlyingpopulation(justaswithOLS).AsweshowedinPart2,OLScanbeappliedtotimeseriesdata,andthesameistrueofinstrumentalvariablesmethods.Section15.7discussessomespecialissuesthatarisewhenIVmeth-461\nd7/14/997:43PMPage462Part3AdvancedTopicsodsareappliedtotimeseriesdata.InSection15.8,wecoverapplicationstopooledcrosssectionsandpaneldata.15.1MOTIVATION:OMITTEDVARIABLESINASIMPLEREGRESSIONMODELWhenfacedwiththeprospectofomittedvariablesbias(orunobservedheterogeneity),wehavesofardiscussedthreeoptions:(1)wecanignoretheproblemandsuffertheconsequencesofbiasedandinconsistentestimators;(2)wecantrytofindanduseasuitableproxyvariablefortheunobservedvariable;(3)wecanassumethattheomittedvariabledoesnotchangeovertimeandusethefixedeffectsorfirst-differencingmeth-odsfromChapters13and14.Thefirstresponsecanbesatisfactoryiftheestimatesarecoupledwiththedirectionofthebiasesforthekeyparameters.Forexample,ifwecansaythattheestimatorofapositiveparameter,saytheeffectofjobtrainingonsubse-quentwages,isbiasedtowardzeroandwehavefoundastatisticallysignificantpositiveestimate,wehavestilllearnedsomething:jobtraininghasapositiveeffectonwages,anditislikelythatwehaveunderestimatedtheeffect.Unfortunately,theoppositecase,whereourestimatesmaybetoolargeinmagnitude,oftenoccurs,whichmakesitverydifficultforustodrawanyusefulconclusions.TheproxyvariablesolutiondiscussedinSection9.2canalsoproducesatisfyingresults,butitisnotalwayspossibletofindagoodproxy.Thisapproachattemptstosolvetheomittedvariableproblembyreplacingtheunobservablewithaproxyvariable.Anotherapproachleavestheunobservedvariableintheerrorterm,butratherthanestimatingthemodelbyOLS,itusesanestimationmethodthatrecognizesthepresenceoftheomittedvariable.Thisiswhatthemethodofinstrumentalvariablesdoes.Forillustration,considertheproblemofunobservedabilityinawageequationforworkingadults.Asimplemodelislog(wage)01educ2abile,whereeistheerrorterm.InChapter9,weshowedhow,undercertainassumptions,aproxyvariablesuchasIQcanbesubstitutedforability,andthenaconsistentestimatorisavailablefromtheregressionoflog(wage)oneduc,IQ.Suppose,however,thataproxyvariableisnotavailable(ordoesnothavetheproper-tiesneededtoproduceaconsistentestimatorof1).Then,weputabilintotheerrorterm,andweareleftwiththesimpleregressionmodellog(wage)01educu,(15.1)whereucontainsabil.Ofcourse,ifequation(15.1)isestimatedbyOLS,abiasedandinconsistentestimatorof1resultsifeducandabilarecorrelated.Itturnsoutthatwecanstilluseequation(15.1)asthebasisforestimation,providedwecanfindaninstrumentalvariableforeduc.Todescribethisapproach,thesimpleregressionmodeliswrittenas462\nd7/14/997:43PMPage463Chapter15InstrumentalVariablesEstimationandTwoStageLeastSquaresy01xu,(15.2)wherewethinkthatxanduarecorrelated:Cov(x,u)0.(15.3)Themethodofinstrumentalvariablesworkswhetherornotxanduarecorrelated,but,forreasonswewillseelater,OLSshouldbeusedifxisuncorrelatedwithu.Inordertoobtainconsistentestimatorsof0and1whenxanduarecorrelated,weneedsomeadditionalinformation.Theinformationcomesbywayofanewvariablethatsatisfiescertainproperties.Supposethatwehaveanobservablevariablezthatsat-isfiesthesetwoassumptions:(1)zisuncorrelatedwithu,thatis,Cov(z,u)0;(15.4)(2)ziscorrelatedwithx,thatis,Cov(z,x)0.(15.5)Thenwecallzaninstrumentalvariableforx.Sometimes,requirement(15.4)issummarizedbysayingthat“zisexogenousinequation(15.2).”Inthecontextofomittedvariables,thismeansthatzshouldhavenopartialeffectonyandzshouldnotbecorrelatedwithotherfactorsthataffecty.Equation(15.5)meansthatzmustberelated,eitherpositivelyornegatively,totheendogenousexplanatoryvariablex.Thereisaveryimportantdifferencebetweenthetworequirementsforaninstru-mentalvariable.Because(15.4)isacovariancebetweenzandtheunobservableerroru,itcanneverbecheckedoreventested:wemustmaintainthisassumptionbyappealingtoeconomicbehaviororagutfeeling.Bycontrast,theconditionthatziscorrelatedwithx(inthepopulation)canbetested,givenarandomsamplefromthepopulation.Theeasiestwaytodothisistoestimateasimpleregressionbetweenxandz.Inthepop-ulation,wehavex01zv.(15.6)Then,because1Cov(z,x)/Var(z),assumption(15.5)holdsifandonlyif10.Thus,weshouldbeabletorejectthenullhypothesisH0:10(15.7)againstthetwo-sidedalternativeH0:10,atasufficientlysmallsignificancelevel(say,5%or1%).Ifthisisthecase,thenwecanbefairlyconfidentthat(15.5)holds.Forthelog(wage)equationin(15.1),aninstrumentalvariablezforeducmustbe(1)uncorrelatedwithability(andanyotherunobservablefactorsaffectingwage)and(2)correlatedwitheducation.Somethingsuchasthelastdigitofanindividual’ssocial463\nd7/14/997:43PMPage464Part3AdvancedTopicssecuritynumberalmostcertainlysatisfiesthefirstrequirement:itisuncorrelatedwithabilitybecauseitisdeterminedrandomly.However,thisvariableisnotcorrelatedwitheducation,soitmakesapoorinstrumentalvariableforeduc.WhatwehavecalledaproxyvariablefortheomittedvariablemakesapoorIVfortheoppositereason.Forexample,inthelog(wage)examplewithomittedability,aproxyvariableforabilmustbeashighlycorrelatedaspossiblewithabil.Aninstru-mentalvariablemustbeuncorrelatedwithabil.Therefore,whileIQisagoodcandi-dateasaproxyvariableforabil,itisnotagoodinstrumentalvariableforeduc.Therequirementsarelessclear-cutforotherpossibleinstrumentalvariablecandi-dates.Inwageequations,laboreconomistshaveusedfamilybackgroundvariablesasIVsforeducation.Forexample,mother’seducation(motheduc)ispositivelycorrelatedwithchild’seducation,ascanbeseenbycollectingasampleofdataonworkingpeo-pleandrunningasimpleregressionofeduconmotheduc.Therefore,motheducsatis-fiesequation(15.5).Theproblemisthatmother’seducationmightalsobecorrelatedwithchild’sability(throughmother’sabilityandperhapsqualityofnurturingatanearlyage).AnotherIVchoiceforeducin(15.1)isnumberofsiblingswhilegrowingup(sibs).Typically,moresiblingsisassociatedwithloweraveragelevelsofeducation.Thus,ifnumberofsiblingsisuncorrelatedwithability,itcanactasaninstrumentalvariableforeduc.Asasecondexample,considertheproblemofestimatingthecausaleffectofskip-pingclassesonfinalexamscore.Inasimpleregressionframework,wehavescore01skippedu,(15.8)wherescoreisthefinalexamscore,andskippedisthetotalnumberoflecturesmissedduringthesemester.Wecertainlymightbeworriedthatskippediscorrelatedwithotherfactorsinu:betterstudentsmightmissfewerclasses.Thus,asimpleregressionofscoreonskippedmaynotgiveusagoodestimateofthecausaleffectofmissingclasses.WhatmightbeagoodIVforskipped?Weneedsomethingthathasnodirecteffectonscoreandisnotcorrelatedwithstudentability.Atthesametime,theIVmustbecor-relatedwithskipped.Oneoptionistousedistancebetweenlivingquartersandcampus.Somestudentsatalargeuniversitywillcommutetocampus,whichmayincreasethelikelihoodofmissinglectures(duetobadweather,oversleeping,andsoon).Thus,skippedmaybepositivelycorrelatedwithdistance;thiscanbecheckedbyregressingskippedondistanceanddoingattest,asdescribedearlier.Isdistanceuncorrelatedwithu?Inthesimpleregressionmodel(15.8),somefactorsinumaybecorrelatedwithdistance.Forexample,studentsfromlow-incomefamiliesmayliveoffcampus;ifincomeaffectsstudentperformance,thiscouldcausedistancetobecorrelatedwithu.Section15.2showshowtouseIVinthecontextofmultipleregression,sothatotherfactorsaffectingscorecanbeincludeddirectlyinthemodel.Then,distancemightbeagoodIVforskipped.AnIVapproachmaynotbenecessaryatallifagoodproxyexistsforstudentability,suchascumulativeGPApriortothesemester.Wenowdemonstratethattheavailabilityofaninstrumentalvariablecanbeusedtoconsistentlyestimatetheparametersinequation(15.2).Inparticular,weshowthat464\nd7/14/997:43PMPage465Chapter15InstrumentalVariablesEstimationandTwoStageLeastSquaresassumptions(15.4)and(15.5)[equivalently,(15.4)and(15.7)]servetoidentifytheparameter1.Identificationofaparameterinthiscontextmeansthatwecanwrite1intermsofpopulationmomentsthatcanbeestimatedusingasampleofdata.Towrite1intermsofpopulationcovariances,weuseequation(15.2):thecovariancebetweenzandyisCov(z,y)1Cov(z,x)Cov(z,u).Now,underassumption(15.4),Cov(z,u)0,andunderassumption(15.5),Cov(z,x)0.Thus,wecansolvefor1asCov(z,y)1.(15.9)Cov(z,x)[Noticehowthissimplealgebrafailsifzandxareuncorrelated,thatis,ifCov(z,x)0.]Equation(15.9)showsthat1isthepopulationcovariancebetweenzandy,dividedbythepopulationcovariancebetweenzandx,whichshowsthat1isidentified.Givenarandomsample,weestimatethepopulationquantitiesbythesampleanalogs.Aftercancelingthesamplesizesinthenumeratoranddenominator,wegettheinstrumentalvariables(IV)estimatorof1:n(ziz¯)(yiy¯)i1ˆ1n.(15.10)(ziz¯)(xix¯)i1Givenasampleofdataonx,y,andz,itissimpletoobtaintheIVestimatorin(15.10).TheIVestimatorof0issimplyˆ0y¯ˆ1x¯,whichlooksjustliketheOLSinterceptestimatorexceptthattheslopeestimator,ˆ1,isnowtheIVestimator.Itisnoaccidentthatwhenzx,weobtaintheOLSestimatorof1.Inotherwords,whenxisexogenous,itcanbeusedasitsownIV,andtheIVestimatorisidenticaltotheOLSestimator.AsimpleapplicationofthelawoflargenumbersshowsthattheIVestimatoriscon-sistentfor1:plim(ˆ1)1,providedassumptions(15.4)and(15.5)aresatisfied.Ifeitherassumptionfails,theIVestimatorsarenotconsistent(moreonthislater).OnefeatureoftheIVestimatoristhat,whenxanduareinfactcorrelated—sothatinstru-mentalvariablesestimationisactuallyneeded—itisessentiallyneverunbiased.Thismeansthat,insmallsamples,theIVestimatorcanhaveasubstantialbias,whichisonereasonwhylargesamplesarepreferred.StatisticalInferencewiththeIVEstimatorGiventhesimilarstructureoftheIVandOLSestimators,itisnotsurprisingthattheIVestimatorhasanapproximatenormaldistributioninlargesamplesizes.Toperforminferenceon1,weneedastandarderrorthatcanbeusedtocomputetstatisticsandconfidenceintervals.Theusualapproachistoimposeahomoskedasticityassumption,justasinthecaseofOLS.Now,thehomoskedasticityassumptionisstatedconditional465\nd7/14/997:43PMPage466Part3AdvancedTopicsontheinstrumentalvariable,z,nottheendogenousexplanatoryvariable,x.Alongwiththepreviousassumptionsonu,x,andz,weadd22E(uz)Var(u).(15.11)Itcanbeshownthat,under(15.4),(15.5),and(15.11),theasymptoticvarianceofˆ1is2,(15.12)22nxx,z222wherexisthepopulationvarianceofx,isthepopulationvarianceofu,andx,zisthesquareofthepopulationcorrelationbetweenxandz.Thistellsushowhighlycor-relatedxandzareinthepopulation.AswiththeOLSestimator,theasymptoticvari-anceoftheIVestimatordecreasestozeroattherateof1/n,wherenisthesamplesize.Equation(15.12)isinterestingforacoupleofreasons.First,itprovidesawaytoobtainastandarderrorfortheIVestimator.Allquantitiesin(15.12)canbeconsistently2estimatedgivenarandomsample.Toestimatex,wesimplycomputethesamplevari-2anceofxi;toestimatex,z,wecanruntheregressionofxionzitoobtaintheR-squared,22sayRx,z.Finally,toestimate,wecanusetheIVresiduals,uˆiyiˆ0ˆ1xi,i1,2,…,n,2whereˆ0andˆ1aretheIVestimates.Aconsistentestimatoroflooksjustlikethe2estimatoroffromasimpleOLSregression:n122ˆuˆi,n2i1whereitisstandardtousethedegreesoffreedomcorrection(eventhoughthishaslit-tleeffectasthesamplesizegrows).The(asymptotic)standarderrorofˆ1isthesquarerootoftheestimatedasymptoticvariance,thelatterofwhichisgivenby2ˆ,(15.13)2SSTxRx,zwhereSSTxisthetotalsumofsquaresofthexi.[RecallthatthesamplevarianceofxiisSSTx/n,andsothesamplesizescanceltogiveus(15.13).]Theresultingstandarderrorcanbeusedtoconstructeithertstatisticsforhypothesesinvolving1orconfi-denceintervalsfor1.ˆ0alsohasastandarderrorthatwedonotpresenthee.Anymod-erneconometricspackagecomputesthestandarderrorafteranyIVestimation.Beforewegiveanexample,itisusefultocomparetheasymptoticvariancesoftheIVandtheOLSestimators(whenxanduareuncorrelated).UndertheGauss-Markov2assumptions,thevarianceoftheOLSestimatoris/SSTx,whilethecomparablefor-222mulafortheIVestimatoris/(SSTxRx,z);theydifferonlyinthatRx,zappearsinthedenominatoroftheIVvariance.SinceanR-squaredisalwayslessthanone,the2SLS2varianceisalwayslargerthantheOLSvariance(whenOLSisvalid).IfRx,zissmall,2thentheIVvariancecanbemuchlargerthantheOLSvariance.Remember,Rx,zmea-466\nd7/14/997:43PMPage467Chapter15InstrumentalVariablesEstimationandTwoStageLeastSquaressuresthestrengthofthelinearrelationshipbetweenxandzinthesample.Ifxandzare2onlyslightlycorrelated,Rx,zcanbesmall,andthiscantranslateintoaverylargesam-plingvariancefortheIVestimator.Themorehighlycorrelatedziswithx,thecloser2Rx,zistoone,andthesmalleristhevarianceoftheIVestimator.Inthecasethatzx,2Rx,z1,andwegettheOLSvariance,asexpected.ThepreviousdiscussionhighlightsanimportantcostofperformingIVestimationwhenxanduareuncorrelated:theasymptoticvarianceoftheIVestimatorisalwayslarger,andsometimesmuchlarger,thantheasymptoticvarianceoftheOLSestimator.EXAMPLE15.1(EstimatingtheReturntoEducationforMarriedWomen)WeusethedataonmarriedworkingwomeninMROZ.RAWtoestimatethereturntoedu-cationinthesimpleregressionmodellog(wage)01educu.(15.14)Forcomparison,wefirstobtaintheOLSestimates:(log(wˆage).185)(.109)educlog(wageˆ)(.185)(.014)educ(15.15)2n428,R.118.Theestimatefor1impliesanalmost11%returnforanotheryearofeducation.Next,weusefather’seducation(fatheduc)asaninstrumentalvariableforeduc.Wehavetomaintainthatfatheducisuncorrelatedwithu.Thesecondrequirementisthateducandfatheducarecorrelated.Wecancheckthisveryeasilyusingasimpleregressionofeduconfatheduc(usingonlytheworkingwomeninthesample):edˆuc(10.24)(.269)fatheducedˆuc0(0.28)(.029)fatheduc(15.16)2n428,R.173.Thetstatisticonfatheducis9.28,whichindicatesthateducandfatheduchaveastatisti-callysignificantpositivecorrelation.(Infact,fatheducexplainsabout17%ofthevariationineducinthesample.)UsingfatheducasanIVforeducgives(log(wˆage).441)(.059)educlog(wageˆ)(.446)(.035)educ(15.17)2n428,R.093.TheIVestimateofthereturntoeducationis5.9%,whichisaboutone-halfoftheOLSesti-mate.ThissuggeststhattheOLSestimateistoohighandisconsistentwithomittedabilitybias.Butweshouldrememberthattheseareestimatesfromjustonesample:wecanneverknowwhether.109isabovethetruereturntoeducation,orwhether.059isclosertothe467\nd7/14/997:43PMPage468Part3AdvancedTopicstruereturntoeducation.Further,thestandarderroroftheIVestimateistwoandone-halftimesaslargeastheOLSstandarderror(thisisexpected,forthereasonswegaveearlier).The95%confidenceintervalfor1usingOLSismuchtighterthanthatusingtheIV;infact,theIVconfidenceintervalactuallycontainstheOLSestimate.Therefore,whilethediffer-encesbetween(15.15)and(15.17)arepracticallylarge,wecannotsaywhetherthediffer-enceisstatisticallysignificant.WewillshowhowtotestthisinSection15.5.Inthepreviousexample,theestimatedreturntoeducationusingIVwaslessthanthatusingOLS,whichcorrespondstoourexpectations.Butthisneednothavebeenthecase,asthefollowingexampledemonstrates.EXAMPLE15.2(EstimatingtheReturntoEducationforMen)WenowuseWAGE2.RAWtoestimatethereturntoeducationformen.Weusethevari-ablesibs(numberofsiblings)asaninstrumentforeduc.Thesearenegativelycorrelated,aswecanverifyfromasimpleregression:educˆ(14.14)(.228)sibseduc0(0.11)(.030)sibs2n935,R.057.Thisequationimpliesthateverysiblingisassociatedwith,onaverage,about.23lessofayearofeducation.Ifweassumethatsibsisuncorrelatedwiththeerrortermin(15.14),thentheIVestimatorisconsistent.Estimatingequation(15.14)usingsibsasanIVforeducgiveslog(wˆage)(5.13)(.122)educlog(wˆage)(0.36)(.026)educn935.(TheR-squarediscomputedtobenegative,sowedonotreportit.AdiscussionofR-squaredinthecontextofIVestimationfollows.)Forcomparison,theOLSestimateof1is.059withastandarderrorof.006.Unlikeinthepreviousexample,theIVestimateisnowmuchhigherthantheOLSestimate.Whilewedonotknowwhetherthedifferenceissta-tisticallysignificant,thisdoesnotmeshwiththeomittedabilitybiasfromOLS.Itcouldbethatsibsisalsocorrelatedwithability:moresiblingsmeans,onaverage,lessparentalatten-tion,whichcouldresultinlowerability.AnotherinterpretationisthattheOLSestimatorisbiasedtowardzerobecauseofmeasurementerrorineduc.Thisisnotentirelyconvinc-ingbecause,aswediscussedinSection9.3,educisunlikelytosatisfytheclassicalerrors-in-variablesmodel.Inthepreviousexamples,theendogenousexplanatoryvariable(educ)andtheinstrumentalvariables(fatheduc,sibs)hadquantitativemeaning.Butbothtypescanbebinaryvariables.AngristandKrueger(1991),intheirsimplestanalysis,cameup468\nd7/14/997:43PMPage469Chapter15InstrumentalVariablesEstimationandTwoStageLeastSquareswithacleverbinaryinstrumentalvariableforeduc,usingcensusdataonmenintheUnitedStates.Letfrstqrtbeequaltooneifthemanwasborninthefirstquarteroftheyear,andzerootherwise.Itseemsthattheerrortermin(15.14)—and,inparticular,ability—shouldbeunrelatedtoquarterofbirth.Butfrstqrtalsoneedstobecorrelatedwitheduc.Itturnsoutthatyearsofeducationdodiffersystematicallyinthepopula-tionbasedonquarterofbirth.AngristandKruegerarguedpursuasivelythatthisisduetocompulsoryschoolattendancelawsineffectinallstates.Briefly,studentsbornearlyintheyeartypicallybeginschoolatanolderage.Therefore,theyreachthecom-pulsoryschoolingage(16inmoststates)withsomewhatlesseducationthanstudentswhobeginschoolatayoungerage.Forstudentswhofinishhighschool,AngristandKruegerverifiedthatthereisnorelationshipbetweenyearsofeducationandquarterofbirth.Becauseyearsofeducationvariesonlyslightlyacrossquarterofbirth—which2meansRx,zin(15.13)isverysmall—AngristandKruegerneededaverylargesamplesizetogetareasonablypreciseIVestimate.Using247,199menbornbetween1920and1929,theOLSestimateofthereturntoeducationwas.0801(standarderror.0004),andtheIVestimatewas.0715(.0219);thesearereportedinTableIIIofAngristandKrueger’spaper.NotehowlargethetstatisticisfortheOLSestimate(about200),whereasthetstatisticfortheIVestimateisonly3.26.Thus,theIVestimateisstatisti-callydifferentfromzero,butitsconfidenceintervalismuchwiderthanthatbasedontheOLSestimate.AninterestingfindingbyAngristandKruegeristhattheIVestimatedoesnotdif-fermuchfromtheOLSestimate.Infact,usingmenborninthenextdecade,theIVesti-mateissomewhathigherthantheOLSestimate.OnecouldinterpretthisasshowingthatthereisnoomittedabilitybiaswhenwageequationsareestimatedbyOLS.However,theAngristandKruegerpaperhasbeencriticizedoneconometricgrounds.AsdiscussedbyBound,Jaeger,andBaker(1995),itisnotobviousthatseasonofbirthisunrelatedtounobservedfactorsthataffectwage.Aswewillexplaininthenextsub-section,evenasmallamountofcorrelationbetweenzanducancauseseriousproblemsfortheIVestimator.Forpolicyanalysis,theendogenousexplanatoryvariableisoftenabinaryvariable.Forexample,Angrist(1990)studiedtheeffectthatbeingaveteranintheVietnamwarhadonlifetimeearnings.Asimplemodelislog(earns)01veteranu,(15.18)whereveteranisabinaryvariable.TheproblemwithestimatingthisequationbyOLSisthattheremaybeaself-selectionproblem,aswementionedinChapter7:perhapspeoplewhogetthemostoutofthemilitarychoosetojoin,orthedecisiontojoiniscor-relatedwithothercharacteristicsthataffectearnings.Thesewillcauseveteranandutobecorrelated.AngristpointedoutthattheVietnamdraftlotteryprovidedanaturalexperiment(seealsoChapter13)thatcreatedaninstrumentalvariableforveteran.YoungmenweregivenlotterynumbersthatdeterminedwhethertheywouldbecalledtoserveinVietnam.Sincethenumbersgivenwere(eventually)randomlyassigned,itseemsplausiblethatdraftlotterynumberisuncorrelatedwiththeerrortermu.Butthose469\nd7/14/997:43PMPage470Part3AdvancedTopicswithalowenoughnumberhadtoserveinQUESTION15.1Vietnam,sothattheprobabilityofbeingIfsomemenwhowereassignedlowdraftlotterynumbersobtainedaveteraniscorrelatedwithlotterynum-additionalschoolingtoreducetheprobabilityofbeingdrafted,isber.Ifbothofthesearetrue,draftlot-lotterynumberagoodinstrumentforveteranin(15.18)?terynumberisagoodIVcandidateforveteran.Itisalsopossibletohaveabinaryendogenousexplanatoryvariableandabinaryinstrumentalvariable.SeeProblem15.1foranexample.PropertiesofIVwithaPoorInstrumentalVariableWehavealreadyseenthat,whileIVisconsistentwhenzanduareuncorrelatedandzandxhaveanypositiveornegativecorrelation,IVestimatescanhavelargestandarderrors,especiallyifzandxareonlyweaklycorrelated.Weakcorrelationbetweenzandxcanhaveevenmoreseriousconsequences:theIVestimatorcanhavealargeasymp-toticbiasevenifzanduareonlymoderatelycorrelated.WecanseethisbystudyingtheprobabilitylimitoftheIVestimatorwhenzanduarepossiblycorrelated.ThiscanbederivedintermsofpopulationcorrelationsandstandarddeviationsasCorr(z,u)uplimˆ11,(15.19)Corr(z,x)xwhereuandxarethestandarddeviationsofuandxinthepopulation,respectively.Theinterestingpartofthisequationinvolvesthecorrelationterms.Itshowsthat,evenifCorr(z,u)issmall,theinconsistencyintheIVestimatorcanbeverylargeifCorr(z,x)isalsosmall.Thus,evenifwefocusonlyonconsistency,itisnotnecessarilybettertouseIVthanOLSifthecorrelationbetweenzanduissmallerthanthatbetweenxandu.UsingthefactthatCorr(x,u)Cov(x,u)/(xu)alongwithequation(5.3),wecanwritetheplimoftheOLSestimator—callit˜1—asuplim˜11Corr(x,u).(15.20)xComparingtheseformulasshowsthatIVispreferredtoOLSonasymptoticbiasgroundswhenCorr(z,u)/Corr(z,x)Corr(x,u).IntheAngristandKrueger(1991)examplementionedearlier,wherexisyearsofschoolingandzisabinaryvariableindicatingquarterofbirth,thecorrelationbetweenzandxisverysmall.Bound,Jaeger,andBaker(1995)discussedreasonswhyquarterofbirthandumightbesomewhatcorrelated.Fromequation(15.19),weseethatthiscanleadtoasubstantialbiasintheIVestimator.Whenzandxarenotcorrelatedatall,thingsareespeciallybad,whetherornotzisuncorrelatedwithu.ThefollowingexampleillustrateswhyweshouldalwayschecktoseeiftheendogenousexplanatoryvariableiscorrelatedwiththeIVcandidate.470\nd7/14/997:43PMPage471Chapter15InstrumentalVariablesEstimationandTwoStageLeastSquaresEXAMPLE15.3(EstimatingtheEffectofSmokingonBirthWeight)InChapter6,weestimatedtheeffectofcigarettesmokingonchildbirthweight.Withoutotherexplanatoryvariables,themodelislog(bwght)01packsu,(15.21)wherepacksisthenumberofpackssmokedbythemotherperday.Wemightworrythatpacksiscorrelatedwithotherhealthfactorsortheavailabilityofgoodprenatalcare,sothatpacksandumightbecorrelated.Apossibleinstrumentalvariableforpacksistheaveragepriceofcigarettesinthestateofresidence,cigprice.Wewillassumethatcigpriceanduareuncorrelated(eventhoughstatesupportforhealthcarecouldbecorrelatedwithcigarettetaxes).Ifcigarettesareatypicalconsumptiongood,basiceconomictheorysuggeststhatpacksandcigpricearenegativelycorrelated,sothatcigpricecanbeusedasanIVforpacks.Tocheckthis,weregresspacksoncigprice,usingthedatainBWGHT.RAW:pacˆks(.067)(.0003)cigpricepacˆks(.103)(.0008)cigprice22n1,388,R.0000,R¯.0006.Thisindicatesnorelationshipbetweensmokingduringpregnancyandcigaretteprices,whichisperhapsnottoosurprisinggiventheaddictivenatureofcigarettesmoking.Becausepacksandcigpricearenotcorrelated,weshouldnotusecigpriceasanIVforpacksin(15.21).Butwhathappensifwedo?TheIVresultswouldbelog(bwˆght)(4.45)(2.99)packslog(bwˆght)(0.91)(8.70)packsn1,388(thereportedR-squaredisnegative).Thecoefficientonpacksishugeandofanunexpectedsign.Thestandarderrorisalsoverylarge,sopacksisnotsignificant.ButtheestimatesaremeaninglessbecausecigpricefailstheonerequirementofanIVthatwecanalwaystest:assumption(15.5).ComputingR-SquaredAfterIVEstimationMostregressionpackagescomputeanR-squaredafterIVestimation,usingthestandard2formula:R1SSR/SST,whereSSRisthesumofsquaredIVresiduals,andSSTisthetotalsumofsquaresofy.UnlikeinthecaseofOLS,theR-squaredfromIVesti-mationcanbenegativebecauseSSRforIVcanactuallybelargerthanSST.AlthoughitdoesnotreallyhurttoreporttheR-squaredforIVestimation,itisnotveryuseful,either.Whenxanduarecorrelated,wecannotdecomposethevarianceofyinto21Var(x)Var(u),andsotheR-squaredhasnonaturalinterpretation.Inaddition,as471\nd7/14/997:43PMPage472Part3AdvancedTopicswewilldiscussinSection15.3,theseR-squaredscannotbeusedintheusualwaytocomputeFtestsofjointrestrictions.IfourgoalwastoproducethelargestR-squared,wewouldalwaysuseOLS.IVmethodsareintendedtoprovidebetterestimatesoftheceterisparibuseffectofxonywhenxanduarecorrelated;goodness-of-fitisnotafactor.AhighR-squaredresultingfromOLSisoflittlecomfortifwecannotconsistentlyestimate1.15.2IVESTIMATIONOFTHEMULTIPLEREGRESSIONMODELTheIVestimatorforthesimpleregressionmodeliseasilyextendedtothemultipleregressioncase.Webeginwiththecasewhereonlyoneoftheexplanatoryvariablesiscorrelatedwiththeerror.Infact,considerastandardlinearmodelwithtwoexplanatoryvariables:y101y22z1u1.(15.22)Wecallthisastructuralequationtoemphasizethatweareinterestedinthej,whichsimplymeansthattheequationissupposedtomeasureacausalrelationship.Weuseanewnotationheretodistinguishendogenousfromexogenousvariables.Thedepen-dentvariabley1isclearlyendogenous,asitiscorrelatedwithu1.Thevariablesy2andz1aretheexplanatoryvariables,andu1istheerror.Asusual,weassumethattheexpectedvalueofu1iszero:E(u1)0.Weusez1toindicatethatthisvariableisexoge-nousin(15.22)(z1isuncorrelatedwithu1).Weusey2toindicatethatthisvariableissuspectedofbeingcorrelatedwithu1.Wedonotspecifywhyy2andu1arecorrelated,butfornowitisbesttothinkofu1ascontaininganomittedvariablecorrelatedwithy2.Thenotationinequation(15.22)originatesinsimultaneousequationsmodels(whichwecoverinChapter16),butweuseitmoregenerallytoeasilydistinguishexogenousfromendogenousvariablesinamultipleregressionmodel.Anexampleof(15.22)islog(wage)01educ2experu1,(15.23)wherey1log(wage),y2educ,andz1exper.Inotherwords,weassumethatexperisexogenousin(15.23),butweallowthateduc—fortheusualreasons—iscorrelatedwithu1.Weknowthatif(15.22)isestimatedbyOLS,alloftheestimatorswillbebiasedandinconsistent.Thus,wefollowthestrategysuggestedintheprevioussectionandseekaninstrumentalvariablefory2.Sincez1isassumedtobeuncorrelatedwithu1,canweusez1asaninstrumentfory2,assumingy2andz1arecorrelated?Theanswerisno.Sincez1itselfappearsasanexplanatoryvariablein(15.22),itcannotserveasaninstru-mentalvariablefory2.Weneedanotherexogenousvariable—callitz2—thatdoesnotappearin(15.22).Therefore,keyassumptionsarethatz1andz2areuncorrelatedwithu1;wealsoassumethatu1haszeroexpectedvalue,whichiswithoutlossofgeneralitywhentheequationcontainsanintercept:472\nd7/14/997:43PMPage473Chapter15InstrumentalVariablesEstimationandTwoStageLeastSquaresE(u1)0,Cov(z1,u1)0,andCov(z2,u1)0.(15.24)Giventhezeromeanassumption,thelattertwoassumptionsareequivalenttoE(z1u1)E(z2u1)0,andsothemethodofmomentsapproachsuggestsobtainingestimatorsˆ0,ˆ1,andˆ2bysolvingthesamplecounterpartsof(15.24):n(yi1ˆ0ˆ1yi2ˆ2zi1)0i1nzi1(yi1ˆ0ˆ1yi2ˆ2zi1)0(15.25)i1nzi2(yi1ˆ0ˆ1yi2ˆ2zi1)0.i1Thisisasetofthreelinearequationsinthethreeunknownsˆ0,ˆ1,andˆ2,anditiseas-ilysolvedgiventhedataony1,y2,z1,andz2.Theestimatorsarecalledinstrumentalvariablesestimators.Ifwethinky2isexogenousandwechoosez2y2,equations(15.25)areexactlythefirstorderconditionsfortheOLSestimators;seeequations(3.13).Westillneedtheinstrumentalvariablez2tobecorrelatedwithy2,butthesenseinwhichthesetwovariablesmustbecorrelatediscomplicatedbythepresenceofz1inequation(15.22).Wenowneedtostatetheassumptionintermsofpartialcorrelation.Theeasiestwaytostatetheconditionistowritetheendogenousexplanatoryvariableasalinearfunctionoftheexogenousvariablesandanerrorterm:y201z12z2v2,(15.26)where,bydefinition,E(v2)0,Cov(z1,v2)0,andCov(z2,v2)0,andthejareunknownparameters.Thekeyidentificationcondition[alongwith(15.24)]isthatQUESTION15.220.(15.27)Supposewewishtoestimatetheeffectofmarijuanausageoncol-legegradepointaverage.Forthepopulationofcollegeseniorsatauniversity,letdaysuseddenotethenumberofdaysinthepastInotherwords,afterpartiallingoutz1,y2monthonwhichastudentsmokedmarijuanaandconsidertheandz2arestillcorrelated.Thiscorrelationstructuralequationcanbepositiveornegative,butitcannotbezero.Testing(15.27)iseasy:weestimatecolGPA01daysused2SATu.(15.26)byOLSanduseattest(possibly(i)LetpercHSdenotethepercentofastudent’shighschoolmakingitrobusttoheteroskedasticity).Wegraduatingclassthatreportedregularuseofmarijuana.Ifthisisanshouldalwaystestthisassumption.Un-IVcandidatefordaysused,writethereducedformfordaysused.Doyouthink(15.27)islikelytobetrue?fortunately,wecannottestthatz1andz2(ii)DoyouthinkpercHSistrulyexogenousinthestructuralareuncorrelatedwithu1;thismustbetakenequation?Whatproblemsmighttherebe?onfaith.473\nd7/14/997:43PMPage474Part3AdvancedTopicsEquation(15.26)isanexampleofareducedformequation,whichmeansthatwehavewrittenanendogenousvariableintermsofexogenousvariables.Thisnamecomesfromsimultaneousequationsmodels—whichwestudyinthenextchapter—butitisausefulconceptwheneverwehaveanendogenousexplanatoryvariable.Thenamehelpsdistinguishitfromthestructuralequation(15.22).Addingmoreexogenousexplanatoryvariablestothemodelisstraightforward.Writethestructuralmodelasy101y22z1…kzk1u1,(15.28)wherey2isthoughttobecorrelatedwithu1.Letzkbeavariablenotin(15.28)thatisalsoexogenous.Therefore,weassumethatE(u1)0,Cov(zj,u1)0,j1,…,k.(15.29)Thereducedformfory2isy201z1…k1zk1kzkv2,(15.30)andweneedsomepartialcorrelationbetweenzkandy2:k0.(15.31)Under(15.29)and(15.31),zkisavalidIVfory2.(Wedonotcareabouttheremainingj;someorallofthemcouldbezero.)Itmakessensetothinkthatz1,…,zk1serveastheirownIVs;therefore,thelistofexogenousvariablesisoftencalledthelistofinstrumentalvariables.Aminoradditionalassumptionisthattherearenoperfectlinearrelationshipsamongtheexogenousvariables;thisisanalogoustotheassumptionofnoperfectcollinearityinthecontextofOLS.Forstandardstatisticalinference,weneedtoassumehomoskedasticityofu1.WegiveacarefulstatementoftheseassumptionsinamoregeneralsettinginSection15.3.EXAMPLE15.4(UsingCollegeProximityasanIVforEducation)Card(1995)usedwageandeducationdataforasampleofmenin1976toestimatethereturntoeducation.Heusedadummyvariableforwhethersomeonegrewupnearafour-yearcollege(nearc4)asaninstrumentalvariableforeducation.Inalog(wage)equation,heincludedotherstandardcontrols:experience,ablackdummyvariable,dummyvariablesforlivinginanSMSAandlivinginthesouth,andafullsetofregionaldummyvariablesandanSMSAdummyforwherethemanwaslivingin1966.Inorderfornearc4tobeavalidinstru-ment,itmustbeuncorrelatedwiththeerrorterminthewageequation—weassumethis—anditmustbepartiallycorrelatedwitheduc.Tocheckthelatterrequirement,weregresseduconnearc4andalloftheexogenousvariablesappearingintheequation.(Thatis,weestimatethereducedformforeduc.)UsingthedatainCARD.RAW,weobtain,incon-densedform,474\nd7/14/997:43PMPage475Chapter15InstrumentalVariablesEstimationandTwoStageLeastSquaresedˆuc(16.64)(.320)nearc4(.413)exper…edˆuc0(0.24)(.088)nearc4(.034)exper…(15.32)2n3,010,R.477.Weareinterestedinthecoefficientandtstatisticonnearc4.Thecoefficientimpliesthatin1976,otherthingsbeingfixed(experience,race,region,andsoon),peoplewholivednearacollegein1966had,onaverage,aboutone-thirdofayearmoreeducationthanthosewhodidnotgrowupnearacollege.Thetstatisticonnearc4is3.64,whichgivesap-valuethatiszerointhefirstthreedecimals.Therefore,ifnearc4isuncorrelatedwithunobservedfactorsintheerrorterm,wecanusenearc4asanIVforeduc.TheOLSandIVestimatesaregiveninTable15.1.Interestingly,theIVestimateofthereturntoeducationisalmosttwiceaslargeastheOLSestimate,butthestandarderroroftheIVestimateisover18timeslargerthantheOLSstandarderror.The95%confidenceintervalfortheIVestimateisfrom.024and.239,whichisaverywiderange.Largercon-Table15.1DependentVariable:log(wage)ExplanatoryVariablesOLSIVeduc.075.132(.003)(.055)exper.085.108(.007)(.024)2exper.0023.0023(.0003)(.0003)black.199.147(.018)(.054)smsa.136.112(.020)(.032)south.148.145(.026)(.027)Observations3,0103,010R-squared.300.238Othercontrols:smsa66,reg662,…,reg669475\nd7/14/997:43PMPage476Part3AdvancedTopicsfidenceintervalsisapricewemustpaytogetaconsistentestimatorofthereturntoedu-cationwhenwethinkeducisendogenous.Asdiscussedearlier,weshouldnotmakeanythingofthesmallerR-squaredintheIVestimation:bydefinition,theOLSR-squaredwillalwaysbelargerbecauseOLSminimizesthesumofsquaredresiduals.15.3TWOSTAGELEASTSQUARESIntheprevioussection,weassumedthatwehadasingleendogenousexplanatoryvari-able(y2),alongwithoneinstrumentalvariablefory2.Itoftenhappensthatwehavemorethanoneexogenousvariablethatisexcludedfromthestructuralmodelandmightbecorrelatedwithy2,whichmeanstheyarevalidIVsfory2.Inthissection,wediscusshowtousemultipleinstrumentalvariables.ASingleEndogenousExplanatoryVariableConsideragainthestructuralmodel(15.22),whichhasoneendogenousandoneexoge-nousexplanatoryvariable.Supposenowthatwehavetwoexogenousvariablesexcludedfrom(15.22):z2andz3.Ourassumptionsthatz2andz3donotappearin(15.22)andareuncorrelatedwiththeerroru1areknownasexclusionrestrictions.Ifz2andz3arebothcorrelatedwithy2,wecouldjustuseeachasanIV,asintheprevioussection.ButthenwewouldhavetwoIVestimators,andneitherofthesewould,ingeneral,beefficient.Sinceeachofz1,z2,andz3isuncorrelatedwithu1,anylinearcombinationisalsouncorrelatedwithu1,andthereforeanylinearcombinationoftheexogenousvariablesisavalidIV.TofindthebestIV,wechoosethelinearcombi-nationthatismosthighlycorrelatedwithy2.Thisturnsouttobegivenbythereducedformequationfory2.Writey201z12z23z3v2,(15.33)whereE(v2)0,Cov(z1,v2)0,Cov(z2,v2)0,andCov(z3,v2)0.ThenthebestIVfory2(undertheassumptionsgiveninthechapterappendix)isthelin-earcombinationofthezjin(15.33),whichwecally*2:y*201z12z23z3.(15.34)ForthisIVnottobeperfectlycorrelatedwithz1weneedatleastoneof2or3tobedifferentfromzero:20or30.(15.35)Thisisthekeyidentificationassumption,onceweassumethezjareallexogenous.(Thevalueof1isirrelevant.)Thestructuralequation(15.22)isnotidentifiedif20and30.WecantestH0:20and30against(15.35)usinganFstatistic.476\nd7/14/997:43PMPage477Chapter15InstrumentalVariablesEstimationandTwoStageLeastSquaresAusefulwaytothinkof(15.33)isthatitbreaksy2intotwopieces.Thefirstisy*2;thisisthepartofy2thatisuncorrelatedwiththeerrorterm,u1.Thesecondpieceisv2,andthispartispossiblycorrelatedwithu1—whichiswhyy2ispossiblyendogenous.Givendataonthezj,wecancomputey*2foreachobservation,providedweknowthepopulationparametersj.Thisisnevertrueinpractice.Nevertheless,aswesawintheprevioussection,wecanalwaysestimatethereducedformbyOLS.Thus,usingthesample,weregressy2onz1,z2,andz3andobtainthefittedvalues:yˆ2ˆ0ˆ1z1ˆ2z2ˆ3z3(15.36)(thatis,wehaveyˆi2foreachi).Atthispoint,weshouldverifythatz2andz3arejointlysignificantin(15.33)atareasonablysmallsignificancelevel(nolargerthan5%).Ifz2andz3arenotjointlysignificantin(15.33),thenwearewastingourtimewithIVesti-mation.Oncewehaveyˆ2,wecanuseitastheIVfory2.Thethreeequationsforestimating0,1,and2arethefirsttwoequationsof(15.25),withthethirdreplacedbynyˆi2(yi1ˆ0ˆ1yi2ˆ2zi1)0.(15.37)i1SolvingthethreeequationsinthreeunknownsgivesustheIVestimators.Withmultipleinstruments,theIVestimatorisalsocalledthetwostageleastsquares(2SLS)estimator.Thereasonissimple.UsingthealgebraofOLS,itcanbeshownthatwhenweuseyˆ2astheIVfory2,theIVestimatesˆ0,ˆ1,andˆ2areidenti-caltotheOLSestimatesfromtheregressionofy1onyˆ2andz1.(15.38)Inotherwords,wecanobtainthe2SLSestimatorintwostages.Thefirststageistoruntheregressionin(15.36),whereweobtainthefittedvaluesyˆ2.ThesecondstageistheOLSregression(15.38).Becauseweuseyˆ2inplaceofy2,the2SLSestimatescandif-fersubstantiallyfromtheOLSestimates.Someeconomistsliketointerprettheregressionin(15.38)asfollows.Thefittedvalue,yˆ2,istheestimatedversionofy*2,andy*2isuncorrelatedwithu1.Therefore,2SLSfirst“purges”y2ofitscorrelationwithu1beforedoingtheOLSregression(15.38).Thisisfoundtobetruebypluggingy2y*2v2into(15.22):y101y*22z1u11v2.(15.39)Now,thecompositeerroru11v2haszeromeanandisuncorrelatedwithy*2andz1,whichiswhytheOLSregressionin(15.38)works.Mosteconometricspackageshavespecialcommandsfor2SLS,sothereisnoneedtoperformthetwostagesexplicitly.Infact,inmostcases,youshouldavoiddoingthesecondstagemanually,asthestandarderrorsandteststatisticsobtainedinthiswayarenotvalid.[Thereasonisthattheerrortermin(15.39)includesv2,butthestandarderrorsinvolvethevarianceofu1only.]Anyregressionsoftwarethatsupports2SLSasks477\nd7/14/997:43PMPage478Part3AdvancedTopicsforthedependentvariable,thelistofexplanatoryvariables(bothexogenousandendogenous),andtheentirelistofinstrumentalvariables(thatis,allexogenousvari-ables).TheoutputistypicallyquitesimilartothatforOLS.Inmodel(15.28)withasingleIVfory2,theIVestimatorfromSection15.2isiden-ticaltothe2SLSestimator.Therefore,whenwehaveoneIVforeachendogenousexplanatoryvariable,wecancalltheestimationmethodIVor2SLS.Addingmoreexogenousvariableschangesverylittle.Forexample,supposethewageequationis2log(wage)01educ2exper3experu1,(15.40)2whereu1isuncorrelatedwithbothexperandexper.Supposethatwealsothinkmotherandfather’seducationsareuncorrelatedwithu1.ThenwecanusebothoftheseasIVsforeduc.Thereducedformequationforeducis2educ01exper2exper3motheduc4fatheducv2,(15.41)andidentificationrequiresthat30or40(orboth,ofcourse).EXAMPLE15.5(ReturntoEducationforWorkingWomen)Weestimateequation(15.40)usingthedatainMROZ.RAW.First,wetestH0:30,40in(15.41)usinganFtest.TheresultisF55.40,andp-value.0000.Asexpected,educis(partially)correlatedwithparents’education.Whenweestimate(15.40)by2SLS,weobtain,inequationform,2logˆ(wage)(.048)(.061)educ(.044)exper(.0009)exper2logˆ(wage)(.400)(.031)educ(.013)exper(.0004)exper2n428,R.136.Theestimatedreturntoeducationisabout6.1%,comparedwithanOLSestimateofabout10.8%.Becauseofitsrelativelylargestandarderror,the2SLSestimateisbarelysignificantatthe5%levelagainstatwo-sidedalternative.Theassumptionsneededfor2SLStohavethedesiredlargesamplepropertiesaregiveninthechapterappendix,butitisusefultobrieflysummarizethemhere.Ifwewritethestructuralequationasin(15.28),y101y22z1…kzk1u1,(15.42)thenweassumeeachzjtobeuncorrelatedwithu1.Inaddition,weneedatleastoneexogenousvariablenotin(15.42)thatispartiallycorrelatedwithy2.Thisensurescon-sistency.Fortheusual2SLSstandarderrorsandtstatisticstobeasymptoticallyvalid,wealsoneedahomoskedasticityassumption:thevarianceofthestructuralerror,u1,478\nd7/14/997:43PMPage479Chapter15InstrumentalVariablesEstimationandTwoStageLeastSquarescannotdependonanyoftheexogenousvariables.Fortimeseriesapplications,weneedmoreassumptions,aswewillseeinSection15.7.Multicollinearityand2SLSInChapter3,weintroducedtheproblemofmulticollinearityandshowedhowcorrela-tionamongregressorscanleadtolargestandarderrorsfortheOLSestimates.Multicollinearitycanbeevenmoreseriouswith2SLS.Toseewhy,the(asymptotic)varianceofthe2SLSestimatorof1canbeapproximatedas2,(15.43)2SST2(1R2)22whereVar(u1),SST2isthetotalvariationinyˆ2,andR2istheR-squaredfromaregressionofyˆ2onallotherexogenousvariablesappearinginthestructuralequation.Therearetworeasonswhythevarianceofthe2SLSislargerthanthatforOLS.First,yˆ2,byconstruction,haslessvariationthany2.(Remember:totalsumofsquaresexplainedsumofsquaresresidualsumofsquares;thevariationiny2isthetotalsumofsquares,whilethevariationinyˆ2istheexplainedsumofsquares.)Second,thecor-relationbetweenyˆ2andtheexogenousvariablesin(15.42)isoftenmuchhigherthanthecorrelationbetweeny2andthesevariables.Thisessentiallydefinesthemulti-collinearityproblemin2SLS.Asanillustration,considerExample15.4.Wheneducisregressedontheexogenous2variablesinTable15.1,R.475;thisisamoderatedegreeofmulticollinearity,buttheimportantthingisthattheOLSstandarderroronˆeducisquitesmall.Whenweobtainthefirststagefittedvalues,edˆuc,andregresstheseontheexogenousvariablesinTable215.1,R.995,whichindicatesaveryhighdegreeofmulticollinearitybetweenedˆucandtheremainingexogenousvariablesinthetable.(ThishighR-squaredisnottoosur-prisingbecauseedˆucisafunctionofalltheexogenousvariablesinTable15.1,plus2nearc4.)Equation(15.43)showsthatanR2closetoonecanresultinaverylargestan-darderrorforthe2SLSestimator.ButaswithOLS,alargesamplesizecanhelpoffset2alargeR2.MultipleEndogenousExplanatoryVariablesTwostageleastsquarescanalsobeusedinmodelswithmorethanoneendogenousexplanatoryvariable.Forexample,considerthemodely101y22y33z14z25z3u1,(15.44)whereE(u1)0,andu1isuncorrelatedwithz1,z2,andz3.Thevariablesy2andy3areendogenousexplanatoryvariables:eachmaybecorrelatedwithu1.Toestimate(15.44)by2SLS,weneedatleasttwoexogenousvariablesthatdonotappearin(15.44)butthatarecorrelatedwithy2andy3.Supposewehavetwoexcludedexogenousvariables,sayz4andz5.Then,fromouranalysisofasingleendogenousexplanatoryvariable,weneedeitherz4orz5toappearinthereducedformsofy2andy3.(Asbefore,wecanuseFstatisticstotestthis.)Whilethisisnecessaryforidentifi-479\nd7/14/997:43PMPage480Part3AdvancedTopicscation,unfortunately,itisnotsufficient.Supposethatz4appearsineachreducedform,butz5appearsinneither.Then,wedonotreallyhavetwoexogenousvariablespartiallycorrelatedwithy2andy3.Twostageleastsquareswillnotproduceconsistentestima-torsofthej.Generally,whenwehavemorethanoneendogenousexplanatoryvariableinaregressionmodel,identificationcanfailinseveralcomplicatedways.Butwecaneas-ilystateanecessaryconditionforidentification,whichiscalledtheordercondition.ORDERCONDITIONFORIDENTIFICATIONOFANEQUATION:Weneedatleastasmanyexcludedexogenousvariablesasthereareincludedendogenousexplanatoryvari-ablesinthestructuralequation.Theorderconditionissimpletocheck,asitonlyQUESTION15.3involvescountingendogenousandexoge-Thefollowingmodelexplainsviolentcrimerates,atthecitylevel,innousvariables.Thesufficientconditionfortermsofabinaryvariableforwhetherguncontrollawsexistandidentificationiscalledtherankcondition.othercontrols:Wehaveseenspecialcasesoftherankviolent01guncontrol2unem3populconditionbefore—forexample,inthedis-4percblck5age18_21….cussionsurroundingequation(15.35).ASomeresearchershaveestimatedsimilarequationsusingvariablesgeneralstatementoftherankconditionsuchasthenumberofNationalRifleAssociationmembersinthecityrequiresmatrixalgebraandisbeyondtheandthenumberofsubscriberstogunmagazinesasinstrumentalscopeofthistext.[SeeWooldridge(1999,variablesforguncontrol[see,forexample,KleckandPattersonChapter5).](1993)].Aretheseconvincinginstruments?TestingMultipleHypothesesAfter2SLSEstimationWemustbecarefulwhentestingmultiplehypothesesinamodelestimatedby2SLS.ItistemptingtouseeitherthesumofsquaredresidualsortheR-squaredformoftheFstatistic,aswelearnedwithOLSinChapter4.ThefactthattheR-squaredin2SLScanbenegativesuggeststhattheusualwayofcomputingFstatisticsmightnotbeappro-priate;thisisthecase.Infact,ifweusethe2SLSresidualstocomputetheSSRsforboththerestrictedandunrestrictedmodels,thereisnoguaranteethatSSRrSSRur;ifthereverseistrue,theFstatisticwouldbenegative.Itispossibletocombinethesumofsquaredresidualsfromthesecondstageregres-sion[suchas(15.38)]withSSRurtoobtainastatisticwithanapproximateFdistribu-tioninlargesamples.Becausemanyeconometricspackageshavesimple-to-usetestcommandsthatcanbeusedtotestmultiplehypothesesafter2SLSestimation,weomitthedetails.DavidsonandMacKinnon(1993)andWooldridge(1999,Chapter5)con-taindiscussionsofhowtocomputeF-typestatisticsfor2SLS.15.4IVSOLUTIONSTOERRORS-IN-VARIABLESPROBLEMSIntheprevioussections,wepresentedtheuseofinstrumentalvariablesasawaytosolvetheomittedvariablesproblem,buttheycanalsobeusedtodealwiththemea-surementerrorproblem.Asanillustration,considerthemodel480\nd7/14/997:43PMPage481Chapter15InstrumentalVariablesEstimationandTwoStageLeastSquaresy01x*12x2u,(15.45)whereyandx2areobservedbutx*1isnot.Letx1beanobservedmeasurementofx*1:x1x*1e1,wheree1isthemeasurementerror.InChapter9,weshowedthatcorrelationbetweenx1ande1causesOLS,wherex1isusedinplaceofx*1,tobebiasedandincon-sistent.Wecanseethisbywritingy01x12x2(u1e1).(15.46)Iftheclassicalerrors-in-variables(CEV)assumptionshold,thebiasintheOLSesti-matorof1istowardszero.Withoutfurtherassumptions,wecandonothingaboutthis.Insomecases,wecanuseanIVproceduretosolvethemeasurementerrorproblem.In(15.45),weassumethatuisuncorrelatedwithx*1,x1,andx2;intheCEVcase,weassumethate1isuncorrelatedwithx*1andx2.Theseimplythatx2isexogenousin(15.46),butthatx1iscorrelatedwithe1.WhatweneedisanIVforx1.SuchanIVmustbecorrelatedwithx1,uncorrelatedwithu—sothatitmustbeexcludedfrom(15.45)—anduncorrelatedwiththemeasurementerror,e1.Onepossibilityistoobtainasecondmeasurementonx*1,sayz1.Sinceitisx*1thataffectsy,itisonlynaturaltoassumethatz1isuncorrelatedwithu.Ifwewritez1x*1a1,wherea1isthemeasurementerrorinz1,thenwemustassumethata1ande1areuncorrelated.Inotherwords,x1andz1bothmismeasurex*1,buttheirmeasurementerrorsareuncorrelated.Certainly,x1andz1arecorrelatedthroughtheirdependenceonx*1,sowecanusez1asanIVforx1.Wheremightwegettwomeasurementsonavariable?Sometimes,whenagroupofworkersisaskedfortheirannualsalary,theiremployerscanprovideasecondmeasure.Formarriedcouples,eachspousecanindependentlyreportthelevelofsavingsorfam-ilyincome.IntheAshenfelterandKrueger(1994)studycitedinSection14.3,eachtwinwasaskedabouthisorhersibling’syearsofeducation;thisgivesasecondmeasurethatcanbeusedasanIVforself-reportededucationinawageequation.(AshenfelterandKruegercombineddifferencingandIVtoaccountfortheomittedabilityproblemaswell;moreonthisinSection15.8.)Generally,though,havingtwomeasuresofanexplanatoryvariableisrare.AnalternativeistouseotherexogenousvariablesasIVsforapotentiallymismea-suredvariable.Forexample,ouruseofmotheducandfatheducasIVsforeducinExample15.5canservethispurpose.Ifwethinkthateduceduc*e1,thentheIVestimatesinExample15.5donotsufferfrommeasurementerrorifmotheducandfatheducareuncorrelatedwiththemeasurementerror,e1.Thisisprobablymorerea-sonablethanassumingmotheducandfatheducareuncorrelatedwithability,whichiscontainedinuin(15.45).IVmethodscanalsobeadoptedwhenusingthingsliketestscorestocontrolforunobservedcharacteristics.InSection9.2,weshowedthat,undercertainassumptions,proxyvariablescanbeusedtosolvetheomittedvariablesproblem.InExample9.3,weusedIQasaproxyvariableforunobservedability.ThissimplyentailsaddingIQtothemodelandperforminganOLSregression.Butthereisanalternativethatworkswhen481\nd7/14/997:43PMPage482Part3AdvancedTopicsIQdoesnotfullysatisfytheproxyvariableassumptions.Toillustrate,writeawageequationas2log(wage)01educ2exper3experabilu,(15.47)whereweagainhavetheomittedabilityproblem.Butwehavetwotestscoresthatareindicatorsofability.Weassumethatthescorescanbewrittenastest11abile1andtest21abile2,where10,10.Sinceitisabilitythataffectswage,wecanassumethattest1andtest2areuncorrelatedwithu.Ifwewriteabilintermsofthefirsttestscoreandplugtheresultinto(15.47),weget2log(wage)01educ2exper3exper(15.48)1test1(u1e1),where11/1.Now,ifweassumethate1isuncorrelatedwithalltheexplanatoryvariablesin(15.47),includingabil,thene1andtest1mustbecorrelated.[Noticethateducisnotendogenousin(15.48);however,test1is.]Thismeansthatestimating(15.48)byOLSwillproduceinconsistentestimatorsofthej(and1).Undertheassumptionswehavemade,test1doesnotsatisfytheproxyvariableassumptions.Ifweassumethate2isalsouncorrelatedwithalltheexplanatoryvariablesin(15.47)andthate1ande2areuncorrelated,thene1isuncorrelatedwiththesecondtestscore,test2.Therefore,test2canbeusedasanIVfortest1.EXAMPLE15.6(UsingTwoTestScoresasIndicatorsofAbility)WeusethedatainWAGE2.RAWtoimplementtheprecedingprocedure,whereIQplaystheroleofthefirsttestscore,andKWW(knowledgeoftheworldofwork)isthesecondtestscore.TheexplanatoryvariablesarethesameasinExample9.3:educ,exper,tenure,mar-ried,south,urban,andblack.RatherthanaddingIQanddoingOLS,asincolumn(2)ofTable9.2,weaddIQanduseKWWasitsinstrument.Thecoefficientoneducis.025(se.017).Thisisalowestimate,anditisnotstatisticallydifferentfromzero.Thisisapuzzlingfinding,anditsuggeststhatoneofourassumptionsfails;perhapse1ande2arecorrelated.15.5TESTINGFORENDOGENEITYANDTESTINGOVERIDENTIFYINGRESTRICTIONSInthissection,wedescribetwoimportanttestsinthecontextofinstrumentalvariablesestimation.482\nd7/14/997:43PMPage483Chapter15InstrumentalVariablesEstimationandTwoStageLeastSquaresTestingforEndogeneityThe2SLSestimatorislessefficientthanOLSwhentheexplanatoryvariablesareexogenous;aswehaveseen,the2SLSestimatescanhaveverylargestandarderrors.Therefore,itisusefultohaveatestforendogeneityofanexplanatoryvariablethatshowswhether2SLSisevennecessary.Obtainingsuchatestisrathersimple.Toillustrate,supposewehaveasinglesuspectedendogenousvariable,y101y22z13z2u1,(15.49)wherez1andz2areexogenous.Wehavetwoadditionalexogenousvariables,z3andz4,whichdonotappearin(15.49).Ify2isuncorrelatedwithu1,weshouldestimate(15.49)byOLS.Howcanwetestthis?Hausman(1978)suggesteddirectlycomparingtheOLSand2SLSestimatesanddeterminingwhetherthedifferencesarestatisticallysignifi-cant.Afterall,bothOLSand2SLSareconsistentifallvariablesareexogenous.If2SLSandOLSdiffersignificantly,weconcludethaty2mustbeendogenous(maintainingthatthezjareexogenous).ItisagoodideatocomputeOLSand2SLStoseeiftheestimatesarepracticallydifferent.Todeterminewhetherthedifferencesarestatisticallysignificant,itiseasiertousearegressiontest.Thisisbasedonestimatingthereducedformfory2,whichinthiscaseisy201z12z23z34z4v2.(15.50)Now,sinceeachzjisuncorrelatedwithu1,y2isuncorrelatedwithu1ifandonlyifv2isuncorrelatedwithu1;thisiswhatwewishtotest.Writeu11v2e1,wheree1isuncorrelatedwithv2andhaszeromean.Then,u1andv2areuncorrelatedifandonlyif10.Theeasiestwaytotestthisistoincludev2asanadditionalregressorin(15.49)andtodoattest.Thereisonlyoneproblemwithimplementingthis:v2isnotobserved,becauseitistheerrortermin(15.50).Becausewecanestimatethereducedformfory2byOLS,wecanobtainthereducedformresiduals,vˆ2.Therefore,weestimatey101y22z13z21vˆ2error(15.51)byOLSandtestH0:10usingatstatistic.IfwerejectH0atasmallsignificancelevel,weconcludethaty2isendogenousbecausev2andu1arecorrelated.EXAMPLE15.7(ReturntoEducationforWorkingWomen)Wecantestforendogeneityofeducin(15.40)byobtainingtheresidualsvˆ2fromestimat-ingthereducedform(15.41)—usingonlyworkingwomen—andincludingthesein(15.40).Whenwedothis,thecoefficientonvˆ2isˆ1.058,andt1.67.Thisismoderateevi-denceofpositivecorrelationbetweenu1andv2.Itisprobablyagoodideatoreportbothestimatesbecausethe2SLSestimateofthereturntoeducation(6.1%)iswell-belowtheOLSestimate(10.8%).483\nd7/14/997:43PMPage484Part3AdvancedTopicsTESTINGFORENDOGENEITYOFASINGLEEXPLANATORYVARIABLE:(i)Estimatethereducedformfory2byregressingitonallexogenousvariables(includingthoseinthestructuralequationandtheadditionalIVs).Obtaintheresidu-als,vˆ2.(ii)Addvˆ2tothestructuralequation(whichincludesy2)andtestforsignificanceofvˆ2usinganOLSregression.Ifthecoefficientonvˆ2isstatisticallydifferentfromzero,weconcludethaty2isindeedendogenous.Wemightwanttouseaheteroskedasticity-robustttest.Aninterestingfeatureoftheregressionfrompart(ii)isthattheestimatesonallofthevariables(exceptvˆ2)areidenticaltothe2SLSestimates.Forexample,estimating(15.51)byOLSgivesˆjthatareidenticaltothe2SLSestimatesfromequation(15.49).Thisisasimplewaytoseeifyouhavedonetheproperregressionintestingforendo-geneity.Italsogivesanotherinterpretationof2SLS:includingvˆ2intheOLSregression(15.51)clearsuptheendogeneityofy2.Wecanalsotestforendogeneityofmultipleexplanatoryvariables.Foreachsus-pectedendogenousvariable,weobtainthereducedformresiduals,asinpart(i).Then,wetestforjointsignificanceoftheseresidualsinthestructuralequation,usinganFtest.Jointsignificanceindicatesthatatleastonesuspectedexplanatoryvariableisendogenous.Thenumberofexclusionrestrictionstestedisthenumberofsuspectedendogenousexplanatoryvariables.TestingOveridentificationRestrictionsWhenweintroducedthesimpleinstrumentalvariablesestimatorinSection15.1,weemphasizedthatanIVmustsatisfytworequirements:itmustbeuncorrelatedwiththeerrorandcorrelatedwiththeendogenousexplanatoryvariable.WehaveseeninfairlycomplicatedmodelshowtodecidewhetherthesecondrequirementcanbetestedusingatoranFtestinthereducedformregression.WeclaimedthatthefirstrequirementcannotbetestedbecauseitinvolvesacorrelationbetweentheIVandanunobservederror.However,ifwehavemorethanoneinstrumentalvariable,wecaneffectivelytestwhethersomeofthemareuncorrelatedwiththestructuralerror.Asanexample,againconsiderequation(15.49)withtwoadditionalinstrumentalvariables,z3andz4.Weknowwecanestimate(15.49)usingonlyz3asanIVfory2.GiventheIVestimates,wecancomputetheresiduals,uˆ1y1ˆ0ˆ1y2ˆ2z1ˆ3z2.Becausez4isnotusedatallintheestimation,wecancheckwhetherz4anduˆ1arecorrelatedinthesample.Iftheyare,z4isnotavalidIVfory2.Ofcourse,thistellsusnothingaboutwhetherz3andu1arecorrelated;infact,forthistobeausefultest,wemustassumethatz3andu1areuncorrelated.Nevertheless,ifz3andz4arechosenusingthesamelogic—suchasmother’seducationandfather’seducation—findingthatz4iscorrelatedwithu1castsdoubtonusingz3asanIV.Becausetherolesofz3andz4canbereversed,wecanalsotestwhetherz3iscorre-latedwithu1,providedz4andu1areassumedtobeuncorrelated.Whichtestshouldweuse?Itturnsoutthatourtestchoicedoesnotmatter.WemustassumethatatleastoneIVisexogenous.Then,wecantesttheoveridentifyingrestrictionsthatareusedin2SLS.Forourpurposes,thenumberofoveridentifyingrestrictionsissimplythenum-484\nd7/14/997:43PMPage485Chapter15InstrumentalVariablesEstimationandTwoStageLeastSquaresberofextrainstrumentalvariables.Supposewehaveonlyoneendogenousexplanatoryvariable.IfwehaveonlyasingleIVfory2,wehavenooveridentifyingrestrictions,andthereisnothingthatcanbetested.IfwehavetwoIVsfory2,asinthepreviousexam-ple,wehaveoneoveridentifyingrestriction.IfwehavethreeIVs,wehavetwoover-identifyingrestrictions,andsoon.Testingoveridentifyingrestrictionsisrathersimple.Wemustobtainthe2SLSresid-ualsandthenrunanauxiliaryregression.TESTINGOVERIDENTIFYINGRESTRICTIONS:(i)Estimatethestructuralequationby2SLSandobtainthe2SLSresiduals,uˆ1.2(ii)Regressuˆ1onallexogenousvariables.ObtaintheR-squared,sayR1.22(iii)UnderthenullhypothesisthatallIVsareuncorrelatedwithu1,nR1~ªq,whereqisthenumberofinstrumentalvariablesfromoutsidethemodelminusthetotalnum-2berofendogenousexplanatoryvariables.IfnR1exceeds(say)the5%criticalvaluein2theqdistribution,werejectH0andconcludethatatleastsomeoftheIVsarenotexogenous.EXAMPLE15.8(ReturntoEducationforWorkingWomen)WhenweusemotheducandfatheducasIVsforeducin(15.40),wehaveasingleover-2identifyingrestriction.Regressingthe2SLSresidualsuˆ1onexper,exper,motheduc,and22fatheducproducesR1.0009.Therefore,nR1428(.0009).3852,whichisaverysmall2valueina1distribution(p-value.535).Therefore,theparents’educationvariablespasstheoveridentificationtest.Whenweaddhusband’seducationtotheIVlist,wegettwo2overidentifyingrestrictions,andnR11.11(p-value.574).Therefore,itseemsreason-abletoaddhuseductotheIVlist,asthisreducesthestandarderrorofthe2SLSesti-mate:the2SLSestimateoneducusingallthreeinstrumentsis.080(se.022),sothismakeseducmuchmoresignificantthanwhenhuseducisnotusedasanIV(ˆeduc.061,se.031).Inthepreviousexample,wealludedtoageneralfactabout2SLS:underthestan-dard2SLSassumptions,addinginstrumentstothelistimprovestheasymptoticeffi-ciencyofthe2SLS.Butthisrequiresthatanynewinstrumentsareinfactexogenous—otherwise,2SLSwillnotevenbeconsistent—anditisonlyanasymptoticresult.Withthetypicalsamplesizesavailable,addingtoomanyinstruments—thatis,increasingthenumberofoveridentifyingrestrictions—cancauseseverebiasesin2SLS.Adetaileddiscussionwouldtakeustoofarafield.AniceillustrationisgivenbyBound,Jaeger,andBaker(1995)whoarguethatthe2SLSestimatesofthereturntoeducationobtainedbyAngristandKrueger(1991),usingmanyinstrumentalvariables,arelikelytobeseriouslybiased(evenwithhundredsofthousandsofobservations!).Theoveridentificationtestcanbeusedwheneverwehavemoreinstrumentsthanweneed.Ifwehavejustenoughinstruments,themodelissaidtobejustidentified,andthe485\nd7/14/997:43PMPage486Part3AdvancedTopicsR-squaredinpart(ii)willbeidenticallyzero.Aswementionedearlier,wecannottestexogeneityoftheinstrumentsinthejustidentifiedcase.Thetestcanbemaderobusttoheteroskedasticityofarbitraryform;fordetails,seeWooldridge(1999,Chapter5).15.62SLSWITHHETEROSKEDASTICITYHeteroskedasticityinthecontextof2SLSraisesessentiallythesameissuesaswithOLS.Mostimportantly,itispossibletoobtainstandarderrorsandteststatisticsthatare(asymptotically)robusttoheteroskedasticityofarbitraryandunknownform.Somesoftwarepackagesdothisroutinely.Wecanalsotestforheteroskedasticity,usingananalogoftheBreusch-PagantestthatwecoveredinChapter8.Letuˆdenotethe2SLSresidualsandletz1,z2,…,zmdenotealltheexogenousvariables(incudingthoseusedasIVsfortheendogenousexplanatoryvariables).Then,underreasonableassumptions[spelledout,forexample,inWooldridge(1999,Chapter5)],anasymptoticallyvalidstatisticistheusualFstatis-2ticforjointsignificanceinaregressionofuˆonz1,z2,…,zm.Thenullhypothesisofhomoskedasticityisrejectedifthezjarejointlysignificant.IfweapplythistoExample15.8,usingmotheduc,fatheduc,andhuseducasinstru-mentsforeduc,weobtainF5,4222.53,andp-value.029.Thisisevidenceofhet-eroskedasticityatthe5%level.Wemightwanttocomputeheteroskedasticity-robuststandarderrorstoaccountforthis.Ifweknowhowtheerrorvariancedependsontheexogenousvariables,wecanuseaweighted2SLSprocedure,essentiallythesameasinSection8.4.AfterestimatingamodelforVar(uz1,z2,…,zm),wedividethedependentvariable,theexplanatoryvari-ables,andalltheinstrumentalvariablesforobservationibyhˆi,wherehˆidenotestheestimatedvariance.(Theconstant,whichisbothanexplanatoryvariableandanIV,isdividedbyhˆi;seeSection8.4.)Then,weapply2SLSonthetransformedequationusingthetransformedinstruments.15.7APPLYING2SLSTOTIMESERIESEQUATIONSWhenweapply2SLStotimeseriesdata,manyoftheconsiderationsthataroseforOLSinChapters10,11,and12arerelevant.Writethestructuralequationforeachtimeperiodasyt01xt1…kxtkut,(15.52)whereoneormoreoftheexplanatoryvariablesxtjmightbecorrelatedwithut.Denotethesetofexogenousvariablesbyzt1,…,ztm:E(ut)0,Cov(ztj,ut)0,j1,…,m.Anyexogenousexplanatoryvariableisalsoaztj.Foridentification,itisnecessarythatmk(wehaveasmanyexogenousvariablesasexplanatoryvariables).486\nd7/14/997:43PMPage487Chapter15InstrumentalVariablesEstimationandTwoStageLeastSquaresThemechanicsof2SLSareidenticalfortimeseriesorcross-sectionaldata,butfortimeseriesdatathestatisticalpropertiesof2SLSdependonthetrendingandcorrela-tionpropertiesoftheunderlyingsequences.Inparticular,wemustbecarefultoincludetrendsifwehavetrendingdependentorexplanatoryvariables.Sinceatimetrendisexogenous,itcanalwaysserveasitsowninstrumentalvariable.Thesameistrueofsea-sonaldummyvariables,ifmonthlyorquarterlydataareused.QUESTION15.4SeriesthathavestrongpersistenceAmodeltotesttheeffectofgrowthingovernmentspendingon(haveunitroots)mustbeusedwithcare,growthinoutputisjustaswithOLS.Often,differencingtheequationiswarrantedbeforeestimation,gGDPt01gGOVt2INVRATt3gLABtut,andthisappliestotheinstrumentsaswell.wheregindicatesgrowth,GDPisrealgrossdomesticproduct,GOVUnderanalogsoftheassumptionsinisrealgovernmentspending,INVRATistheratioofgrossdomesticChapter11fortheasymptoticpropertiesofinvestmenttoGDP,andLABissizeofthelaborforce.[SeeequationOLS,2SLSusingtimeseriesdataiscon-(6)inRam(1986).]Underwhatassumptionswouldadummyvari-ableindicatingwhetherthepresidentinyeart1isaRepublicansistentandasymptoticallynormallydis-beasuitableIVforgGOVt?tributed.Infact,ifwereplacetheexplanatoryvariableswiththeinstrumen-talvariablesinstatingtheassumptions,weonlyneedtoaddtheidentificationassump-tionsfor2SLS.Forexample,thehomoskedasticityassumptionisstatedas22E(utzt1,…,ztm),(15.53)andthenoserialcorrelationassumptionisstatedasE(utuszt,zs)0,forallts,(15.54)whereztdenotesallexogenousvariablesattimet.Afullstatementoftheassumptionsisgiveninthechapterappendix.Wewillprovideexamplesof2SLSfortimeseriesproblemsinChapter16;seealsoProblem15.15.AsinthecaseofOLS,thenoserialcorrelationassumptioncanoftenbeviolatedwithtimeseriesdata.Fortunately,itisveryeasytotestforAR(1)serialcorrelation.Ifwewriteutut1etandplugthisintoequation(15.52),wegetyt01xt1…kxtkut1et,t2.(15.55)TotestH0:10,wemustreplaceut1withthe2SLSresiduals,uˆt1.Further,ifxtjisendogenousin(15.52),thenitisendogenousin(15.55),sowestillneedtouseanIV.Becauseetisuncorrelatedwithallpastvaluesofut,uˆt1canbeusedasitsowninstru-ment.TESTINGFORAR(1)SERIALCORRELATIONAFTER2SLS:(i)Estimate(15.52)by2SLSandobtainthe2SLSresiduals,uˆt.(ii)Estimate487\nd7/14/997:43PMPage488Part3AdvancedTopicsyt01xt1…kxtkuˆt1errort,t2,…,nby2SLS,usingthesameinstrumentsfrompart(i),inadditiontouˆt1.Usethetstatis-ticonˆtotestH0:0.AswiththeOLSversionofthistestfromChapter12,thetstatisticonlyhasasymp-toticjustification,butittendstoworkwellinpractice.Aheteroskedasticity-robustver-sioncanbeusedtoguardagainstheteroskedasticity.Further,laggedresidualscanbeaddedtotheequationtotestforhigherformsofserialcorrelationusingajointFtest.Whathappensifwedetectserialcorrelation?Someeconometricspackageswillcomputestandarderrorsthatarerobusttofairlygeneralformsofserialcorrelationandheteroskedasticity.Thisisanice,simplewaytogoifyoureconometricspackagedoesthis.ThecomputationsareverysimilartothoseinSection12.5forOLS.SeeWooldridge(1995)forformulasandothercomputationalmethods.AnalternativeistousetheAR(1)modelandcorrectforserialcorrelation.Thepro-cedureissimilartothatforOLSandplacesadditionalrestrictionsontheinstrumentalvariables.Thequasi-differencedequationisthesameasinequation(12.32):y˜t0(1)1x˜t1…kx˜tket,t2,(15.56)wherex˜tjxtjxt1,j.(Wecanusethet1observationjustasinSection12.3,butweomitthatforsimplicityhere.)Thequestionis:Whatcanweuseasinstrumentalvari-ables?Itseemsnaturaltousethequasi-differencedinstruments,z˜tjztjzt1,j.Thisonlyworks,however,ifin(15.52),theoriginalerrorutisuncorrelatedwiththeinstru-mentsattimest,t1,andt1.Thatis,theinstrumentalvariablesmustbestrictlyexogenousin(15.52).ThisrulesoutlaggeddependentvariablesasIVs,forexample.ItalsoeliminatescaseswherefuturemovementsintheIVsreacttocurrentandpastchangesintheerror,ut.2SLSWITHAR(1)ERRORS:(i)Estimate(15.52)by2SLSandobtainthe2SLSresiduals,uˆt,t1,2,…,n.(ii)Obtainˆfromtheregressionofuˆtonuˆt1,t2,…,nandconstructthequasi-differencedvariablesy˜tytˆyt1,x˜tjxtjˆxt1,j,andz˜tjztjˆzt1,jfort2.(Remember,inmostcasessomeoftheIVswillalsobeexplanatoryvariables.)(iii)Estimate(15.56)(whereisreplacedwithˆ)by2SLS,usingthez˜tjastheinstruments.Assumingthat(15.56)satisfiesthe2SLSassumptionsinthechapterappendix,theusual2SLSteststatisticsareasymptoticallyvalid.15.8APPLYING2SLSTOPOOLEDCROSSSECTIONSANDPANELDATAApplyinginstrumentalvariablesmethodstoindependentlypooledcrosssectionsraisesnonewdifficulties.AswithmodelsestimatedbyOLS,weshouldoftenincludetimeperioddummyvariablestoallowforaggregatetimeeffects.Thesedummyvariablesareexogenous—becausethepassageoftimeisexogenous—andsotheyactastheirowninstruments.488\nd7/14/997:43PMPage489Chapter15InstrumentalVariablesEstimationandTwoStageLeastSquaresEXAMPLE15.9(EffectofEducationonFertility)InExample13.1,weusedthepooledcrosssectioninFERTIL1.RAWtoestimatetheeffectofeducationonwomen’sfertility,controllingforvariousotherfactors.AsinSander(1992),weallowforthepossibilitythateducisendogenousintheequation.Asinstrumentalvari-ablesforeduc,weusemotherandfather’seducationlevels(meduc,feduc).The2SLSesti-mateofeducis.153(se.039),comparedwiththeOLSestimate.128(se.018).The2SLSestimateshowsasomewhatlargereffectofeducationonfertility,butthe2SLSstandardisovertwiceaslargeastheOLSstandarderror.(Infact,the95%confidenceinter-valbasedon2SLSeasilycontainstheOLSestimate.)TheOLSand2SLSestimatesofeducarenotstatisticallydifferent,ascanbeseenbytestingforendogeneityofeducasinSection15.5:whenthereducedformresidual,vˆ2,isincludedwiththeotherregressorsinTable13.1(includingeduc),itststatisticis.702,whichisnotsignificantatanyreasonablelevel.Therefore,inthiscase,weconcludethatthedifferencebetween2SLSandOLSisduetosamplingerror.Instrumentalvariablesestimationcanbecombinedwithpaneldatamethods,par-ticularlyfirstdifferencing,toconsistentlyestimateparametersinthepresenceofunob-servedeffectsandendogeneityinoneormoretime-varyingexplanatoryvariables.Thefollowingsimpleexampleillustratesthiscombinationofmethods.EXAMPLE15.10(JobTrainingandWorkerProductivity)Supposewewanttoestimatetheeffectofanotherhourofjobtrainingonworkerpro-ductivity.Forthetwoyears1987and1988,considerthesimplepaneldatamodellog(scrapit)00d88t1hrsempitaiuit,t1,2,wherescrapitisfirmi’sscraprateinyeart,andhrsempitishoursofjobtrainingperemployee.Asusual,weallowdifferentyearinterceptsandaconstant,unobservedfirmeffect,ai.ForthereasonsdiscussedinSection13.2,wemightbeconcernedthathrsempitiscor-relatedwithai,thelatterofwhichcontainsunmeasuredworkerability.Asbefore,wedif-ferencetoremoveai:log(scrapi)01hrsempiui.(15.57)Normally,wewouldestimatethisequationbyOLS.Butwhatifuiiscorrelatedwithhrsempi?Forexample,afirmmighthiremoreskilledworkers,whileatthesametimereducingthelevelofjobtraining.Inthiscase,weneedaninstrumentalvariableforhrsempi.Generally,suchanIVwouldbehardtofind,butwecanexploitthefactthatsomefirmsreceivedjobtraininggrantsin1988.Ifweassumethatgrantdesignationisuncorrelatedwithui—somethingthatisreasonable,becausethegrantsweregivenatthe489\nd7/14/997:43PMPage490Part3AdvancedTopicsbeginningof1988—thengrantiisvalidasanIV,providedhrsempandgrantarecor-related.UsingthedatainJTRAIN.RAWdifferencedbetween1987and1988,thefirststageregressionishrsˆemp(0.51)(27.88)granthrsˆemp(1.56)0(3.13)grant2n45,R.392.Thisconfirmsthatthechangeinhoursofjobtrainingperemployeeisstronglypositivelyrelatedtoreceivingajobtraininggrantin1988.Infact,receivingajobtraininggrantincreasedper-employeetrainingbyalmost28hours,andgrantdesignationaccountedforalmost40%ofthevariationinhrsemp.Twostageleastsquaresestimationof(15.57)gives(log(sˆcrap).033)(.014)hrsemplog(scrap)(.127)(.008)hrsemp2n45,R.016.Thismeansthat10morehoursofjobtrainingperworkerareestimatedtoreducethescrapratebyabout14%.Forthefirmsinthesample,theaverageamountofjobtrainingin1988wasabout17hoursperworker,withaminimumofzeroandamaximumof88.Forcomparison,OLSestimationof(15.57)givesˆ1.0076(se.0045),sothe2SLSestimateof1isalmosttwiceaslargeinmagnitudeandisslightlymorestatisticallysignif-icant.WhenT3,thedifferencedequationmaycontainserialcorrelation.ThesametestandcorrectionforAR(1)serialcorrelationfromSection15.7canbeused,whereallregressionsarepooledacrossiaswellast.UnobservedeffectsmodelscontaininglaggeddependentvariablesalsorequireIVmethodsforconsistentestimation.Thereasonisthat,afterdifferencing,yi,t1iscor-relatedwithuitbecauseyi,t1andui,t1arecorrelated.WecanusetwoormorelagsofyasIVsforyi,t1.[SeeWooldridge(1999,Chapter11)fordetails.]Instrumentalvariablesafterdifferencingcanbeusedonmatchedpairssamplesaswell.AshenfelterandKrueger(1994)differencedthewageequationacrosstwinstoeliminateunobservedability:log(wage2)log(wage1)01(educ2,2educ1,1)(u2u1),whereeduc1,1isyearsofschoolingforthefirsttwinasreportedbythefirsttwin,andeduc2,2isyearsofschoolingforthesecondtwinasreportedbythesecondtwin.Toaccountforpossiblemeasurementerrorintheself-reportedschoolingmeasures,AshenfelterandKruegerused(educ2,1educ1,2)asanIVfor(educ2,2educ1,1),whereeduc2,1isyearsofschoolingforthesecondtwinasreportedbythefirsttwin,andeduc1,2isyearsofschoolingforthefirsttwinasreportedbythesecondtwin.TheIVestimateof1is.167(t3.88),comparedwiththeOLSestimateonthefirstdiffer-encesof.092(t3.83)[seeAshenfelterandKrueger(1994,Table3)].490\nd7/14/997:43PMPage491Chapter15InstrumentalVariablesEstimationandTwoStageLeastSquaresSUMMARYInChapter15,wehaveintroducedthemethodofinstrumentalvariablesasawaytoconsistentlyestimatetheparametersinalinearmodelwhenoneormoreexplanatoryvariablesareendogenous.Aninstrumentalvariablemusthavetwoproperties:(1)itmustbeexogenous,thatis,uncorrelatedwiththeerrortermofthestructuralequation;(2)itmustbepartiallycorrelatedwiththeendogenousexplanatoryvariable.Findingavariablewiththesetwopropertiesisoftenchallenging.Themethodoftwostageleastsquares,whichallowsformoreinstrumentalvari-ablesthanwehaveexplanatoryvariables,isusedroutinelyintheempiricalsocialsci-ences.Whenusedproperly,itcanallowustoestimateceterisparibuseffectsinthepresenceofendogenousexplanatoryvariables.Thisistrueincross-sectional,timeseries,andpaneldataapplications.Butwheninstrumentsarepoor—whichmeanstheyarecorrelatedwiththeerrorterm,onlyweaklycorrelatedwiththeendogenousexplana-toryvariable,orboth—then2SLScanbeworsethanOLS.Whenwehavevalidinstrumentalvariables,wecantestwhetheranexplanatoryvariableisendogenous,usingthetestinSection15.5.Inaddition,whilewecannevertestwhetherallIVsareexogenous,wecantestthatatleastsomeofthemare—assumingthatwehavemoreinstrumentsthanweneedforconsistentestimation(thatis,themodelisoveridentified).Heteroskedasticityandserialcorrelationcanbetestedforanddealtwithusingmethodssimilartothecaseofmodelswithexogenousexplana-toryvariables.Inthischapter,weusedomittedvariablesandmeasurementerrortoillustratethemethodofinstrumentalvariables.IVmethodsarealsoindispensableforsimultaneousequationsmodels,whichwewillcoverinChapter16.KEYTERMSEndogenousExplanatoryVariablesNaturalExperimentErrors-in-VariablesOmittedVariablesExclusionRestrictionsOrderConditionExogenousExplanatoryVariablesOveridentifyingRestrictionsExogenousVariablesRankConditionIdentificationReducedFormEquationInstrumentalVariablesStructuralEquationInstrumentalVariables(IV)EstimatorTwoStageLeastSquares(2SLS)EstimatorPROBLEMS15.1Considerasimplemodeltoestimatetheeffectofpersonalcomputer(PC)owner-shiponcollegegradepointaverageforgraduatingseniorsatalargepublicuniversity:GPA01PCu,wherePCisabinaryvariableindicatingPCownership.(i)WhymightPCownershipbecorrelatedwithu?491\nd7/14/997:43PMPage492Part3AdvancedTopics(ii)ExplainwhyPCislikelytoberelatedtoparents’annualincome.DoesthismeanparentalincomeisagoodIVforPC?Whyorwhynot?(iii)Supposethat,fouryearsago,theuniversitygavegrantstobuycomput-erstoroughlyone-halfoftheincomingstudents,andthestudentswhoreceivedgrantswererandomlychosen.CarefullyexplainhowyouwouldusethisinformationtoconstructaninstrumentalvariableforPC.15.2Supposethatyouwishtoestimatetheeffectofclassattendanceonstudentper-formance,asinExample6.3.Abasicmodelisstndfnl01atndrte2priGPA3ACTu,wherethevariablesaredefinedasinChapter6.(i)Letdistbethedistancefromthestudents’livingquarterstothelecturehall.Doyouthinkdistisuncorrelatedwithu?(ii)Assumingthatdistanduareuncorrelated,whatotherassumptionmustdistsatisfyinordertobeavalidIVforatndrte?(iii)Suppose,asinequation(6.18),weaddtheinteractiontermpriGPAatndrte:stndfnl01atndrte2priGPA3ACT4priGPAatndrteu.Ifatndrteiscorrelatedwithu,then,ingeneral,soispriGPAatndrte.WhatmightbeagoodIVforpriGPAatndrte?[Hint:IfE(upriGPA,ACT,dist)0,ashappenswhenpriGPA,ACT,anddistareallexogenous,thenanyfunctionofpriGPAanddistisuncorrelatedwithu.]15.3Considerthesimpleregressionmodely01xuandletzbeabinaryinstrumentalvariableforx.Use(15.10)toshowthattheIVesti-matorˆ1canbewrittenasˆ1(y¯1y¯0)/(x¯1x¯0),wherey¯0andx¯0arethesampleaveragesofyiandxioverthepartofthesamplewithzi0,andwherey¯1andx¯1arethesampleaveragesofyiandxioverthepartofthesam-plewithzi1.Thisestimator,knownasagroupingestimator,wasfirstsuggestedbyWald(1940).15.4Supposethat,foragivenstateintheUnitedStates,youwishtouseannualtimeseriesdatatoestimatetheeffectofthestate-levelminimumwageontheemploymentofthose18to25yearsold(EMP).AsimplemodelisgEMPt01gMINt2gPOPt3gGSPt4gGDPtut,whereMINtistheminimumwage,inrealdollars,POPtisthepopulationfrom18to25yearsold,GSPtisgrossstateproduct,andGDPtisU.S.grossdomesticproduct.Thegprefixindicatesthegrowthratefromyeart1toyeart,whichwouldtypicallybeapproximatedbythedifferenceinthelogs.492\nd7/14/997:43PMPage493Chapter15InstrumentalVariablesEstimationandTwoStageLeastSquares(i)Ifweareworriedthatthestatechoosesitsminimumwagepartlybasedonunobserved(tous)factorsthataffectyouthemployment,whatistheproblemwithOLSestimation?(ii)LetUSMINtbetheU.S.minimumwage,whichisalsomeasuredinrealterms.DoyouthinkgUSMINtisuncorrelatedwithut?(iii)Bylaw,anystate’sminimumwagemustbeatleastaslargeastheU.S.minimum.ExplainwhythismakesgUSMINtapotentialIVcandidateforgMINt.15.5Refertoequations(15.19)and(15.20).Assumethatux,sothatthepopula-tionvariationintheerrortermisthesameasitisinx.Supposethattheinstrumentalvariable,z,isslightlycorrelatedwithu:Corr(z,u).1.Supposealsothatzandxhaveasomewhatstrongercorrelation:Corr(z,x).2.(i)WhatistheasymptoticbiasintheIVestimator?(ii)HowmuchcorrelationwouldhavetoexistbetweenxandubeforeOLShasmoreasymptoticbiasthan2SLS?15.6(i)Inthemodelwithoneendogenousexplanatoryvariable,oneexogenousexplanatoryvariable,andoneextraexogenousvariable,takethereducedformfory2,(15.26),andplugitintothestructuralequation(15.22).Thisgivesthereducedformfory1:y101z12z2v1.Findthejintermsofthejandthej.(ii)Findthereducedformerror,v1,intermsofu1,v2,andtheparameters.(iii)Howwouldyouconsistentlyestimatethej?15.7Thefollowingisasimplemodeltomeasuretheeffectofaschoolchoiceprogramonstandardizedtestperformance[seeRouse(1998)formotivation]:score01choice2famincu1,wherescoreisthescoreonastatewidetest,choiceisabinaryvariableindicatingwhetherastudentattendedachoiceschoolinthelastyear,andfamincisfamilyincome.TheIVforchoiceisgrant,thedollaramountgrantedtostudentstousefortuitionatchoiceschools.Thegrantamountdifferedbyfamilyincomelevel,whichiswhywecontrolforfamincintheequation.(i)Evenwithfamincintheequation,whymightchoicebecorrelatedwithu1?(ii)Ifwithineachincomeclass,thegrantamountswereassignedrandomly,isgrantuncorrelatedwithu1?(iii)Writethereducedformequationforchoice.Whatisneededforgranttobepartiallycorrelatedwithchoice?(iv)Writethereducedformequationforscore.Explainwhythisisuseful.(Hint:Howdoyouinterpretthecoefficientongrant?)15.8Supposeyouwanttotestwhethergirlswhoattendagirls’highschooldobetterinmaththangirlswhoattendcoedschools.YouhavearandomsampleofseniorhighschoolgirlsfromastateintheUnitedStates,andscoreisthescoreonastandardized493\nd7/14/997:43PMPage494Part3AdvancedTopicsmathtest.Letgirlhsbeadummyvariableindicatingwhetherastudentattendsagirls’highschool.(i)Whatotherfactorswouldyoucontrolforintheequation?(Youshouldbeabletoreasonablycollectdataonthesefactors.)(ii)Writeanequationrelatingscoretogirlhsandtheotherfactorsyoulistedinpart(i).(iii)Supposethatparentalsupportandmotivationareunmeasuredfactorsintheerrorterminpart(ii).Aretheselikelytobecorrelatedwithgirlhs?Explain.(iv)Discusstheassumptionsneededforthenumberofgirls’highschoolswithinatwenty-mileradiusofagirl’shometobeavalidIVforgirlhs.15.9Supposethat,inequation(15.8),youdonothaveagoodinstrumentalvariablecandidateforskipped.Butyouhavetwootherpiecesofinformationonstudents:com-binedSATscoreandcumulativeGPApriortothesemester.WhatwouldyoudoinsteadofIVestimation?15.10Inarecentarticle,EvansandSchwab(1995)studiedtheeffectsofattendingaCatholichighschoolontheprobabilityofattendingcollege.Forconcreteness,letcol-legebeabinaryvariableequaltounityifastudentattendscollege,andzerootherwise.LetCathHSbeabinaryvariableequaltooneifthestudentattendsaCatholichighschool.Alinearprobabilitymodeliscollege01CathHSotherfactorsu,wheretheotherfactorsincludegender,race,familyincome,andparentaleducation.(i)WhymightCathHSbecorrelatedwithu?(ii)EvansandSchwabhavedataonastandardizedtestscoretakenwheneachstudentwasasophomore.WhatcanbedonewiththesevariablestoimprovetheceterisparibusestimateofattendingaCatholichighschool?(iii)LetCathRelbeabinaryvariableequaltooneifthestudentisCatholic.DiscussthetworequirementsneededforthistobeavalidIVforCathHSintheprecedingequation.Whichofthesecanbetested?(iv)Notsurprisingly,beingCatholichasasignificanteffectonattendingaCatholichighschool.DoyouthinkCathRelisaconvincinginstrumentforCathHS?15.11Considerasimpletimeseriesmodelwheretheexplanatoryvariablehasclassicalmeasurementerror:yt01x*tut(15.58)xtx*tet,whereuthaszeromeanandisuncorrelatedwithx*tandet.Weobserveytandxtonly.Assumethatethaszeromeanandisuncorrelatedwithx*tandthatx*talsohasazeromean(thislastassumptionisonlytosimplifythealgebra).494\nd7/14/997:43PMPage495Chapter15InstrumentalVariablesEstimationandTwoStageLeastSquares(i)Writex*txtetandplugthisinto(15.58).Showthattheerrorterminthenewequation,sayvt,isnegativelycorrelatedwithxtif10.WhatdoesthisimplyabouttheOLSestimatorof1fromtheregres-sionofytonxt?(ii)Inadditiontothepreviousassumptions,assumethatutandetareuncor-relatedwithallpastvaluesofx*tandet;inparticular,withx*t1andet1.ShowthatE(xt1vt)0,wherevtistheerrorterminthemodelfrompart(i).(iii)Arextandxt1likelytobecorrelated?Explain.(iv)Whatdoparts(ii)and(iii)suggestasausefulstrategyforconsistentlyestimating0and1?COMPUTEREXERCISES15.12UsethedatainWAGE2.RAWforthisexercise.(i)InExample15.2,usingsibsasaninstrumentforeduc,theIVestimateofthereturntoeducationis.122.ToconvinceyourselfthatusingsibsasanIVforeducisnotthesameasjustpluggingsibsinforeducandrunninganOLSregression,runtheregressionoflog(wage)onsibsandexplainyourfindings.(ii)Thevariablebrthordisbirthorder(brthordisoneforafirst-bornchild,twoforasecond-bornchild,andsoon).Explainwhyeducandbrthordmightbenegativelycorrelated.Regresseduconbrthordtodeterminewhetherthereisastatisticallysignificantnegativecorrelation.(iii)UsebrthordasanIVforeducinequation(15.1).Reportandinterprettheresults.(iv)Now,supposethatweincludenumberofsiblingsasanexplanatoryvariableinthewageequation;thiscontrolsforfamilybackground,tosomeextent:log(wage)01educ2sibsu.SupposethatwewanttousebrthordasanIVforeduc,assumingthatsibsisexogenous.Thereducedformforeduciseduc01sibs2brthordv.Stateandtesttheidentificationassumption.(v)Estimatetheequationfrompart(iv)usingbrthordasanIVforeduc(andsibsasitsownIV).Commentonthestandarderrorsforˆeducandˆsibs.(vi)Usingthefittedvaluesfrompart(iv),edˆuc,computethecorrelationbetweenedˆucandsibs.Usethisresulttoexplainyourfindingsfrompart(v).15.13ThedatainFERTIL2.RAWincludes,forwomeninBotswanaduring1988,infor-mationonnumberofchildren,yearsofeducation,age,andreligiousandeconomicsta-tusvariables.495\nd7/14/997:43PMPage496Part3AdvancedTopics(i)EstimatethismodelbyOLS2children01educ2age3ageuandinterprettheestimates.Inparticular,holdingagefixed,whatistheestimatedeffectofanotheryearofeducationonfertility?If100womenreceiveanotheryearofeducation,howmanyfewerchildrenaretheyexpectedtohave?(ii)Frsthalfisadummyvariableequaltooneifthewomanwasborndur-ingthefirstsixmonthsoftheyear.Assumingthatfrsthalfisuncorre-latedwiththeerrortermfrompart(i),showthatfrsthalfisareasonableIVcandidateforeduc.(Hint:Youneedtodoaregression.)(iii)Estimatethemodelfrompart(i)byusingfrsthalfasanIVforeduc.ComparetheestimatedeffectofeducationwiththeOLSestimatefrompart(i).(iv)Addthebinaryvariableselectric,tv,andbicycletothemodelandassumetheseareexogenous.EstimatetheequationbyOLSand2SLSandcomparetheestimatedcoefficientsoneduc.Interpretthecoeffi-cientontvandexplainwhytelevisionownershiphasanegativeeffectonfertility.15.14UsethedatainCARD.RAWforthisexercise.(i)TheequationweestimatedinExample15.4canbewrittenaslog(wage)01educ2exper…u,wheretheotherexplanatoryvariablesarelistedinTable15.1.InorderforIVtobeconsistent,theIVforeduc,nearc4,mustbeuncorrelatedwithu.Couldnearc4becorrelatedwiththingsintheerrorterm,suchasunobservedability?Explain.(ii)Forasubsampleofthemeninthedataset,anIQscoreisavailable.RegressIQonnearc4tocheckwhetheraverageIQscoresvarybywhetherthemangrewupnearafour-yearcollege.Whatdoyoucon-clude?(iii)NowregressIQonnearc4,smsa66,andthe1966regionaldummyvari-ablesreg662,…,reg669.AreIQandnearc4relatedafterthegeographicdummyvariableshavebeenpartialledout?Reconcilethiswithyourfindingsfrompart(ii).(iv)Fromparts(ii)and(iii),whatdoyouconcludeabouttheimportanceofcontrollingforsmsa66andthe1966regionaldummiesinthelog(wage)equation?15.15UsethedatainINTDEF.RAWforthisexercise.Asimpleequationrelatingthethree-month,T-Billratetotheinflationrate(constructedfromtheconsumerpriceindex)isi3t01inftut.(i)EstimatethisequationbyOLS,omittingthefirsttimeperiodforlatercomparisons.Reporttheresultsintheusualform.496\nd7/14/997:43PMPage497Chapter15InstrumentalVariablesEstimationandTwoStageLeastSquares(ii)Someeconomistsfeelthattheconsumerpriceindexmismeasuresthetruerateofinflation,sothattheOLSfrompart(i)suffersfrommea-surementerrorbias.Reestimatetheequationfrompart(i),usinginft1asanIVforinft.HowdoestheIVestimateof1comparewiththeOLSestimate?(iii)Nowfirstdifferencetheequation:i3t01inftut.EstimatethisbyOLSandcomparetheestimateof1withthepreviousestimates.(iv)Canyouuseinft1asanIVforinftinthedifferencedequationinpart(iii)?Explain.(Hint:Areinftandinft1sufficientlycorrelated?)15.16UsethedatainCARD.RAWforthisexercise.(i)InTable15.1,thedifferencebetweentheIVandOLSestimatesofthereturntoeducationareeconomicallyimportant.Obtainthereducedformresiduals,vˆ2,from(15.32).(SeeTable15.1fortheothervariablestoincludeintheregression.)Usethesetotestwhethereducisexoge-nous;thatis,determineifthedifferencebetweenOLSandIVisstatis-ticallysignificant.(ii)Estimatetheequationby2SLS,addingnearc2asaninstrument.Doesthecoefficientoneducchangemuch?(iii)Testthesingleoveridentifyingrestrictionfrompart(ii).15.17UsethedatainMURDER.RAWforthisexercise.Thevariablemrdrteisthemur-derrate,thatis,thenumberofmurdersper100,000people.Thevariableexecisthetotalnumberofprisonersexecutedforthecurrentandpriortwoyears;unemisthestateunemploymentrate.(i)Howmanystatesexecutedatleastoneprisonerin1991,1992,or1993?Whichstatehadthemostexecutions?(ii)Usingthetwoyears1990and1993,doapooledregressionofmrdrteond93,exec,andunem.Whatdoyoumakeofthecoefficientonexec?(iii)Usingthechangesfrom1990to1993only(foratotalof51observa-tions),estimatetheequationmrdrte01exec2unemubyOLSandreporttheresultsintheusualform.Now,doescapitalpun-ishmentappeartohaveadeterrenteffect?(iv)Thechangeinexecutionsmaybeatleastpartlyrelatedtochangesintheexpectedmurderrate,sothatexeciscorrelatedwithuinpart(iii).Itmightbereasonabletoassumethatexec1isuncorrelatedwithu.(Afterall,exec1dependsonexecutionsthatoccuredthreeormoreyearsago.)Regressexeconexec1toseeiftheyaresufficientlycor-related;interpretthecoefficientonexec1.(v)Reestimatetheequationfrompart(iii),usingexec1asanIVforexec.Assumethatunemisexogenous.Howdoyourconclusionschangefrompart(iii)?497\nd7/14/997:43PMPage498Part3AdvancedTopics15.18UsethedatainPHILLIPS.RAWforthisexercise.(i)InExample11.5,weestimatedanexpectationsaugmentedPhillipscurveoftheforminft01unemtet,whereinftinftinft1.InestimatingthisequationbyOLS,weassumedthatthesupplyshock,et,wasuncorrelatedwithunemt.Ifthisisfalse,whatcanbesaidabouttheOLSestimatorof1?(ii)Supposethatetisunpredictablegivenallpastinformation:E(etinft1,unemt1,…)0.Explainwhythismakesunemt1agoodIVcandidateforunemt.(iii)Regressunemtonunemt1.Areunemtandunemt1significantlycorre-lated?(iv)EstimatetheexpectationsaugmentedPhillipscurvebyIV.ReporttheresultsintheusualformandcomparethemwiththeOLSestimatesfromExample11.5.APPENDIX15AAssumptionsforTwoStageLeastSquaresThisappendixcoverstheassumptionsunderwhich2SLShasdesirablelargesampleproperties.Wefirststatetheassumptionsforcross-sectionalapplicationsunderrandomsampling.Then,wediscusswhatneedstobeaddedforthemtoapplytotimeseriesandpaneldata.ASSUMPTION2SLS.1(LINEARINPARAMETERS)Themodelinthepopulationcanbewrittenasy01x12x2…kxku,where0,1,…,karetheunknownparameters(constants)ofinterest,anduisanunob-servablerandomerrororrandomdisturbanceterm.Theinstrumentalvariablesaredenotedzj.ASSUMPTION2SLS.2(RANDOMSAMPLING)Wehavearandomsampleony,thexj,andthezj.ASSUMPTION2SLS.3(EXOGENOUSINSTRUMENTALVARIABLES)Theerrortermuhaszeromean,andeachIVisuncorrelatedwithu.RememberthatanyxjthatisuncorrelatedwithualsoactsasanIV.498\nd7/14/997:43PMPage499Chapter15InstrumentalVariablesEstimationandTwoStageLeastSquaresASSUMPTION2SLS.4(RANKCONDITION)(i)Therearenoperfectlinearrelationshipsamongtheinstrumentalvariables.(ii)Therankconditionforidentificationholds.Withasingleendogenousexplanatoryvariable,asinequation(15.42),therankcon-ditioniseasilydescribed.Letz1,…,zmdenotetheexogenousvariables,wherezk,…,zmdonotappearinthestructuralmodel(15.42).Thereducedformofy2isy201z12z2…k1zk1kzk…mzmv2.Then,weneedatleastoneofk,…,mtobenonzero.Thisrequiresatleastoneexoge-nousvariablethatdoesnotappearin(15.42)(theordercondition).Statingtherankcon-ditionwithtwoormoreendogenousexplanatoryvariablesrequiresmatrixalgebra.[SeeWooldridge(1999,Chapter5).]THEOREM15A.1UnderAssumptions2SLS.1through2SLS.4,the2SLSestimatorisconsistent.ASSUMPTION2SLS.5(HOMOSKEDASTICITY)22Letzdenotethecollectionofallinstrumentalvariables.ThenE(uz).THEOREM15A.2UnderAssumptions2SLS.1through2SLS.5,the2SLSestimatorsareasymptoticallynormallydistributed.Consistentestimatorsoftheasymptoticvariancearegivenasinequationn(15.43),where2isreplacedwithˆ2(nk1)2uˆ2,andtheuˆarethe2SLSiii1residuals.The2SLSestimatorisalsothebestIVestimatorunderthefiveassumptionsgiven.Westatetheresulthere.AproofcanbefoundinWooldridge(1999).THEOREM15A.3UnderAssumptions2SLS.1through2SLS.5,the2SLSestimatorisasymptoticallyefficientintheclassofIVestimatorsthatuseslinearcombinationsoftheexogenousvariablesasinstru-ments.Ifthehomoskedasticityassumptiondoesnothold,the2SLSestimatorsarestillasymptoticallynormal,butthestandarderrors(andtandFstatistics)needtobeadjusted;manyeconometricspackagesdothisroutinely.Moreover,the2SLSestimatorisnolongertheasymptoticallyefficientIVestimator,ingeneral.Wewillnotstudymoreefficientestimatorshere[seeWooldridge(1999,Chapter8)].Fortimeseriesapplications,wemustaddsomeassumptions.First,aswithOLS,wemustassumethatallseries(includingtheIVs)areweaklydependent:thisensuresthatthelawoflargenumbersandthecentrallimittheoremhold.Fortheusualstandard499\nd7/14/997:43PMPage500Part3AdvancedTopicserrorsandteststatisticstobevalid,aswellasforasymptoticefficiency,wemustaddanoserialcorrelationassumption.ASSUMPTION2SLS.6(NOSERIALCORRELATION)Equation(15.54)holds.Asimilarnoserialcorrelationassumptionisneededinpaneldataapplications.TestsandcorrectionsforserialcorrelationwerediscussedinSection15.7.500\nChapterSixteenSimultaneousEquationsModelsnthepreviouschapter,weshowedhowthemethodofinstrumentalvariablescanIsolvetwokindsofendogeneityproblems:omittedvariablesandmeasurementerror.Conceptually,theseproblemsarestraightforward.Intheomittedvariablescase,thereisavariable(ormorethanone)thatwewouldliketoholdfixedwhenestimatingtheceterisparibuseffectofoneormoreoftheobservedexplanatoryvariables.Inthemeasurementerrorcase,wewouldliketoestimatetheeffectofcertainexplanatoryvariablesony,butwehavemismeasuredoneormorevariables.Inbothcases,wecouldestimatetheparametersofinterestbyOLSifwecouldcollectbetterdata.Anotherimportantformofendogeneityofexplanatoryvariablesissimultaneity.Thisariseswhenoneormoreoftheexplanatoryvariablesisjointlydeterminedwiththedependentvariable,typicallythroughanequilibriummechanism(aswewillseelater).Inthischapter,westudymethodsforestimatingsimplesimultaneousequationsmodels(SEMs).WhileacompletetreatmentofSEMsisbeyondthescopeofthistext,weareabletocovermodelsthatarewidelyused.Theleadingmethodforestimatingsimultaneousequationsmodelsisthemethodofinstrumentalvariables.Therefore,thesolutiontothesimultaneityproblemisessentiallythesameastheIVsolutionstotheomittedvariablesandmeasurementerrorproblems.However,craftingandinterpretingSEMsischallenging.Therefore,webeginbydis-cussingthenatureandscopeofsimultaneousequationsmodelsinSection16.1.InSection16.2,weconfirmthatOLSappliedtoanequationinasimultaneoussystemisgenerallybiasedandinconsistent.Section16.3providesageneraldescriptionofidentificationandestimationinatwo-equationsystem,whileSection16.4brieflycoversmodelswithmorethantwoequations.Simultaneousequationsmodelsareusedtomodelaggregatetimeseries,andinSection16.5weincludeadiscussionofsomespecialissuesthatariseinsuchmod-els.Section16.6touchesonsimultaneousequationsmodelswithpaneldata.16.1THENATUREOFSIMULTANEOUSEQUATIONSMODELSThemostimportantpointtorememberinusingsimultaneousequationsmodelsisthateachequationinthesystemshouldhaveaceterisparibus,causalinterpretation.501\nPart3AdvancedTopicsBecauseweonlyobservetheoutcomesinequilibrium,wearerequiredtousecounter-factualreasoninginconstructingtheequationsofasimultaneousequationsmodel.Wemustthinkintermsofpotentialaswellasactualoutcomes.TheclassicexampleofanSEMisasupplyanddemandequationforsomecommod-ityorinputtoproduction(suchaslabor).Forconcreteness,lethsdenotetheannuallaborhourssuppliedbyworkersinagriculture,measuredatthecountylevel,andletwdenotetheaveragehourlywageofferedtosuchworkers.Asimplelaborsupplyfunctionishs1w1z1u1,(16.1)wherez1issomeobservedvariableaffectinglaborsupply—say,theaveragemanufac-turingwageinthecounty.Theerrorterm,u1,containsotherfactorsthataffectlaborsupply.[Manyofthesefactorsareobservedandcouldbeincludedinequation(16.1);toillustratethebasicconcepts,weincludeonlyonesuchfactor,z1.]Equation(16.1)isanexampleofastructuralequation.Thisnamecomesfromthefactthatthelaborsup-plyfunctionisderivablefromeconomictheoryandhasacausalinterpretation.Thecoefficient1measureshowlaborsupplychangeswhenthewagechanges;ifhsandwareinlogarithmicform,1isthelaborsupplyelasticity.Typically,weexpect1tobepositive(althougheconomictheorydoesnotruleout10).Laborsupplyelasticitiesareimportantfordetermininghowworkerswillchangethenumberofhourstheydesiretoworkwhentaxratesonwageincomechange.Ifz1ismanufacturingwage,weexpect10:otherfactorsequal,ifthemanufacturingwageincreases,moreworkerswillgointomanufacturingthanintoagriculture.Whenwegraphlaborsupply,wesketchhoursasafunctionofwage,withz1andu1heldfixed.Achangeinz1shiftsthelaborsupplyfunction,asdoesashiftinu1.Thedif-ferenceisthatz1isobservedwhileu1isnot.Sometimes,z1iscalledanobservedsup-plyshifter,andu1iscalledanunobservedsupplyshifter.Howdoesequation(16.1)differfromthosewehavestudiedpreviously?Thedif-ferenceissubtle.Whileequation(16.1)issupposedtoholdforallpossiblevaluesofwage,wecannotgenerallyviewwageasvaryingexogenouslyforacrosssectionofcounties.Ifwecouldrunanexperimentwherewevarythelevelofagriculturalandmanufacturingwagesacrossasampleofcountiesandsurveyworkerstoobtainthelaborsupplyhs,thenwecouldestimate(16.1)byOLS.Unfortunately,thisisnotaman-ageableexperiment.Instead,wemustcollectdataonaveragewagesinthesetwosec-torsalongwithhowmanypersonhourswerespentinagriculturalproduction.Indecidinghowtoanalyzethesedata,wemustunderstandthattheyarebestdescribedbytheinteractionoflaborsupplyanddemand.Undertheassumptionthatlabormarketsclear,weactuallyobserveequilibriumvaluesofwagesandhoursworked.Todescribehowequilibriumwagesandhoursaredetermined,weneedtobringinthedemandforlabor,whichwesupposeisgivenbyhd2w2z2u2,(16.2)wherehdishoursdemanded.Aswiththesupplyfunction,wegraphhoursdemandedasafunctionofwage,w,keepingz2andu2fixed.Thevariablez2—say,agriculturallandarea—isanobservabledemandshifter,whileu2isanunobservabledemandshifter.502\nChapter16SimultaneousEquationsModelsJustaswiththelaborsupplyequation,thelabordemandequationisastructuralequation:itcanbeobtainedfromtheprofitmaximizationconsiderationsoffarmers.Ifhdandwareinlogarithmicform,2isthelabordemandelasticity.Economictheorytellsusthat20.Becauselaborandlandarecomplementsinproduction,weexpect20.Noticehowequations(16.1)and(16.2)describeentirelydifferentrelationships.Laborsupplyisabehavioralequationforworkers,andlabordemandisabehavioralrelationshipforfarmers.Eachequationhasaceterisparibusinterpretationandstandsonitsown.Theybecomelinkedinaneconometricanalysisonlybecauseobservedwageandhoursaredeterminedbytheintersectionofsupplyanddemand.Inotherwords,foreachcountyi,observedhourshiandobservedwagewiaredeterminedbytheequilibriumconditionhishid.(16.3)Becauseweobserveonlyequilibriumhoursforeachcountyi,wedenoteobservedhoursbyhi.Whenwecombinetheequilibriumconditionin(16.3)withthelaborsupplyanddemandequations,wegethi1wi1zi1ui1(16.4)andhi2wi2zi2ui2,(16.5)whereweexplicitlyincludetheisubscripttoemphasizethathiandwiaretheequilib-riumobservedvaluesforeachcounty.Thesetwoequationsconstituteasimultaneousequationsmodel(SEM),whichhasseveralimportantfeatures.First,givenzi1,zi2,ui1,andui2,thesetwoequationsdeterminehiandwi.(Actually,wemustassumethat12,whichmeansthattheslopesofthesupplyanddemandfunctionsdiffer;seeProblem16.1.)Forthisreason,hiandwiaretheendogenousvariablesinthisSEM.Whataboutzi1andzi2?Becausetheyaredeterminedoutsideofthemodel,weviewthemasexoge-nousvariables.Fromastatisticalstandpoint,thekeyassumptionconcerningzi1andzi2isthattheyarebothuncorrelatedwiththesupplyanddemanderrors,ui1andui2,respec-tively.Theseareexamplesofstructuralerrorsbecausetheyappearinthestructuralequations.Asecondimportantpointisthat,withoutincludingz1andz2inthemodel,thereisnowaytotellwhichequationisthesupplyfunctionandwhichisthedemandfunction.Whenz1representsmanufacturingwage,economicreasoningtellsusthatitisafactorinagriculturallaborsupplybecauseitisameasureoftheopportunitycostofworkinginagriculture;whenz2standsforagriculturallandarea,productiontheoryimpliesthatitappearsinthelabordemandfunction.Therefore,weknowthat(16.4)representslaborsupplyand(16.5)representslabordemand.Ifz1andz2arethesame—forexample,averageeducationlevelofadultsinthecounty,whichcanaffectbothsupplyanddemand—thentheequationslookidentical,andthereisnohopeofestimatingeither503\nPart3AdvancedTopicsone.Inanutshell,thisillustratestheidentificationprobleminsimultaneousequationsmodels,whichwewilldiscussmoregenerallyinSection16.3.ThemostconvincingexamplesofSEMshavethesameflavorassupplyanddemandexamples.Eachequationshouldhaveabehavioral,ceterisparibusinterpreta-tiononitsown.Becauseweonlyobserveequilibriumoutcomes,specifyinganSEMrequiresustoasksuchcounterfactualquestionsas:Howmuchlaborwouldworkersprovideifthewageweredifferentfromitsequilibriumvalue?Example16.1demon-stratesthisfeature.EXAMPLE16.1(MurderRatesandSizeofthePoliceForce)Citiesoftenwanttodeterminehowmuchadditionallawenforcementwilldecreasetheirmurderrates.Asimplecross-sectionalmodeltoaddressthisquestionismurdpc1polpc1011incpcu1,(16.6)wheremurdpcismurderspercapita,polpcisnumberofpoliceofficerspercapita,andincpcisincomepercapita.(Henceforth,wedonotincludeanisubscript.)Wetakeincomepercapitaasexogenousinthisequation.Inpractice,wewouldincludeotherfactors,suchasageandgenderdistributions,educationlevels,perhapsgeographicvariables,andvariablesthatmeasureseverityofpunishment.Tofixideas,weconsiderequation(16.6).Thequestionwehopetoansweris:Ifacityexogenouslyincreasesitspoliceforce,willthatincrease,onaverage,lowerthemurderrate?Ifwecouldexogenouslychoosepoliceforcesizesforarandomsampleofcities,wecouldestimate(16.6)byOLS.Certainlywecannotrunsuchanexperiment.Butcanwethinkofpoliceforcesizeasbeingexogenouslydetermined,anyway?Probablynot.Acity’sspendingonlawenforce-mentisatleastpartlydeterminedbyitsexpectedmurderrate.Toreflectthis,wepostu-lateasecondrelationship:polpc2murdpc20otherfactors.(16.7)Weexpectthat20:otherfactorsbeingequal,citieswithhigher(expected)murderrateswillhavemorepoliceofficerspercapita.Oncewespecifytheotherfactorsin(16.7),wehaveatwo-equationsimultaneousequationsmodel.Wearereallyonlyinterestedinequa-tion(16.6),but,aswewillseeinSection16.3,weneedtoknowpreciselyhowthesecondequationisspecifiedinordertoestimatethefirst.Finally,noticethat(16.7)describesbehaviorbycityofficials,while(16.6)describestheactionsofpotentialmurderers.Thisgiveseachequationaclearceterisparibusinterpreta-tion,whichmakesequations(16.6)and(16.7)anappropriatesimultaneousequationsmodel.WenextgiveanexampleofaninappropriateuseofSEMs.504\nChapter16SimultaneousEquationsModelsEXAMPLE16.2(HousingExpendituresandSaving)Supposethat,forarandomhouseholdinthepopulation,weassumethatannualhousingexpendituresandsavingarejointlydeterminedbyhousing1saving1011inc12educ13ageu1(16.8)andsaving2housing2021inc22educ23ageu2,(16.9)whereincisannualincomeandeducandagearemeasuredinyears.Initially,itmayseemthattheseequationsareasensiblewaytoviewhowhousingandsavingexpendituresaredetermined.Butwehavetoask:Whatvaluewouldoneoftheseequationsbewithouttheother?Neitherhasapreciseceterisparibusinterpretationbecausehousingandsavingarechosenbythesamehousehold.Itmakesnosensetoaskthefollowingquestion:Ifsavingwereexogeneouslychanged,howwouldthataffecthousing?Anymodelbasedoneco-nomicprinciples,particularlyutilitymaximization,wouldhavehouseholdsoptimallychoos-inghousingandsavingasfunctionsofincandtherelativepricesofhousingandsaving.Thevariableseducandagewouldaffectpreferencesforconsumption,saving,andrisk.Therefore,housingandsavingwouldeachbefunctionsofincome,education,age,andothervariablesthataffecttheutilitymaximizationproblem(suchasdifferentratesofreturnonhousingandothersaving).EvenifwedecidedthattheSEMin(16.8)and(16.9)madesense,thereisnowaytoestimatetheparameters.(WediscussthisproblemmoregenerallyinSection16.3.)Thetwoequationsareindistinguishable,unlessweassumethatincome,education,orageappearsinoneequationbutnottheother,whichwouldmakenosense.ThoughthismakesapoorSEMexample,wemightbeinterestedintestingwhether,otherfactorsbeingfixed,thereisatradeoffbetweenhousingexpendituresandsaving.Butthenwewouldjustestimate,say,(16.8)byOLS,unlessthereisanomittedvariableormea-surementerrorproblem.Example16.2hasthecharacteristicsoftoomanySEMapplications.Thekeyfea-tureisthatbothequationsrepresentthebehaviorofthesameeconomicagent,andsoneitherequationcanstandonitsown.Bycontrast,supplyanddemandexamplesQUESTION16.1andExample16.1havenaturalceterispari-businterpretations.Basiceconomicrea-PindyckandRubinfeld(1992,Section11.6)describeamodelofsoning,supportedinsomecasesbysimpleadvertisingwheremonopolisticfirmschooseprofitmaximizinglev-elsofpriceandadvertisingexpenditures.Doesthismeanweshouldmodels,canhelpususeSEMsintelligentlyuseanSEMtomodelthesevariablesatthefirmlevel?(andknowwhennottouseanSEM).505\nPart3AdvancedTopics16.2SIMULTANEITYBIASINOLSItisusefultosee,inasimplemodel,thatanexplanatoryvariablethatisdeterminedsimultaneouslywiththedependentvariableisgenerallycorrelatedwiththeerrorterm,whichleadstobiasandinconsistencyinOLS.Weconsiderthetwo-equationstructuralmodely11y21z1u1(16.10)y22y12z2u2(16.11)andfocusonestimatingthefirstequation.Thevariablesz1andz2areexogenous,sothateachisuncorrelatedwithu1andu2.Forsimplicity,wesuppresstheinterceptineachequation.Toshowthaty2isgenerallycorrelatedwithu1,wesolvethetwoequationsfory2intermsoftheexogenousvariablesandtheerrorterm.Ifweplugtheright-handsideof(16.10)infory1in(16.11),wegety22(1y21z1u1)2z2u2or(121)y221z12z22u1u2.(16.12)Now,wemustmakeanassumptionabouttheparametersinordertosolvefory2:211.(16.13)Whetherthisassumptionisrestrictivedependsontheapplication.InExample16.1,wethinkthat10and20,whichimplies120;therefore,(16.13)isveryrea-sonableforExample16.1.Providedcondition(16.13)holds,wecandivide(16.12)by(121)andwritey2asy221z122z2v2,(16.14)where2121/(121),222/(121),andv2(2u1u2)/(121).Equation(16.14),whichexpressesy2intermsoftheexogenousvariablesandtheerrorterms,isthereducedformfory2,aconceptweintroducedinChapter15inthecontextofinstrumentalvariablesestimation.Theparameters21and22arecalledreducedformparameters;noticehowtheyarenonlinearfunctionsofthestructuralparame-ters,whichappearinthestructuralequations,(16.10)and(16.11).Thereducedformerror,v2,isalinearfunctionofthestructuralerrorterms,u1andu2.Becauseu1andu2areeachuncorrelatedwithz1andz2,v2isalsouncorrelatedwithz1andz2.Therefore,wecanconsistentlyestimate21and22byOLS,somethingthatisusedfortwostageleastsquaresestimation(whichwereturntointhenextsection).Inaddition,thereducedformparametersaresometimesofdirectinterest,althoughwearefocusinghereonestimatingequation(16.10).506\nChapter16SimultaneousEquationsModelsAreducedformalsoexistsfory1underassumption(16.13);thealgebraissimilartothatusedtoobtain(16.14).Ithasthesamepropertiesasthereducedformequationfory2.Wecanuseequation(16.14)toshowthat,exceptunderspecialassumptions,OLSestimationofequation(16.10)willproducebiasedandinconsistentestimatorsof1and1inequation(16.10).Becausez1andu1areuncorrelatedbyassumption,theissueiswhethery2andu1areuncorrelated.Fromthereducedformin(16.14),weseethaty2andu1arecorrelatedifandonlyifv2andu1arecorrelated(becausez1andz2areassumedexogenous).Butv2isalinearfunctionofu1andu2,soitisgenerallycorre-latedwithu1.Infact,ifweassumethatu1andu2areuncorrelated,thenv2andu1mustbecorrelatedwhenever20.Evenif2equalszero—whichmeansthaty1doesnotappearinequation(16.11)—v2andu1willbecorrelatedifu1andu2arecorrelated.When20andu1andu2areuncorrelated,y2andu1arealsouncorrelated.Thesearefairlystrongrequirements:if20,y2isnotsimultaneouslydeterminedwithy1.Ifweaddzerocorrelationbetweenu1andu2,thisrulesoutomittedvariablesormea-surementerrorinu1thatarecorrelatedwithy2.WeshouldnotbesurprisedthatOLSestimationofequation(16.10)worksinthiscase.Wheny2iscorrelatedwithu1becauseofsimultaneity,wesaythatOLSsuffersfromsimultaneitybias.Obtainingthedirectionofthebiasinthecoefficientsisgenerallycomplicated,aswesawwithomittedvariablesbiasinChapters3and5.Butinsimplemodels,wecandeterminethedirectionofthebias.Forexample,supposethatwesim-plifyequation(16.10)bydroppingz1fromtheequation,andweassumethatu1andu2areuncorrelated.Then,thecovariancebetweeny2andu1is2Cov(y2,u1)Cov(y2,v2)[2/(121)]E(u1)2[2/(121)]1,2where1Var(u1)0.Therefore,theasymptoticbias(orinconsistency)intheOLSestimatorof1hasthesamesignas2/(121).If20and211,theasymp-toticbiasispositive.[Unfortunately,justasinourcalculationofomittedvariablesbiasfromSection3.3,theconclusionsdonotcarryovertomoregeneralmodels.Buttheydoserveasausefulguide.]Forexample,inExample16.1,wethink20and210,whichmeansthattheOLSestimatorof1wouldhaveapositivebias.If10,OLSwould,onaverage,estimateapositiveimpactofmorepoliceonthemurderrate;generally,theestimatorof1isattenuatedtowardzero.IfweapplyOLStoequation(16.6),wearelikelytounderestimatetheeffectivenessofalargerpoliceforce.16.3IDENTIFYINGANDESTIMATINGASTRUCTURALEQUATIONAswesawintheprevioussection,OLSisbiasedandinconsistentwhenappliedtoastructuralequationinasimultaneousequationssystem.InChapter15,welearnedthatthemethodoftwostageleastsquarescanbeusedtosolvetheproblemofendogenousexplanatoryvariables.Wenowshowhow2SLScanbeappliedtoSEMs.Themechanicsof2SLSaresimilartothoseinChapter15.Thedifferenceisthat,becausewespecifyastructuralequationforeachendogenousvariable,wecanimme-507\nPart3AdvancedTopicsdiatelyseewhethersufficientIVsareavailabletoestimateeitherequation.Webeginbydiscussingtheidentificationproblem.IdentificationinaTwo-EquationSystemWementionedthenotionofidentificationinChapter15.WhenweestimateamodelbyOLS,thekeyidentificationconditionisthateachexplanatoryvariableisuncorrelatedwiththeerrorterm.AswedemonstratedinSection16.2,thismajorconditionnolongerholds,ingeneral,forSEMs.However,ifwehavesomeinstrumentalvariables,wecanstillidentify(orconsistentlyestimate)theparametersinanSEMequation,justaswithomittedvariablesormeasurementerror.Beforeweconsiderageneraltwo-equationSEM,itisusefultogainintuitionbyconsideringasimplesupplyanddemandexample.Writethesysteminequilibriumform(thatis,withqsqdqimposed)asq1p1z1u1(16.15)orq2pu2.(16.16)Forconcreteness,letqbepercapitamilkconsumptionatthecountylevel,letpbetheaveragepricepergallonofmilkinthecounty,andletz1bethepriceofcattlefeed,whichweassumeisexogenoustothesupplyanddemandequationsformilk.Thismeansthat(16.15)mustbethesupplyfunction,asthepriceofcattlefeedwouldshiftsupply(10)butnotdemand.Thedemandfunctioncontainsnoobserveddemandshifters.Givenarandomsampleon(q,p,z1),whichoftheseequationscanbeestimated?Thatis,whichisanidentifiedequation?Itturnsoutthatthedemandequation,(16.16),isidentified,butthesupplyequationisnot.ThisiseasytoseebyusingourrulesforIVestimationfromChapter15:wecanusez1asanIVforpriceinequation(16.16).However,becausez1appearsinequation(16.15),wehavenoIVforpriceinthesupplyequation.Intuitively,thefactthatthedemandequationisidentifiedfollowsbecausewehaveanobservedvariable,z1,thatshiftsthesupplyequationwhilenotaffectingthedemandequation.Givenvariationinz1andnoerrors,wecantraceoutthedemandcurve,asshowninFigure16.1.Thepresenceoftheunobserveddemandshifteru2causesustoestimatethedemandequationwitherror,buttheestimatorswillbeconsistent,providedz1isuncorrelatedwithu2.Thesupplyequationcannotbetracedoutbecausetherearenoexogenousobservedfactorsshiftingthedemandcurve.Itdoesnothelpthatthereareunobservedfactorsshiftingthedemandfunction;weneedsomethingobserved.If,asinthelabordemandfunction(16.2),wehaveanobservedexogenousdemandshifter—suchasincomeinthemilkdemandfunction—thenthesupplyfunctionwouldalsobeidenti-fied.508\nChapter16SimultaneousEquationsModelsFigure16.1Shiftingsupplyequationstraceoutthedemandequation.Eachsupplyequationisdrawnforadifferentvalueoftheexogenousvariable,z1.pricedemandequationsupplyequationsquantityTosummarize:Inthesystemof(16.15)and(16.16),itisthepresenceofanexogenousvariableinthesupplyequationthatallowsustoestimatethedemandequation.Extendingtheidentificationdiscussiontoageneraltwo-equationmodelisnotdif-ficult.Writethetwoequationsasy1101y2z11u1(16.17)andy2202y1z22u2,(16.18)wherey1andy2aretheendogenousvariables,andu1andu2aretheerrorterms.Theinterceptinthefirstequationis10,andtheinterceptinthesecondequationis20.Thevariablez1denotesasetofk1exogenousvariablesappearinginthefirstequation:z1(z11,z12,…,z1k1).Similarly,z2isthesetofk2exogenousvariablesinthesecondequa-tion:z2(z21,z22,…,z2k2).Inmanycases,z1andz2willoverlap.Asashorthandform,weusethenotation509\nPart3AdvancedTopicsz1111z1112z12…1k1z1k1orz2221z2122z22…2k2z2k2;thatis,z11standsforallexogenousvariablesinthefirstequation,witheachmultipliedbyacoefficient,andsimilarlyforz22.(Someauthorsusethenotationz11andz22instead.Ifyouhaveaninterestinthematrixalgebraapproachtoeconometrics,seeAppendixE.)Thefactthatz1andz2generallycontaindifferentexogenousvariablesmeansthatwehaveimposedexclusionrestrictionsonthemodel.Inotherwords,weassumethatcertainexogenousvariablesdonotappearinthefirstequationandothersareabsentfromthesecondequation.Aswesawwiththeprevioussupplyanddemandexamples,thisallowsustodistinguishbetweenthetwostructuralequations.Whencanwesolveequations(16.17)and(16.18)fory1andy2(aslinearfunctionsofallexogenousvariablesandthestructuralerrors,u1andu2)?Theconditionisthesameasthatin(16.13),namely,211.TheproofisvirtuallyidenticaltothesimplemodelinSection16.2.Underthisassumption,reducedformsexistfory1andy2.Thekeyquestionis:Underwhatassumptionscanweestimatetheparametersin,say,(16.17)?Thisistheidentificationissue.Therankconditionforidentificationofequation(16.17)iseasytostate.RANKCONDITIONFORIDENTIFICATIONOFASTRUCTURALEQUATIONThefirstequationinatwo-equationsimultaneousequationsmodelisidentifiedifandonlyifthesecondequationcontainsatleastoneexogenousvariable(withanonzerocoefficient)thatisexcludedfromthefirstequation.Thisisthenecessaryandsufficientconditionforequation(16.17)tobeidentified.Theordercondition,whichwediscussedinChapter15,isnecessaryfortherankcondi-tion.Theorderconditionforidentifyingthefirstequationstatesthatatleastoneexoge-nousvariableisexcludedfromthisequation.Theorderconditionistrivialtocheckoncebothequationshavebeenspecified.Therankconditionrequiresmore:atleastoneoftheexogenousvariablesexcludedfromthefirstequationmusthaveanonzeropop-ulationcoefficientinthesecondequation.Thisensuresthatatleastoneoftheexoge-nousvariablesomittedfromthefirstequationactuallyappearsinthereducedformofy2,sothatwecanusethesevariablesasinstrumentsfory2.WecantestthisusingatoranFtest,asinChapter15;someexamplesfollow.Identificationofthesecondequationis,naturally,justthemirrorimageofthestate-mentforthefirstequation.Also,ifwewritetheequationsasinthelaborsupplyanddemandexampleinSection16.1—sothaty1appearsontheleft-handsideinbothequa-tions,withy2ontheright-handside—theidentificationconditionisidentical.EXAMPLE16.3(LaborSupplyofMarried,WorkingWomen)Toillustratetheidentificationissue,considerlaborsupplyformarriedwomenalreadyintheworkforce.Inplaceofthedemandfunction,wewritethewageofferasafunctionofhours510\nChapter16SimultaneousEquationsModelsandtheusualproductivityvariables.Withtheequilibriumconditionimposed,thetwostruc-turalequationsarehours1log(wage)1011educ12age13kidslt6(16.19)14nwifeincu1andlog(wage)2hours2021educ22exper(16.20)223experu2.Thevariableageisthewoman’sage,inyears,kidslt6isthenumberofchildrenlessthansixyearsold,nwifeincisthewoman’snonwageincome(whichincludeshusband’searnings),andeducandexperareyearsofeducationandpriorexperience,respectively.Allvariablesexcepthoursandlog(wage)areassumedtobeexogenous.(Thisisatenuousassumption,aseducmightbecorrelatedwithomittedabilityineitherequation.Butforillustrationpur-poses,weignoretheomittedabilityproblem.)Thefunctionalforminthissystem—wherehoursappearsinlevelformbutwageisinlogarithmicform—ispopularinlaboreconom-ics.Wecanwritethissystemasinequations(16.17)and(16.18)bydefiningy1hoursandy2log(wage).Thefirstequationisthesupplyfunction.Itsatisfiestheorderconditionbecausetwo2exogenousvariables,experandexper,areomittedfromthelaborsupplyequation.Theseexclusionrestrictionsarecrucialassumptions:weareassumingthat,oncewage,education,age,numberofsmallchildren,andotherincomearecontrolledfor,pastexperiencehasnoeffectoncurrentlaborsupply.Onecouldcertainlyquestionthisassumption,butweuseitforillustration.Givenequations(16.19)and(16.20),therankconditionforidentifyingthefirstequation2isthatatleastoneofexperandexperhasanonzerocoefficientinequation(16.20).If220and230,therearenoexogenousvariablesappearinginthesecondequationthatdonotalsoappearinthefirst(educappearsinboth).Wecanstatetherankconditionforiden-tificationof(16.19)equivalentlyintermsofthereducedformforlog(wage),whichislog(wage)2021educ22age23kidslt6(16.21)224nwifeinc25exper26experv2.Foridentification,weneed250or260,somethingwecantestusingastandardFstatistic,aswediscussedinChapter15.Thewageofferequation,(16.20),isidentifiedifatleastoneofage,kidslt6,ornwifeinchasanonzerocoefficientin(16.19).Thisisidenticaltoassumingthatthereducedformforhours—whichhasthesameformastheright-handsideof(16.21)—dependsonatleastoneofage,kidslt6,ornwifeinc.Inspecifyingthewageofferequation,weareassumingthatage,kidslt6,andnwifeinchavenoeffectontheofferedwage,oncehours,education,andexperienceareaccountedfor.Thesewouldbepoorassumptionsifthesevariablessomehowhavedirecteffectsonproductivity,orifwomenarediscriminatedagainstbasedontheirageornumberofsmallchildren.511\nPart3AdvancedTopicsInExample16.3,wetakethepopulationofinteresttobemarriedwomenwhoareintheworkforce(sothatequilibriumhoursarepositive).Thisexcludesthegroupofmar-riedwomenwhochoosenottoworkoutsidethehome.Includingsuchwomeninthemodelraisessomedifficultproblems.Forinstance,ifawomandoesnotwork,wecan-notobserveherwageoffer.WetouchontheseissuesinChapter17;butfornow,wemustthinkofequations(16.19)and(16.20)asholdingonlyforwomenwhohavehours0.EXAMPLE16.4(InflationandOpenness)Romer(1993)proposestheoreticalmodelsofinflationwhichimplythatmore“open”coun-triesshouldhavelowerinflationrates.Hisempiricalanalysisexplainsaverageannualinfla-tionrates(since1973)intermsoftheaverageshareofimportsingrossdomestic(ornational)productsince1973—whichishismeasureofopenness.InadditiontoestimatingthekeyequationbyOLS,heusesinstrumentalvariables.WhileRomerdoesnotspecifybothequationsinasimultaneoussystem,hehasinmindatwo-equationsystem:inf101open11log(pcinc)u1(16.22)open202inf21log(pcinc)22log(land)u2,(16.23)wherepcincis1980percapitaincome,inU.S.dollars(assumedtobeexogenous),andlandisthelandareaofthecountry,insquaremiles(alsoassumedtobeexogenous).Equation(16.22)istheoneofinterest,withthehypothesisthat10.(Moreopeneconomieshavelowerinflationrates.)Thesecondequationreflectsthefactthatthedegreeofopennessmightdependontheaverageinflationrate,aswellasotherfactors.Thevariablelog(pcinc)appearsinbothequations,butlog(land)isassumedtoappearonlyinthesecondequation.TheideaQUESTION16.2isthat,ceterisparibus,asmallercountryislikelytobemoreopen(so220).Ifwehavemoneysupplygrowthsince1973foreachcountry,whichweassumeisexogenous,doesthishelpidentifyequation(16.23)?Usingtheidentificationrulethatwasstatedearlier,equation(16.22)isidentified,provided220.Equation(16.23)isnotidentifiedbecauseitcontainsbothexogenousvariables.Butweareinterestedin(16.22).Estimationby2SLSOncewehavedeterminedthatanequationisidentified,wecanestimateitbytwostageleastsquares.Theinstrumentalvariablesconsistoftheexogenousvariablesappearingineitherequation.EXAMPLE16.5(LaborSupplyofMarried,WorkingWomen)Weusethedataonworking,marriedwomeninMROZ.RAWtoestimatethelaborsupplyequation(16.19)by2SLS.Thefullsetofinstrumentsincludeseduc,age,kidslt6,nwifeinc,2exper,andexper.Theestimatedlaborsupplycurveis512\nChapter16SimultaneousEquationsModelshouˆrs(2,225.66)(1,639.56)log(wage)(183.75)educhouˆrs2,(574.56)1,(470.58)log(wage)1(59.10)educ(16.24)(7.81)age(198.15)kidslt6,10.17)nwifeincn428,(9.38)age(182.93)(6.61)whichshowsthatthelaborsupplycurveslopesupward.Theestimatedcoefficientonlog(wage)hasthefollowinginterpretation:holdingotherfactorsfixed,houˆrs16.4(%wage).Wecancalculatelaborsupplyelasticitiesbymultiplyingbothsidesofthislastequationby100/hours:100(houˆrs/hours)(1,640/hours)(%wage)or%houˆrs(1,640/hours)(%wage),whichimpliesthatthelaborsupplyelasticity(withrespecttowage)issimply1,640/hours.[Theelasticityisnotconstantinthismodelbecausehours,notlog(hours),isthedependentvariablein(16.24).]Attheaveragehoursworked,1,303,theestimatedelasticityis1,640/1,3031.26,whichimpliesagreaterthan1%increaseinhoursworkedgivena1%increaseinwage.Thisisalargeestimatedelasticity.Athigherhours,theelasticitywillbesmaller;atlowerhours,suchashours800,theelasticityisovertwo.Forcomparison,when(16.19)isestimatedbyOLS,thecoefficientonlog(wage)is2.05(se54.88),whichimpliesnolaborsupplyeffectonhoursworked.Toconfirmthatlog(wage)isinfactendogenousin(16.19),wecancarryoutthetestfromSection15.5.Whenweaddthereducedformresidualsvˆ2totheequationandestimatebyOLS,thetsta-tisticonvˆ2is6.61,whichisverysignificant,andsolog(wage)appearstobeendogenous.Thewageofferequation(16.20)canalsobeestimatedby2SLS.Theresultis(log(wageˆ).656)(.00013)hours(.110)educlog(wageˆ)(.338)(.00025)hours(.016)educ(16.25)2(.035)exper(.00071)exper,n428.2(.019)exper(.00045)exper,n428.Thisdiffersfrompreviouswageequationsinthathoursisincludedasanexplanatoryvari-ableand2SLSisusedtoaccountforendogeneityofhours(andweassumeeducandexperareexogenous).Thecoefficientonhoursisstatisticallyinsignificant,whichmeansthatthereisnoevidencethatthewageofferincreaseswithhoursworked.TheothercoefficientsaresimilartowhatwegetbydroppinghoursandestimatingtheequationbyOLS.Estimatingtheeffectofopennessoninflationbyinstrumentalvariablesisalsostraightforward.513\nPart3AdvancedTopicsEXAMPLE16.6(InflationandOpenness)Beforeweestimate(16.22)usingthedatainOPENNESS.RAW,wechecktoseewhetheropenhassufficientpartialcorrelationwiththeproposedIV,log(land).Thereducedformregressionisopˆen(117.08)0(.546)log(pcinc)(7.57)log(land)opˆen1(15.85)(1.493)log(pcinc)(0.81)log(land)2n114,R.449.Thetstatisticonlog(land)isovernineinabsolutevalue,whichverifiesRomer’sassertionthatsmallercountriesaremoreopen.Thefactthatlog(pcinc)issoinsignificantinthisregressionisirrelevant.Estimating(16.22)usinglog(land)asanIVforopengivesinˆf(26.90)(.337)open0(.376)log(pcinc),n114.(16.26)inˆf(15.40)(.144)open(2.015)log(pcinc),n114.Thecoefficientonopenisstatisticallysignifi-QUESTION16.3cantataboutthe1%levelagainstaone-HowwouldyoutestwhetherthedifferencebetweentheOLSandsidedalternative(10).TheeffectisIVestimatesonopenarestatisticallydifferent?economicallyimportantaswell:foreverypercentagepointincreaseintheimportshareofGDP,annualinflationisaboutone-thirdofapercentagepointlower.Forcompar-ison,theOLSestimateis.215(se.095).16.4SYSTEMSWITHMORETHANTWOEQUATIONSSimultaneousequationsmodelscanconsistofmorethantwoequations.Studyinggen-eralidentificationofthesemodelsisdifficultandrequiresmatrixalgebra.Onceanequationinageneralsystemhasbeenshowntobeidentified,itcanbeestimatedby2SLS.IdentificationinSystemswithThreeorMoreEquationsWewilluseathree-equationsystemtoillustratetheissuesthatariseintheidentifica-tionofcomplicatedSEMs.Withinterceptssuppressed,writethemodelasy112y213y311z1u1(16.27)y221y121z122z223z3u2(16.28)y332y231z132z233z334z4u3,(16.29)wheretheygaretheendogenousvariables,andthezjareexogenous.Thefirstsubscriptontheparametersindicatestheequationnumber,whilethesecondindicatesthevari-514\nChapter16SimultaneousEquationsModelsablenumber;weuseforparametersonendogenousvariablesandforparametersonexogenousvariables.Whichoftheseequationscanbeestimated?ShowingthatanequationinanSEMwithmorethantwoequationsisidentifiedisgenerallydifficult,butitiseasytoseewhencertainequationsarenotidentified.Insystem(16.27)through(16.29),wecaneasilyseethat(16.29)fallsintothiscategory.Becauseeveryexogenousvariableappearsinthisequation,wehavenoIVsfory2.Therefore,wecannotconsistentlyesti-matetheparametersofthisequation.ForthereasonswediscussedinSection16.2,OLSestimationwillnotusuallybeconsistent.Whataboutequation(16.27)?Thingslookpromisingbecausez2,z3,andz4areallexcludedfromtheequation—thisisanotherexampleofexclusionrestrictions.Whiletherearetwoendogenousvariablesinthisequation,wehavethreepotentialIVsfory2andy3.Therefore,equation(16.27)passestheordercondition.Forcompleteness,westatetheorderconditionforgeneralSEMs.ORDERCONDITIONFORIDENTIFICATIONAnequationinanySEMsatisfiestheorderconditionforidentificationifthenumberofexcludedexogenousvariablesfromtheequationisatleastaslargeasthenumberofright-handsideendogenousvariables.Thesecondequation,(16.28),alsopassestheorderconditionbecausethereisoneexcludedexogenousvariable,z4,andoneright-handsideendogenousvariable,y1.AswediscussedinChapter15andintheprevioussection,theorderconditionisonlynecessary,notsufficient,foridentification.Forexample,if340,z4appearsnowhereinthesystem,whichmeansitisnotcorrelatedwithy1,y2,ory3.If340,thenthesecondequationisnotidentified,becausez4isuselessasanIVfory1.Thisagainillustratesthatidentificationofanequationdependsonthevaluesoftheparame-ters(whichwecanneverknowforsure)intheotherequations.TherearemanysubtlewaysthatidentificationcanfailincomplicatedSEMs.Toobtainsufficientconditions,weneedtoextendtherankconditionforidentificationintwo-equationsystems.Thisispossible,butitrequiresmatrixalgebra[see,forexample,Wooldridge(1999,Chapter9)].Inmanyapplications,oneassumesthat,unlessthereisobviouslyfailureofidentification,anequationthatsatisfiestheorderconditionisiden-tified.ThenomenclatureonoveridentifiedandjustidentifiedequationsfromChapter15originatedwithSEMs.Intermsoftheordercondition,(16.27)isanoveridentifiedequationbecauseweneedonlytwoIVs(fory2andy3)butwehavethreeavailable(z2,z3,andz4);thereisoneoveridentifyingrestrictioninthisequation.Ingeneral,thenum-berofoveridentifyingrestrictionsequalsthetotalnumberofexogenousvariablesinthesystem,minusthetotalnumberofexplanatoryvariablesintheequation.ThesecanbetestedusingtheoveridentificationtestfromSection15.5.Equation(16.28)isajustidentifiedequation,andthethirdequationisanunidentifiedequation.EstimationRegardlessofthenumberofequationsinanSEM,eachidentifiedequationcanbeesti-matedby2SLS.Theinstrumentsforaparticularequationconsistoftheexogenousvari-515\nPart3AdvancedTopicsablesappearinganywhereinthesystem.Testsforendogeneity,heteroskedasticity,ser-ialcorrelation,andoveridentifyingrestrictionscanbeobtained,justasinChapter15.Itturnsoutthat,whenanysystemwithtwoormoreequationsiscorrectlyspecifiedandcertainadditionalassumptionshold,systemestimationmethodsaregenerallymoreefficientthanestimatingeachequationby2SLS.ThemostcommonsystemestimationmethodinthecontextofSEMsisthreestageleastsquares.Thesemethods,withorwithoutendogenousexplanatoryvariables,arebeyondthescopeofthistext.[See,forexample,Wooldridge(1999,Chapters7and8).]16.5SIMULTANEOUSEQUATIONSMODELSWITHTIMESERIESAmongtheearliestapplicationsofSEMswasestimationoflargesystemsofsimulta-neousequationswhichwereusedtodescribeacountry’seconomy.AsimpleKeynesianmodelofaggregatedemand(thatignoresexportsandimports)isCt01(YtTt)2rtut1(16.30)It01rtut2(16.31)YtCtItGt,(16.32)whereCtisconsumption,Ytisincome,Ttistaxreceipts,rtistheinterestrate,Itisinvestment,andGtisgovernmentspending.[See,forexample,Mankiw(1994,Chapter9).]Forconcreteness,assumetrepresentsyear.Thefirstequationisanaggregateconsumptionfunction,whereconsumptiondependsondisposableincome,theinterestrate,andtheunobservedstructuralerrorut1.Thesecondequationisaverysimpleinvestmentfunction.Equation(16.32)isaniden-titythatisaresultofnationalincomeaccounting:itholdsbydefinition,withouterror.Thus,thereisnosenseinwhichweestimate(16.32);butweneedthisequationtoroundoutthemodel.Becausetherearethreeequationsinthesystem,theremustalsobethreeendoge-nousvariables.Giventhefirsttwoequations,itisclearthatweintendforCtandIttobeendogenous.Inaddition,becauseoftheaccountingidentity,Ytisendogenous.Wewouldassume,atleastinthismodel,thatTt,rt,andGtareexogenous,sothattheyareuncorrelatedwithut1andut2.(Wewilldiscussproblemswiththiskindofassumptionlater.)Ifrtisexogenous,thenOLSestimationofequation(16.31)isnatural.Thecon-sumptionfunction,however,dependsondisposableincome,whichisendogenousbecauseYtis.Wehavetwoinstrumentsavailableunderthemaintainedexogeneityassumptions:TtandGt.Therefore,ifwefollowourprescriptionforestimatingcross-sectionalequations,wewouldestimate(16.30)by2SLSusinginstruments(Tt,Gt,rt).Modelssuchas(16.30)through(16.32)areseldomestimatednow,forseveralgoodreasons.First,itisverydifficulttojustify,atanaggregatelevel,theassumptionthattaxes,interestrates,andgovernmentspendingareexogenous.Taxesclearlydependdirectlyonincome;forexample,withasinglemarginalincometaxratetinyeart,TttYt.Wecaneasilyallowthisbyreplacing(YtTt)with(1t)Ytin(16.30),and516\nChapter16SimultaneousEquationsModelswecanstillestimatetheequationby2SLSifweassumethatgovernmentspendingisexogenous.Wecouldalsoaddthetaxratetotheinstrumentlist,ifitisexogenous.Butaregovernmentspendingandtaxratesreallyexogenous?Theycertainlycouldbeinprinciple,ifthegovernmentsetsspendingandtaxratesindependentlyofwhatishap-peningintheeconomy.Butitisadifficultcasetomakeinreality:governmentspend-inggenerallydependsonthelevelofincome,andathighlevelsofincome,thesametaxreceiptsarecollectedforlowermarginaltaxrates.Inaddition,assumingthatinter-estratesareexogenousisextremelyquestionable.Wecouldspecifyamorerealisticmodelthatincludesmoneydemandandsupply,andtheninterestratescouldbejointlydeterminedwithCt,It,andYt.Butthenfindingenoughexogenousvariablestoidentifytheequationsbecomesquitedifficult(andthefollowingproblemswiththesemodelsstillpertain).Somehavearguedthatcertaincomponentsofgovernmentspending,suchasdefensespending—see,forexample,Hall(1988)andRamey(1991)—areexogenousinavarietyofsimultaneousequationsapplications.Butthisisnotuniversallyagreedupon,andinanycase,defensespendingisnotalwaysappropriatelycorrelatedwiththeendogenousexplanatoryvariables[seeShea(1993)fordiscussionandProblem16.14foranexample].Asecondproblemwithamodelsuchas(16.30)through(16.32)isthatitiscom-pletelystatic.Especiallywithmonthlyorquarterlydata,butevenwithannualdata,weoftenexpectadjustmentlags.(OneargumentinfavorofstaticKeynesian-typemodelsisthattheyareintendedtodescribethelongrunwithoutworryingaboutshort-rundynamics.)Allowingdynamicsisnotverydifficult.Forexample,wecouldaddlaggedincometoequation(16.31):It01rt2Yt1ut2.(16.33)Inotherwords,weaddalaggedendogenousvariable(butnotIt1)totheinvestmentequation.CanwetreatYt1asexogenousinthisequation?Undercertainassumptionsonut2,theanswerisyes.ButwetypicallycallalaggedendogenousvariableinanSEMapredeterminedvariable.Lagsofexogenousvariablesarealsopredetermined.Ifweassumethatut2isuncorrelatedwithcurrentexogenousvariables(whichisstandard)andallpastendogenousandexogenousvariables,thenYt1isuncorrelatedwithut2.Givenexogeneityofrt,wecanestimate(16.33)byOLS.Ifweaddlaggedconsumptionto(16.30),wecantreatCt1asexogenousinthisequationunderthesameassumptionsonut1thatwemadeforut2inthepreviouspara-graph.CurrentdisposableincomeisstillendogenousinCt01(YtTt)2rt3Ct1ut1,(16.34)sowecouldestimatethisequationby2SLSusinginstruments(Tt,Gt,rt,Ct1);ifinvest-mentisdeterminedby(16.33),Yt1shouldbeaddedtotheinstrumentlist.[Toseewhy,use(16.32),(16.33),and(16.34)tofindthereducedformforYtintermsoftheexoge-nousandpredeterminedvariables:Tt,rt,Gt,Ct1,andYt1.BecauseYt1showsupinthisreducedform,itshouldbeusedasanIV.]517\nPart3AdvancedTopicsThepresenceofdynamicsinaggregateSEMsis,atleastforthepurposesoffore-casting,aclearimprovementoverstaticSEMs.Buttherearestillsomeimportantprob-lemswithestimatingSEMsusingaggregatetimeseriesdata,someofwhichwediscussedinChapters11and15.RecallthatthevalidityoftheusualOLSor2SLSinferenceproceduresintimeseriesapplicationshingesonthenotionofweakdepen-dence.Unfortunately,seriessuchasaggregrateconsumption,income,investment,andeveninterestratesseemtoviolatetheweakdependencerequirements.(Inthetermi-nologyofChapter11,theyhaveunitroots.)Theseseriesalsotendtohaveexponentialtrends,althoughthiscanbepartlyovercomebyusingthelogarithmictransformationandassumingdifferentfunctionalforms.Generally,eventhelargesample,letalonethesmallsample,propertiesofOLSand2SLSarecomplicatedanddependentonvariousassumptionswhentheyareappliedtoequationswithI(1)variables.WewillbrieflytouchontheseissuesinChapter18.Anadvanced,generaltreatmentisgivenbyHamilton(1994).DoesthepreviousdiscussionmeanthatSEMsarenotusefullyappliedtotimeseriesdata?Notatall.Theproblemswithtrendsandhighpersistencecanbeavoidedbyspec-ifyingsystemsinfirstdifferencesorgrowthrates.ButoneshouldrecognizethatthisisadifferentSEMthanonespecifiedinlevels.[Forexample,ifwespecifyconsumptiongrowthasafunctionofdisposableincomegrowthandinterestratechanges,thisisdif-ferentfrom(16.30).]Also,aswediscussedearlier,incorporatingdynamicsisnotespe-ciallydifficult.Finally,theproblemoffindingtrulyexogenousvariablestoincludeinSEMsisofteneasierwithdisaggregateddata.Forexample,formanufacturingindus-tries,Shea(1993)describeshowoutput(or,moreprecisely,growthinoutput)inotherindustriescanbeusedasaninstrumentinestimatingsupplyfunctions.Ramey(1991)alsocontainsaconvincinganalysisofestimatingindustrycostfunctionsbyinstrumen-talvariablesusingtimeseriesdata.Thenextexampleshowshowaggregatedatacanbeusedtotestanimportanteco-nomictheory,thepermanentincometheoryofconsumption,usuallycalledtheperma-nentincomehypothesis(PIH).Theapproachusedinthisexampleisnot,strictlyspeaking,basedonasimultaneousequationsmodel,butwecanthinkofconsumptionandincomegrowth(aswellasinterestrates)asbeingjointlydetermined.EXAMPLE16.7(TestingthePermanentIncomeHypothesis)CampbellandMankiw(1990)usedinstrumentalvariablesmethodstotestvariousversionsofthepermanentincomehypothesis.Wewillusetheannualdatafrom1959through1995inCONSUMP.RAWtomimiconeoftheiranalyses.CampbellandMankiwusedquarterlydatarunningthrough1985.OneequationestimatedbyCampbellandMankiw(usingournotation)isgct01gyt2r3tut,(16.35)wheregctlog(ct)isannualgrowthinrealpercapitaconsumption(excludingdurables),gytisgrowthinrealdisposableincome,andr3tisthe(expost)realinterestrateasmea-suredbythereturnonthree-month,T-billrates:r3ti3tinft,wheretheinflationrateis518\nChapter16SimultaneousEquationsModelsbasedontheconsumerpriceindex.Thegrowthratesofconsumptionanddisposableincomearenottrending,andtheyareweaklydependent;wewillassumethisisthecaseforr3taswell,sothatwecanapplystandardasymptotictheory.Thekeyfeatureofequation(16.35)isthatthePIHimpliesthattheerrortermuthasazeromeanconditionalonallinformationobservedattimet1orearlier:E(utIt1)0.However,utisnotnecessarilyuncorrelatedwithgytorr3t;atraditionalwaytothinkaboutthisisthatthesevariablesarejointlydetermined,butwearenotwritingdownafullthree-equationsystem.Becauseutisuncorrelatedwithallvariablesdatedt1orearlier,validinstrumentsforestimating(16.35)arelaggedvaluesofgc,gy,andr3(andlagsofotherobservablevari-ables,butwewillnotusethosehere).Whatarethehypothesesofinterest?ThepureformofthePIHhas120.CampbellandMankiwarguethat1ispositiveifsomefrac-tionofthepopulationconsumescurrentincome,ratherthanpermanentincome.ThePIHwithanonconstantrealinterestrateimpliesthat20.Whenweestimate(16.35)by2SLS,usinginstrumentsgc1,gy1,andr31,weobtaingˆct(.0081)(.586)gyt(.00027)r3tgˆct(.0032)(.135)gyt(.00076)r3t(16.36)2n35,R.678.Therefore,thepureformofthePIHisstronglyrejectedbecausethecoefficientongyiseco-nomicallylarge(a1%increaseindisposableincomeincreasesconsumptionbyover.5%)andstatisticallysignificant(t4.34).Bycontrast,therealinterestratecoefficientisverysmallandstatisticallyinsignificant.ThesefindingsarequalitativelythesameasCampbellandMankiw’s.ThePIHalsoimpliesthattheerrors{ut}areseriallyuncorrelated.After2SLSestimation,weobtaintheresiduals,uˆt,andincludeuˆt1asanadditionalexplanatoryvariablein(16.36);itactsasitsowninstrument(seeSection15.7).Thecoefficientonuˆt1isˆ.187(se.133),sothereissomeevidenceofpositiveserialcorrelation,althoughnotatthe5%sig-nificancelevel.CampbellandMankiw(1990)discusswhy,withtheavailablequarterlydata,positiveserialcorrelationmightbefoundintheerrorsevenifthePIHholds;someofthoseconcernscarryovertoannualdata.UsinggrowthratesoftrendingorI(1)QUESTION16.4variablesinSEMsisfairlycommoninSupposethatforaparticularcityyouhavemonthlydataonpertimeseriesapplications.Forexample,capitaconsumptionoffish,percapitaincome,thepriceoffish,andShea(1993)estimatesindustrysupplythepricesofchickenandbeef;incomeandchickenandbeefpricescurvesspecifiedintermsofgrowthrates.areexogenous.AssumethatthereisnoseasonalityinthedemandIfastructuralmodelcontainsatimefunctionforfish,butthereisinthesupplyoffish.Howcanyouusetrend—whichmaycaptureexogenous,thisinformationtoestimateaconstantelasticitydemand-for-fishequation?Specifyanequationanddiscussidentification.(Hint:Youtrendingfactorsthatarenotdirectlymod-shouldhaveeleveninstrumentalvariablesforthepriceoffish.)eled—thenthetrendactsasitsownIV.519\nPart3AdvancedTopics16.6SIMULTANEOUSEQUATIONSMODELSWITHPANELDATASimultaneousequationsmodelsalsoariseinpaneldatacontexts.Forexample,wecanimagineestimatinglaborsupplyandwageofferequations,asinExample16.3,foragroupofpeopleworkingoveragivenperiodoftime.Inadditiontoallowingforsimul-taneousdeterminationofvariableswithineachtimeperiod,wecanallowforunob-servedeffectsineachequation.Inalaborsupplyfunction,itwouldbeusefultoallowanunobservedtasteforleisurethatdoesnotchangeovertime.ThebasicapproachtoestimatingSEMswithpaneldatainvolvestwosteps:(1)eliminatetheunobservedeffectsfromtheequationsofinterestusingthefixedeffectstransformationorfirstdifferencing;(2)findinstrumentalvariablesfortheendogenousvariablesinthetransformedequation.Thiscanbeverychallenging,becauseforacon-vincinganalysisweneedtofindinstrumentsthatchangeovertime.Toseewhy,writeanSEMforpaneldataasyit11yit2zit11ai1uit1(16.37)yit22yit1zit22ai2uit2,(16.38)whereidenotescrosssection,tdenotestimeperiod,andzit11orzit22denoteslinearfunctionsofasetofexogenousexplanatoryvariablesineachequation.Themostgen-eralanalysisallowstheunobservedeffects,ai1andai2,tobecorrelatedwithallexplanatoryvariables,eventheelementsinz.However,weassumethattheidiosyn-craticstructuralerrors,uit1anduit2,areuncorrelatedwiththezinbothequationsandacrossalltimeperiods;thisisthesenseinwhichthezareexogenous.Exceptunderspe-cialcircumstances,yit2iscorrelatedwithuit1,andyit1iscorrelatedwithuit2.Supposeweareinterestedinequation(16.37).WecannotestimateitbyOLS,asthecompositeerrorai1uit1ispotentiallycorrelatedwithallexplanatoryvariables.Supposewedifferenceovertimetoremovetheunobservedeffect,ai1:yit11yit2zit11uit1.(16.39)(Asusualwithdifferencingortime-demeaning,wecanonlyestimatetheeffectsofvari-ablesthatchangeovertimeforatleastsomecross-sectionalunits.)Now,theerrorterminthisequationisuncorrelatedwithzit1byassumption.Butyit2anduit1arepossi-blycorrelated.Therefore,weneedanIVforyit2.Aswiththecaseofpurecross-sectionalorpuretimeseriesdata,possibleIVscomefromtheotherequation:elementsinzit2thatarenotalsoinzit1.Inpractice,weneedtime-varyingelementsinzit2thatarenotalsoinzit1.Thisisbecauseweneedaninstru-mentforyit2,andachangeinavariablefromoneperiodtothenextisunlikelytobehighlycorrelatedwiththelevelofexogenousvariables.Infact,ifwedifference(16.38),weseethatthenaturalIVsforyit2arethoseelementsinzit2thatarenotalsoinzit1.Asanexampleoftheproblemsthatcanarise,considerapaneldataversionofthelaborsupplyfunctioninExample16.3.Afterdifferencing,supposewehavetheequationhoursit01log(wageit)(otherfactorsit),520\nChapter16SimultaneousEquationsModelsandwewishtouseexperitasaninstrumentforlog(wageit).Theproblemisthat,sincewearelookingatpeoplewhoworkineverytimeperiod,experit1foralliandt.(Eachpersongetsanotheryearofexperienceafterayearpasses.)WecannotuseanIVthatisthesamevalueforalliandt,andsowemustlookelsewhere.Often,participationinanexperimentalprogramcanbeusedtoobtainIVsinpaneldatacontexts.InExample15.10,weusedreceiptofjobtraininggrantsasanIVforthechangeinhoursoftrainingindeterminingtheeffectsofjobtrainingonworkerpro-ductivity.Infact,wecouldviewthatinanSEMcontext:jobtrainingandworkerpro-ductivityarejointlydetermined,butreceivingajobtraininggrantisexogenousinequation(15.57).Wecansometimescomeupwithclever,convincinginstrumentalvariablesinpaneldataapplications,asthefollowingexampleillustrates.EXAMPLE16.8(EffectofPrisonPopulationonViolentCrimeRates)Inordertoestimatethecausaleffectofprisonpopulationincreasesoncrimeratesatthestatelevel,Levitt(1996)usedinstancesofprisonovercrowdinglitigationasinstrumentsforthegrowthinprisonpopulation.TheequationLevittestimatedisinfirstdifferences;wecanwriteanunderlyingfixedeffectsmodelaslog(crimeit)t1log(prisonit)zit11ai1uit1,(16.40)wheretdenotesdifferenttimeintercepts,andcrimeandprisonaremeasuredper100,000people.(Theprisonpopulationvariableismeasuredonthelastdayofthepreviousyear.)Thevectorzit1containsothercontrolslistedinthepaperbyLevitt,includingmeasuresofpolicepercapita,incomepercapita,unemploymentrate,race,andmetropolitanandagedistributionproportions.Differencing(16.40)givestheequationestimatedbyLevitt:log(crimeit)t1log(prisonit)zit11uit1.(16.41)Simultaneitybetweencrimeratesandprisonpopulation,ormorepreciselyinthegrowthrates,makesOLSestimationof(16.41)generallyinconsistent.UsingtheviolentcrimerateandasubsetofthedatafromLevitt(inPRISON.RAW,fortheyears1980through1993,for5114714totalobservations),weobtainthepooledOLSestimateof1whichis.179(se.048).Wealsoestimate(16.41)bypooled2SLS,wheretheinstrumentsforlog(prison)aretwobinaryvariables,oneeachforwhetherafinaldecisionwasreachedonovercrowdinglitigationinthecurrentyearorintheprevioustwoyears.Thepooled2SLSestimateof1is1.020(se.366).Therefore,the2SLSestimatedeffectismuchlarger;notsurprisingly,itismuchlessprecise,too.Levitt(1996)foundsimilarresultswhenusingalongertimeperiod(butwithearlyobservationsmissingforsomestates)andmoreinstru-ments.521\nPart3AdvancedTopicsAnalternativeapproachtoestimatingSEMswithpaneldataistousethefixedeffectstransformationandthentoapplyanIVtechniquesuchaspooled2SLS.Asim-pleprocedureistoestimatethetime-demeanedequationbypooled2SLS,whichwouldlooklikeyit11yit2zit11uit1,t1,2,…,T,(16.42)wherezit1andzit2areIVs.Thisisequivalenttousing2SLSinthedummyvariablefor-mulation,wheretheunit-specificdummyvariablesactastheirowninstruments.AyresandLevitt(1998)applied2SLStoatime-demeanedequationtoestimatetheeffectofLojackelectronictheftpreventiondevicesoncartheftratesincities.If(16.42)isesti-mateddirectly,thenthedfneedstobecorrectedtoN(T1)k1,wherek1isthetotalnumberofelementsin1and1.Includingunit-specificdummyvariablesandapply-ingpooled2SLStotheoriginaldataproducesthecorrectdf.SUMMARYSimultaneousequationsmodelsareappropriatewheneachequationinthesystemhasaceterisparibusinterpretation.Goodexamplesarewhenseparateequationsdescribedifferentsidesofamarketorthebehavioralrelationshipsofdifferenteconomicagents.Supplyanddemandexamplesareleadingcases,buttherearemanyotherapplicationsofSEMsineconomicsandthesocialsciences.AnimportantfeatureofSEMsisthat,byfullyspecifyingthesystem,itisclearwhichvariablesareassumedtobeexogenousandwhichonesappearineachequation.Givenafullsystem,weareabletodeterminewhichequationscanbeidentified(thatis,canbeestimated).Intheimportantcaseofatwo-equationsystem,identificationof(say)thefirstequationiseasytostate:theremustbeatleastoneexogenousvariableexcludedfromthefirstequationthatappearswithanonzerocoefficientinthesecondequation.Asweknowfrompreviouschapters,OLSestimationofanequationthatcontainsanendogenousexplanatoryvariablegenerallyproducesbiasedandinconsistentesti-mators.Instead,2SLScanbeusedtoestimateanyidentifiedequationinasystem.Moreadvancedsystemmethodsareavailable,buttheyarebeyondthescopeofourtreatment.Thedistinctionbetweenomittedvariablesandsimultaneityinapplicationsisnotalwayssharp.Bothproblems,nottomentionmeasurementerror,canappearinthesameequation.Agoodexampleisthelaborsupplyofmarriedwomen.Yearsofeducation(educ)appearsinboththelaborsupplyandthewageofferfunctions[seeequations(16.20)and(16.21)].Ifomittedabilityisintheerrortermofthelaborsupplyfunction,thenwageandeducationarebothendogenous.Theimportantthingisthatanequationestimatedby2SLScanstandonitsown.SEMscanbeappliedtotimeseriesdataaswell.AswithOLSestimation,wemustbeawareoftrending,integratedprocessesinapplying2SLS.ProblemssuchasserialcorrelationcanbehandledasinSection15.7.Wealsogaveanexampleofhowtoesti-mateanSEMusingpaneldata,wheretheequationisfirstdifferencedtoremovetheunobservedeffect.Then,wecanestimatethedifferencedequationbypooled2SLS,just522\nChapter16SimultaneousEquationsModelsasinChapter15.Alternatively,insomecaseswecanusetime-demeaningofallvari-ables,includingtheIVs,andthenapplypooled2SLS;thisisidenticaltoputtingindummiesforeachcross-sectionalobservationandusing2SLS,wherethedummiesactastheirowninstruments.SEMapplicationswithpaneldataareverypowerful,astheyallowustocontrolforunobservedheterogeneitywhiledealingwithsimultaneity.Theyarebecomingmoreandmorecommonandarenotespeciallydifficulttoestimate.KEYTERMSEndogenousVariablesReducedFormExclusionRestrictionsReducedFormErrorExogenousVariablesReducedFormParametersIdentifiedEquationSimultaneityJustIdentifiedEquationSimultaneityBiasLaggedEndogenousVariableSimultaneousEquationsModel(SEM)OrderConditionStructuralEquationOveridentifiedEquationStructuralErrorsPredeterminedVariableStructuralParametersRankConditionUnidentifiedEquationPROBLEMS16.1Writeatwo-equationsystemin“supplyanddemandform,”thatis,withthesamevariabley1(typically,“quantity”)appearingontheleft-handside:y11y21z1u1y12y22z2u2.(i)If10or20,explainwhyareducedformexistsfory1.(Remember,areducedformexpressesy1asalinearfunctionoftheexogenousvariablesandthestructuralerrors.)If10and20,findthereducedformfory2.(ii)If10,20,and12,findthereducedformfory1.Doesy2haveareducedforminthiscase?(iii)Isthecondition12likelytobemetinsupplyanddemandexam-ples?Explain.16.2Letcorndenotepercapitaconsumptionofcorninbushels,atthecountylevel,letpricebethepriceperbushelofcorn,letincomedenotepercapitacountyincome,andletrainfallbeinchesofrainfallduringthelastcorn-growingseason.Thefollowingsimultaneousequationsmodelimposestheequilibriumconditionthatsupplyequalsdemand:corn1price1incomeu1corn2price2rainfallu2.Whichisthesupplyequationandwhichisthedemandequation?Explain.523\nPart3AdvancedTopics16.3InProblem3.3ofChapter3,weestimatedanequationtotestforatradeoffbetweenminutesperweekspentsleeping(sleep)andminutesperweekspentworking(totwrk)forarandomsampleofindividuals.Wealsoincludededucationandageintheequation.Becausesleepandtotwrkarejointlychosenbyeachindividual,istheesti-matedtradeoffbetweensleepingandworkingsubjecttoa“simultaneitybias”criticism?Explain.16.4SupposethatannualearningsandalcoholconsumptionaredeterminedbytheSEMlog(earnings)01alcohol2educu1alcohol01log(earnings)2educ3log(price)u2,wherepriceisalocalpriceindexforalcohol,whichincludesstateandlocaltaxes.Assumethateducandpriceareexogenous.If1,2,1,2,and3arealldifferentfromzero,whichequationisidentified?Howwouldyouestimatethatequation?16.5Asimplemodeltodeterminetheeffectivenessofcondomusageonreducingsex-uallytransmitteddiseasesamongsexuallyactivehighschoolstudentsisinfrate01conuse2percmale3avginc4cityu1,whereinfrateisthepercentofsexuallyactivestudentswhohavecontractedvenerealdisease,conuseisthepercentageofboyswhoclaimtoregularlyusecondoms,avgincisaveragefamilyincome,andcityisadummyvariableindicatingwhetheraschoolisinacity;themodelisattheschoollevel.(i)Interpretingtheprecedingequationinacausal,ceterisparibusfashion,whatshouldbethesignof1?(ii)Whymightinfrateandconusebejointlydetermined?(iii)Ifcondomusageincreaseswiththerateofvenerealdisease,sothat10intheequationconuse01infrateotherfactors,whatisthelikelybiasinestimating1byOLS?(iv)Letcondisbeabinaryvariableequaltounityifaschoolhasaprogramtodistributecondoms.Explainhowthiscanbeusedtoestimate1(andtheotherbetas)byIV.Whatdowehavetoassumeaboutcondisineachequation?16.6Consideralinearprobabilitymodelforwhetheremployersofferapensionplanbasedonthepercentageofworkersbelongingtoaunion,aswellasotherfactors:pension01percunion2avgage3avgeduc4percmale5percmarru1.(i)Whymightpercunionbejointlydeterminedwithpension?(ii)Supposethatyoucansurveyworkersatfirmsandcollectinformationonworkers’families.CanyouthinkofinformationthatcanbeusedtoconstructanIVforpercunion?(iii)HowwouldyoutestwhetheryourvariableisatleastareasonableIVcandidateforpercunion?524\nChapter16SimultaneousEquationsModels16.7Foralargeuniversity,youareaskedtoestimatethedemandforticketstowomen’sbasketballgames.Youcancollecttimeseriesdataover10seasons,foratotalofabout150observations.OnepossiblemodelislATTENDt01lPRICEt2WINPERCt3RIVALt4WEEKENDt5tut,wherePRICEtisthepriceofadmission,probablymeasuredinrealterms—say,deflat-ingbyaregionalconsumerpriceindex—WINPERCtistheteam’scurrentwinningper-centage,RIVALtisadummyvariableindicatingagameagainstarival,andWEEKENDtisadummyvariableindicatingwhetherthegameisonaweekend.Theldenotesnat-urallogarithm,sothatthedemandfunctionhasaconstantpriceelasticity.(i)Whyisitagoodideatohaveatimetrendintheequation?(ii)Thesupplyofticketsisfixedbythestadiumcapacity;assumethishasnotchangedoverthe10years.Thismeansthatquantitysupplieddoesnotvarywithprice.Doesthismeanthatpriceisnecessarilyexogenousinthedemandequation?(Hint:Theanswerisno.)(iii)Supposethatthenominalpriceofadmissionchangesslowly—say,atthebeginningofeachseason.Theathleticofficechoosespricebasedpartlyonlastseason’saverageattendance,aswellaslastseason’steamsuccess.Underwhatassumptionsislastseason’swinningpercentage(SEASPERCt1)avalidinstrumentalvariableforlPRICEt?(iv)Doesitseemreasonabletoincludethe(logofthe)realpriceofmen’sbasketballgamesintheequation?Explain.Whatsigndoeseconomictheorypredictforitscoefficient?Canyouthinkofanothervariablerelatedtomen’sbasketballthatmightbelonginthewomen’sattendanceequation?(v)Ifyouareworriedthatsomeoftheseries,particularlylATTENDandlPRICE,haveunitroots,howmightyouchangetheestimatedequation?(vi)Ifsomegamesaresoldout,whatproblemsdoesthiscauseforestimat-ingthedemandfunction?(Hint:Ifagameissoldout,doyouneces-sarilyobservethetruedemand?)16.8Howbigistheeffectofper-studentschoolexpendituresonlocalhousingvalues?LetHPRICEbethemedianhousingpriceinaschooldistrictandletEXPENDbeper-studentexpenditures.Usingpaneldatafortheyears1992,1994,and1996,wepostu-latethemodellHPRICEitt1lEXPENDit2lPOLICEit3lMEDINCit4PROPTAXitai1uit1,wherePOLICEitispercapitapoliceexpenditures,MEDINCitismedianincome,andPROPTAXitisthepropertytaxrate;ldenotesnaturallogarithm.Expendituresandhous-ingpricearesimultaneouslydeterminedbecausethevalueofhomesdirectlyaffectstherevenuesavailableforfundingschools.Supposethat,in1994,thewayschoolswerefundedwasdrasticallychanged:ratherthanbeingraisedbylocalpropertytaxes,schoolfundingwaslargelydeterminedatthestatelevel.LetlSTATEALLitdenotethelogofthestateallocationfordistrictiinyeart,525\nPart3AdvancedTopicswhichisexogenousintheprecedingequation,oncewecontrolforexpendituresandadistrictfixedeffect.Howwouldyouestimatethej?COMPUTEREXERCISES16.9UseSMOKE.RAWforthisexercise.(i)Amodeltoestimatetheeffectsofsmokingonannualincome(perhapsthroughlostworkdaysduetoillness,orproductivityeffects)is2log(income)01cigs2educ3age4ageu1,wherecigsisnumberofcigarettessmokedperday,onaverage.Howdoyouinterpret1?(ii)Toreflectthefactthatcigaretteconsumptionmightbejointlydeter-minedwithincome,ademandforcigarettesequationis2cigs01log(income)2educ3age4age5log(cigpric)6restaurnu2,wherecigpricisthepriceofapackofcigarettes(incents),andrestaurnisabinaryvariableequaltounityifthepersonlivesinastatewithrestaurantsmokingrestrictions.Assumingtheseareexogenoustotheindividual,whatsignswouldyouexpectfor5and6?(iii)Underwhatassumptionistheincomeequationfrompart(i)identified?(iv)EstimatetheincomeequationbyOLSanddiscusstheestimateof1.(v)Estimatethereducedformforcigs.(Recallthatthisentailsregressingcigsonallexogenousvariables.)Arelog(cigpric)andrestaurnsignifi-cantinthereducedform?(vi)Nowestimatetheincomeequationby2SLS.Discusshowtheestimateof1compareswiththeOLSestimate.(vii)Doyouthinkthatcigarettepricesandrestaurantsmokingrestrictionsareexogenousintheincomeequation?16.10UseMROZ.RAWforthisexercise.(i)ReestimatethelaborsupplyfunctioninExample16.5,usinglog(hours)asthedependentvariable.Comparetheestimatedelasticity(whichisnowconstant)totheestimateobtainedfromequation(16.24)attheaveragehoursworked.(ii)Inthelaborsupplyequationfrompart(i),alloweductobeendogenousbecauseofomittedability.UsemotheducandfatheducasIVsforeduc.Remember,younowhavetwoendogenousvariablesintheequation.(iii)Testtheoveridentifyingrestrictionsinthe2SLSestimationfrompart(ii).DotheIVspassthetest?16.11UsethedatainOPENNESS.RAWforthisexercise.(i)Becauselog(pcinc)isinsignificantinboth(16.22)andthereducedformforopen,dropitfromtheanalysis.Estimate(16.22)byOLSandIVwithoutlog(pcinv).Doanyimportantconclusionschange?526\nChapter16SimultaneousEquationsModels(ii)Stillleavinglog(pcinc)outoftheanalysis,islandorlog(land)abetterinstrumentforopen?(Hint:Regressopenoneachoftheseseparatelyandjointly.)(iii)Nowreturnto(16.22).Addthedummyvariableoiltotheequationandtreatitasexogenous.EstimatetheequationbyIV.Doesbeinganoilproducerhaveaceterisparibuseffectoninflation?16.12UsethedatainCONSUMP.RAWforthisexercise.(i)InExample16.7,usethemethodfromSection15.5totestthesingleoveridentifyingrestrictioninestimating(16.35).Whatdoyoucon-clude?(ii)CampbellandMankiw(1990)usesecondlagsofallvariablesasIVsbecauseofpotentialdatameasurementproblemsandinformationallags.Reestimate(16.35),usingonlygct2,gyt2,andr3t2asIVs.Howdotheestimatescomparewiththosein(16.36)?(iii)RegressgytontheIVsfrompart(ii)andtestwhethergytissufficientlycorrelatedwiththem.Whyisthisimportant?16.13UsetheEconomicReportofthePresident(1998orlater)toupdatethedatainCONSUMP.RAW,atleastthrough1996.Reestimateequation(16.35).Doanyimpor-tantconclusionschange?16.14UsethedatainCEMENT.RAWforthisexercise.(i)Astatic(inverse)supplyfunctionforthemonthlygrowthincementprice(gprc)asafunctionofgrowthinquantity(gcem)issgprct1gcemt01grprcpet2febt…12dectut,wheregrprcpet(growthinthepriceofpetroleum)isassumedtobeexogenousandfeb,…,decaremonthlydummyvariables.Whatsignsdoyouexpectfor1and1?EstimatetheequationbyOLS.Doesthesupplyfunctionslopeupward?(ii)ThevariablegrdefsisthemonthlygrowthinrealdefensespendingintheUnitedStates.WhatdoyouneedtoassumeaboutgrdefsforittobeagoodIVforgcem?Testwhethergcemispartiallycorrelatedwithgrdefs.(Donotworryaboutpossibleserialcorrelationinthereducedform.)CanyouusegrdefsasanIVinestimatingthesupplyfunction?(iii)Shea(1993)arguesthatthegrowthinoutputofresidential(grres)andnonresidential(grnon)constructionarevalidinstrumentsforgcem.Theideaisthatthesearedemandshiftersthatshouldberoughlyuncorre-slatedwiththesupplyerrorut.Testwhethergcemispartiallycorrelatedwithgrresandgrnon;again,donotworryaboutserialcorrelationinthereducedform.(iv)Estimatethesupplyfunction,usinggrresandgrnonasIVsforgcem.Whatdoyouconcludeaboutthestaticsupplyfunctionforcement?[Thedynamicsupplyfunctionis,apparently,upwardsloping;seeShea(1993).]16.15RefertoExample13.9andthedatainCRIME4.RAW.527\nPart3AdvancedTopics(i)Supposethat,afterdifferencingtoremovetheunobservedeffect,youthinklog(polpc)issimultaneouslydeterminedwithlog(crmrte);inparticular,increasesincrimeareassociatedwithincreasesinpoliceofficers.Howdoesthishelptoexplainthepositivecoefficientonlog(polpc)inequation(13.33)?(ii)Thevariabletaxpcisthetaxescollectedperpersoninthecounty.Doesitseemreasonabletoexcludethisfromthecrimeequation?(iii)Estimatethereducedformforlog(polpc)usingpooledOLS,includ-ingthepotentialIV,log(taxpc).Doesitlooklikelog(taxpc)isagoodIVcandidate?Explain.(iv)Supposethat,inseveraloftheyears,thestateofNorthCarolinaawardedgrantstosomecountiestoincreasethesizeoftheircountypoliceforce.Howcouldyouusethisinformationtoestimatetheeffectofadditionalpoliceofficersonthecrimerate?528\nd7/14/998:28PMPage529ChapterSeventeenLimitedDependentVariableModelsandSampleSelectionCorrectionsnChapter7,westudiedthelinearprobabilitymodel,whichissimplyanapplicationIofthemultipleregressionmodeltoabinarydependentvariable.Abinarydependentvariableisanexampleofalimiteddependentvariable(LDV).AnLDVisbroadlydefinedasadependentvariablewhoserangeofvaluesissubstantivelyrestricted.Abinaryvariabletakesononlytwovalues,zeroandone.Wehaveseenseveralotherexamplesoflimiteddependentvariables:participationpercentageinapensionplanmustbebetweenzeroand100,thenumberoftimesanindividualisarrestedinagivenyearisanonnegativeinteger,andcollegegradepointaverageisbetweenzeroand4.0atmostcolleges.Mosteconomicvariableswewouldliketoexplainarelimitedinsomeway,oftenbecausetheymustbepositive.Forexample,hourlywage,housingprice,andnominalinterestratesmustbegreaterthanzero.Butnotallsuchvariablesneedspecialtreat-ment.Ifastrictlypositivevariabletakesonmanydifferentvalues,aspecialeconomet-ricmodelisrarelynecessary.Whenyisdiscreteandtakesonasmallnumberofvalues,itmakesnosensetotreatitasanapproximatelycontinuousvariable.Discretenessofydoesnotinitselfmeanthatlinearmodelsareinappropriate.However,aswesawinChapter7forbinaryresponse,thelinearprobabilitymodelhascertaindrawbacks.InSection17.1,wediscusslogitandprobitmodels,whichovercometheshortcomingsoftheLPM;thedisadvantageisthattheyaremoredifficulttointerpret.Otherkindsoflimiteddependentvariablesariseineconometricanalysis,especiallywhenthebehaviorofindividuals,families,orfirmsisbeingmodeled.Optimizingbehavioroftenleadstocornersolutionsforsomenontrivialfractionofthepopulation;thatis,itisoptimaltochooseazeroquantityordollarvalue.Forexample,duringanygivenyear,asignificantnumberoffamilieswillmakezerocharitablecontributions.Therefore,annualfamilycharitablecontributionshasapopulationdistributionthatisspreadoutoveralargerangeofpositivevalues,butwithapileupatthevaluezero.Whileitcouldbethatalinearmodelisappropriateforcapturingtheexpectedvalueofcharitablecontributions,alinearmodelwilllikelyleadtonegativepredictionsforsomefamilies.Takingthenaturallogisnotpossiblebecausemanyobservationsarezero.TheTobitmodel,whichwecoverinSection17.2,isexplicitlydesignedtomodelcornersolutiondependentvariables.529\nd7/14/998:28PMPage530Part3AdvancedTopicsAnotherimportantkindofLDVisacountvariable,whichtakesonnonnegativeintegervalues.Section17.3illustrateshowPoissonregressionmodelsarewell-suitedformodelingcountvariables.Insomecases,weobservelimiteddependentvariablesduetodatacensoring,atopicweintroduceinSection17.4.Thegeneralproblemofsampleselection,whereweobserveanonrandomsamplefromtheunderlyingpopulation,istreatedinSection17.5.Limiteddependentvariablemodelscanbeusedfortimeseriesandpaneldata,buttheyaremostoftenappliedtocross-sectionaldata.Sampleselectionproblemsareusu-allyconfinedtocross-sectionalorpaneldata.Wefocusoncross-sectionalapplicationsinthischapter.Wooldridge(1999)presentstheseproblemsinthecontextofpaneldatamodelsandprovidesmanymoredetailsforcross-sectionalandpaneldataapplications.17.1LOGITANDPROBITMODELSFORBINARYRESPONSEThelinearprobabilitymodelissimpletoestimateanduse,butithassomedrawbacksthatwediscussedinSection7.5.Thetwomostimportantdisadvantagesarethatthefit-tedprobabilitiescanbelessthanzeroorgreaterthanoneandthepartialeffectofanyexplanatoryvariable(appearinginlevelform)isconstant.TheselimitationsoftheLPMcanbeovercomebyusingmoresophisticatedbinaryresponsemodels.Inabinaryresponsemodel,interestliesprimarilyintheresponseprobabilityP(y1x)P(y1x1,x2,…,xk),(17.1)whereweusextodenotethefullsetofexplanatoryvariables.Forexample,whenyisanemploymentindicator,xmightcontainvariousindividualcharacteristicssuchaseducation,age,maritalstatus,andotherfactorsthataffectemploymentstatus,includ-ingabinaryindicatorvariableforparticipationinarecentjobtrainingprogram.SpecifyingLogitandProbitModelsIntheLPM,weassumethattheresponseprobabilityislinearinasetofparameters,j;seeequation(7.27).ToavoidtheLPMlimitations,consideraclassofbinaryresponsemodelsoftheformP(y1x)G(01x1…kxk)G(0x),(17.2)whereGisafunctiontakingonvaluesstrictlybetweenzeroandone:0G(z)1,forallrealnumbersz.Thisensuresthattheestimatedresponseprobabilitiesarestrictlybetweenzeroandone.Asinearlierchapters,wewritex1x1…kxk.VariousnonlinearfunctionshavebeensuggestedforthefunctionGinordertomakesurethattheprobabilitiesarebetweenzeroandone.Thetwowewillcoverhereareusedinthevastmajorityofapplications(alongwiththeLPM).Inthelogitmodel,Gisthelogisticfunction:530\nd7/14/998:28PMPage531Chapter17LimitedDependentVariableModelsandSampleSelectionCorrectionsG(z)exp(z)/[1exp(z)](z),(17.3)whichisbetweenzeroandoneforallrealnumbersz.Thisisthecumulativedistribu-tionfunctionforastandardlogisticrandomvariable.Intheprobitmodel,Gisthestan-dardnormalcumulativedistributionfunction(cdf),whichisexpressedasanintegral:zG(z)(z)(v)dv,(17.4)where(z)isthestandardnormaldensity1/22(z)(2)exp(z/2).(17.5)ThischoiceofGagainensuresthat(17.2)isstrictlybetweenzeroandoneforallval-uesoftheparametersandthexj.TheGfunctionsin(17.3)and(17.4)arebothincreasingfunctions.Eachincreasesmostquicklyatz0,G(z)*0asz*,andG(z)*1asz*.Thelogisticfunc-tionisplottedinFigure17.1.Thestandardnormalcdfhasashapeverysimilartothatofthelogisticcdf.Figure17.1GraphofthelogisticfunctionG(z)exp(z)/[1exp(z)].G(z)exp(z)/[1exp(z)]1.503210123z531\nd7/14/998:28PMPage532Part3AdvancedTopicsLogitandprobitmodelscanbederivedfromanunderlyinglatentvariablemodelthatsatisfiestheclassicallinearmodelassumptions.Lety*beanunobserved,orlatent,variable,determinedbyy*0xe,y1[y*0],(17.6)whereweintroducethenotation1[]todefineabinaryoutcome.Thefunction1[]iscalledtheindicatorfunction,whichtakesonthevalueoneiftheeventinbracketsistrue,andzerootherwise.Therefore,yisoneify*0,andyiszeroify*0.Weassumethateisindependentofxandthateeitherhasthestandardlogisticdistributionorthestandardnormaldistribution.Ineithercase,eissymmetricallydistributedaboutzero,whichmeansthat1G(z)G(z)forallrealnumbersz.Economiststendtofavorthenormalityassumptionfore,whichiswhytheprobitmodelismorepopularthanlogitineconometrics.Inaddition,severalspecificationproblems,whichwetouchonlater,aremosteasilyanalyzedusingprobitbecauseofpropertiesofthenormaldis-tribution.From(17.6)andtheassumptionsgiven,wecanderivetheresponseprobabilityfory:P(y1x)P(y*0x)P[e(0x)x]1G[(0x)]G(0x),whichisexactlythesameas(17.2).Inmostapplicationsofbinaryresponsemodels,theprimarygoalistoexplaintheeffectsofthexjontheresponseprobabilityP(y1x).Thelatentvariableformulationtendstogivetheimpressionthatweareprimarilyinterestedintheeffectsofeachxjony*.Aswewillsee,forlogitandprobit,thedirectionoftheeffectofxjonE(y*x)0xandonE(yx)P(y1x)G(0x)isalwaysthesame.Butthelatentvariabley*rarelyhasawell-definedunitofmeasurement.(Forexample,y*mightbethedifferenceinutilitylevelsfromtwodifferentactions.)Thus,themagnitudesofeachjarenot,bythemselves,especiallyuseful(incontrasttothelinearprobabilitymodel).Formostpurposes,wewanttoestimatetheeffectofxjontheprobabilityofsuccessP(y1x),butthisiscomplicatedbythenonlinearnatureofG().Tofindthepartialeffectofroughlycontinuousvariablesontheresponseprobabil-ity,wemustrelyoncalculus.Ifxjisaroughlycontinuousvariable,itspartialeffectonp(x)P(y1x)isobtainedfromthepartialderivative:p(x)dGg(0x)j,whereg(z)(z).(17.7)xjdzBecauseGisthecdfofacontinuousrandomvariable,gisaprobabilitydensityfunc-tion.Inthelogitandprobitcases,G()isastrictlyincreasingcdf,andsog(z)0forallz.Therefore,thepartialeffectofxjonp(x)dependsonxthroughthepositivequan-tityg(0x),whichmeansthatthepartialeffectalwayshasthesamesignasj.Equation(17.7)showsthattherelativeeffectsofanytwocontinuousexplanatoryvariablesdonotdependonx:theratioofthepartialeffectsforxjandxhisj/h.Inthetypicalcasethatgisasymmetricdensityaboutzero,withauniquemodeatzero,the532\nd7/14/998:28PMPage533Chapter17LimitedDependentVariableModelsandSampleSelectionCorrectionslargesteffectoccurswhen0x0.Forexample,intheprobitcasewithg(z)2(z),g(0)(0)1/2.40.Inthelogitcase,g(z)exp(z)/[1exp(z)],andsog(0).25.If,say,x1isabinaryexplanatoryvariable,thenthepartialeffectfromchangingx1fromzerotoone,holdingallothervariablesfixed,issimplyG(012x2…kxk)G(02x2…kxk).(17.8)Again,thisdependsonallthevaluesoftheotherxj.Forexample,ifyisanemploymentindicatorandx1isadummyvariableindicatingparticipationinajobtrainingprogram,then(17.8)isthechangeintheprobabilityofemploymentduetothejobtrainingpro-gram;thisdependsonothercharacteristicsthataffectemployability,suchaseducationandexperience.Notethatknowingthesignof1issufficientfordeterminingwhethertheprogramhadapositiveornegativeeffect.Buttofindthemagnitudeoftheeffect,wehavetoestimatethequantityin(17.8).Wecanalsousethedifferencein(17.8)forotherkindsofdiscretevariables(suchasnumberofchildren).Ifxkdenotesthisvariable,thentheeffectontheprobabilityofxkgoingfromcktock1issimplyG[01x12x2…k(ck1)](17.9)G(01x12x2…kck).Itisstraightforwardtoincludestandardfunctionalformsamongtheexplanatoryvariables.Forexample,inthemodel2P(y1z)G(01z12z13log(z2)4z3),thepartialeffectofz1onP(y1z)isP(y1z)/z1g(0x)(122z1),andthepartialeffectofz2ontheresponseprobabilityisP(y1z)/z2g(02x)(3/z2),wherex1z12z13log(z2)4z3.Modelswithinteractionsamongexplanatoryvariables,includingthosebetweendiscreteandcontinuousvari-ables,arehandledsimilarly.Whenmeasuringeffectsofdiscretevariables,weshoulduse(17.9).MaximumLikelihoodEstimationofLogitandProbitModelsHowshouldweestimatenonlinearbinaryresponsemodels?ToestimatetheLPM,wecanuseordinaryleastsquares(seeSection7.5)or,insomecases,weightedleastsquares(seeSection8.5).BecauseofthenonlinearnatureofE(yx),OLSandWLSarenotapplicable.Wecouldusenonlinearversionsofthesemethods,butitisnomoredif-ficulttousemaximumlikelihoodestimation(MLE)(seeAppendixBforabriefdis-cussion).Upuntilnow,wehavehadlittleneedforMLE,althoughwedidnotethat,undertheclassicallinearmodelassumptions,theOLSestimatoristhemaximumlike-lihoodestimator(conditionalontheexplanatoryvariables).Forestimatinglimiteddependentvariablemodels,maximumlikelihoodmethodsareindispensable.533\nd7/14/998:28PMPage534Part3AdvancedTopicsAssumethatwehavearandomsampleofsizen.Toobtainthemaximumlikelihoodestimator,conditionalontheexplanatoryvariables,weneedthedensityofyigivenxi.Wecanwritethisasy1yf(yxi;)[G(xi)][1G(xi)],y0,1,(17.10)where,forsimplicity,weabsorbtheinterceptintothevectorxi.Wecaneasilyseethatwheny1,wegetG(xi)andwheny0,weget1G(xi).Thelog-likelihoodfunctionforobservationiisafunctionoftheparametersandthedata(xi,yi)andisobtainedbytakingthelogof(17.10):i()yilog[G(xi)](1yi)log[1G(xi)].(17.11)BecauseG()isstrictlybetweenzeroandoneforlogitandprobit,i()iswell-definedforallvaluesof.Thelog-likelihoodforasamplesizeofnisobtainedbysumming(17.11)acrossnallobservations:()i().TheMLEof,denotedbyˆ,maximizesthislog-i1likelihood.IfG()isthestandardlogitcdf,thenˆisthelogitestimator;ifG()isthestandardnormalcdf,thenˆistheprobitestimator.Becauseofthenonlinearnatureofthemaximizationproblem,wecannotwritefor-mulasforthelogitorprobitmaximumlikelihoodestimates.Inadditiontoraisingcom-putationalissues,thismakesthestatisticaltheoryforlogitandprobitmuchmoredifficultthanOLSoreven2SLS.Nevertheless,thegeneraltheoryof(conditional)MLEforrandomsamplesimpliesthat,underverygeneralconditions,theMLEisconsistent,asymptoticallynormal,andasymptoticallyefficient.[SeeWooldridge(1999,Chapter13)forageneraldiscussion.]Wewilljustusetheresultshere;applyinglogitandpro-bitmodelsisfairlyeasy,providedweunderstandwhatthestatisticsmean.Eachˆjcomeswithan(asymptotic)standarderror,theformulaforwhichiscom-plicatedandpresentedinthechapterappendix.Oncewehavethestandarderrors—andthesearereportedalongwiththecoefficientestimatesbyanypackagethatsupportslogitandprobit—wecanconstruct(asymptotic)ttestsandconfidenceintervals,justaswithOLS,2SLS,andtheotherestimatorswehaveencountered.Inparticular,totestH0:j0,weformthetstatisticˆj/se(ˆj)andcarryoutthetestintheusualway,oncewehavedecidedonaone-ortwo-sidedalternative.TestingMultipleHypothesesWecanalsotestmultiplerestrictionsinlogitandprobitmodels.Inmostcases,thesearetestsofmultipleexclusionrestrictions,asinSection4.5.Wewillfocusonexclusionrestrictionshere.Therearethreewaystotestexclusionrestrictionsforlogitandprobitmodels.TheLagrangemultiplierorscoretestonlyrequiresestimatingthemodelunderthenullhypothesis,justasinthelinearcaseinSection5.2;wewillnotcoverthescoretesthere,sinceitisrarelyneededtotestexclusionrestrictions[seeWooldridge(1999,Chapter15)forotherusesofthescoretestinbinaryresponsemodels].534\nd7/14/998:28PMPage535Chapter17LimitedDependentVariableModelsandSampleSelectionCorrectionsTheWaldtestrequiresestimationofonlytheunrestrictedmodel.Inthelinearmodelcase,theWaldstatistic,afterasimpletransformation,isessentiallytheFstatistic;thereisnoneedtocovertheWaldstatisticseparately.TheformulafortheWaldstatisticisgiveninWooldridge(1999,Chapter15).Thisstatisticiscomputedbyeconometricspackagesthatallowexclusionrestrictionstobetestedaftertheunrestrictedmodelhasbeenestimated.Ithasanasymptoticchi-squaredistribution,withdfequaltothenum-berofrestrictionsbeingtested.Ifboththerestrictedandunrestrictedmodelsareeasytoestimate—asisusuallythecasewithexclusionrestrictions—thenthelikelihoodratio(LR)testbecomesveryattractive.TheLRtestisbasedonthesameconceptastheFtestinalinearmodel.TheFtestmeasurestheincreaseinthesumofsquaredresidualswhenvariablesaredroppedfromthemodel.TheLRtestisbasedonthedifferenceinthelog-likelihoodfunctionsfortheunrestrictedandrestrictedmodels.Theideaisthis.BecausetheMLEmaximizesthelog-likelihoodfunction,droppingvariablesgenerallyleadstoasmaller—oratleastnolarger—log-likelihood.(ThisissimilartothefactthattheR-squaredneverincreaseswhenvariablesaredroppedfromaregression.)Theques-tioniswhetherthefallinthelog-likelihoodislargeenoughtoconcludethatthedroppedvariablesareimportant.Wecanmakethisdecisiononcewehaveateststa-tisticandasetofcriticalvalues.Thelikelihoodratiostatisticistwicethedifferenceinthelog-likelihoods:LR2(urr),(17.12)whereuristhelog-likelihoodvaluefortheunrestrictedmodel,andristhelog-likelihoodvaluefortherestrictedmodel.Becauseurr,LRisnonnegativeandQUESTION17.1usuallystrictlypositive.IncomputingtheAprobitmodeltoexplainwhetherafirmistakenoverbyanotherLRstatistic,itisimportanttoknowthatfirmduringagivenyearisurandrcaneachbenegative.ThisdoesnotchangethewaythatLRiscomputed;P(takeover1x)(01avgprof2mktvalwemustpreservethenegativesigns.3debtearn4ceoten5ceosal6ceoage),Themultiplicationbytwoin(17.12)iswheretakeoverisabinaryresponsevariable,avgprofisthefirm’sneededsothatLRhasanapproximatechi-averageprofitmarginoverseveralprioryears,mktvalismarketvaluesquaredistributionunderH0.Ifwearetest-ofthefirm,debtearnisthedebt-to-earningsratio,andceoten,2ceosal,andceoagearethetenure,annualsalary,andageoftheingqexclusionrestrictions,LR~ªq.Thischiefexecutiveofficer,respectively.Statethenullhypothesisthat,meansthat,totestH0atthe5%level,wethotherfactorsbeingequal,variablesrelatedtotheCEOhavenouseasourcriticalvaluethe95percentileeffectontheprobabilityoftakeover.Howmanydfareinthechi-inthe2distribution.Computingp-valuesqsquaredistributionfortheLRorWaldtest?iseasywithmostsoftwarepackages.InterpretingtheLogitandProbitEstimatesGivenmoderncomputers,fromapracticalperspective,themostdifficultaspectoflogitorprobitmodelsispresentingandinterpretingtheresults.Thecoefficientestimates,theirstandarderrors,andthevalueofthelog-likelihoodfunctionarereportedbyallsoftwarepackagesthatdologitandprobit,andtheseshouldbereportedinanyappli-535\nd7/14/998:28PMPage536Part3AdvancedTopicscation.Thecoefficientsgivethesignsofthepartialeffectsofeachxjontheresponseprobability,andthestatisticalsignificanceofxjisdeterminedbywhetherwecanrejectH0:j0atasufficientlysmallsignificancelevel.Onegoodness-of-fitmeasurethatisusuallyreportedistheso-calledpercentcor-rectlypredicted,whichiscomputedasfollows.Foreachi,wecomputetheestimatedprobabilitythatyitakesonthevalueone,G(ˆ0xiˆ).IfG(ˆ0xiˆ).5,thepre-dictionofyiisunity,andifG(ˆ0xiˆ).5,yiispredictedtobezero.Thepercent-ageoftimesthepredictedyimatchestheactualyi(whichweknowtobezeroorone)isthepercentcorrectlypredicted.Thismeasureissomewhatuseful,butitispossibletogetratherhighpercentagescorrectlypredictedwithoutthemodelbeingofmuchuse.Forexample,supposethatinasamplesizeof200,180observationshaveyi0,and150ofthesearepredictedtobezerousingthepreviousrule.Evenifnoneofourpre-dictionsarecorrectwhenyi1,westillpredict75%oftheoutcomescorrectly.Becauseofexampleslikethis,itmakessensetoreportthepercentcorrectlypredictedforeachofthetwooutcomes.TherearealsovariouspseudoR-squaredmeasuresforbinaryresponse.McFadden(1974)suggeststhemeasure1ur/o,whereuristhelog-likelihoodfunctionfortheestimatedmodel,andoisthelog-likelihoodfunctioninthemodelwithonlyanintercept.ThisisanalogoustotheR-squaredforOLSregression,whichcanbewrittenas1SSRur/SSRo,whereSSRuristhesumofsquaredresiduals,andSSRoisthesameasthetotalsumofsquares.Severalothermeasureshavebeensuggested[see,forexam-ple,Maddala(1983,Chapter2)],butgoodness-of-fitisnotusuallyasimportantassta-tisticalandeconomicalsignificanceoftheexplanatoryvariables.Often,wewanttoestimatetheeffectsofthexjontheresponseprobabilities,P(y1x).Ifxjis(roughly)continuous,thenPˆ(y1x)[g(ˆ0xˆ)ˆj]xj,(17.13)for“small”changesinxj.Sinceg(ˆ0xˆ)dependsonx,wemustcomputeitatinterestingvaluesofx.Oftenthesampleaveragesofthexjarepluggedintogetg(ˆ0x¯ˆ).Thisfactorcanthenbeusedtoadjusteachoftheˆj(oratleastthoseoncontinuousvariables)toobtaintheeffectofaone-unitincreaseinxj.Ifxcontainsnon-linearfunctionsofsomeexplanatoryvariables,suchasnaturallogsorquadratics,wehavetheoptionofpluggingtheaverageintothenonlinearfunctionoraveragingthenonlinearfunction.Togettheeffectfortheaverageunitinthepopulation,itmakessensetousethefirstoption.Ifasoftwarepackageautomaticallyscalesthecoefficientsbyg(ˆ0x¯ˆ),itnecessarilyaveragesthenonlinearfunctions,asitcannottellwhenanexplanatoryvariableisanonlinearfunctionofsomeunderlyingvariable.Thedifferenceisrarelylarge.Sometimes,minimumandmaximumvalues,orlowerandupperquartiles,ofkeyvariablesareusedinobtainingg(ˆ0xˆ),sothatwecanseehowthepartialeffectschangeassomeelementsofxgetlargeorsmall.Equation(17.13)alsosuggestshowtoroughlycomparemagnitudesoftheprobitandlogitslopeestimates.Asmentionedearlier,forprobit,g(0).4,andforlogit,g(0).25.Thus,tomakethelogitandprobitslopeestimatescomparable,wecaneithermultiplytheprobitestimatesby.4/.251.6,orwecanmultiplythelogitesti-536\nd7/14/998:28PMPage537Chapter17LimitedDependentVariableModelsandSampleSelectionCorrectionsmatesby.625.Inthelinearprobabilitymodel,g(0)iseffectively1,andsologitslopeestimatesshouldbedividedby4tomakethemroughlycomparabletotheLPMesti-mates;theprobitslopeestimatesshouldbedividedby2.5tomakethemcomparabletotheLPMslopeestimates.Amoreaccuratecomparisonistomultiplytheprobitslopecoefficientsby(ˆ0x¯ˆ)andthelogitslopecoefficientsbyexp(ˆ0x¯ˆ)/[12exp(ˆ0x¯ˆ)],wheretheestimatesareeitherprobitorlogit,respectively.If,say,xkisabinaryvariable,itmaymakesensetopluginzerooroneforxk,ratherthanx¯k(whichisthefractionofonesinthesample).Puttingintheaveragesforthebinaryvariablesmeansthattheeffectdoesnotreallycorrespondtoaparticularindi-vidual.Butoftentheresultsaresimilar,andthechoiceisreallybasedontaste.Ifxkisadiscretevariable,thenwecanestimatethechangeinthepredictedproba-bilityasitgoesfromcktock1byG[ˆ0ˆ1x¯1…ˆk1x¯k1ˆk(ck1)](17.14)G(ˆ0ˆ1x¯1…ˆk1x¯k1ˆkck).Inparticular,whenxkisabinaryvariable,wesetck0.Ofcourse,wehavetodecidewhattopluginfortheotherexplanatoryvariables;typically,weuseaveragesforroughlycontinuousvariables.EXAMPLE17.1(MarriedWomen’sLaborForceParticipation)WenowusetheMROZ.RAWdatatoestimatethelaborforceparticipationmodelfromExample8.8—seealsoSection7.5—bylogitandprobit.Wealsoreportthelinearproba-bilitymodelestimatesfromExample8.8,usingtheheteroskedasticity-robuststandarderrors.Theresults,withstandarderrorsinparentheses,aregiveninTable17.1.Theestimatesfromthethreemodelstellaconsistentstory.Thesignsofthecoefficientsarethesameacrossmodels,andthesamevariablesarestatisticallysignificantineachmodel.ThepseudoR-squaredfortheLPMisjusttheusualR-squaredreportedforOLS;forlogitandprobitthepseudoR-squaredisthemeasurebasedonthelog-likelihoodsdescribedearlier.Aswehavealreadyemphasized,themagnitudesofthecoefficientsarenotdirectlycomparableacrossthemodels.Usingtheroughrule-of-thumbdiscussedearlier,wecandividethelogitestimatesby4andtheprobitestimatesby2.5tomakethemcomparabletotheLPMestimates.Forexample,forthecoefficientsonkidslt6,thescaledlogitestimateisabout.361,andthescaledprobitestimateisabout.347.Thesearelargerinmagni-tudethantheLPMestimate(forreasonswewillgivelater).Similarly,thescaledcoefficientoneducis.055forlogitand.052forprobit;thesearealsosomewhatgreaterthantheLPMestimateof.038,buttheydonotdiffermuchfromeachother.Ifweevaluatethestandardnormalprobabilitydensityfunction,(ˆ0ˆ1x1…ˆkxk),attheaveragevaluesoftheindependentvariablesinthesample(includingtheaver-2ageofexper),theresultisapproximately.391;thisiscloseenoughto.4tomaketheroughrule-of-thumbforscalingtheprobitcoefficientsusefulinobtainingtheeffectsontheresponseprobability.Inotherwords,toestimatethechangeintheresponseprobabilitygivenaone-unitincreaseinanyindependentvariable,wemultiplythecorrespondingpro-537\nd7/14/998:28PMPage538Part3AdvancedTopicsTable17.1LPM,Logit,andProbitEstimatesofLaborForceParticipationDependentVariable:inlfIndependentLPMLogitProbitVariables(OLS)(MLE)(MLE)nwifeinc.0034.021.012(.0015)(.008)(.005)educ.038.221.131(.007)(.043)(.025)exper.039.206.123(.006)(.032)(.019)2exper.00060.0032.0019(.00018)(.0010)(.0006)age.016.088.053(.002)(.015)(.008)kidslt6.2621.443.868(.032)(0.204)(.119)kidsge6.013.060.036(.013)(.075)(.043)constant.586.425.270(.151)(.860)(.509)PercentCorrectlyPredicted73.473.673.4Log-LikelihoodValue—401.77401.30PseudoR-Squared.264.220.221bitcoefficientby.4.Presumably,ifweevaluatethestandardlogisticfunctionatthemeanvaluesandthelogitestimates,wewouldgetcloseto.25.ThebiggestdifferencebetweentheLPMmodelandthelogitandprobitmodelsisthattheLPMassumesconstantmarginaleffectsforeduc,kidslt6,andsoon,whilethelogitandprobitmodelsimplydiminishingmagnitudesofthepartialeffects.IntheLPM,onemoresmallchildisestimatedtoreducetheprobabilityoflaborforceparticipationbyabout.262,regardlessofhowmanyyoungchildrenthewomanalreadyhas(andregardlessofthe538\nd7/14/998:28PMPage539Chapter17LimitedDependentVariableModelsandSampleSelectionCorrectionslevelsoftheotherexplanatoryvariables).QUESTION17.2WecancontrastthiswiththeestimatedUsingtheprobitestimatesandthecalculusapproximation,whatismarginaleffectfromprobit.Forconcrete-theapproximatechangeintheresponseprobabilitywhenexperness,takeawomanwithnwifeinc20.13,increasesfrom10to11?educ12.3,exper10.6,andage42.5—whichareroughlythesampleaver-ages—andkidsge61.Whatistheestimateddecreaseintheprobabilityofworkingingoingfromzerotoonesmallchild?Weevaluatethestandardnormalcdf,(ˆ0ˆ1x1…ˆkxk),withkidslt61andkidslt60,andtheotherindependentvariablessetattheprecedingvalues.Wegetroughly.373.707.334,whichmeansthatthelaborforceparticipationprobabilityisabout.334lowerwhenawomanhasoneyoungchild.Thisisnotmuchdifferentthanthescaledprobitcoefficientof.347.Ifthewomangoesfromonetotwoyoungchildren,theprobabilityfallsevenmore,butthemarginaleffectisnotaslarge:.117.373.256.Interestingly,theestimatefromthelinearprobabilitymodel,whichissupposedtoestimatetheeffectneartheaverage,isinfactbetweenthesetwoestimates.Thesameissuesconcerningendogenousexplanatoryvariablesinlinearmodelsalsoariseinlogitandprobitmodels.Wedonothavethespacetocoverthem,butitispos-sibletotestandcorrectforendogenousexplanatoryvariablesusingmethodsrelatedtotwostageleastsquares.EvansandSchwab(1995)estimatedaprobitmodelforwhetherastudentattendscollege,wherethekeyexplanatoryvariableisadummyvariableforwhetherthestudentattendsaCatholicschool.EvansandSchwabestimatedamodelbymaximumlikelihoodthatallowsthisvariabletobeconsideredendogenous.[SeeWooldridge(1999,Chapter15)foranexplanationofthesemethods.]Twootherissueshavereceivedattentioninthecontextofprobitmodels.Thefirstisnonnormalityofeinthelatentvariablemodel(17.6).Naturally,ifedoesnothaveastandardnormaldistribution,theresponseprobabilitywillnothavetheprobitform.Someauthorstendtoemphasizetheinconsistencyinestimatingthej,butthisisthewrongfocusunlessweareonlyinterestedinthedirectionoftheeffects.Becausetheresponseprobabilityisunknown,wecouldnotestimatethemagnitudeofpartialeffectsevenifwehadconsistentestimatesofthej.Asecondspecificationproblem,alsodefinedintermsofthelatentvariablemodel,isheteroskedasticityine.IfVar(ex)dependsonx,theresponseprobabilitynolongerhastheformG(0x);instead,itdependsontheformofthevarianceandrequiresmoregeneralestimation.Suchmodelsarenotoftenusedinpractice,sincelogitandprobitwithflexiblefunctionalformsintheindependentvariablestendtoworkwell.Binaryresponsemodelsapplywithlittlemodificationtoindependentlypooledcrosssectionsortootherdatasetswheretheobservationsareindependentbutnotnec-essarilyidenticallydistributed.Oftenyearorothertimeperioddummyvariablesareincludedtoaccountforaggregatetimeeffects.Justaswithlinearmodels,logitandpro-bitcanbeusedtoevaluatetheimpactofcertainpoliciesinthecontextofanaturalexperiment.Thelinearprobabilitymodelcanbeappliedwithpaneldata;typically,itwouldbeestimatedbyfixedeffects(seeChapter14).Logitandprobitmodelswithunobservedeffectshaverecentlybecomepopular.Thesemodelsarecomplicatedbythenonlinear539\nd7/14/998:28PMPage540Part3AdvancedTopicsnatureoftheresponseprobabilities,andtheyaredifficulttoestimateandinterpret.[SeeWooldridge(1999,Chapter15).]17.2THETOBITMODELAnotherimportantkindoflimiteddependentvariableisonethatisroughlycontinuousoverstrictlypositivevaluesbutiszeroforanontrivialfractionofthepopulation.Anexampleistheamountanindividualspendsonalcoholinagivenmonth.Inthepopu-lationofpeopleoverage21intheUnitedStates,thisvariabletakesonawiderangeofvalues.Forsomesignificantfraction,theamountspentonalcoholiszero.Thefollow-ingtreatmentomitsverificationofsomedetailsconcerningtheTobitmodel.[ThesearegiveninWooldridge(1999,Chapter16).]Letybeavariablethatisessentiallycontinuousoverstrictlypositivevaluesbutthattakesonzerowithpositiveprobability.Nothingpreventsusfromusingalinearmodelfory.Infact,alinearmodelmightbeagoodapproximationtoE(yx1,x2,…,xk),espe-ciallyforxjnearthemeanvalues.Butwewouldpossiblyobtainnegativefittedvalues,whichleadstonegativepredictionsfory;thisisanalogoustotheproblemswiththeLPMforbinaryoutcomes.Further,itisoftenusefultohaveanestimateoftheentiredistributionofygiventheexplanatoryvariables.TheTobitmodelismosteasilydefinedasalatentvariablemodel:2y*0xu,ux~Normal(0,)(17.15)ymax(0,y*).(17.16)Thelatentvariabley*satisfiestheclassicallinearmodelassumptions;inparticular,ithasanormal,homoskedasticdistributionwithalinearconditionalmean.Equation(17.16)impliesthattheobservedvariable,y,equalsy*wheny*0,buty0wheny*0.Becausey*isnormallydistributed,yhasacontinuousdistributionoverstrictlypositivevalues.Inparticular,thedensityofygivenxisthesameasthedensityofy*givenxforpositivevalues.Further,P(y0x)P(y*0x)P(ux)P(u/x/)(x/)1(x/),becauseu/hasastandardnormaldistributionandisindependentofx;wehaveabsorbedtheinterceptintoxfornotationalsimplicity.Therefore,if(xi,yi)isarandomdrawfromthepopulation,thedensityofyigivenxiis21/222(2)exp[(yxi)/(2)](1/)[(yxi)/],y0(17.17)P(yi0xi)1(xi/),(17.18)whereisthestandardnormaldensityfunction.From(17.17)and(17.18),wecanobtainthelog-likelihoodfunctionforeachobser-vationi:540\nd7/14/998:28PMPage541Chapter17LimitedDependentVariableModelsandSampleSelectionCorrectionsi(,)1(yi0)log[1(xi/)](17.19)1(yi0)log{(1/)[(yixi)/]};noticehowthisdependson,thestandarddeviationofu,aswellasonthej.Thelog-likelihoodforarandomsampleofsizenisobtainedbysumming(17.19)acrossalli.Themaximumlikelihoodestimatesofandareobtainedbymaximizingthelog-QUESTION17.3likelihood;thisrequiresnumericalmeth-Letybethenumberofextramaritalaffairsforamarriedwomanods,althoughinmostcasesthisiseasilyfromtheU.S.population;wewouldliketoexplainthisvariableindoneusingapackagedroutine.termsofothercharacteristicsofthewoman—inparticular,whetherAsinthecaseoflogitandprobit,eachsheworksoutsideofthehome—herhusband,andherfamily.IsthisagoodcandidateforaTobitmodel?Tobitestimatecomeswithastandarderror,andthesecanbeusedtoconstructtstatis-ticsforeachˆj;thematrixformulausedtofindthestandarderrorsiscomplicatedandwillnotbepresentedhere.[See,forexample,Wooldridge(1999,Chapter16).]TestingmultipleexclusionrestrictionsiseasilydoneusingtheWaldtestorthelike-lihoodratiotest.TheWaldtesthasasimilarformtothelogitorprobitcase;theLRtestisalwaysgivenby(17.12),where,ofcourse,weusetheTobitlog-likelihoodfunctionsfortherestrictedandunrestrictedmodels.InterpretingtheTobitEstimatesUsingmoderncomputers,themaximumlikelihoodestimatesforTobitmodelsareusu-allynotmuchmoredifficulttoobtainthantheOLSestimatesofalinearmodel.Further,theoutputsfromTobitandOLSareoftensimilar.ThismakesittemptingtointerprettheˆjfromTobitasifthesewereestimatesfromalinearregression.Unfortunately,thingsarenotsoeasy.Fromequation(17.15),weseethatthejmeasurethepartialeffectsofthexjonE(y*x),wherey*isthelatentvariable.Sometimes,y*hasaninterestingeconomicmeaning,butmoreoftenitdoesnot.Thevariablewewanttoexplainisy,asthisistheobservedoutcome(suchashoursworkedoramountofcharitablecontributions).Forexample,asapolicymatter,weareinterestedinthesensitivityofhoursworkedtochangesinmarginaltaxrates.WecanestimateP(y0x)from(17.18),which,ofcourse,allowsustoestimateP(y0x).Whathappensifwewanttoestimatetheexpectedvalueofyasafunctionofx?InTobitmodels,twoexpectationsareofparticularinterest:E(yy0,x),whichissometimescalledthe“conditionalexpectation”becauseitisconditionalony0,andE(yx),whichis,unfortunately,calledthe“unconditionalexpectation.”(Bothexpectationsareconditionalontheexplanatoryvariables.)TheexpectationE(yy0,x)tellsus,forgivenvaluesofx,theexpectedvalueofyforthesubpopulationwhereyispositive.GivenE(yy0,x),wecaneasilyfindE(yx):E(yx)P(y0x)E(yy0,x)(x/)E(yy0,x).(17.20)ToobtainE(yy0,x),weusearesultfornormallydistributedrandomvariables:ifz~Normal(0,1),thenE(zzc)(c)/[1(c)]foranyconstantc.ButE(yy541\nd7/14/998:28PMPage542Part3AdvancedTopics0,x)xE(uux)xE[(u/)(u/)x/]x(x/)/(x/),because(c)(c),1(c)(c),andu/hasastandardnormaldistributionindependentofx.WecansummarizethisasE(yy0,x)x(x/),(17.21)where(c)(c)/(c)iscalledtheinverseMillsratio;itistheratiobetweenthestandardnormalpdfandstandardnormalcdf,eachevaluatedatc.Equation(17.21)isimportant.Itshowsthattheexpectedvalueofyconditionalony0isequaltox,plusastrictlypositiveterm,whichistimestheinverseMillsratioevaluatedatx/.ThisequationalsoshowswhyusingOLSonlyforobservationswhereyi0willnotalwaysconsistentlyestimate;essentially,theinverseMillsratioisanomittedvariable,anditisgenerallycorrelatedwiththeelementsofx.Combining(17.20)and(17.21)givesE(yx)(x/)[x(x/)](x/)x(x/),(17.22)wherethesecondequalityfollowsbecause(x/)(x/)(x/).ThisequationshowsthatwhenyfollowsaTobitmodel,E(yx)isanonlinearfunctionofxand,whichmakespartialeffectsdifficulttoobtain.ThisisoneofthecostsofusingaTobitmodel.Ifxjisacontinuousvariable,wecanfindthepartialeffectsusingcalculus.First,dE(yy0,x)/xjjj(x/),dcassumingthatxjisnotfunctionallyrelatedtootherregressors.Bydifferentiating(c)(c)/(c)andusingd/dc(c)andd/dcc(c),itcanbeshownthatd/dc(c)[c(c)].Therefore,E(yy0,x)/xjj{1(x/)[x/(x/)]}.(17.23)ThisshowsthatthepartialeffectofxjonE(yy0,x)isnotdeterminedjustbyj.Theadjustmentfactorisgivenbytheterminbrackets,{},anddependsonalinearfunctionofx,x/(01x1…kxk)/.Itcanbeshownthattheadjustmentfactorisstrictlybetweenzeroandone.Inpractice,wecanestimate(17.23)bypluggingintheMLEsofthejand.Aswithlogitandprobitmodels,wemustpluginvaluesforthexj,usuallythemeanvaluesorotherinterestingvalues.Alloftheusualeconomicquantitiessuchaselasticitiescanbecomputed.Forexam-ple,theelasticityofywithrespecttox1,conditionalony0,isE(yy0,x)x1.(17.24)x1E(yy0,x)Thiscanbecomputedwhenx1appearsinvariousfunctionalforms,includinglevel,log-arithmic,andquadraticforms.542\nd7/14/998:28PMPage543Chapter17LimitedDependentVariableModelsandSampleSelectionCorrectionsIfx1isabinaryvariable,theeffectofinterestisobtainedasthedifferencebetweenE(yy0,x),withx11andx10.Partialeffectsinvolvingotherdiscretevariables(suchasnumberofchildren)canbehandledsimilarly.Wecanuse(17.22)tofindthepartialderivativeofE(yx)withrespecttocontinu-ousxj.Thisderivativeaccountsforthefactthatpeoplestartingaty0mightchoosey0whenxjchanges:E(yx)P(y0x)E(yy0,x)E(yy0,x)P(y0x).(17.25)xjxjxjBecauseP(y0x)(x/),P(y0x)(j/)(x/),(17.26)xjandsowecanestimateeachtermin(17.25),onceweplugintheMLEsofthejandandparticularvaluesofthexj.Remarkably,whenweplug(17.23)and(17.26)into(17.25)andusethefactthat(c)(c)(c)foranyc,weobtainE(yx)j(x/).(17.27)xjEquation(17.27)allowsustoroughlycompareOLSandTobitestimates.TheOLScoefficientsaredirectestimatesofE(yx)/xj.TomaketheTobitestimatescompara-ble,wemultiplythembytheadjustmentfactoratthemeanvaluesofthexj,(x¯ˆ/ˆ).Becausethisisjustavalueofthestandardnormalcdf,itisalwaysbetweenzeroandone.Since(x/)P(y0x),equation(17.27)showsthattheadjustmentfactorapproachesoneasP(y0x)approachesone.(Intheextremecasewhereyi0foralli,TobitandOLSproduceidenticalestimates.)EXAMPLE17.2(MarriedWomen’sAnnualLaborSupply)ThefileMROZ.RAWincludesdataonhoursworkedfor753marriedwomen,428ofwhomworkedforawageoutsidethehomeduringtheyear;325ofthewomenworkedzerohours.Forthewomenwhoworkedpositivehours,therangeisfairlybroad,extendingfrom12to4,950.Thus,annualhoursworkedisagoodcandidateforaTobitmodel.Wealsoestimatealinearmodel(usingall753observations)byOLS.TheresultsaregiveninTable17.2.Thistablehasseveralnoteworthyfeatures.First,theTobitcoefficientestimateshavethesamesignasthecorrespondingOLSestimates,andthestatisticalsignificanceoftheestimatesissimilar.(Possibleexceptionsarethecoefficientsonnwifeincandkidsge6,butthetstatisticshavesimilarmagnitudes.)Second,whileitistemptingtocomparethemag-nitudesoftheOLSandTobitestimates,thisisnotveryinformative.Wemustbecareful543\nd7/14/998:28PMPage544Part3AdvancedTopicsTable17.2OLSandTobitEstimationofAnnualHoursWorkedDependentVariable:hoursIndependentLinearTobitVariables(OLS)(MLE)nwifeinc3.458.81(2.54)(4.46)educ28.7680.65(12.95)(21.58)exper65.67131.56(9.96)(17.28)2exper.7001.86(.325)(0.54)age30.5154.41(4.36)(7.42)kidslt6442.09894.02(58.85)(111.88)kidsge632.7816.22(23.18)(38.64)constant1,330.48965.31(270.78)(446.44)Log-LikelihoodValue—3,819.09R-Squared.266.274ˆ750.181,122.02nottothinkthat,becausetheTobitcoefficientonkidslt6isroughlytwicethatoftheOLScoefficient,theTobitmodelimpliesamuchgreaterresponseofhoursworkedtoyoungchildren.WecanmultiplytheTobitestimatesbytheadjustmentfactorsin(17.23)and(17.27),evaluatedattheestimatesandthemeanvalues,toobtainthepartialeffectsonthecondi-tionalexpectations.Thefactorin(17.23)isabout.451.Forexample,conditionalonhoursbeingpositive,ayearofeducation(startingfromthemeanvaluesofallvariables)isesti-544\nd7/14/998:28PMPage545Chapter17LimitedDependentVariableModelsandSampleSelectionCorrectionsmatedtoincreaseexpectedhoursbyabout.451(80.65)36.4hours.ThisissomewhatlargerthantheOLSestimate.Usingtheapproximationforonemoreyoungchildgivesadropofabout(.451)(894.02)403.2inexpectedhours.Ofcourse,thisdoesnotmakesenseforawomanwhoworkslessthan403.2hours.Itwouldbebettertoestimatetheexpectedvaluesattwodifferentvaluesofkidslt6andtoformthedifference,ratherthantousethecalculusapproximation.Thefactorin(17.27),againevaluatedatthemeanvaluesofthexj,isabout.645.Therefore,themagnitudesoftheeffectsofeachxjonexpectedhours—thatis,whenweaccountforpeoplewhoinitiallydonotwork,aswellasthosewhooriginallydowork—arelargerthanwhenweconditiononhours0.WehavereportedanR-squaredforboththelinearregressionandtheTobitmodels.TheR-squaredforOLSistheusualone.ForTobit,theR-squaredisthesquareofthecorrelationcoefficientbetweenyiandyˆi,whereyˆi(xiˆ/ˆ)xiˆˆ(xiˆ/ˆ)istheestimateofE(yxxi).ThisismotivatedbythefactthattheusualR-squaredforOLSisequaltothesquaredcorrelationbetweentheyiandthefittedvalues[seeequation(3.29)].InnonlinearmodelssuchastheTobitmodel,thesquaredcorrelationcoefficientisnotidenticaltoanR-squaredbasedonasumofsquaredresidualsasin(3.28).Thisisbecausethefittedval-ues,asdefinedearlier,andtheresiduals,yiyˆi,arenotuncorrelatedinthesample.AnR-squareddefinedasthesquaredcorrelationcoefficientbetweenyiandyˆihastheadvan-tageofalwaysbeingbetweenzeroandone;anR-squaredbasedonasumofsquaredresid-ualsneednothavethisfeature.Wecanseethat,basedontheR-squaredmeasures,theTobitconditionalmeanfunc-tionfitsthehoursdatasomewhat,butnotsubstantially,better.However,weshouldrememberthattheTobitestimatesarenotchosentomaximizeanR-squared—theymaxi-mizethelog-likelihoodfunction—whereastheOLSestimatesarethevaluesthatdopro-ducethehighestR-squared.SpecificationIssuesinTobitModelsTheTobitmodel,andinparticulartheformulasfortheexpectationsin(17.21)and(17.22),relycruciallyonnormalityandhomoskedasticityintheunderlyinglatentvari-ablemodel.WhenE(yx)01x1…kxk,weknowfromChapter5thatcon-ditionalnormalityofydoesnotplayaroleinunbiasedness,consistency,orlargesampleinference.HeteroskedasticitydoesnotaffectunbiasednessorconsistencyofOLS,althoughwemustcomputerobuststandarderrorsandteststatisticstoperformapproximateinference.InaTobitmodel,ifanyoftheassumptionsin(17.15)fail,thenitishardtoknowwhattheTobitMLEisestimating.Nevertheless,formoderatedepar-turesfromtheassumptions,theTobitmodelislikelytoprovidegoodestimatesofthepartialeffectsontheconditionalmeans.Itispossibletoallowformoregeneralassump-tionsin(17.15),butsuchmodelsaremuchmorecomplicatedtoestimateandinterpret.OnepotentiallyimportantlimitationoftheTobitmodel,atleastincertainapplica-tions,isthattheexpectedvalueconditionalony0iscloselylinkedtotheprobabil-itythaty0.Thisisclearfromequations(17.23)and(17.26).Inparticular,theeffectofxjonP(y0x)isproportionaltoj,asistheeffectonE(yy0,x),wherebothfunctionsmultiplyingjarepositiveanddependonxonlythroughx/.Thisrulesout545\nd7/14/998:28PMPage546Part3AdvancedTopicssomeinterestingpossibilities.Forexample,considertherelationshipbetweenthevalueofalifeinsurancepolicyandaperson’sage.Youngpeoplemaybelesslikelytohavelifeinsuranceatall,sotheprobabilitythaty0increaseswithage(atleastuptoapoint).Conditionalonhavinglifeinsurance,thevalueofpoliciesmightdecreasewithage,sincelifeinsurancebecomeslessimportantaspeopleneartheendoftheirlives.ThispossibilityisnotallowedforintheTobitmodel.OnewaytoinformallyevaluatewhethertheTobitmodelisappropriateistoesti-mateaprobitmodelwherethebinaryoutcome,sayw,equalsoneify0,andw0ify0.Then,from(17.18),wfollowsaprobitmodel,wherethecoefficientonxjisjj/.Thismeanswecanestimatetheratioofjtobyprobit,foreachj.IftheTobitmodelholds,theprobitestimate,ˆj,shouldbe“close”toˆj/ˆ,whereˆjandˆaretheTobitestimates.Thesewillneverbeidenticalbecauseofsamplingerror.Butwecanlookforcertainproblematicsigns.Forexample,ifˆjissignificantandnegative,butˆjispositive,theTobitmodelmightnotbeappropriate.Or,ifˆjandˆjarethesamesign,butˆj/ˆismuchlargerorsmallerthanˆj,thiscouldalsoindicateproblems.Weshouldnotworrytoomuchaboutsignchangesormagnitudedifferencesonexplanatoryvariablesthatareinsignificantinbothmodels.Intheannualhoursworkedexample,ˆ1,122.02.WhenwedividetheTobitco-efficientonnwifeincbyˆ,weobtain8.81/1,122.02.0079;theprobitcoeffi-cientonnwifeincisabout.012,whichisdifferent,butnotdramaticallyso.Onkidslt6,thecoefficientestimateoverˆisabout.797,comparedwiththeprobitesti-mateof.868.Again,thisisnotahugedifference,butitindicatesthathavingsmallchildrenhasalargereffectontheinitiallaborforceparticipationdecisionthanonhowmanyhoursawomanchoosestoworkoncesheisinthelaborforce.(Tobiteffectivelyaveragesthesetwoeffectstogether.)Wedonotknowwhethertheeffectsarestatisti-callydifferent,buttheyareofthesameorderofmagnitude.WhathappensifweconcludethattheTobitmodelisinappropriate?Therearemod-els,usuallycalledhurdleortwo-partmodels,thatcanbeusedwhenTobitseemsunsuit-able.TheseallhavethepropertythatP(y0x)andE(yx,y0)dependondifferentparameters,andsoxjcanhaveverydissimilareffectsonthesetwofunctions.[SeeWooldridge(1999,Chapter16)foradescriptionofthesemodels.]17.3THEPOISSONREGRESSIONMODELAnotherkindofnonnegativedependentvariableisacountvariable,whichcantakeonnonnegativeintegervalues:{0,1,2,…}.Weareespeciallyinterestedincaseswhereytakesonrelativelyfewvalues,includingzero.Examplesincludethenumberofchildreneverborntoawoman,thenumberoftimessomeoneisarrestedinayear,orthenum-berofpatentsappliedforbyafirminayear.ForthesamereasonsdiscussedforbinaryandTobitresponses,alinearmodelforE(yx1,…,xk)mightnotprovidethebestfitoverallvaluesoftheexplanatoryvariables.(Nevertheless,itisalwaysinformativetostartwithalinearmodel,aswedidinExample3.5.)AswithaTobitoutcome,wecannottakethelogarithmofacountvariablebecauseittakesonthevaluezero.Aprofitableapproachistomodeltheexpectedvalueasanexponentialfunction:546\nd7/14/998:28PMPage547Chapter17LimitedDependentVariableModelsandSampleSelectionCorrectionsE(yx1,x2,…,xk)exp(01x1…kxk).(17.28)Becauseexp()isalwayspositive,(17.28)ensuresthatpredictedvaluesforywillalsobepositive.While(17.28)ismorecomplicatedthanalinearmodel,webasicallyalreadyknowhowtointerpretthecoefficients.Takingthelogofequation(17.28)showsthatlog[E(yx1,x2,…,xk)]01x1…kxk,(17.29)sothatthelogoftheexpectedvalueislinear.Therefore,usingtheapproximationprop-ertiesofthelogfunctionthatwehaveusedofteninpreviouschapters,%E(yx)(100j)xj.Inotherwords,100jisroughlythepercentagechangeinE(yx),givenaone-unitincreaseinxj.Sometimes,amoreaccurateestimateisneeded,andwecaneasilyfindonebylookingatdiscretechangesintheexpectedvalue.Keepallexplanatoryvariables01exceptxkfixedandletxkbetheinitialvalueandxkthesubsequentvalue.Then,thepro-portionatechangeintheexpectedvalueis10[exp(0xk1k1kxk)/exp(0xk1k1kxk)]1exp(kxk)1,10wherexk1k1isshorthandfor1x1…k1xk1,andxkxkxk.Whenxk1—forexample,ifxkisadummyvariablethatwechangefromzerotoone—thenthechangeisexp(k)1.Givenˆk,weobtainexp(ˆk)1andmultiplythisby100toturntheproportionatechangeintoapercentagechange.Byreasoningsimilartothelinearmodel,ifjmultiplieslog(xj),thenjisanelas-ticity.Thebottomlineisthat,forpracticalpurposes,wecaninterpretthecoefficientsinequation(17.28)asifwehavealinearmodel,withlog(y)asthedependentvariable.Therearesomesubtledifferencesthatweneednotstudyhere.Because(17.28)isnonlinearinitsparameters—remember,exp()isanonlinearfunction—wecannotuselinearregressionmethods.Wecouldusenonlinearleastsquares,which,justaswithOLS,minimizesthesumofsquaredresiduals.Itturnsout,however,thatallstandardcountdatadistributionsexhibitheteroskedasticity,andnonlinearleastsquaresdoesnotexploitthis[seeWooldridge(1999,Chapter12)].Instead,wewillrelyonmaximumlikelihoodandtheimportantrelatedmethodofquasi-maximumlikelihoodestimation.InChapter4,weintroducednormalityasthestandarddistributionalassumptionforlinearregression.Thenormalityassumptionisreasonablefor(roughly)continuousdependentvariablesthatcantakeonalargerangeofvalues.Acountvariablecannothaveanormaldistribution(sincethenormaldistributionisforcontinuousvariablesthatcantakeonallvalues),andifittakesonveryfewvalues,thedistributioncanbeverydifferentfromnormal.Instead,thenominaldistributionforcountdataisthePoissondistribution.Becauseweareinterestedintheeffectofexplanatoryvariablesony,wemustlookatthePoissondistributionconditionalonx.ThePoissondistributionisentirelydeter-minedbyitsmean,soweonlyneedtospecifyE(yx).Weassumethishasthesame547\nd7/14/998:28PMPage548Part3AdvancedTopicsformas(17.28),whichwewriteinshorthandasexp(x).Then,theprobabilitythatyequalsthevalueh,conditionalonx,ishP(yhx)exp[exp(x)][exp(x)]/h!,h0,1,…,whereh!denotesfactorial(seeAppendixB).Thisdistribution,whichisthebasisforthePoissonregressionmodel,allowsustofindconditionalprobabilitiesforanyvaluesoftheexplanatoryvariables.Forexample,P(y0x)exp[exp(x)].Oncewehaveesti-matesofthej,wecanplugthemintotheprobabilitiesforvariousvaluesofx.Givenarandomsample{(xi,yi):i1,2,…,n},wecanconstructthelog-likelihoodfunction:nn()i(){yixiexp(xi)},(17.30)i1i1wherewedropthetermlog(yi!)becauseitdoesnotdependon.Thislog-likelihoodfunctionissimpletomaximize,althoughthePoissonMLEsarenotobtainedinclosedform.ThestandarderrorsofthePoissonestimatesˆjareeasytoobtainafterthelog-likelihoodfunctionhasbeenmaximized;theformulaisinthechapterappendix.Thesearereportedalongwiththeˆjbyanysoftwarepackage.WhilePoissonMLEanalysisisanaturalfirststepforcountdata,itisoftenmuchtoorestrictive.AlloftheprobabilitiesandhighermomentsofthePoissondistributionaredeterminedentirelybythemean.Inparticular,thevarianceisequaltothemean:Var(yx)E(yx).(17.31)Thisisrestrictiveandhasbeenshowntobeviolatedinmanyapplications.Fortunately,thePoissondistributionhasaverynicerobustnessproperty:whetherornotthePoissondistributionholds,westillgetconsistent,asymptoticallynormalestimatorsofthej.(ThisisanalogoustotheOLSestimator,whichisconsistentandasymptoticallynormalwhetherornotthenormalityassumptionholds;yetOLSistheMLEundernormality.)[SeeWooldridge(1999,Chapter19)fordetails.]WhenweusePoissonMLE,butwedonotassumethatthePoissondistributionisentirelycorrect,wecalltheanalysisquasi-maximumlikelihoodestimation(QMLE).ThePoissonQMLEisveryhandybecauseitisprogrammedinmanyeconometricspackages.However,unlessthePoissonvarianceassumption(17.31)holds,thestandarderrorsneedtobeadjusted.Asimpleadjustmenttothestandarderrorsisavailablewhenweassumethatthevarianceisproportionaltothemean:2Var(yx)E(yx),(17.32)22where0isanunknownparameter.When1,weobtainthePoissonvariance2assumption.When1,thevarianceisgreaterthanthemeanforallx;thisiscalledoverdispersionbecausethevarianceislargerthaninthePoissoncase,anditis548\nd7/14/998:28PMPage549Chapter17LimitedDependentVariableModelsandSampleSelectionCorrections2observedinmanyapplicationsofcountregressions.Thecase1,calledunderdis-persion,ismuchlesscommonbutisallowedin(17.32).Under(17.32),itiseasytoadjusttheusualPoissonMLEstandarderrors.LetˆjdenotethePoissonQMLEanddefinetheresidualsasuˆiyiyˆi,whereyˆiexp(ˆ0ˆ1xi1…ˆkxik)isthefittedvalue.Asusual,theresidualforobservation2iisthedifferencebetweenyianditsfittedvalue.Aconsistentestimatorofisn12(nk1)uˆi/yˆi,wherethedivisionbyyˆiistheproperheteroskedasticityadjust-i1ment,andnk1isthedfgivennobservationsandk1estimatesˆ0,ˆ1,…,ˆk.2Lettingˆbethepositivesquarerootofˆ,wemultiplytheusualPoissonstandarderrorsbyˆ.Ifˆisnotablygreaterthanone,thecorrectedstandarderrorscanbemuchbiggerthanthenominal,generallyincorrect,PoissonMLEstandarderrors.Even(17.32)isnotentirelygeneral.Justasinthelinearmodel,wecanobtainstan-darderrorsforthePoissonQMLEthatdonotrestrictthevarianceatall.[SeeWooldridge(1999)forfurtherexplanation.]UnderthePoissondistributionalassumption,wecanusethelikelihoodratiostatis-tictotestexclusionrestrictions,which,asalways,hastheformin(17.12).IfwehaveQUESTION17.4qexclusionrestrictions,thestatisticisdis-2Supposethatweobtainˆ22.HowwilltheadjustedstandardtributedapproximatelyasqundertheerrorscomparewiththeusualPoissonMLEstandarderrors?Hownull.Underthelessrestrictiveassumptionwillthequasi-LRstatisticcomparewiththeusualLRstatistic?(17.32),asimpleadjustmentisavailable(andthenwecallthestatisticthequasi-22likelihoodratiostatistic):wedivide(17.12)byˆ,whereˆisobtainedfromtheunrestrictedmodel.EXAMPLE17.3(PoissonRegressionforNumberofArrests)WenowapplythePoissonregressionmodeltothearrestdataused,amongotherplaces,inExample9.1.Thedependentvariable,narr86,isthenumberoftimesamanisarrestedduring1986.Thisvariableiszerofor1,970outofthe2,725meninthesample,andonlyeightvaluesofnarr86aregreaterthanfive.Thus,aPoissonregressionmodelismoreappro-priatethanalinearregressionmodel.Table17.3alsopresentstheresultsofOLSestimationofalinearregressionmodel.ThestandarderrorsforOLSaretheusualones;wecouldcertainlyhavemadetheserobusttoheteroskedasticity.ThestandarderrorsforPoissonregressionaretheusualmaxi-mumlikelihoodstandarderrors.Becauseˆ1.232,thestandarderrorsforPoissonregres-sionshouldbeinflatedbythisfactor(soeachcorrectedstandarderrorisabout23%higher).Forexample,amorereliablestandarderrorfortottimeis1.23(.015).0185,whichgivesatstatisticofabout1.3.Theadjustmenttothestandarderrorsreducesthesig-nificanceofallvariables,butseveralofthemarestillverystatisticallysignificant.TheOLSandPoissoncoefficientsarenotdirectlycomparable,andtheyhaveverydif-ferentmeanings.Forexample,thecoefficientonpcnvimpliesthat,ifpcnv.10,theexpectednumberofarrestsfallsby.013(pcnvistheproportionofpriorarreststhatledto549\nd7/14/998:28PMPage550Part3AdvancedTopicsTable17.3DeterminantsofNumberofArrestsforYoungMenDependentVariable:narr86IndependentLinearExponentialVariables(OLS)(PoissonQMLE)pcnv.132.402(.040)(.085)avgsen.011.024(.012)(.020)tottime.012.024(.009)(.015)ptime86.041.099(.009)(.021)qemp86.051.038(.014)(.029)inc86.0015.0081(.0003)(.0010)black.327.661(.045)(.074)hispan.194.500(.040)(.074)born60.022.051(.033)(.064)constant.577.600(.038)(.067)Log-LikelihoodValue—2,248.76R-Squared.073.077ˆ.8291.232550\nd7/14/998:28PMPage551Chapter17LimitedDependentVariableModelsandSampleSelectionCorrectionsconviction).ThePoissoncoefficientimpliesthatpcnv.10reducesexpectedarrestsbyabout4%[.402(.10).0402,andwemultiplythisby100togetthepercentageeffect].Asapolicymatter,thissuggestswecanreduceoverallarrestsbyabout4%ifwecanincreasetheprobabilityofconvictionby.1.ThePoissoncoefficientonblackimpliesthat,otherbeingfactorsequal,theexpectednumberofarrestsforablackmanisabout66%higherthanforawhiteman.Thecoeffi-cientishighlystatisticallysignificant,asisthecoefficientonhispan.AswiththeTobitapplicationinTable17.2,wereportanR-squaredforPoissonregres-sion.Thissquaredcorrelationcoefficientbetweenyiandyˆiexp(ˆ0ˆ1xi1…ˆkxik).Themotivationforthisgoodness-of-fitmeasureisthesameasfortheTobitmodel.Weseethattheexponentialregressionmodel,estimatedbyPoissonQMLE,fitsslightlybetter.RememberthattheOLSestimatesarechosentomaximizetheR-squared,butthePoissonestimatesarenot.(Theyareselectedtomaximizethelog-likelihoodfunction.)Othercountdataregressionmodelshavebeenproposedandusedinapplications,whichgeneralizethePoissondistributioninavarietyofways.Ifweareinterestedintheeffectsofthexjonthemeanresponse,thereislittlereasontogobeyondPoissonregres-sion:itissimple,oftengivesgoodresults,andhastherobustnesspropertydiscussedearlier.Infact,wecouldapplyPoissonregressiontoaythatisaTobit-likeoutcome,provided(17.28)holds.Thismightgivegoodestimatesofthemeaneffects.ExtensionsofPoissonregressionaremoreusefulwhenweareinterestedinestimatingprobabili-ties,suchasP(y1x).[See,forexample,CameronandTrivedi(1998).]17.4CENSOREDANDTRUNCATEDREGRESSIONMODELSAmodelwithasimilarstatisticalstructuretothatoftheTobitmodeliscalledthecen-soredregressionmodel.Whiletheterms“Tobit”and“censoredregression”haveoftenbeenusedinterchangeablyineconometrics,inpracticethereisaveryimportantdiffer-ence.TheTobitmodelisappliedtooutcomevariablesthatareroughlycontinuousoverpositivevaluesbuthaveapositiveprobabilityofequalingzero.Wesawanexampleofthisinthecaseofmarriedwomen’slaborsupplyinExample17.2anddiscussedotherexamplessuchasamountofcharitablecontributions.UnliketheTobitmodel,thecen-soredregressionmodelarisesduetodatacensoring.Inparticular,theunderlyingdependentvariableisroughlycontinuous—and,wewillassume,normallydistributed,conditionalontheexplanatoryvariables—butitiscensoredbeloworaboveacertainvalueduetothewaywecollectthedataortoinstitutionalconstraints.Inasense,theproblemsolvedbycensoredregressionisamissingdataproblem,butwehaveusefulinformationonthenatureofthemissingdata.Atruncatedregressionmodelariseswhenweexclude,onthebasisofy,asubsetofthepopulationinoursamplingscheme.Inotherwords,wedonothavearandomsamplefromtheunderlyingpopulation,butweknowtherulethatwasusedtoincludeunitsinthesample.Thisruleisdeterminedbywhetheryisaboveorbelowacertainthreshold.Wemorefullyexplainthedifferencebetweencensoredandtruncatedregres-sionmodelslater.551\nd7/14/998:28PMPage552Part3AdvancedTopicsCensoredRegressionModelsWhilecensoredregressionmodelscanbedefinedwithoutdistributionalassumptions,inthissubsection,westudythecensorednormalregressionmodel.Thevariablewewouldliketoexplain,y,followstheclassicallinearmodel.Foremphasis,weputanisubscriptonarandomdrawfromthepopulation:2yi0xiui,uixi,ci~Normal(0,)(17.33)wimin(yi,ci).(17.34)Ratherthanobservingyi,weonlyobserveitifitislessthanacensoringvalue,ci.Noticethat(17.33)includestheassumptionthatuiisindependentofci(atleastoncewecon-ditiononthexi).(Forconcreteness,weexplicitlyconsidercensoringfromabove,QUESTION17.5orrightcensoring;theproblemofcensor-Letmvpibethemarginalvalueproductforworkeri;thisisthepriceingfrombelow,orleftcensoring,ishan-ofafirm’sgoodmultipliedbythemarginalproductoftheworker.dledsimilarly.)Assumemvpiisalinearfunctionofexogenousvariables,suchasOneexampleofrightdatacensoringiseducation,experience,andsoon,aswellasbeinganunobservabletopcoding.Whenavariableistopcoded,error.Underperfectcompetitionandwithoutinstitutionalcon-weknowitsvalueonlyuptoacertainstraints,eachworkerispaidhisorhermarginalvalueproduct.Letminwageidenotetheminimumwageforworkeri,whichvariesbythreshold.Forresponsesgreaterthanthestate.Weobservewagei,whichisthelargerofmvpiandminwagei.threshold,weonlyknowthatthevariableWritetheappropriatemodelfortheobservedwage.isatleastaslargeasthethreshold.Forexample,insomesurveys,familywealthistopcoded.Supposethatrespondentsareaskedtheirwealth,butpeopleareallowedtorespondwith“morethan$500,000.”Then,weobserveactualwealthforthoserespondentswhosewealthislessthan$500,000butnotforthosewhosewealthisgreaterthan$500,000.Inthiscase,thecensoringthreshold,ci,isthesameforalli.Inmanysituations,thecensoringthresholdchangeswithindividualorfamilycharacter-istics.Ifweobservedarandomsamplefor(x,y),wewouldsimplyestimatebyOLS,andstatisticalinferencewouldbestandard.(Weagainabsorbtheinterceptintoxforsim-plicity.)Thecensoringcausesproblems.UsingargumentssimilartotheTobitmodel,anOLSregressionusingonlytheuncensoredobservations—thatis,thosewithyici—producesinconsistentestimatorsofthej.AnOLSregressionofwionxi,usingallobservations,doesnotconsistentlyestimatethej,unlessthereisnocensor-ing.ThisissimilartotheTobitcase,buttheproblemismuchdifferent.IntheTobitmodel,wearemodelingeconomicbehavior,whichoftenyieldszerooutcomes;theTobitmodelissupposedtoreflectthis.Withcensoredregression,wehaveadatacol-lectionproblembecause,forsomereason,thedataarecensored.2Undertheassumptionsin(17.33)and(17.34),wecanestimate(and)bymax-imumlikelihood,givenarandomsampleon(xi,wi).Forthis,weneedthedensityofwi,given(xi,ci).Foruncensoredobservations,wiyi,andthedensityofwiisthesameas2thatforyi:Normal(xi,).Forcensoredobservations,weneedtheprobabilitythatwiequalsthecensoringvalue,ci,givenxi:P(wicixi)P(yicixi)P(uicixi)1[(cixi)/].552\nd7/14/998:28PMPage553Chapter17LimitedDependentVariableModelsandSampleSelectionCorrectionsWecancombinethesetwopartstoobtainthedensityofwi,givenxiandci:f(wxi,ci)1[(cixi)/],wci,(17.35)(1/)[(wxi)/],wci.(17.36)Thelog-likelihoodforobservationiisobtainedbytakingthenaturallogofthedensityforeachi.Wecanmaximizethesumoftheseacrossi,withrespecttothejand,toobtaintheMLEs.Itisgoodtoknowthatwecaninterpretthejjustasinalinearregressionmodelunderrandomsampling.ThisismuchdifferentthantheTobitapplications,wheretheexpectationsofinterestarenonlinearfunctionsofthej.Animportantapplicationofcensoredregressionmodelsisdurationanalysis.Adurationisavariablethatmeasuresthetimebeforeacertaineventoccurs.Forexam-ple,wemightwishtoexplainthenumberofdaysbeforeafelonreleasedfromprisonisarrested.Forsomefelons,thismayneverhappen,oritmayhappenaftersuchalongtimethatwemustcensorthedurationinordertoanalyzethedata.Indurationapplicationsofcensorednormalregression,aswellasintopcoding,weoftenusethenaturallogasthedependentvariable,whichmeanswealsotakethelogofthecensoringthresholdin(17.34).Aswehaveseenthroughoutthistext,usingthelogtransformationforthedependentvariablecausestheparameterstobeinterpretedaspercentagechanges.Further,aswithmanypositivevariables,thelogofadurationtyp-icallyhasadistributionclosertonormalthanthedurationitself.EXAMPLE17.4(DurationofRecidivism)ThefileRECID.RAWcontainsdataonthetimeinmonthsuntilaninmateinaNorthCarolinaprisonisarrestedafterbeingreleasedfromprison;callthisdurat.Someinmatespartici-patedinaworkprogramwhileinprison.Wealsocontrolforavarietyofdemographicvari-ables,aswellasformeasuresofprisonandcriminalhistory.Outof1,445inmates,893hadnotbeenarrestedduringtheperiodtheywerefollowed;therefore,theseobservationsarecensored.Thecensoringtimesdifferedamonginmates,rangingfrom70to81months.Table17.4givestheresultsofcensorednormalregressionforlog(durat).Eachofthecoefficients,whenmultipliedby100,givestheestimatedpercentagechangeinexpecteddurationgivenaceterisparibusincreaseofoneunitinthecorrespondingexplanatoryvari-able.SeveralofthecoefficientsinTable17.4areinteresting.Thevariablespriors(numberofpriorconvictions)andtserved(totalmonthsspentinprison)havenegativeeffectsonthetimeuntilthenextarrestoccurs.Thissuggeststhatthesevariablesmeasureproclivityforcriminalactivityratherthanrepresentingadeterrenteffect.Forexample,aninmatewithonemorepriorconvictionhasadurationuntilnextarrestthatisalmost14%less.Ayearoftimeservedreducesdurationbyabout10012(.019)22.8%.Asomewhatsurprisingfindingisthatamanservingtimeforafelonyhasanestimatedexpecteddurationthatisalmost56%(exp(.444)1.56)longerthanamanservingtimeforanonfelony.553\nd7/14/998:28PMPage554Part3AdvancedTopicsTable17.4CensoredRegressionEstimationofCriminalRecidivismDependentVariable:log(durat)IndependentVariablesworkprg.063(.120)priors.137(.021)tserved.019(.003)felon.444(.145)alcohol.635(.144)drugs.298(.133)black.543(.117)married.341(.140)educ.023(.025)age.0039(.0006)constant4.099(0.348)Log-LikelihoodValue1,597.06ˆ1.810554\nd7/14/998:28PMPage555Chapter17LimitedDependentVariableModelsandSampleSelectionCorrectionsThosewithahistoryofdrugoralcoholabusehavesubstantiallyshorterexpecteddura-tionsuntilthenextarrest.(Thevariablesalcoholanddrugsarebinaryvariables.)Oldermen,andmenwhoweremarriedatthetimeofincarceration,areexpectedtohavesignificantlylongerdurationsuntilnextarrest.Blackmenhavesubstantiallyshorterdurations,ontheorderof42%[exp(.543)1.42].Thekeypolicyvariable,workprg,doesnothavethedesiredeffect.Thepointestimateisthat,otherthingsbeingequal,menwhoparticipatedintheworkprogramhaveesti-matedrecidivismdurationsthatareabout6.3%shorterthanmenwhodidnotparticipate.Thecoefficienthasasmalltstatistic,sowewouldprobablyconcludethattheworkpro-gramhasnoeffect.Thiscouldbeduetoaself-selectionproblem,oritcouldbeaproductofthewaymenwereassignedtotheprogram.Ofcourse,itmaysimplybethatthepro-gramwasineffective.Inthisexample,itiscrucialtoaccountforthecensoring,especiallybecausealmost62%ofthedurationsarecensored.IfweapplystraightOLStotheentiresampleandtreatthecensoreddurationsasiftheywereuncensored,thecoefficientestimatesaremarkedlydifferent.Infact,theyareallshrunktowardzero.Forexample,thecoefficientonpriorsbecomes.059(se.009),andthatonalcoholbecomes.262(se.060).Whilethedirectionsoftheeffectsarethesame,theimportanceofthesevariablesisgreatlydiminished.Thecensoredregressionestimatesaremuchmorereliable.ThereareotherwaysofmeasuringtheeffectsofeachoftheexplanatoryvariablesinTable17.4ontheduration,ratherthanfocusingonlyontheexpectedduration.Atreatmentofmoderndurationanalysisisbeyondthescopeofthistext.[Foranintro-duction,seeWooldridge(1999,Chapter20).]Ifanyoftheassumptionsofthecensorednormalregressionmodelareviolated—inparticular,ifthereisheteroskedasticityornonnormality—theMLEsaregenerallyinconsistent.Thisshowsthatthecensoringispotentiallyverycostly,asOLSusinganuncensoredsamplerequiresneithernormalitynorhomoskedasticityforconsistency.Therearemethodsthatdonotrequireustoassumeadistribution,buttheyaremoreadvanced.[SeeWooldridge(1999,Chapter16).]TruncatedRegressionModelsAtruncatedregressionmodelissimilartoacensoredregressionmodel,butitdiffersinonemajorrespect:inatruncatedregressionmodel,wedonotobserveanyinformationaboutacertainsegmentofthepopulation.Thistypicallyhappenswhenasurveytargetsaparticularsubsetofthepopulationand,perhapsduetocostconsiderations,entirelyignorestheotherpartofthepopulation.Forexample,HausmanandWise(1977)useddatafromanegativeincometaxexperimenttostudyvariousdeterminantsofearnings.Tobeincludedinthestudy,afamilyhadtohaveincomelessthan1.5timesthe1967povertyline,wherethepovertylinedependedonfamilysize.Thetruncatednormalregressionmodelbeginswithanunderlyingpopulationmodelthatsatisfiestheclassicallinearmodelassumptions:555\nd7/14/998:28PMPage556Part3AdvancedTopics2y0xu,ux~Normal(0,).(17.37)Recallthatthisisastrongsetofassumptions,becauseumustnotonlybeindependentofx,butalsonormallydistributed.Wefocusonthismodelbecauserelaxingtheassumptionsisdifficult.Under(17.37)weknowthat,givenarandomsamplefromthepopulation,OLSisthemostefficientestimationprocedure.Theproblemarisesbecausewedonotobservearandomsamplefromthepopulation:AssumptionMLR.2isviolated.Inparticular,arandomdraw(xi,yi)isobservedonlyifyici,whereciisthetruncationthresholdthatcandependonexogenousvariables—inparticular,thexi.(IntheHausmanandWiseexample,cidependsonfamilysize.)Thismeansthat,if{(xi,yi):i1,…,n}isourobservedsample,thenyiisnecessarilylessthanorequaltoci.Thisdiffersfromthecen-soredregressionmodel,whereyicanbelargerthanci;wesimplydonotobserveyiifyici.Inacensoredregressionmodel,weobservexiforanyrandomlydrawnobser-vationfromthepopulation;inthetruncatedmodel,weonlyobservexiifyici.Toestimatethej(alongwith),weneedthedistributionofyi,giventhatyiciandxi.Thisiswrittenas2f(yxi,)g(yxi,ci)2,yci,(17.38)F(cixi,)22wheref(yxi,)denotesthenormaldensitywithmean0xiandvariance,and2F(cixi,)isthenormalcdfwiththesamemeanandvariance,evaluatedatci.Thisexpressionforthedensity,conditionalonyici,makesintuitivesense:itisthepopu-lationdensityfory,givenx,dividedbytheprobabilitythatyiislessthanorequaltoci(givenxi),P(yicixi).Ineffect,werenormalizethedensitybydividingbythearea2underf(|xi,)thatistotheleftofci.Ifwetakethelogof(17.38),sumacrossalli,andmaximizetheresultwithrespect2tothejand,weobtainthemaximumlikelihoodestimators.Thisleadstoconsis-tent,approximatelynormalestimators.Theinference,includingstandarderrorsandlog-likelihoodstatistics,isstandard.WecouldanalyzethedatafromExample17.4asatruncatedsampleifwedropalldataonanobservationwheneveritiscensored.Thiswouldgiveus552observationsfromatruncatednormaldistribution,wherethetruncationpointdiffersacrossi.However,wewouldneveranalyzedurationdata(ortopcodeddata)inthisway,asiteliminatesusefulinformation.Thefactthatweknowalowerboundfor893durations,alongwiththeexplanatoryvariables,isusefulinformation;censoredregressionusesthisinformation,whiletruncatedregressiondoesnot.AbetterexampleisgiveninHausmanandWise(1977),wheretheyemphasizethatOLSappliedtoasampletruncatedfromabovegenerallyproducesestimatorsbiasedtowardzero.Intuitively,thismakessense.Supposethattherelationshipofinterestisbetweenincomeandeducationlevels.Ifweonlyobservepeoplewhoseincomeisbelowacertainthreshold,weareloppingofftheupperend.Thistendstoflattentheestimatedlinerelativetothetrueregressionlineinthewholepopulation.SeeFigure17.2forthecaseofasingleexplanatoryvariableandthesametruncationpointforeachobservation.556\nd7/14/998:28PMPage557Chapter17LimitedDependentVariableModelsandSampleSelectionCorrectionsFigure17.2Atrue,orpopulationregressionline,andtheincorrectregressionlineforthetruncatedpopulationwithincomesbelow$50,000.income(inthousands150ofdollars)trueregressionline50regressionlinefortruncatedpopulation151020educ(inyears)Aswithcensoredregression,iftheunderlyinghomoskedasticnormalassumptionin(17.37)isviolated,thetruncatednormalMLEisbiasedandinconsistent.Methodsthatdonotrequiretheseassumptionsareavailable;seeWooldridge(1999,Chapter17)fordiscussionandreferences.17.5SAMPLESELECTIONCORRECTIONSTruncatedregressionisaspecialcaseofageneralproblemknownasnonrandomsam-pleselection.Butsurveydesignisnottheonlycauseofnonrandomsampleselection.Often,respondentsfailtoprovideanswerstocertainquestions,whichleadstomissingdataforthedependentorindependentvariables.Becausewecannotusetheseobserva-tionsinourestimation,weshouldwonderwhetherdroppingthemleadstobiasinourestimators.Anothergeneralexampleisusuallycalledincidentaltruncation.Here,wedonotobserveybecauseoftheoutcomeofanothervariable.Theleadingexampleisestimat-ingtheso-calledwageofferfunctionfromlaboreconomics.Interestliesinhowvariousfactors,suchaseducation,affectthewageanindividualcouldearninthelaborforce.Forpeoplewhoareintheworkforce,weobservethewageofferasthecurrentwage.But,forthosecurrentlyoutoftheworkforce,wedonotobservethewageoffer.557\nd7/14/998:28PMPage558Part3AdvancedTopicsBecauseworkingmaybesystematicallycorrelatedwithunobservablesthataffectthewageoffer,usingonlyworkingpeople—aswehaveinallwageexamplessofar—mightproducebiasedestimatorsoftheparametersinthewageofferequation.Nonrandomsampleselectioncanalsoarisewhenwehavepaneldata.Inthesim-plestcase,wehavetwoyearsofdata,but,duetoattrition,somepeopleleavethesam-ple.Thisisparticularlyaprobleminpolicyanalysis,whereattritionmayberelatedtotheeffectivenessofaprogram.WhenisOLSontheSelectedSampleConsistent?InSection9.4,weprovidedabriefdiscussionofthekindsofsampleselectionthatcanbeignored.Thekeydistinctionisbetweenexogenousandendogenoussampleselection.InthetruncatedTobitcase,weclearlyhaveendogenoussampleselection,andOLSisbiasedandinconsistent.Ontheotherhand,ifoursampleisdeterminedsolelybyanexogenousexplanatoryvariable,wehaveexogenoussampleselection.Casesbetweentheseextremesarelessclear,andwenowprovidecarefuldefinitionsandassumptionsforthem.Thepopulationmodelisy01x1…kxku,E(ux1,x2,…,xk)0.(17.39)Itisusefultowritethepopulationmodelforarandomdrawasyixiui,(17.40)whereweusexiasshorthandfor01xi12xi2…kxik.Now,letnbethesizeofarandomsamplefromthepopulation.Ifwecouldobserveyiandeachxijforalli,wewouldsimplyuseOLS.Assumethat,forsomereason,eitheryiorsomeoftheindependentvariablesarenotobservedforcertaini.Foratleastsomeobservations,weobservethefullsetofvariables.Defineaselectionindicatorsiforeachibysi1ifweobserveallof(yi,xi),andsi0otherwise.Thus,si1indicatesthatwewillusetheobservationinouranalysis;si0meanstheobservationwillnotbeused.WeareinterestedinthestatisticalpropertiesoftheOLSestimatorsusingtheselectedsample,thatis,usingobservationsforwhichsi1.Therefore,weusefewerthannobserva-tions,sayn1.ItturnsouttobeeasytoobtainconditionsunderwhichOLSisconsistent(andevenunbiased).Effectively,ratherthanestimating(17.40),wecanonlyestimatetheequa-tionsiyisixisiui.(17.41)Whensi1,wesimplyhave(17.40);whensi0,wesimplyhave000,whichclearlytellsusnothingabout.Regressingsiyionsixifori1,2,…,nisthesameasregressingyionxiusingtheobservationsforwhichsi1.Thus,wecanlearnabouttheconsistencyoftheˆjbystudying(17.41)onarandomsample.FromouranalysisinChapter5,theOLSestimatorsfrom(17.41)areconsistentiftheerrortermhaszeromeanandisuncorrelatedwitheachexplanatoryvariable.Inthe558\nd7/14/998:28PMPage559Chapter17LimitedDependentVariableModelsandSampleSelectionCorrectionspopulation,thezeromeanassumptionisE(su)0,andthezerocorrelationassump-tionscanbestatedasE[(sxj)(su)]E(sxju)0,(17.42)wheres,xj,anduarerandomvariablesrepresentingthepopulation;wehaveusedthe2factthatssbecausesisabinaryvariable.Condition(17.42)isdifferentfromwhatweneedifweobserveallvariablesforarandomsample:E(xju)0.Therefore,inthepopulation,weneedutobeuncorrelatedwithsxj.ThekeyconditionforunbiasednessisE(susx1,…,sxk)0.Asusual,thisisastrongerassumptionthanthatneededforconsistency.Ifsisafunctiononlyoftheexplanatoryvariables,thensxjisjustafunctionofx1,x2,…,xk;bytheconditionalmeanassumptionin(17.39),sxjisalsouncorrelatedwithu.Infact,E(susx1,…,sxk)sE(usx1,…,sxk)0,becauseE(ux1,…,xk)0.Thisisthecaseofexogenoussampleselection,wheresi1isdeterminedentirelybyxi1,…,xik.Asanexample,ifweareestimatingawageequationwheretheexplanatoryvariablesareeducation,experience,tenure,gender,maritalstatus,andsoon—whichareassumedtobeexogenous—wecanselectthesampleonthebasisofanyoralloftheexplanatoryvariables.Ifsampleselectionisentirelyrandominthesensethatsiisindependentof(xi,ui),thenE(sxju)E(s)E(xju)0,becauseE(xju)0under(17.39).Therefore,ifwebeginwitharandomsampleandrandomlydropobservations,OLSisstillconsistent.Infact,OLSisagainunbiasedinthiscase,providedthereisnotperfectmulticollinearityintheselectedsample.Ifsdependsontheexplanatoryvariablesandadditionalrandomtermsthatareinde-pendentofxandu,OLSisalsoconsistentandunbiased.Forexample,supposethatIQscoreisanexplanatoryvariableinawageequation,butIQismissingforsomepeople.Supposewethinkthatselectioncanbedescribedbys1ifIQv,ands0ifIQv,wherevisanunobservedrandomvariablethatisindependentofIQ,u,andtheotherexplanatoryvariables.ThismeansthatwearemorelikelytoobserveanIQthatishigh,butthereisalwayssomechanceofnotobservinganyIQ.Conditionalontheexplanatoryvariables,sisindependentofu,whichmeansthatE(ux1,…,xk,s)E(ux1,…,xk),andthelastexpectationiszerobyassumptiononthepopulationmodel.222IfweaddthehomoskedasticityassumptionE(ux,s)E(u),thentheusualOLSstandarderrorsandteststatisticsarevalid.Sofar,wehaveshownseveralsituationswhereOLSontheselectedsampleisunbi-ased,oratleastconsistent.WhenisOLSontheselectedsampleinconsistent?Wealreadysawoneexample:regressionusingatruncatedsample.Whenthetruncationisfromabove,si1ifyici,whereciisthetruncationthreshold.Equivalently,si1ifuicixi.Becausesidependsdirectlyonui,sianduiwillnotbeuncorrelated,evenconditionalonxi.ThisiswhyOLSontheselectedsampledoesnotconsistentlyestimatethej.Therearelessobviouswaysthatsanducanbecorrelated;weconsiderthisinthenextsubsection.TheresultsonconsistencyofOLSextendtoinstrumentalvariablesestimation.IftheIVsaredenotedzhinthepopulation,thekeyconditionforconsistencyof2SLSisE(szhu)0,whichholdsifE(uz,s)0.Therefore,ifselectionisdeterminedentirely559\nd7/14/998:28PMPage560Part3AdvancedTopicsbytheexogenousvariablesz,orifsdependsonotherfactorsthatareindependentofuandz,then2SLSontheselectedsampleisgenerallyconsistent.Wedoneedtoassumethattheexplanatoryandinstrumentalvariablesareappropriatelycorrelatedintheselectedpartofthepopulation.Wooldridge(1999,Chapter17)containsprecisestate-mentsoftheseassumptions.Itcanalsobeshownthat,whenselectionisentirelyafunctionoftheexogenousvariables,maximumlikelihoodestimationofanonlinearmodel—suchasalogitorpro-bitmodel—producesconsistent,asymptoticallynormalestimators,andtheusualstan-darderrorsandteststatisticsarevalid.[Again,seeWooldridge(1999,Chapter17).]IncidentalTruncationAswementionedearlier,acommonformofsampleselectioniscalledincidentaltrun-cation.Weagainstartwiththepopulationmodelin(17.39).However,weassumethatwewillalwaysobservetheexplanatoryvariablesxj.Theproblemis,weonlyobserveyforasubsetofthepopulation.Theruledeterminingwhetherweobserveydoesnotodependdirectlyontheoutcomeofy.Aleadingexampleiswhenylog(wage),whereowageisthewageoffer,orthehourlywagethatanindividualcouldreceiveinthelabormarket.Ifthepersonisactuallyworkingatthetimeofthesurvey,thenweobservethewageofferbecauseweassumeitistheobservedwage.Butforpeopleoutoftheworkoforce,wecannotobservewage.Therefore,thetruncationofwageofferisincidentalbecauseitdependsonanothervariable,namely,laborforceparticipation.Importantly,wewouldgenerallyobserveallotherinformationaboutanindividual,suchaseduca-tion,priorexperience,gender,maritalstatus,andsoon.Theusualapproachtoincidentaltruncationistoaddanexplicitselectionequationtothepopulationmodelofinterest:yxu,E(ux)0(17.43)s1[zv0],(17.44)wheres1ifweobservey,andzerootherwise.Weassumethatelementsofxandzarealwaysobserved,andwewritex01x1…kxkandz01z1…mzm.Theequationofprimaryinterestis(17.43),andwecouldestimatebyOLSgivenarandomsample.Theselectionequation,(17.44),dependsonobservedvariables,zh,andanunobservederror,v.Astandardassumption,whichwewillmake,isthatzisexogenousin(17.43):E(ux,z)0.Infact,forthefollowingproposedmethodstoworkwell,wewillrequirethatxbeastrictsubsetofz:anyxjisalsoanelementofz,andwehavesomeelementsofzthatarenotalsoinx.Wewillseelaterwhythisiscrucial.Theerrortermvinthesampleselectionequationisassumedtobeindependentofz(andthereforex).Wealsoassumethatvhasastandardnormaldistribution.Wecaneasilyseethatcorrelationbetweenuandvgenerallycausesasampleselectionproblem.Toseewhy,assumethat(u,v)isindependentofz.Then,takingtheexpectationof(17.43),conditionalonzandv,andusingthefactthatxisasubsetofzgives560\nd7/14/998:28PMPage561Chapter17LimitedDependentVariableModelsandSampleSelectionCorrectionsE(yz,v)xE(uz,v)xE(uv),whereE(uz,v)E(uv)because(u,v)isindependentofz.Now,ifuandvarejointlynormal(withzeromean),thenE(uv)vforsomeparameter.Therefore,E(yz,v)xv.Wedonotobservev,butwecanusethisequationtocomputeE(yz,s)andthenspe-cializethistos1.Wenowhave:E(yz,s)xE(vz,s).Becausesandvarerelatedby(17.44),andvhasastandardnormaldistribution,wecanshowthatE(vz,s)issimplytheinverseMillsratio,(z),whens1.ThisleadstotheimportantequationE(yz,s1)x(z).(17.45)Equation(17.45)showsthattheexpectedvalueofy,givenzandobservabilityofy,isequaltox,plusanadditionaltermthatdependsontheinverseMillsratioevaluatedatz.Remember,wehopetoestimate.Thisequationshowsthatwecandosousingonlytheselectedsample,providedweincludetheterm(z)asanadditionalregres-sor.If0,(z)doesnotappear,andOLSofyonxusingtheselectedsamplecon-sistentlyestimates.Otherwise,wehaveeffectivelyomittedavariable,(z),whichisgenerallycorrelatedwithx.Whendoes0?Theansweriswhenuandvareuncor-related.Becauseisunknown,wecannotevaluate(zi)foreachi.However,fromtheassumptionswehavemade,sgivenzfollowsaprobitmodel:P(s1z)(z).(17.46)Therefore,wecanestimatebyprobitofsionzi,usingtheentiresample.Inasecondstep,wecanestimate.Wesummarizetheprocedure,whichhasrecentlybeendubbedtheHeckitmethodintheeconometricsliteratureaftertheworkofHeckman(1976).SAMPLESELECTIONCORRECTION(i)Usingallnobservations,estimateaprobitmodelofsionziandobtaintheesti-matesˆh.ComputetheinverseMillsratio,ˆi(ziˆ)foreachi.(Actually,weonlyneedthesefortheiwithsi1.)(ii)Usingtheselectedsample,thatis,theobservationsforwhichsi1(say,n1ofthem),runtheregressionofyionxi,ˆi.(17.47)Theˆjareconsistentandapproximatelynormallydistributed.561\nd7/14/998:28PMPage562Part3AdvancedTopicsAsimpletestofselectionbiasisavailablefromregression(17.47).Namely,wecanusetheusualtstatisticonˆiasatestofH0:0.UnderH0,thereisnosampleselec-tionproblem.When0,theusualOLSstandarderrorsreportedfrom(17.47)arenotexactlycorrect.Thisisbecausetheydonotaccountforestimationof,whichusesthesameobservationsinregression(17.47),andmore.Someeconometricspackagescomputecorrectedstandarderrors.[Unfortunately,itisnotassimpleasaheteroskedasticityadjustment.SeeWooldridge(1999,Chapter6)forfurtherdiscussion.]Inmanycases,theadjustmentsdonotleadtoimportantdifferences,butitishardtoknowthatbefore-hand(unlessˆissmallandinsignificant).Werecentlymentionedthatxshouldbeastrictsubsetofz.Thishastwoimplica-tions.First,anyelementthatappearsasanexplanatoryvariablein(17.43)shouldalsobeanexplanatoryvariableintheselectionequation.Whileinrarecasesitmakessensetoexcludeelementsfromtheselectionequation,includingallelementsofxinzisnotverycostly;excludingthemcanleadtoinconsistencyiftheyareincorrectlyexcluded.Asecondmajorimplicationisthatwehaveatleastoneelementofzthatisnotalsoinx.Thismeansthatweneedavariablethataffectsselectionbutdoesnothaveapar-tialeffectony.Thisisnotabsolutelynecessarytoapplytheprocedure—infact,wecanmechanicallycarryoutthetwostepswhenzx—buttheresultsareusuallylessthanconvincingunlesswehaveanexclusionrestrictionin(17.43).ThereasonforthisisthatwhiletheinverseMillsratioisanonlinearfunctionofz,itisoftenwell-approximatedbyalinearfunction.Ifzx,ˆicanbehighlycorrelatedwiththeelementsofxi.Asweknow,suchmulticollinearitycanleadtoveryhighstandarderrorsfortheˆj.Intuitively,ifwedonothaveavariablethataffectsselectionbutnoty,itisextremelydifficult,ifnotimpossible,todistinguishsampleselectionfromamisspecifiedfunctionalformin(17.43).EXAMPLE17.5(WageOfferEquationforMarriedWomen)WeapplythesampleselectioncorrectiontothedataonmarriedwomeninMROZ.RAW.Recallthatofthe753womeninthesample,428workedforawageduringtheyear.Thewageofferequationisstandard,withlog(wage)asthedependentvariableandeduc,exper,2andexperastheexplanatoryvariables.Inordertotestandcorrectforsampleselectionbias—duetounobservabilityofthewageofferfornonworkingwomen—weneedtoesti-mateaprobitmodelforlaborforceparticipation.Inadditiontotheeducationandexperi-encevariables,weincludethefactorsinTable17.1:otherincome,age,numberofyoungchildren,andnumberofolderchildren.Thefactthatthesefourvariablesareexcludedfromthewageofferequationisanassumption:weassumethat,giventheproductivityfactors,nwifeinc,age,kidslt6,andkidsge6havenoeffectonthewageoffer.ItisclearfromtheprobitresultsinTable17.1thatatleastageandkidslt6haveastrongeffectonlaborforceparticipation.Table17.5containstheresultsfromOLSandHeckit.[ThestandarderrorsfortheHeckitresultsarejusttheusualOLSstandarderrorsfromregression(17.47).]Thereisnoevidenceofasampleselectionprobleminestimatingthewageofferequation.Thecoefficientonˆhasaverysmalltstatistic(.239),andsowefailtorejectH0:0.Justasimportantly,there562\nd7/14/998:28PMPage563Chapter17LimitedDependentVariableModelsandSampleSelectionCorrectionsTable17.5WageOfferEquationforMarriedWomenDependentVariable:log(wage)IndependentVariablesOLSHeckiteduc.108.109(.014)(.016)exper.042.044(.012)(.016)2exper.00081.00086(.00039)(.00044)constant.522.578(.199)(.307)ˆ—.032(.134)SampleSize428428R-Squared.157.157arenopracticallylargedifferencesintheestimatedslopecoefficientsinTable17.5.Theesti-matedreturnstoeducationdifferbyonlyone-tenthofapercentagepoint.Analternativetotheprecedingtwo-stepestimationmethodisfullmaximumlikeli-hoodestimation.Thisismorecomplicatedasitrequiresobtainingthejointdistributionofyands.Itoftenmakessensetotestforsampleselectionusingthepreviousproce-dure;ifthereisnoevidenceofsampleselection,thereisnoreasontocontinue.Ifwedetectsampleselectionbias,wecaneitherusethetwo-stepestimatesorestimatetheregressionandselectionequationsjointlybyMLE.[SeeWooldridge(1999,Chapter17).]InExample17.5,weknowmorethanjustwhetherawomanworkedduringtheyear:weknowhowmanyhourseachwomanworked.Itturnsoutthatwecanusethisinformationinanalternativesampleselectionprocedure.InplaceoftheinverseMillsratioˆi,weusetheTobitresiduals,sayvˆi,whicharecomputedasvˆiyixiˆwhen-everyi0.Itcanbeshownthattheregressionin(17.47)withvˆiinplaceofˆialsopro-ducesconsistentestimatesofthej,andthestandardtstatisticonvˆiisavalidtestfor563\nd7/14/998:28PMPage564Part3AdvancedTopicssampleselectionbias.Thisapproachhastheadvantageofusingmoreinformation,butitislesswidelyapplicable.[SeeWooldridge(1999,Chapter17).]Therearemanymoretopicsconcerningsampleselection.Oneworthmentioningismodelswithendogenousexplanatoryvariablesinadditiontopossiblesampleselectionbias.Writeamodelwithasingleendogenousexplanatoryvariableasy11y2z11u1,(17.48)wherey1isonlyobservedwhens1,andy2mayonlybeobservedalongwithy1.Anexampleiswheny1isthepercentageofvotesreceivedbyanincumbent,andy2isthepercentoftotalexpendituresaccountedforbytheincumbent.Forincumbentswhodonotrun,wecannotobservey1ory2.Ifwehaveexogenousfactorsthataffectthedeci-siontorunandthatarecorrelatedwithcampaignexpenditures,wecanconsistentlyestimate1andtheelementsof1byinstrumentalvariables.Tobeconvincing,weneedtwoexogenousvariablesthatdonotappearin(17.48).Effectively,oneshouldaffecttheselectiondecision,andoneshouldbecorrelatedwithy2[theusualrequire-mentforestimating(17.48)by2SLS].Briefly,themethodistoestimatetheselectionequationbyprobit,whereallexogenousvariablesappearintheprobitequation.Then,weaddtheinverseMillsratioto(17.48)andestimatetheequationby2SLS.TheinverseMillsratioactsasitsowninstrument,asitdependsonlyonexogenousvariables.Weuseallexogenousvariablesastheotherinstruments.Asbefore,wecanusethetstatis-ticonˆiasatestforselectionbias.[SeeWooldridge(1999,Chapter17)forfurtherinformation.]SUMMARYInthischapter,wehavecoveredseveraladvancedmethodsthatareoftenusedinappli-cations,especiallyinmicroeconomics.Logitandprobitmodelsareusedforbinaryresponsevariables.Thesemodelshavesomeadvantagesoverthelinearprobabilitymodel:fittedprobabilitiesarebetweenzeroandone,andthepartialeffectsdiminish.Theprimarycosttologitandprobitisthattheyarehardertointerpret.TheTobitmodelisapplicabletononnegativeoutcomesthatpileupatzerobutalsotakeonabroadrangeofpositivevalues.Manyindividualchoicevariables,suchaslaborsupply,amountoflifeinsurance,andamountofpensionfundinvestedinstocks,havethisfeature.Aswithlogitandprobit,theexpectedvaluesofygivenx—eithercon-ditionalony0orunconditionally—dependonxandinnonlinearways.Wegavetheexpressionsfortheseexpectationsaswellasformulasforthepartialeffectsofeachxjontheexpectations.ThesecanbeestimatedaftertheTobitmodelhasbeenestimatedbymaximumlikelihood.Whenthedependentvariableisacountvariable—thatis,ittakesonnonnegative,integervalues—aPoissonregressionmodelisappropriate.Theexpectedvalueofygiventhexjhasanexponentialform.Thisgivestheparameterinterpretationsassemi-elasticitiesorelasticities,dependingonwhetherxjisinlevelorlogarithmicform.Inshort,wecaninterprettheparametersasiftheyareinalinearmodelwithlog(y)asthedependentvariable.TheparameterscanbeestimatedbyMLE.However,becausethePoissondistributionimposesequalityofthevarianceandmean,itisoftennecessaryto564\nd7/14/998:28PMPage565Chapter17LimitedDependentVariableModelsandSampleSelectionCorrectionscomputestandarderrorsandteststatisticsthatallowforover-orunderdispersion.ThesearesimpleadjustmentstotheusualMLEstandarderrorsandstatistics.Censoredandtruncatedregressionmodelshandlespecifickindsofmissingdataproblems.Incensoredregression,thedependentvariableiscensoredaboveorbelowathreshold.Wecanuseinformationonthecensoredoutcomesbecausewealwaysobservetheexplanatoryvariables,asindurationapplicationsortopcodingofobserva-tions.Atruncatedregressionmodelariseswhenapartofthepopulationisexcludedentirely:weobservenoinformationonunitsthatarenotcoveredbythesamplingscheme.Thisisaspecialcaseofasampleselectionproblem.Section17.5givesasystematictreatmentofnonrandomsampleselection.WeshowedthatexogenoussampleselectiondoesnotaffectconsistencyofOLSwhenitisappliedtothesubsample,butendogenoussampleselectiondoes.Weshowedhowtotestandcorrectforsampleselectionbiasforthegeneralproblemofincidentaltrunca-tion,whereobservationsaremissingonyduetotheoutcomeofanothervariable(suchaslaborforceparticipation).Heckman’smethodisrelativelyeasytoimplementinthesesituations.KEYTERMSBinaryResponseModelsMaximumLikelihoodEstimation(MLE)CensoredRegressionModelNonrandomSampleSelectionCornerSolutionsOverdispersionCountVariablePercentCorrectlyPredictedDataCensoringPoissonDistributionDurationAnalysisPoissonRegressionModelExogenousSampleSelectionProbitModelHeckitMethodPseudoR-SquaredIncidentalTruncationQuasi-LikelihoodRatioStatisticInverseMillsRatioQuasi-MaximumLikelihoodEstimationLatentVariableModel(QMLE)LikelihoodRatioStatisticSelectedSampleLimitedDependentVariable(LDV)TobitModelLogitModelTopCodingLog-LikelihoodFunctionTruncatedRegressionModelPROBLEMS17.1(i)Forabinaryresponsey,lety¯betheproportionofonesinthesample(whichisequaltothesampleaverageoftheyi).Letqˆ0bethepercentcorrectlypre-dictedfortheoutcomey0andletqˆ1bethepercentcorrectlypredictedfortheoutcomey1.Ifpˆistheoverallpercentcorrectlypredicted,showthatpˆisaweightedaverageofqˆ0andqˆ1:pˆ(1y¯)qˆ0y¯qˆ1.(ii)Inasampleof300,supposethaty¯.70,sothatthereare210outcomeswithyi1and90withyi0.Supposethatthepercentcorrectlypre-565\nd7/14/998:28PMPage566Part3AdvancedTopicsdictedwheny0is80,andthepercentcorrectlypredictedwheny1is40.Findtheoverallpercentcorrectlypredicted.17.2Letgradbeadummyvariableforwhetherastudent-athleteatalargeuniversitygraduatesinfiveyears.LethsGPAandSATbehighschoolgradepointaverageandSATscore.Letstudybethenumberofhoursspentperweekinorganizedstudyhall.Supposethat,usingdataon420student-athletes,thefollowinglogitmodelisobtained:ˆP(grad1hsGPA,SAT,study)(1.17.24hsGPA.00058SAT.073study),where(z)exp(z)/[1exp(z)]isthelogitfunction.HoldinghsGPAfixedat3.0andSATfixedat1,200,computetheestimateddifferenceinthegraduationprobabilityforsomeonewhospent10hoursperweekinstudyhallandsomeonewhospentfivehoursperweek.17.3(Requirescalculus)(i)SupposeintheTobitmodelthatx1log(z1),andthisistheonlyplacez1appearsinx.ShowthatE(yy0,x)(1/z1){1(x/)[x/(x/)]},(17.49)z1where1isthecoefficientonlog(z1).2(ii)Ifx1z1,andx2z1,showthatE(yy0,x)(122z1){1(x/)[x/(x/)]},z12where1isthecoefficientonz1,and2isthecoefficientonz1.17.4Letmvpibethemarginalvalueproductforworkeri,whichisthepriceofafirm’sgoodmultipliedbythemarginalproductoftheworker.Assumethatlog(mvpi)01xi1…kxikuiwageimax(mvpi,minwagei),wheretheexplanatoryvariablesincludeeducation,experience,andsoon,andminwageiistheminimumwagerelevantforpersoni.Writelog(wagei)intermsoflog(mvpi)andlog(minwagei).17.5(Requirescalculus)Letpatentsbethenumberofpatentsappliedforbyafirmduringagivenyear.AssumethattheconditionalexpectationofpatentsgivensalesandRDis2E(patentssales,RD)exp[01log(sales)2RD3RD],wheresalesisannualfirmsales,andRDistotalspendingonresearchanddevelopmentoverthepast10years.(i)Howwouldyouestimatethej?Justifyyouranswerbydiscussingthenatureofpatents.566\nd7/14/998:28PMPage567Chapter17LimitedDependentVariableModelsandSampleSelectionCorrections(ii)Howdoyouinterpret1?(iii)FindthepartialeffectofRDonE(patentssales,RD).17.6ConsiderafamilysavingfunctionforthepopulationofallfamiliesintheUnitedStates:sav01inc2hhsize3educ4ageu,wherehhsizeishouseholdsize,educisyearsofeducationofthehouseholdhead,andageisageofthehouseholdhead.AssumethatE(uinc,hhsize,educ,age)0.(i)Supposethatthesampleincludesonlyfamilieswhoseheadisover25yearsold.IfweuseOLSonsuchasample,dowegetunbiasedestima-torsofthej?Explain.(ii)Nowsupposeoursampleincludesonlymarriedcoupleswithoutchil-dren.Canweestimatealloftheparametersinthesavingequation?Whichonescanweestimate?(iii)Supposeweexcludefromoursamplefamiliesthatsavemorethan$25,000peryear.DoesOLSproduceconsistentestimatorsofthej?17.7Supposeyouarehiredbyauniversitytostudythefactorsthatdeterminewhetherstudentsadmittedtotheuniversityactuallycometotheuniversity.Youaregivenalargerandomsampleofstudentswhowereadmittedthepreviousyear.Youhaveinformationonwhethereachstudentchosetoattend,highschoolperformance,familyincome,finan-cialaidoffered,race,andgeographicvariables.Someonesaystoyou,“Anyanalysisofthatdatawillleadtobiasedresultsbecauseitisnotarandomsampleofallcollegeappli-cants,butonlythosewhoapplytothisuniversity.”Whatdoyouthinkofthiscriticism?COMPUTEREXERCISES17.8UsethedatainPNTSPRD.RAWforthisexercise.(i)ThevariablefavwinisabinaryvariableiftheteamfavoredbytheLasVegaspointspreadwins.AlinearprobabilitymodeltoestimatetheprobabilitythatthefavoredteamwinsisP(favwin1spread)01spread.Explainwhy,ifthespreadincorporatesallrelevantinformation,weexpect0.5.(ii)Estimatethemodelfrompart(i)byOLS.TestH0:0.5againstatwo-sidedalternative.Useboththeusualandheteroskedasticity-robuststandarderrors.(iii)Isspreadstatisticallysignificant?Whatistheestimatedprobabilitythatthefavoredteamwinswhenspread10?(iv)Now,estimateaprobitmodelforP(favwin1spread).Interpretandtestthenullhypothesisthattheinterceptiszero.[Hint:Rememberthat(0).5.](v)Usetheprobitmodeltoestimatetheprobabilitythatthefavoredteamwinswhenspread10.ComparethiswiththeLPMestimatefrompart(iii).567\nd7/14/998:28PMPage568Part3AdvancedTopics(vi)Addthevariablesfavhome,fav25,andund25totheprobitmodelandtestjointsignificanceofthesevariablesusingthelikelihoodratiotest.(Howmanydfareinthechi-squaredistribution?)Interpretthisresult,focusingonthequestionofwhetherthespreadincorporatesallobserv-ableinformationpriortoagame.17.9UsethedatainLOANAPP.RAWforthisexercise;seealsoProblem7.16.(i)Estimateaprobitmodelofapproveonwhite.Findtheestimatedprob-abilityofloanapprovalforbothwhitesandnonwhites.Howdothesecomparewiththelinearprobabilityestimates?(ii)Now,addthevariableshrat,obrat,loanprc,unem,male,married,dep,sch,cosign,chist,pubrec,mortlat1,mortlat2,andvrtotheprobitmodel.Istherestatisticallysignificantevidenceofdiscriminationagainstnonwhites?(iii)Estimatethemodelfrompart(ii)bylogit.Comparethecoefficientonwhitetotheprobitestimate.(iv)Howwouldyoucomparethesizeofthediscriminationeffectbetweenprobitandlogit?17.10UsethedatainFRINGE.RAWforthisexercise.(i)Forwhatpercentageoftheworkersinthesampleispensionequaltozero?Whatistherangeofpensionforworkerswithnonzeropensionbenefits?WhyisaTobitmodelappropriateformodelingpension?(ii)EstimateaTobitmodelexplainingpensionintermsofexper,age,tenure,educ,depends,married,white,andmale.Dowhitesandmaleshavestatisticallysignificanthigherexpectedpensionbenefits?(iii)Usetheresultsfrompart(ii)toestimatethedifferenceinexpectedpen-sionbenefitsforawhitemaleandanonwhitefemale,bothofwhomare35yearsold,singlewithnodependents,have16yearsofeducation,and10yearsofexperience.(iv)AdduniontotheTobitmodelandcommentonitssignificance.(v)ApplytheTobitmodelfrompart(iv)butwithperatio,thepension-earningsratio,asthedependentvariable.(Noticethatthisisafractionbetweenzeroandone,but,whileitoftentakesonthevaluezero,itnevergetsclosetobeingunity.Thus,aTobitmodelisfineasanapproxima-tion.)Doesgenderorracehaveaneffectonthepension-earningsratio?22217.11InExample9.1,weaddedthequadratictermspcnv,ptime86,andinc86toalinearmodelfornarr86.(i)UsethedatainCRIME1.RAWtoaddthesesametermstothePoissonregressioninExample17.3.n2212(ii)Computetheestimateofgivenbyˆ(nk1)uˆi/yˆi.Isi1thereevidenceofoverdispersion?HowshouldthePoissonMLEstan-darderrorsbeadjusted?(iii)Usetheresultsfromparts(i)and(ii)andTable17.3tocomputethequasi-likelihoodratiostatisticforjointsignificanceofthethreequa-draticterms.Whatdoyouconclude?568\nd7/14/998:28PMPage569Chapter17LimitedDependentVariableModelsandSampleSelectionCorrections17.12RefertoTable13.1inChapter13.There,weusedthedatainFERTIL1.RAWtoestimatealinearmodelforkids,thenumberofchildreneverborntoawoman.(i)EstimateaPoissonregressionmodelforkids,usingthesamevariablesinTable13.1.Interpretthecoefficientony82.(ii)Whatistheestimatedpercentagedifferenceinfertilitybetweenablackwomanandanonblackwoman,holdingotherfactorsfixed?(iii)Obtainˆ.Isthereevidenceofover-orunderdispersion?(iv)ComputethefittedvaluesfromthePoissonregressionandtheR-squaredasthesquaredcorrelationbetweenkidsiandkiˆdsi.ComparethiswiththeR-squaredforthelinearregressionmodel.17.13UsethedatainRECID.RAWtoestimatethemodelfromExample17.4byOLS,usingonlythe552uncensoreddurations.CommentgenerallyonhowtheseestimatescomparewiththoseinTable17.4.17.14UsetheMROZ.RAWdataforthisexercise.(i)Usingthe428womenwhowereintheworkforce,estimatethereturn2toeducationbyOLSincludingexper,exper,nwifeinc,age,kidslt6,andkidsge6asexplanatoryvariables.Reportyourestimateoneducanditsstandarderror.(ii)NowestimatethereturntoeducationbyHeckit,whereallexogenousvariablesshowupinthesecond-stageregression.Inotherwords,the2regressionislog(wage)oneduc,exper,exper,nwifeinc,age,kidslt6,kidsge6,andˆ.Comparetheestimatedreturntoeducationanditsstan-darderrortothatfrompart(i).(iii)Usingonlythe428observationsforworkingwomen,regressˆoneduc,2exper,exper,nwifeinc,age,kidslt6,andkidsge6.HowbigistheR-squared?Howdoesthishelpexplainyourfindingsfrompart(ii)?(Hint:Thinkmulticollinearity.)APPENDIX17AAsymptoticStandardErrorsinLimitedDependentVariableModelsDerivationsoftheasymptoticstandarderrorsforthemodelsandmethodsintroducedinthischapterarewellbeyondthescopeofthistext.Notonlydothederivationsrequirematrixalgebra,buttheyalsorequireadvancedasymptotictheoryofnonlinearestima-tion.Thebackgroundneededforacarefulanalysisofthesemethodsandseveralderiva-tionsaregiveninWooldridge(1999).Itisinstructivetoseetheformulasforobtainingtheasymptoticstandarderrorsforatleastsomeofthemethods.GiventhebinaryresponsemodelP(y1x)G(x),whereG()isthelogitorprobitfunction,andisthek1vectorofparameters,theasymptoticvariancematrixofˆisestimatedas569\nd7/14/998:28PMPage570Part3AdvancedTopicsn21[g(xiˆ)]xixiAvaˆr(ˆ),(17.50)i1G(xiˆ)[1G(xiˆ)]whichisakkmatrix.(SeeAppendixDforasummaryofmatrixalgebra.)Withoutthetermsinvolvingg()andG(),thisformulalooksalotliketheestimatedvariance2matrixfortheOLSestimator,minusthetermˆ.Theexpressionin(17.50)accountsforthenonlinearnatureoftheresponseprobability—thatis,thenonlinearnatureofG()—aswellastheparticularformofheteroskedasticityinabinaryresponsemode:Var(yx)G(x)[1G(x)].Thesquarerootsofthediagonalelementsof(17.50)aretheasymptoticstandarderrorsoftheˆj,andtheyareroutinelyreportedbyeconometricssoftwarethatsupportslogitandprobitanalysis.Oncewehavethese,(asymptotic)tstatisticsandconfidenceintervalsareobtainedintheusualways.Thematrixin(17.50)isalsothebasisforWaldtestsofmultiplerestrictionson[seeWooldridge(1999,Chapter15)].TheasymptoticvariancematrixforTobitismorecomplicatedbuthasasimilarstructure.Notethatwecanobtainastandarderrorforˆaswell.Theasymptoticvari-2anceforPoissonregression,allowingfor1in(17.32),hasaformmuchlike(17.50):n12.Avaˆr(ˆ)ˆexp(xiˆ)xixii1Thesquarerootsofthediagonalelementsofthismatrixaretheasymptoticstandard2errors.IfthePoissonassumptionholds,wecandropˆfromtheformula(because21).Asymptoticstandarderrorsforcensoredregression,truncatedregression,andtheHeckitsampleselectioncorrectionaremorecomplicated,althoughtheysharefeatureswiththepreviousformulas.SeeWooldridge(1999)fordetails.570\nd7/14/998:36PMPage571ChapterEighteenAdvancedTimeSeriesTopicsnthischapter,wecoversomemoreadvancedtopicsintimeserieseconometrics.InIChapters10,11,and12,weemphasizedinseveralplacesthatusingtimeseriesdatainregressionanalysisrequiressomecareduetothetrending,persistentnatureofmanyeconomictimeseries.Inadditiontostudyingtopicssuchasinfinitedistributedlagmodelsandforecasting,wealsodiscusssomerecentadvancesinanalyzingtimeseriesprocesseswithunitroots.InSection18.1,wedescribeinfinitedistributedlagmodels,whichallowachangeinanexplanatoryvariabletoaffectallfuturevaluesofthedependentvariable.Conceptually,thesemodelsarestraightforwardextensionsofthefinitedistributedlagmodelsinChapter10;butestimatingthesemodelsposessomeinterestingchallenges.InSection18.2,weshowhowtoformallytestforunitrootsinatimeseriesprocess.RecallfromChapter11thatweexcludedunitrootprocessestoapplytheusualasymp-totictheory.Becausethepresenceofaunitrootimpliesthatashocktodayhasalong-lastingimpact,determiningwhetheraprocesshasaunitrootisofinterestinitsownright.Wecoverthenotionofspuriousregressionbetweentwotimeseriesprocesses,eachofwhichhasaunitroot,inSection18.3.Themainresultisthateveniftwounitrootseriesareindependent,itisquitelikelythattheregressionofoneontheotherwillyieldastatisticallysignificanttstatistic.Thisemphasizesthepotentiallyseriousconse-quencesofusingstandardinferencewhenthedependentandindependentvariablesareintegratedprocesses.TheissueofcointegrationapplieswhentwoseriesareI(1),butalinearcombina-tionofthemisI(0);inthiscase,theregressionofoneontheotherisnotspurious,butinsteadtellsussomethingaboutthelong-runrelationshipbetweenthem.Cointegrationbetweentwoseriesalsoimpliesaparticularkindofmodel,calledanerrorcorrectionmodel,fortheshort-termdynamics.WecoverthesemodelsinSection18.4.InSection18.5,weprovideanoverviewofforecastingandbringtogetherallofthetoolsinthisandpreviouschapterstoshowhowregressionmethodscanbeusedtofore-castfutureoutcomesofatimeseries.Theforecastingliteratureisvast,sowefocusonlyonthemostcommonregression-basedmethods.WealsotouchontherelatedtopicofGrangercausality.571\nd7/14/998:36PMPage572Part3AdvancedTopics18.1INFINITEDISTRIBUTEDLAGMODELSLet{(yt,zt):t…,2,1,0,1,2,…}beabivariatetimeseriesprocess(whichisonlypartiallyobserved).Aninfinitedistributedlag(IDL)modelrelatingyttocurrentandallpastvaluesofzisyt0zt1zt12zt2…ut,(18.1)wherethesumonlaggedzextendsbacktotheindefinitepast.Thismodelisonlyanapproximationtoreality,asnoeconomicprocessstartedinfinitelyfarintothepast.Comparedwithafinitedistributedlagmodel,anIDLmodeldoesnotrequirethatwetruncatethelagataparticularvalue.Inorderformodel(18.1)tomakesense,thelagcoefficients,j,musttendtozeroasj*.Thisisnottosaythat2issmallerinmagnitudethan1;itonlymeansthattheimpactofztjonytmusteventuallybecomesmallasjgetslarge.Inmostapplica-tions,thismakeseconomicsenseaswell:thedistantpastofzshouldbelessimportantforexplainingythantherecentpastofz.Evenifwedecidethat(18.1)isausefulmodel,weclearlycannotestimateitwith-outsomerestrictions.Forone,weonlyobserveafinitehistoryofdata.Equation(18.1)involvesaninfinitenumberofparameters,0,1,2,…,whichcannotbeestimatedwith-outrestrictions.Later,weplacerestrictionsonthejthatallowustoestimate(18.1).Aswithfinitedistributedlagmodels,theimpactpropensityin(18.1)issimply0(seeChapter10).Generally,thehhavethesameinterpretationasinanFDL.Supposethatzs0foralls0andthatz01andzs0foralls1;inotherwords,attimet0,zincreasestemporarilybyoneunitandthenrevertstoitsinitiallevelofzero.Foranyh0,wehaveyhhuhforallh0,andsoE(yh)h,(18.2)whereweusethestandardassumptionthatuhhaszeromean.ItfollowsthathisthechangeinE(yh),givenaone-unit,temporarychangeinzattimezero.WejustsaidthathmustbetendingtozeroashgetslargefortheIDLtomakesense.Thismeansthatatemporarychangeinzhasnolong-runeffectonexpectedy:E(yh)h*ash*.Weassumedthattheprocesszstartsatzs0andthattheone-unitincreaseoccurredatt0.Thesewereonlyforthepurposeofillustration.Moregenerally,ifztemporarilyincreasesbyoneunit(fromanyinitiallevel)attimet,thenhmeasuresthechangeintheexpectedvalueofyafterhperiods.Thelagdistribution,whichishplot-tedasafunctionofh,showstheexpectedpaththatfutureyfollowgiventheone-unit,temporaryincreaseinz.Thelongrunpropensityinmodel(18.1)isthesumofallofthelagcoefficients:LRP0123…,(18.3)whereweassumethattheinfinitesumiswell-defined.Becausethejmustconvergetozero,theLRPcanoftenbewell-approximatedbyafinitesumoftheform0572\nd7/14/998:36PMPage573Chapter18AdvancedTimeSeriesTopics1…pforsufficientlylargep.TointerprettheLRP,supposethattheprocessztissteadyatzs0fors0.Att0,theprocesspermanentlyincreasesbyoneunit.Forexample,ifztisthepercentagechangeinthemoneysupplyandytistheinflationrate,thenweareinterestedintheeffectsofapermanentincreaseofonepercentagepointinmoneysupplygrowth.Then,bysubstitutingzs0fors0andzt1fort0,wehaveyh01…huh,whereh0isanyhorizon.Becauseuthasazeromeanforallt,wehaveE(yh)01…h.(18.4)[Itisusefultocompare(18.4)and(18.2).]Asthehorizonincreases,thatis,ash*,theright-handsideof(18.4)is,bydefinition,thelongrunpropensity.Thus,theLRPmeasuresthelong-runchangeintheex-pectedvalueofygivenaone-unit,perma-QUESTION18.1nentincreaseinz.Supposethatzs0fors0andthatz01,z11,andzs0ThepreviousderivationoftheLRP,andfors1.FindE(y1),E(y0),andE(yh)forh1.Whathappensastheinterpretationofj,usedthefactthath*?theerrorshaveazeromean;asusual,thisisnotmuchofanassumption,providedaninterceptisincludedinthemodel.Acloserexaminationofourreasoningshowsthatweassumedthatthechangeinzduringanytimeperiodhadnoeffectontheexpectedvalueofut.ThisistheinfinitedistributedlagversionofthestrictexogeneityassumptionthatweintroducedinChapter10(inparticular,AssumptionTS.2).Formally,E(ut…,zt2,zt1,zt,zt1,…)0,(18.5)sothattheexpectedvalueofutdoesnotdependonthezinanytimeperiod.While(18.5)isnaturalforsomeapplications,itrulesoutotherimportantpossibilities.Ineffect,(18.5)doesnotallowfeedbackfromyttofuturezbecausezthmustbeuncor-relatedwithutforh0.Intheinflation/moneysupplygrowthexample,whereytisinflationandztismoneysupplygrowth,(18.5)rulesoutfuturechangesinmoneysup-plygrowththataretiedtochangesintoday’sinflationrate.Giventhatmoneysupplypolicyoftenattemptstokeepinterestratesandinflationatcertainlevels,thismightbeunrealistic.Oneapproachtoestimatingthej,whichwecoverinthenextsubsection,requiresastrictexogeneityassumptioninordertoproduceconsistentestimatorsofthej.AweakerassumptionisE(utzt,zt1,…)0.(18.6)Under(18.6),theerrorisuncorrelatedwithcurrentandpastz,butitmaybecorrelatedwithfuturez;thisallowszttobeavariablethatfollowspolicyrulesthatdependonpasty.Sometimes,(18.6)issufficienttoestimatethej;weexplainthisinthenextsubsection.573\nd7/14/998:36PMPage574Part3AdvancedTopicsOnethingtorememberisthatneither(18.5)nor(18.6)saysanythingabouttheser-ialcorrelationpropertiesof{ut}.(Thisisjustasinfinitedistributedlagmodels.)Ifany-thing,wemightexpectthe{ut}tobeseriallycorrelatedbecause(18.1)isnotgenerallydynamicallycompleteinthesensediscussedinSection11.4.Wewillstudytheserialcorrelationproblemlater.HowdoweinterpretthelagcoefficientsandtheLRPif(18.6)holdsbut(18.5)doesnot?Theansweris:thesamewayasbefore.Wecanstilldothepreviousthought(orcounterfactual)experiment,eventhoughthedataweobservearegeneratedbysomefeedbackbetweenytandfuturez.Forexample,wecancertainlyaskaboutthelong-runeffectofapermanentincreaseinmoneysupplygrowthoninflation,eventhoughthedataonmoneysupplygrowthcannotbecharacterizedasstrictlyexogenous.TheGeometric(orKoyck)DistributedLagBecausetherearegenerallyaninfinitenumberofj,wecannotconsistentlyestimatethemwithoutsomerestrictions.Thesimplestversionof(18.1),whichstillmakesthemodeldependonaninfinitenumberoflags,isthegeometric(orKoyck)distributedlag.Inthismodel,thejdependononlytwoparameters:jj,1,j0,1,2,….(18.7)Theparametersandmaybepositiveornegative,butmustbelessthanoneinabsolutevalue.Thisensuresthatj*0asj*0.Infact,thisconvergencehappensatjaveryfastrate.(Forexample,with.5andj10,1/1024.001.)TheimpactpropensityintheGDLissimply0,andsothesignoftheIPisdeterminedbythesignof.If0,say,and0,thenalllagcoefficientsarepos-jitive.If0,thelagcoefficientsalternateinsign(isnegativeforoddj).Thelongrunpropensityismoredifficulttoobtain,butwecanuseastandardresultonthesum2jofageometricseries:for1,1……1/(1),andsoLRP/(1).TheLRPhasthesamesignas.Ifweplug(18.7)into(18.1),westillhaveamodelthatdependsonthezbacktotheindefinitepast.Nevertheless,asimplesubtractionyieldsanestimablemodel.WritetheIDLattimestandt1as:2ytztzt1zt2…ut(18.8)and2yt1zt1zt2zt3…ut1.(18.9)Ifwemultiplythesecondequationbyandsubtractitfromthefirst,allbutafewofthetermscancel:ytyt1(1)ztutut1,574\nd7/14/998:36PMPage575Chapter18AdvancedTimeSeriesTopicswhichwecanwriteasyt0ztyt1utut1,(18.10)where0(1).Thisequationlookslikeastandardmodelwithalaggeddepen-dentvariable,whereztappearscontemporaneously.Becauseisthecoefficientonztandisthecoefficientonyt1,itappearsthatwecanestimatetheseparameters.[If,forsomereason,weareinterestedin,wecanalwaysobtainˆˆ0/(1ˆ)afteresti-matingand0.]Thesimplicityof(18.10)issomewhatmisleading.Theerrorterminthisequation,utut1,isgenerallycorrelatedwithyt1.From(18.9),itisprettyclearthatut1andyt1arecorrelated.Therefore,ifwewrite(18.10)asyt0ztyt1vt,(18.11)wherevtutut1,thenwegenerallyhavecorrelationbetweenvtandyt1.Withoutfurtherassumptions,OLSestimationof(18.11)producesinconsistentestimatesofand.Onecasewherevtmustbecorrelatedwithyt1occurswhenutisindependentofztandallpastvaluesofzandy.Then,(18.8)isdynamicallycomplete,andutisuncorre-latedwithyt1.From(18.9),thecovariancebetweenvtandyt1isVar(ut1)2u,whichiszeroonlyif0.Wecaneasilyseethatvtisseriallycorrelated:2because{ut}isseriallyuncorrelated,E(vtvt1)E(utut1)E(ut1)E(utut2)22E(ut1ut2)u.Forj1,E(vtvtj)0.Thus,{vt}isamovingaverageprocessoforderone(seeSection11.1).Thisgivesanexampleofamodel—whichisderivedfromtheoriginalmodelofinterest—thathasalaggeddependentvariableandapartic-ularkindofserialcorrelation.Ifwemakethestrictexogeneityassumption(18.5),thenztisuncorrelatedwithutandut1,andthereforewithvt.Thus,ifwecanfindasuitableinstrumentalvariableforyt1,thenwecanestimate(18.11)byIV.WhatisagoodIVcandidateforyt1?Byassumption,utandut1arebothuncorrelatedwithzt1,andsovtisuncorrelatedwithzt1.If0,zt1andyt1arecorrelated,evenafterpartiallingoutzt.Therefore,wecanuseinstruments(zt,zt1)toestimate(18.11).Generally,thestandarderrorsneedtobeadjustedforserialcorrelationinthe{vt},aswediscussedinSection15.7.AnalternativetoIVestimationexploitsthefactthat{ut}maycontainaspecifickindofserialcorrelation.Inparticular,inadditionto(18.6),supposethat{ut}followstheAR(1)modelutut1et(18.12)E(etzt,yt1,zt1,…)0.(18.13)Itisimportanttonoticethattheappearingin(18.12)isthesameparametermultiply-ingyt1in(18.11).If(18.12)and(18.13)hold,wecanwriteyt0ztyt1et,(18.14)575\nd7/14/998:36PMPage576Part3AdvancedTopicswhichisadynamicallycompletemodelunder(18.13).FromChapter11,wecanobtainconsistent,asymptoticallynormalestimatorsoftheparametersbyOLS.Thisisveryconvenient,asthereisnoneedtodealwithserialcorrelationintheerrors.Ifetsatisfies2thehomoskedasticityassumptionVar(etzt,yt1)e,theusualinferenceapplies.Oncewehaveestimatedand,wecaneasilyestimatetheLRP:LRˆPˆ/(1ˆ).Thesimplicityofthisprocedurereliesonthepotentiallystrongassumptionthat{ut}followsanAR(1)processwiththesameappearingin(18.7).Thisisusuallynoworsethanassumingthe{ut}areseriallyuncorrelated.Nevertheless,becauseconsistencyoftheestimatorsreliesheavilyonthisassumption,itisagoodideatotestit.Asimpletestbeginsbyspecifying{ut}asanAR(1)processwithadifferentparameter,sayutut1et.McClainandWooldridge(1995)deviseasimpleLagrangemultipliertestofH0:thatcanbecomputedafterOLSestimationof(18.14).Thegeometricdistributedlagmodelextendstomultipleexplanatoryvariables—sothatwehaveaninfiniteDLineachexplanatoryvariable—butthenwemustbeabletojwritethecoefficientonztj,hash.Inotherwords,whilehisdifferentforeachexplanatoryvariable,isthesame.Thus,wecanwriteyt01zt1…kztkyt1vt.(18.15)Thesameissuesthataroseinthecasewithonezariseinthecasewithmanyz.Underthenaturalextensionof(18.12)and(18.13)—justreplaceztwithzt(zt1,…,ztk)—OLSisconsistentandasymptoticallynormal.Or,anIVmethodcanbeused.RationalDistributedLagModelsThegeometricDLimpliesafairlyrestrictivelagdistribution.When0and0,thejarepositiveandmonotonicallydecliningtozero.Itispossibletohavemoregen-eralinfinitedistributedlagmodels.TheGDLisaspecialcaseofwhatisgenerallycalledarationaldistributedlag(RDL)model.Ageneraltreatmentisbeyondourscope—Harvey(1990)isagoodreference—butwecancoveronesimple,usefulexten-sion.SuchanRDLmodelismosteasilydescribedbyaddingalagofztoequation(18.11):yt00ztyt11zt1vt,(18.16)wherevtutut1,asbefore.Byrepeatedsubstitution,itcanbeshownthat(18.16)isequivalenttotheinfinitedistributedlagmodel2yt0(ztzt1zt2…)21(zt1zt2zt3…)ut0zt(01)zt1(01)zt22(01)zt3…ut,whereweagainneedtheassumption1.Fromthislastequation,wecanreadoffthelagdistribution.Inparticular,theimpactpropensityis0,whilethecoefficientonh1zthis(01)forh1.Therefore,thismodelallowstheimpactpropensityto576\nd7/14/998:36PMPage577Chapter18AdvancedTimeSeriesTopicsFigure18.1Lagdistributionfortherationaldistributedlag(18.16)with.5,01,and11.coefficient.50510lag1differinsignfromtheotherlagcoefficients,evenif0.However,if0,thehhavethesamesignas(01)forallh1.ThelagdistributionisplottedinFigure18.1for.5,01,and11.Theeasiestwaytocomputethelongrunpropensityistosetyandzattheirlong-runvaluesforallt,sayy*andz*,andthenfindthechangeiny*withrespecttoz*(seealsoProblem10.3).Wehavey*00z*y*1z*,andsolvinggivesy*0/(1)(01)/(1)z*.Now,weusethefactthatLRPy*/z*:LRP(01)/(1).Because1,theLRPhasthesamesignas01,andtheLRPiszeroifandonlyif010,asinFigure18.1.EXAMPLE18.1(HousingInvestmentandResidentialPriceInflation)WeestimateboththebasicgeometricandtherationaldistributedlagmodelsbyapplyingOLSto(18.14)and(18.16),respectively.Thedependentvariableislog(invpc)afteralineartimetrendhasbeenremoved[thatis,welinearlydetrendlog(invpc)].Forzt,weusethegrowthinthepriceindex.Thisallowsustoestimatehowresidentialpriceinflationaffectsmovementsinhousinginvestmentarounditstrend.Theresultsoftheestimation,usingthedatainHSEINV.RAW,aregiveninTable18.1.577\nd7/14/998:36PMPage578Part3AdvancedTopicsTable18.1DistributedLagModelsforHousingInvestmentDependentVariable:log(invpc),detrendedIndependentGeometricRationalVariablesDLDLgprice3.1083.256(0.933)(0.970)y1.340.547(.132)(.152)gprice1—2.936(0.973)constant.001.578(.018)(.307)LongRunPropensity4.688.706SampleSize4140AdjustedR-Squared.375.504Thegeometricdistributedlagmodelisclearlyrejectedbythedata,asgprice1isverysig-nificant.TheadjustedR-squaredsalsoshowthattheRDLmodelfitsmuchbetter.Thetwomodelsgiveverydifferentestimatesofthelongrunpropensity.Ifweincor-rectlyusetheGDL,theestimatedLRPisalmostfive:apermanentonepercentagepointincreaseinresidentialpriceinflationincreaseslong-termhousinginvestmentby4.7%(aboveitstrendvalue).Economically,thisseemsimplausible.TheLRPestimatedfromtherationaldistributedlagmodelisbelowone.Infact,wecannotrejectthenullhypothesisH0:010atanyreasonablesignificancelevel(p-value.83),sothereisnoevidencethattheLRPisdifferentfromzero.Thisisagoodexampleofhowmisspecifyingthedynam-icsofamodelbyomittingrelevantlagscanleadtoerroneousconclusions.18.2TESTINGFORUNITROOTSWenowturntotheimportantproblemoftestingforunitroots.InChapter11,wegavesomevague,necessarilyinformalguidelinestodecidewhetheraseriesisI(1)ornot.Inmanycases,itisusefultohaveaformaltestforaunitroot.Aswewillsee,suchtestsmustbeappliedwithcaution.578\nd7/14/998:36PMPage579Chapter18AdvancedTimeSeriesTopicsThesimplestapproachtotestingforaunitrootbeginswithanAR(1)model:ytyt1et,t1,2,…,(18.17)wherey0istheobservedinitialvalue.Throughoutthissection,welet{et}denoteaprocessthathaszeromean,givenpastobservedy:E(etyt1,yt2,…,y0)0.(18.18)[Under(18.18),{et}issaidtobeamartingaledifferencesequencewithrespectto{yt1,yt2,…}.If{et}isassumedtobei.i.d.withzeromeanandisindependentofy0,thenitalsosatisfies(18.18).]If{yt}follows(18.17),ithasaunitrootifandonlyif1.If0and1,{yt}followsarandomwalkwithoutdrift[withtheinnovationsetsatisfying(18.18)].If0and1,{yt}isarandomwalkwithdrift,whichmeansthatE(yt)isalinearfunctionoft.Aunitrootprocesswithdriftbehavesverydifferentlyfromonewithoutdrift.Nevertheless,itiscommontoleaveunspecifiedunderthenullhypothesis,andthisistheapproachwetake.Therefore,thenullhypothesisisthat{yt}hasaunitroot:H0:1.(18.19)Inalmostallcases,weareinterestedintheone-sidedalternativeH1:1.(18.20)(Inpractice,thismeans01,as0foraseriesthatwesuspecthasaunitrootwouldbeveryrare.)ThealternativeH1:1isnotusuallyconsidered,sinceitimpliesthatytisexplosive.Infact,if0,ythasanexponentialtrendinitsmeanwhen1.When1,{yt}isastableAR(1)process,whichmeansitisweaklydependenthorasymptoticallyuncorrelated.RecallfromChapter11thatCorr(yt,yth)*0when1.Therefore,testing(18.19)inmodel(18.17),withthealternativegivenby(18.20),isreallyatestofwhether{yt}isI(1)againstthealternativethat{yt}isI(0).[ThereasonwedonottakethenulltobeI(0)inthissetupisthat{yt}isI(0)foranyvalueofstrictlybetween1and1,somethingthatclassicalhypothesistestingdoesnothandleeasily.TherearetestswherethenullhypothesisisI(0)againstthealterna-tiveofI(1),butthesetakeadifferentapproach.See,forexample,Kwiatkowski,Phillips,Schmidt,andShin(1992).]Aconvenientequationforcarryingouttheunitroottestistosubtractyt1frombothsidesof(18.17)andtodefine1:ytyt1et.(18.21)Under(18.18),thisisadynamicallycompletemodel,andsoitseemsstraightforwardtotestH0:0againstH1:0.Theproblemisthat,underH0,yt1isI(1),andsotheusualcentrallimittheoremthatunderliestheasymptoticstandardnormaldistribu-579\nd7/14/998:36PMPage580Part3AdvancedTopicstionforthetstatisticdoesnotapply:thetstatisticdoesnothaveanapproximatestan-dardnormaldistributioneveninlargesamplesizes.TheasymptoticdistributionofthetstatisticunderH0hascometobeknownastheDickey-FullerdistributionafterDickeyandFuller(1979).Whilewecannotusetheusualcriticalvalues,wecanusetheusualtstatisticforˆin(18.21),atleastoncetheappropriatecriticalvalueshavebeentabulated.Theresult-ingtestisknownastheDickey-Fuller(DF)testforaunitroot.Thetheoryusedtoobtaintheasymptoticcriticalvaluesisrathercomplicatedandiscoveredinadvancedtextsontimeserieseconometrics.[See,forexample,Banerjee,Dolado,Galbraith,andHendry(1993),orBDGHforshort.]Bycontrast,usingtheseresultsisveryeasy.Thecriticalvaluesforthetstatistichavebeentabulatedbyseveralauthors,beginningwiththeoriginalworkbyDickeyandFuller(1979).Table18.2containsthelargesamplecriticalvaluesforvarioussignificancelevels,takenfromBDGH(1993,Table4.2).(CriticalvaluesadjustedforsmallsamplesizesareavailableinBDGH.)Table18.2AsymptoticCriticalValuesforUnitRoottTest:NoTimeTrendSignificanceLevel1%2.5%5%10%CriticalValue3.433.122.862.57WerejectthenullhypothesisH0:0againstH1:0iftˆc,wherecisoneofthenegativevaluesinTable18.2.Forexample,tocarryoutthetestatthe5%signifi-cancelevel,werejectiftˆ2.86.Thisrequiresatstatisticwithamuchlargermag-nitudethanifweusedthestandardnormalcriticalvalue,whichwouldbe1.65.Ifweusethestandardnormalcriticalvaluetotestforaunitroot,wewouldrejectH0muchmoreoftenthan5%ofthetimewhenH0istrue.EXAMPLE18.2(UnitRootTestforThree-MonthT-BillRates)WeusethequarterlydatainINTQRT.RAWtotestforaunitrootinthree-monthT-billrates.Whenweestimate(18.20),weobtainrˆ3t(.625)(.091)r3t1rˆ3t(.261)(.037)r3t1(18.22)2n123,R.048,wherewekeepwithourconventionofreportingstandarderrorsinparenthesesbelowtheestimates.Wemustrememberthatthesestandarderrorscannotbeusedtoconstructusualconfidenceintervalsortocarryouttraditionalttestsbecausethesedonotbehaveinthe580\nd7/14/998:36PMPage581Chapter18AdvancedTimeSeriesTopicsusualwayswhenthereisaunitroot.Thecoefficientonr3t1showsthattheestimateofisˆ1ˆ.909.Whilethisislessthanunity,wedonotknowwhetheritisstatisticallylessthanone.Thetstatisticonr3t1is.091/.0372.46.FromTable18.2,the10%criticalvalueis2.57;therefore,wefailtorejectH0:1againstH1:1atthe10%level.Aswithotherhypothesestests,whenwefailtorejectH0,wedonotsaythatweacceptH0.Why?SupposewetestH0:.9inthepreviousexampleusingastandardttest—whichisasymptoticallyvalid,becauseytisI(0)underH0.Then,weobtaint.001/.037,whichisverysmallandprovidesnoevidenceagainst.9.Yet,itmakesnosensetoaccept1and.9.Whenwefailtorejectaunitroot,asinthepreviousexample,weshouldonlycon-cludethatthedatadonotprovidestrongevidenceagainstH0.Inthisexample,thetestdoesprovidessomeevidenceagainstH0becausethetstatisticisclosetothe10%crit-icalvalue.(Ideally,wewouldcomputeap-value,butthisrequiresspecialsoftwarebecauseofthenonnormaldistribution.)Inaddition,whileˆ.91impliesafairamountofpersistencein{r3t},thecorrelationbetweenobservationswhichare10periodsapartforanAR(1)modelwith.9isabout.35,ratherthanalmostoneif1.Whathappensifwenowwanttouser3tasanexplanatoryvariableinaregressionanalysis?Theoutcomeoftheunitroottestimpliesweshouldbeextremelycautious:ifr3tdoeshaveaunitroot,theusualasymptoticapproximationsneednothold(aswedis-cussedinChapter11).Onesolutionistousethefirstdifferenceofr3tinanyanalysis.AswewillseeinSection18.4,thatisnottheonlypossibility.Wealsoneedtotestforunitrootsinmodelswithmorecomplicateddynamics.If{yt}follows(18.17)with1,thenytisseriallyuncorrelated.Wecaneasilyallow{yt}tofollowanARmodelmodelbyaugmentingequation(18.21)withadditionallags.Forexample,ytyt11yt1et,(18.23)where11.Thisensuresthat,underH0:0,{yt}followsastableAR(1)model.UnderthealternativeH1:0,itcanbeshownthat{yt}followsastableAR(2)model.Moregenerally,wecanaddplagsofyttotheequationtoaccountforthedynam-icsintheprocess.Thewaywetestthenullhypothesisofaunitrootisverysimilar:weruntheregressionofytonyt1,yt1,…,ytp(18.24)andcarryoutthettestonˆ,thecoefficientonyt1,justasbefore.Thisextendedver-sionoftheDickey-FullertestisusuallycalledtheaugmentedDickey-Fullertestbecausetheregressionhasbeenaugmentedwiththelaggedchanges,yth.Thecriti-calvaluesandrejectionrulearethesameasbefore.Theinclusionofthelaggedchangesin(18.24)isintendedtocleanupanyserialcorrelationinyt.Themorelagsweincludein(18.24),themoreinitialobservationswelose.Ifweincludetoomanylags,thesmall581\nd7/14/998:36PMPage582Part3AdvancedTopicssamplepowerofthetestgenerallysuffers.Butifweincludetoofewlags,thesizeofthetestwillbeincorrect,evenasymptotically,becausethevalidityofthecriticalvaluesinTable18.2reliesonthedynamicsbeingcompletelymodeled.Oftenthelaglengthisdictatedbythefrequencyofthedata(aswellasthesamplesize).Forannualdata,oneortwolagsusuallysuffice.Formonthlydata,wemightincludetwelvelags.Buttherearenohardrulestofollowinanycase.Interestingly,thetstatisticsonthelaggedchangeshaveapproximatetdistributions.TheFstatisticsforjointsignificanceofanygroupoftermsytharealsoasymptoti-callyvalid.(ThesemaintainthehomoskedasticityassumptiondiscussedinSection11.5.)Therefore,wecanusestandardteststodeterminewhetherwehaveenoughlaggedchangesin(18.24).EXAMPLE18.3(UnitRootTestforAnnualU.S.Inflation)WeuseannualdataonU.S.inflation,basedontheCPI,totestforaunitrootininflation(seePHILLIPS.RAW).Theseriesspanstheyearsfrom1948through1996.AllowingforonelagofinftintheaugmentedDickey-Fullerregressiongivesinˆft(1.36)0(.310)inft1(.138)inft1inˆft0(.261)(.103)inft1(.126)inft12n47,R.172.Thetstatisticfortheunitroottestis.310/.1033.01.Becausethe5%criticalvalueis2.86,werejecttheunitroothypothesisatthe5%level.Theestimateofisabout.690.Together,thisisreasonablystrongevidenceagainstaunitrootininflation.Thelaginft1hasatstatisticofabout1.10,sowedonotneedtoincludeit,butwecouldnotknowthisaheadoftime.Ifwedropinft1,theevidenceagainstaunitrootisslightlystronger:ˆ.335(ˆ.665),andtˆ3.13.Forseriesthathavecleartimetrends,weneedtomodifythetestforunitroots.Atrend-stationaryprocess—whichhasalineartrendinitsmeanbutisI(0)aboutitstrend—canbemistakenforaunitrootprocessifwedonotcontrolforatimetrendintheDickey-Fullerregression.Inotherwords,ifwecarryouttheusualDForaugmentedDFtestonatrendingbutI(0)series,wewillprobablyhavelittlepowerforrejectingaunitroot.Toallowforserieswithtimetrends,wechangethebasicequationtoyttyt1et,(18.25)whereagainthenullhypothesisisH0:0,andthealternativeisH1:0.Underthealternative,{yt}isatrend-stationaryprocess.Ifythasaunitroot,thenyttet,andsothechangeinythasameanlinearintunless0.[ItcanbeshownthatE(yt)isactuallyaquadraticint.]Itisunusualforthefirstdifferenceofaneco-nomicseriestohavealineartrend,andsoamoreappropriatenullhypothesisisprob-582\nd7/14/998:36PMPage583Chapter18AdvancedTimeSeriesTopicsablyH0:0,0.WhileitispossibletotestthisjointhypothesisusinganFtest—butwithmodifiedcriticalvalues—itiscommontoonlytestH0:0usingattest.Wefollowthatapproachhere.[SeeBDGH(1993,Section4.4)formoredetailsonthejointtest.]Whenweincludeatimetrendintheregression,thecriticalvaluesofthetestchange.Intuitively,thisisbecausedetrendingaunitrootprocesstendstomakeitlookmorelikeanI(0)process.Therefore,werequirealargermagnitudeforthetstatisticinordertorejectH0.TheDickey-FullercriticalvaluesforthettestthatincludesatimetrendaregiveninTable18.3;theyaretakenfromBDGH(1993,Table4.2).Table18.3AsymptoticCriticalValuesforUnitRoottTest:LinearTimeTrendSignificanceLevel1%2.5%5%10%CriticalValue3.963.663.413.12Forexample,torejectaunitrootatthe5%level,weneedthetstatisticonˆtobelessthan3.41,ascomparedwith2.86withoutatimetrend.Wecanaugmentequation(18.25)withlagsofyttoaccountforserialcorrelation,justasinthecasewithoutatrend.Thisdoesnotchangehowwecarryoutthetest.EXAMPLE18.4(UnitRootintheLogofU.S.RealGrossDomesticProduct)WecanapplytheunitroottestwithatimetrendtotheU.S.GDPdatainINVEN.RAW.Theseannualdatacovertheyearsfrom1959through1995.Wetestwhetherlog(GDPt)hasaunitroot.Thisserieshasapronouncedtrendthatlooksroughlylinear.Weincludeasinglelagoflog(GDPt),whichissimplythegrowthinGDP(indecimalform),toaccountfordynamics:gGDˆPt(1.65)(.0059)t(.210)log(GDPt1)(.264)gDGPt1gGDˆPt0(.67)(.0027)t(.087)log(GDPt1)(.165)gDGPt1(18.26)2n35,R.268.Fromthisequation,wegetˆ1.21.79,whichisclearlylessthanone.Butwecan-notrejectaunitrootinthelogofGDP:thetstatisticonlog(GDPt1)is.210/.0872.41,whichiswell-abovethe10%criticalvalueof3.12.ThetstatisticongGDPt1is1.60,whichisalmostsignificantatthe10%levelagainstatwo-sidedalternative.Whatshouldweconcludeaboutaunitroot?Again,wecannotrejectaunitroot,butthepointestimateofisnotespeciallyclosetoone.Whenwehaveasmallsamplesize—andn35isconsideredtobeprettysmall—itisverydifficulttorejectthenullhypothesisofaunitrootiftheprocesshassomethingclosetoaunitroot.Usingmoredataoverlongertimeperiods,manyresearchershaveconcludedthatthereislittleevidenceagainsttheunit583\nd7/14/998:36PMPage584Part3AdvancedTopicsroothypothesisforlog(GDP).ThishasledmostofthemtoassumethatthegrowthinGDPisI(0),whichmeansthatlog(GDP)isI(1).Unfortunately,givencurrentlyavailablesamplesizes,wecannothavemuchconfidenceinthisconclusion.Ifweomitthetimetrend,thereismuchlessevidenceagainstH0,asˆ.023andtˆ1.92.Here,theestimateofismuchclosertoone,butthiscanbemisleadingduetotheomittedtimetrend.Itistemptingtocomparethetstatisticonthetimetrendin(18.26),withthecriti-calvaluefromastandardnormalortdistribution,toseewhetherthetimetrendissig-nificant.Unfortunately,thetstatisticonthetrenddoesnothaveanasymptoticstandardnormaldistribution(unless1).Theasymptoticdistributionofthiststatisticisknown,butitisrarelyused.Typically,werelyonintuition(orplotsofthetimeseries)todecidewhethertoincludeatrendintheDFtest.Therearemanyothervariantsonunitroottests.Inoneversionthatisonlyapplic-abletoseriesthatareclearlynottrending,theinterceptisomittedfromtheregression;thatis,issettozeroin(18.21).ThisvariantoftheDickey-Fullertestisrarelyusedbecauseofbiasesinducedif0.Also,wecanallowformorecomplicatedtimetrends,suchasquadratic.Again,thisisseldomused.Anotherclassoftestsattemptstoaccountforserialcorrelationinytinadifferentmannerthanbyincludinglagsin(18.21)or(18.25).Theapproachisrelatedtotheser-ialcorrelation-robuststandarderrorsfortheOLSestimatorsthatwediscussedinSection12.5.Theideaistobeasagnosticaspossibleaboutserialcorrelationinyt.Inpractice,the(augmented)Dickey-Fullertesthasheldupprettywell.[SeeBDGH(1993,Section4.3)foradiscussiononothertests.]18.3SPURIOUSREGRESSIONInacross-sectionalenvironment,weusethephrase“spuriouscorrelation”todescribeasituationwheretwovariablesarerelatedthroughtheircorrelationwithathirdvari-able.Inparticular,ifweregressyonx,wefindasignificantrelationship.Butwhenwecontrolforanothervariable,sayz,thepartialeffectofxonybecomeszero.Naturally,thiscanalsohappenintimeseriescontextswithI(0)variables.AswediscussedinSection10.5,itispossibletofindaspuriousrelationshipbetweentimeseriesthathaveincreasingordecreasingtrends.Providedtheseriesareweaklydependentabouttheirtimetrends,theproblemiseffectivelysolvedbyinclud-ingatimetrendintheregressionmodel.Whenwearedealingwithprocessesthatareintegratedoforderone,thereisanadditionalcomplication.Evenifthetwoserieshavemeansthatarenottrending,asim-pleregressioninvolvingtwoindependentI(1)serieswilloftenresultinasignificanttstatistic.Tobemoreprecise,let{xt}and{yt}berandomwalksgeneratedbyxtxt1at(18.27)584\nd7/14/998:36PMPage585Chapter18AdvancedTimeSeriesTopicsandytyt1et,t1,2,…,(18.28)where{at}and{et}areindependent,identicallydistributedinnovations,withmeanzero22andvariancesaande,respectively.Forconcreteness,taketheinitialvaluestobex0y00.Assumefurtherthat{at}and{et}areindependentprocesses.Thisimpliesthat{xt}and{yt}arealsoindependent.Butwhatifwerunthesimpleregressionyˆtˆ0ˆ1xt(18.29)andobtaintheusualtstatisticforˆ1andtheusualR-squared?Becauseytandxtareindependent,wewouldhopethatplimˆ10.Evenmoreimportantly,ifwetestH0:10againstH1:10atthe5%level,wehopethatthetstatisticforˆ1isinsignif-icant95%ofthetime.Throughasimulation,GrangerandNewbold(1974)showedthatthisisnotthecase:eventhoughytandxtareindependent,theregressionofytonxtyieldsastatisticallysignificanttstatisticalargepercentageofthetime,muchlargerthanthenominalsignificancelevel.GrangerandNewboldcalledthisthespuriousregressionproblem:thereisnosenseinwhichyandxarerelated,butanOLSregres-sionusingtheusualtstatisticswilloftenindicatearelationship.RecentsimulationresultsaregivenbyDavidsonandMacKinnon(1993,Table19.1),whereatandetaregeneratedasindependent,identicallydistributednormalQUESTION18.2randomvariables,and10,000differentsamplesaregenerated.ForasamplesizeUndertheprecedingsetup,where{xt}and{yt}aregeneratedby(18.27)and(18.28)and{et}and{at}arei.i.d.sequences,whatistheofn50atthe5%significancelevel,theplimoftheslopecoefficient,sayˆ1,fromtheregressionofytonstandardtstatisticforH0:10againstxt?Describethebehaviorofthetstatisticofˆ1.thetwo-sidedalternativerejectsH0about66.2%ofthetimeunderH0,ratherthan5%ofthetime.Asthesamplesizeincreases,thingsgetworse:withn250,thenullisrejected84.7%ofthetime!Hereisonewaytoseewhatishappeningwhenweregressthelevelofyonthelevelofx.Writethemodelunderlying(18.27)asyt01xtut.(18.30)Forthetstatisticofˆ1tohaveanapproximatestandardnormaldistributioninlargesamples,ataminimum,{ut}shouldbeameanzero,seriallyuncorrelatedprocess.ButunderH0:10,yt0ut,andbecause{yt}isarandomwalkstartingaty00,tequation(18.30)holdsunderH0onlyif00and,moreimportantly,ifutytej.j1Inotherwords,{ut}isarandomwalkunderH0.Thisclearlyviolateseventheasymp-toticversionoftheGauss-MarkovassumptionsfromChapter11.Includingatimetrenddoesnotreallychangetheconclusion.Ifytorxtisarandomwalkwithdriftandatimetrendisnotincluded,thespuriousregressionproblemiseven585\nd7/14/998:36PMPage586Part3AdvancedTopicsworse.Thesamequalitativeconclusionsholdif{at}and{et}aregeneralI(0)processes,ratherthani.i.d.sequences.Inadditiontotheusualtstatisticnothavingalimitingstandardnormaldistribu-tion—infact,itincreasestoinfinityasn*—thebehaviorofR-squaredisnonstan-dard.Incross-sectionalcontextsorinregressionswithI(0)timeseriesvariables,the22R-squaredconvergesinprobabilitytothepopulationR-squared:1u/y.ThisisnotthecaseinspuriousregressionswithI(1)processes.RatherthantheR-squaredhavingawell-definedplim,itactuallyconvergestoarandomvariable.Formalizingthisnotioniswell-beyondthescopeofthiscourse.[AdiscussionoftheasymptoticpropertiesofthetstatisticandtheR-squaredcanbefoundinBDGH(Section3.1).]TheimplicationisthattheR-squaredislargewithhighprobability,eventhough{yt}and{xt}areinde-pendenttimeseriesprocesses.Thesameconsiderationsarisewithmultipleindependentvariables,eachofwhichmaybeI(1)orsomeofwhichmaybeI(0).If{yt}isI(1)andatleastsomeoftheexplanatoryvariablesareI(1),theregressionresultsmaybespurious.ThepossibilityofspuriousregressionwithI(1)variablesisquiteimportantandhasledeconomiststoreexaminemanyaggregatetimeseriesregressionswhosetstatisticswereverysignificantandwhoseR-squaredswereextremelyhigh.Inthenextsection,weshowthatregressinganI(1)dependentvariableonanI(1)independentvariablecanbeinformative,butonlyifthesevariablesarerelatedinaprecisesense.18.4COINTEGRATIONANDERRORCORRECTIONMODELSThediscussionofspuriousregressionintheprevioussectioncertainlymakesonewaryofusingthelevelsofI(1)variablesinregressionanalysis.Inearlierchapters,wesug-gestedthatI(1)variablesshouldbedifferencedbeforetheyareusedinlinearregressionmodels,whethertheyareestimatedbyOLSorinstrumentalvariables.Thisiscertainlyasafecoursetofollow,anditistheapproachusedinmanytimeseriesregressionsafterGrangerandNewbold’soriginalpaperonthespuriousregression.Unfortunately,alwaysdifferencingI(1)variableslimitsthescopeofthequestionsthatwecananswer.CointegrationThenotionofcointegration,whichwasgivenaformaltreatmentinEngleandGranger(1987),makesregressionsinvolvingI(1)variablespotentiallymeaningful.Afulltreat-mentofcointegrationismathematicallyinvolved,butwecandescribethebasicissuesandmethodsthatareusedinmanyapplications.If{yt:t0,1,…}and{xt:t0,1,…}aretwoI(1)processes,then,ingeneral,ytxtisanI(1)processforanynumber.Nevertheless,itispossiblethatforsome0,ytxtisanI(0)process,whichmeansithasconstantmean,constantvariance,QUESTION18.3autocorrelationsthatdependonlyonthetimedistancebetweenanytwovariablesinLet{(yt,xt):t1,2,…}beabivariatetimeserieswhereeachseriesisI(1)withoutdrift.Explainwhy,ifytandxtarecointegrated,ytandtheseries,anditisasymptoticallyuncorre-xt1arealsocointegrated.lated.Ifsuchaexists,wesaythatyand586\nd7/14/998:36PMPage587Chapter18AdvancedTimeSeriesTopicsxarecointegrated,andwecallthecointegrationparameter.[Alternatively,wecouldlookatxtytfor0:ifytxtisI(0),thenxt(1/)ytisI(0).Therefore,thelinearcombinationofytandxtisnotunique,butifwefixthecoefficientonytatunity,thenisunique.SeeProblem18.3.Forconcreteness,weconsiderlinearcombinationsoftheformytxt.]Forthesakeofillustration,take1,supposethaty0x00,andwriteytyt1rt,xtxt1vt,where{rt}and{vt}aretwoI(0)processeswithzeromeans.Then,ytandxthaveatendencytowanderaroundandnotreturntotheinitialvalueofzerowithanyregularity.Bycontrast,ifytxtisI(0),ithaszeromeananddoesreturntozerowithsomeregularity.Asaspecificexample,letr6tbetheannualizedinterestrateforsix-month,T-bills(attheendofquartert)andletr3tbetheannualizedinterestrateforthree-month,T-bills.(Thesearetypicallycalledbondequivalentyields,andtheyarereportedinthefinancialpages.)InExample18.2,usingthedatainINTQRT.RAW,wefoundlittleevi-denceagainstthehypothesisthatr3thasaunitroot;thesameistrueofr6t.Definethespreadbetweensix-andthree-month,T-billratesassprtr6tr3t.Then,usingequa-tion(18.21),theDickey-Fullertstatisticforsprtis7.71(withˆ.67orˆ.33).Therefore,westronglyrejectaunitrootforsprtinfavorofI(0).Theupshotofthisisthatwhiler6tandr3teachappeartobeunitrootprocesses,thedifferencebetweenthemisanI(0)process.Inotherwords,r6andr3arecointegrated.Cointegrationinthisexample,asinmanyexamples,hasaneconomicinterpretation.Ifr6andr3werenotcointegrated,thedifferencebetweeninterestratescouldbecomeverylarge,withnotendencyforthemtocomebacktogether.Basedonasimplearbi-trageargument,thisseemsunlikely.Supposethatthespreadsprtcontinuestogrowforseveraltimeperiods,makingsix-monthT-billsamuchmoredesirableinvestment.Then,investorswouldshiftawayfromthree-monthandtowardsix-monthT-bills,dri-vingupthepriceofsix-monthT-bills,whileloweringthepriceofthree-monthT-bills.Sinceinterestratesareinverselyrelatedtoprice,thiswouldlowerr6andincreaser3,untilthespreadisreduced.Therefore,largedeviationsbetweenr6andr3arenotexpectedtocontinue:thespreadhasatendencytoreturntoitsmeanvalue.(Thespreadactuallyhasaslightlypositivemeanbecauselong-terminvestorsaremorerewardedrel-ativetoshort-terminvestors.)Thereisanotherwaytocharacterizethefactthatsprtwillnotdeviateforlongperi-odsfromitsaveragevalue:r6andr3havealong-runrelationship.Todescribewhatwemeanbythis,letE(sprt)denotetheexpectedvalueofthespread.Then,wecanwriter6tr3tet,where{et}isazeromean,I(0)process.Theequilibriumorlong-runrelationshipoccurswhenet0,orr6*r3*.Atanytimeperiod,therecanbedeviationsfromequi-librium,buttheywillbetemporary:thereareeconomicforcesthatdriver6andr3backtowardtheequilibriumrelationship.Intheinterestrateexample,weusedeconomicreasoningtotellusthevalueofifytandxtarecointegrated.Ifwehaveahypothesizedvalueof,thentestingwhethertwoseriesarecointegratediseasy:wesimplydefineanewvariable,stytxt,andapplyeithertheusualDForaugmentedDFtestto{st}.Ifwerejectaunitrootin{st}587\nd7/14/998:36PMPage588Part3AdvancedTopicsinfavoroftheI(0)alternative,thenwefindthatytandxtarecointegrated.Inotherwords,thenullhypothesisisthatytandxtarenotcointegrated.Testingforcointegrationismoredifficultwhenthe(potential)cointegrationparam-eterisunknown.Ratherthantestforaunitrootin{st},wemustfirstestimate.Ifytandxtarecointegrated,itturnsoutthattheOLSestimatorˆfromtheregressionytˆˆxt(18.31)isconsistentfor.Theproblemisthatthenullhypothesisstatesthatthetwoseriesarenotcointegrated,whichmeansthat,underH0,wearerunningaspuriousregression.Fortunately,itispossibletotabulatecriticalvaluesevenwhenisestimated,whereweapplytheDickey-FulleroraugmentedDickey-Fullertesttotheresiduals,sayuˆtytˆˆxt,from(18.31).Theonlydifferenceisthatthecriticalvaluesaccountforesti-mationof.TheasymptoticcriticalvaluesaregiveninTable18.4.ThesearetakenfromDavidsonandMacKinnon(1993,Table20.2).Table18.4AsymptoticCriticalValuesforCointegrationTest:NoTimeTrendSignificanceLevel1%2.5%5%10%CriticalValue3.903.593.343.04Inthebasictest,weruntheregressionofuˆtonuˆt1andcomparethetstatisticonuˆt1tothedesiredcriticalvalueinTable18.4.Ifthetstatisticisbelowthecriticalvalue,wehaveevidencethatytxtisI(0)forsome;thatis,ytandxtarecointegrated.Wecanaddlagsofuˆttoaccountforserialcorrelation.IfwecomparethecriticalvaluesinTable18.4withthoseinTable18.2,wemustgetatstatisticmuchlargerinmagnitudetofindcointegrationthanifweusedtheusualDFcriticalvalues.ThisisbecauseOLS,whichminimizesthesumofsquaredresiduals,tendstoproduceresidualsthatlooklikeanI(0)sequenceevenifytandxtarenotcointegrated.Ifytandxtarenotcointegrated,aregressionofytonxtisspuriousandtellsusnoth-ingmeaningful:thereisnolong-runrelationshipbetweenyandx.Wecanstillrunaregressioninvolvingthefirstdifferences,ytandxt,includinglags.Butweshouldinterprettheseregressionsforwhattheyare:theyexplainthedifferenceinyintermsofthedifferenceinxandhavenothingnecessarilytodowitharelationshipinlevels.Ifytandxtarecointegrated,wecanusethistospecifymoregeneraldynamicmod-els,aswewillseeinthenextsubsection.Thepreviousdiscussionassumesthatneitherytnorxthasadrift.Thisisreasonableforinterestratesbutnotforothertimeseries.Ifytandxtcontaindriftterms,E(yt)andE(xt)arelinear(usuallyincreasing)functionsoftime.Thestrictdefinitionofcointe-grationrequiresytxttobeI(0)withoutatrend.Toseewhatthisentails,writeyttgtandxttht,where{gt}and{ht}areI(1)processes,isthedriftinyt588\nd7/14/998:36PMPage589Chapter18AdvancedTimeSeriesTopics[E(yt)],andisthedriftinxt[E(xt)].Now,ifytandxtarecointegrated,theremustexistsuchthatgthtisI(0).Butthenytxt()t(gtht),whichisgenerallyatrend-stationaryprocess.Thestrictformofcointegrationrequiresthattherenotbeatrend,whichmeans.ForI(1)processeswithdrift,itispos-siblethatthestochasticparts—thatis,gtandht—arecointegrated,butthattheparame-terwhichcausesgthttobeI(0)doesnoteliminatethelineartimetrend.Wecantestforcointegrationbetweengtandht,withouttakingastandonthetrendpart,byrunningtheregressionyˆtˆˆtˆxt(18.32)andapplyingtheusualDForaugmentedDFtesttotheresidualsuˆt.Theasymptoticcrit-icalvaluesaregiveninTable18.5[fromDavidsonandMacKinnon(1993,Table20.2)].Table18.5AsymptoticCriticalValuesforCointegrationTest:LinearTimeTrendSignificanceLevel1%2.5%5%10%CriticalValue4.324.033.783.50Afindingofcointegrationinthiscaseleavesopenthepossibilitythatytxthasalin-eartrend.ButatleastitisnotI(1).EXAMPLE18.5(CointegrationBetweenFertilityandPersonalExemption)InChapters10and11,westudiedvariousmodelstoestimatetherelationshipbetweenthegeneralfertilityrate(gfr)andtherealvalueofthepersonaltaxexemption(pe)intheUnitedStates.Thestaticregressionresultsinlevelsandfirstdifferencesarenotablydifferent.Theregressioninlevels,withatimetrendincluded,givesanOLScoefficientonpeequalto.1872(se.035)andR.500.Infirstdifferences(withoutatrend),thecoefficientonpeis2.043(se.028),andR.032.Whilethereareotherreasonsforthesedifferences—suchasmisspecifieddistributedlagdynamics—thediscrepancybetweenthelevelsandchangesregressionssuggeststhatweshouldtestforcointegration.Ofcourse,thispre-sumesthatgfrandpeareI(1)processes.Thisappearstobethecase:theaugmentedDFtests,withasinglelaggedchangeandalineartimetrend,eachyieldtstatisticsofabout1.47,andtheestimatedrhosareclosetoone.Whenweobtaintheresidualsfromtheregressionofgfrontandpeandapplytheaug-mentedDFtestwithonelag,weobtainatstatisticonuˆt1of2.43,whichisnowherenearthe10%criticalvalue,3.50.Therefore,wemustconcludethatthereislittleevidenceofcointegrationbetweengfrandpe,evenallowingforseparatetrends.Itisverylikelythattheearlierregressionresultsweobtainedinlevelssufferfromthespuriousregressionproblem.589\nd7/14/998:36PMPage590Part3AdvancedTopicsThegoodnewsisthat,whenweusedfirstdifferencesandallowedfortwolags—seeequation(11.27)—wefoundanoverallpositiveandsignificantlong-runeffectofpeongfr.Ifwethinktwoseriesarecointegrated,weoftenwanttotesthypothesesaboutthecointegratingparameter.Forexample,atheorymaystatethatthecointegratingpara-meterisone.Ideally,wecoulduseatstatistictotestthishypothesis.Weexplicitlycoverthecasewithouttimetrends,althoughtheextensiontothelin-eartrendcaseisimmediate.WhenytandxtareI(1)andcointegrated,wecanwriteytxtut,(18.33)whereutisazeromean,I(0)process.Generally,{ut}containsserialcorrelation,butweknowfromChapter11thatthisdoesnotaffectconsistencyofOLS.Asmentionedear-lier,OLSappliedto(18.33)consistentlyestimates(and).Unfortunately,becausextisI(1),theusualinferenceproceduresdonotnecessarilyapply:OLSisnotasymptoti-callynormallydistributed,andthetstatisticforˆdoesnotnecessarilyhaveanapprox-imatetdistribution.WedoknowfromChapter10that,if{xt}isstrictlyexogenous—seeAssumptionTS.2—andtheerrorsarehomoskedastic,seriallyuncor-related,andnormallydistributedtheOLSestimatorisalsonormallydistributed(con-ditionalontheexplanatoryvariables),andthetstatistichasanexacttdistribution.Unfortunately,theseassumptionsaretoostrongtoapplytomostsituations.Thenotionofcointegrationimpliesnothingabouttherelationshipbetween{xt}and{ut}and,exceptforrequiringthatutisI(0),doesnotrestricttheserialdependenceinut.Fortunately,thefeatureof(18.33)thatmakesinferencethemostdifficult—thelackofstrictexogeneityof{xt}—canbefixed.BecausextisI(1),thepropernotionofstrictexogeneityisthatutisuncorrelatedwithxs,foralltands.Wecanalwaysarrangethisforanewsetoferrors,atleastapproximately,bywritingutasafunctionofthexsforallsclosetot.Forexample,ut0xt1xt12xt2(18.34)1xt12xt2et,where,byconstruction,etisuncorrelatedwitheachxsappearingintheequation.Thehopeisthatetisuncorrelatedwithfurtherlagsandleadsofxs.Weknowthat,asstgetslarge,thecorrelationbetweenetandxsapproacheszero,becausetheseareI(0)processes.Now,ifweplug(18.34)into(18.33),weobtainyt0xt0xt1xt12xt2(18.35)1xt12xt2et.Thisequationlooksabitstrangebecausefuturexsappearwithbothcurrentandlaggedxt.Thekeyisthatthecoefficientonxtisstill,and,byconstruction,xtisnowstrictlyexogenousinthisequation.Thestrictexogeneityassumptionistheimportantconditionneededtoobtainanapproximatelynormaltstatisticforˆ.590\nd7/14/998:36PMPage591Chapter18AdvancedTimeSeriesTopicsTheOLSestimatoroffrom(18.35)iscalledtheleadsandlagsestimatorofbecauseofthewayitemploysx.[See,forexample,StockandWatson(1991).]Theonlyissuewemustworryaboutin(18.35)isthepossibilityofserialcorrelationin{et}.Thiscanbedealtwithbycomputingaserialcorrelation-robuststandarderrorforˆ(asdescribedinSection12.5)orbyusingastandardAR(1)correction(suchasCochrane-Orcutt).EXAMPLE18.6(CointegratingParameterforInterestRates)Earlier,wetestedforcointegrationbetweenr6andr3—six-andthree-month,T-billrates—byassumingthatthecointegratingparameterwasequaltoone.Thisledustofindcointe-grationand,naturally,toconcludethatthecointegratingparameterisequaltounity.Nevertheless,letusestimatethecointegratingparameterdirectlyandtestH0:1.Weapplytheleadsandlagsestimatorwithtwoleadsandtwolagsofr3,aswellasthecon-temporaneouschange.Theestimateofisˆ1.038,andtheusualOLSstandarderroris.0081.Therefore,thetstatisticforH0:1is(1.0381)/.00814.69,whichisastrongstatisticalrejectionofH0.(Ofcourse,whether1.038iseconomicallydifferentfromoneisarelevantconsideration.)Thereislittleevidenceofserialcorrelationintheresiduals,andsowecanusethiststatisticashavinganapproximatenormaldistribution.[Forcomparison,theOLSestimateofwithoutther3terms—andusingfourmoreobservations—is1.026(se.0077).Butthetstatisticfrom(18.33)isnotnecessarilyvalid.]Therearemanyotherestimatorsofcointegratingparameters,andthiscontinuestobeaveryactiveareaofresearch.Thenotionofcointegrationappliestomorethantwoprocesses,buttheinterpretation,testing,andestimationaremuchmorecomplicated.Oneissueisthat,evenafterwenormalizeacoefficienttobeone,therecanbemanycointegratingrelationships.BDGHprovidesomediscussionandseveralreferences.ErrorCorrectionModelsInadditiontolearningaboutapotentiallong-runrelationshipbetweentwoseries,theconceptofcointegrationenrichesthekindsofdynamicmodelsatourdisposal.IfytandxtareI(1)processesandarenotcointegrated,wemightestimateadynamicmodelinfirstdifferences.Asanexample,considertheequationyt01yt10xt1xt1ut,(18.36)whereuthaszeromeangivenxt,yt1,xt1,andfurtherlags.Thisisessentiallyequation(18.16),butinfirstdifferencesratherthaninlevels.Ifweviewthisasaration-aldistributedlagmodel,wecanfindtheimpactpropensity,longrunpropensity,andlagdistributionforyasadistributedlaginx.Ifytandxtarecointegratedwithparameter,thenwehaveadditionalI(0)variableswhichwecanincludein(18.36).Letstytxt,sothatstisI(0),andassumeforthe591\nd7/14/998:36PMPage592Part3AdvancedTopicssakeofsimplicitythatsthaszeromean.Now,wecanincludelagsofstintheequation.Inthesimplestcase,weincludeonelagofst:yt01yt10xt1xt1st1ut(18.37)01yt10xt1xt1(yt1xt1)ut,whereE(utIt1)0,andIt1containsinformationonxtandallpastvaluesofxandy.Theterm(yt1xt1)iscalledtheerrorcorrectionterm,and(18.37)isanexam-pleofanerrorcorrectionmodel.(Insomeerrorcorrectionmodels,thecontempora-neouschangeinx,xt,isomitted.Whetheritisincludedornotdependspartlyonthepurposeoftheequation.Inforecasting,xtisrarelyincluded,forreasonswewillseeinSection18.5.)Anerrorcorrectionmodelallowsustostudytheshort-rundynamicsintherelation-shipbetweenyandx.Forsimplicity,considerthemodelwithoutlagsofytandxt:yt00xt(yt1xt1)ut,(18.38)where0.Ifyt1xt1,thenyinthepreviousperiodhasovershottheequilib-rium;because0,theerrorcorrectiontermworkstopushybacktowardstheequi-librium.Similarly,ifyt1xt1,theerrorcorrectionterminducesapositivechangeinybacktowardstheequilibrium.Howdoweestimatetheparametersofanerrorcorrectionmodel?Ifweknow,thisiseasy.Forexample,in(18.38),wesimplyregressytonxtandst1,wherest1(yt1xt1).EXAMPLE18.7(ErrorCorrectionModelforHoldingYields)InProblem11.6,weregressedhy6t,thethree-monthholdingyield(inpercent)frombuy-ingasix-monthT-billattimet1andsellingitattimetasathree-monthT-bill,onhy3t1,thethree-monthholdingyieldfrombuyingathree-monthT-billattimet1.Theexpec-tationshypothesisimpliesthattheslopecoefficientshouldnotbestatisticallydifferentfromone.Itturnsoutthatthereisevidenceofaunitrootin{hy3t},whichcallsintoquestionthestandardregressionanalysis.WewillassumethatbothholdingyieldsareI(1)processes.Theexpectationshypothesisimplies,ataminimum,thathy6tandhy3t1arecointegratedwithequaltoone,whichappearstobethecase(seeExercise18.14).Underthisassumption,anerrorcorrectionmodelishy6t00hy3t1(hy6t1hy3t2)ut,whereuthaszeromean,givenallhy3andhy6datedattimet1andearlier.Thelagsonthevariablesintheerrorcorrectionmodelaredictatedbytheexpectationshypothesis.UsingthedatainINTQRT.RAWgiveshyˆ6t(.090)(1.218)hy3t1(.840)(hy6t1hy3t2)hyˆ6t(.043)1(.264)hy3t1(.244)(hy6t1hy3t2)(18.39)2n122,R.790.592\nd7/14/998:36PMPage593Chapter18AdvancedTimeSeriesTopicsTheerrorcorrectioncoefficientisnegativeQUESTION18.4andverysignificant.Forexample,ifthehold-HowwouldyoutestH0:01,1intheholdingyielderroringyieldonsix-monthbillsisabovethatforcorrectionmodel?three-monthbillsbyonepoint,hy6fallsby.84pointsonaverageinthenextquarter.Interestingly,ˆ.84isnotstatisticallydifferentfrom1,asiseasilyseenbycomputingthe95%confidenceinterval.Inmanyotherexamples,thecointegratingparametermustbeestimated.Then,wereplacest1withsˆt1yt1ˆxt1,whereˆcanbevariousestimatorsof.WehavecoveredthestandardOLSestimatoraswellastheleadsandlagsestimator.Thisraisestheissueabouthowsamplingvariationinˆaffectsinferenceontheotherparametersintheerrorcorrectionmodel.Fortunately,asshownbyEngleandGranger(1987),wecanignorethepreliminaryestimationof(asymptotically).Thisisveryconvenient.TheprocedureofreplacingwithˆiscalledtheEngle-Grangertwo-stepprocedure.18.5FORECASTINGForecastingeconomictimeseriesisveryimportantinsomebranchesofeconomics,anditisanareathatcontinuestobeactivelystudied.Inthissection,wefocusonregression-basedforecastingmethods.Diebold(1998)providesacomprehensiveintro-ductiontoforecasting,includingrecentdevelopments.Weassumeinthissectionthattheprimaryfocusisonforecastingfuturevaluesofatimeseriesprocessandnotnecessarilyonestimatingcausalorstructuraleconomicmodels.Itisusefultofirstcoversomefundamentalsofforecastingthatdonotdependonaspecificmodel.Supposethatattimetwewanttoforecasttheoutcomeofyattimet1,oryt1.Thetimeperiodcouldcorrespondtoayear,aquarter,amonth,aweek,orevenaday.LetItdenoteinformationthatwecanobserveattimet.Thisinformationsetincludesyt,earliervaluesofy,andoftenothervariablesdatedattimetorearlier.Wecancombinethisinformationininnumerablewaystoforecastyt1.Isthereonebestway?Theanswerisyes,providedwespecifythelossassociatedwithforecasterror.Letftdenotetheforecastofyt1madeattimet.Wecallftaone-step-aheadforecast.Theforecasterroriset1yt1ft,whichweobserveoncetheoutcomeonyt1isobserved.Themostcommonmeasureoflossisthesameonethatleadstoordinary2leastsquaresestimationofamultiplelinearregressionmodel:thesquarederror,et1.Thesquaredforecasterrortreatspositiveandnegativepredictionerrorssymmetri-cally,andlargerforecasterrorsreceiverelativelymoreweight.Forexample,errorsof2and2yieldthesameloss,andthelossisfourtimesasgreatasforecasterrorsof1or1.Thesquaredforecasterrorisanexampleofalossfunction.Anotherpopularlossfunctionistheabsolutevalueofthepredictionerror,et1.Forreasonstobeseenshortly,wefocusnowonsquarederrorloss.593\nd7/14/998:36PMPage594Part3AdvancedTopicsGiventhesquarederrorlossfunction,wecandeterminehowtobestusetheinfor-mationattimettoforecastyt1.Butwemustrecognizethatattimet,wedonotknowet1:itisarandomvariable,becauseyt1isarandomvariable.Therefore,anyusefulcriterionforchoosingftmustbebasedonwhatweknowattimet.Itisnaturaltochoosetheforecasttominimizetheexpectedsquaredforecasterror,givenIt:22E(et1It)E[(yt1ft)It].(18.40)Abasicfactfromprobability(seePropertyCE.6inAppendixB)isthattheconditionalexpectation,E(yt1It),minimizes(18.40).Inotherwords,ifwewishtominimizetheexpectedsquaredforecasterrorgiveninformationattimet,ourforecastshouldbetheexpectedvalueofyt1givenvariablesweknowattimet.Formanypopulartimeseriesprocesses,theconditionalexpectationiseasytoobtain.Supposethat{yt:t0,1,…}isamartingaledifferencesequence(MDS)andtakeIttobe{yt,yt1,…,y0},theobservedpastofy.Bydefinition,E(yt1It)0forallt;thebestpredictionofyt1attimetisalwayszero!RecallfromSection18.2thatani.i.d.sequencewithzeromeanisamartingaledifferencesequence.Amartingaledifferencesequenceisoneinwhichthepastisnotusefulforpredict-ingthefuture.Stockreturnsarewidelythoughttobewell-approximatedasanMDSor,perhaps,withapositivemean.ThekeyisthatE(yt1yt,yt1,…)E(yt1):thecondi-tionalmeanisequaltotheunconditionalmean,inwhichcase,pastydonothelptopre-dictfuturey.Aprocess{yt}isamartingaleifE(yt1yt,yt1,…,y0)ytforallt0.[If{yt}isamartingale,then{yt}isamartingaledifferencesequence,whichiswherethelatternamecomesfrom.]Thepredictedvalueofyforthenextperiodisalwaysthevalueofyforthisperiod.AmorecomplicatedexampleistE(yt1It)yt(1)yt1…(1)y0,(18.41)where01isaparameterthatwemustchoose.Thismethodofforecastingiscalledexponentialsmoothingbecausetheweightsonthelaggedydeclinetozeroexponentially.Thereasonforwritingtheexpectationasin(18.41)isthatitleadstoaverysimplerecurrencerelation.Setf0y0.Then,fort1,theforecastscanbeobtainedasftyt(1)ft1.Inotherwords,theforecastofyt1isaweightedaverageofytandtheforecastofytmadeattimet1.Exponentialsmoothingissuitableonlyforveryspecifictimeseriesandrequireschoosing.Regressionmethods,whichweturntonext,aremoreflexible.Thepreviousdiscussionhasfocusedonforecastingyonlyoneperiodahead.Thegeneralissuesthatariseinforecastingythattimet,wherehisanypositiveinteger,aresimilar.Inparticular,ifweuseexpectedsquaredforecasterrorasourmeasureofloss,thebestpredictorisE(ythIt).Whendealingwithamultiple-step-ahead-forecast,weusethenotationft,htoindicatetheforecastofythmadeattimet.594\nd7/14/998:36PMPage595Chapter18AdvancedTimeSeriesTopicsTypesofRegressionModelsUsedforForecastingTherearemanydifferentregressionmodelsthatwecanusetoforecastfuturevaluesofatimeseries.ThefirstregressionmodelfortimeseriesdatafromChapter10wasthestaticmodel.Toseehowwecanforecastwiththismodel,assumethatwehaveasingleexplanatoryvariable:yt01ztut.(18.42)Suppose,forthemoment,thattheparameters0and1areknown.Writethisequationattimet1asyt101zt1ut1.Now,ifzt1isknownattimet,sothatitisanelementofItandE(ut1It)0,thenE(yt1It)01zt1,whereItcontainszt1,yt,zt,…,y1,z1.Theright-handsideofthisequationisthefore-castofyt1attimet.Thiskindofforecastisusuallycalledaconditionalforecastbecauseitisconditionalonknowingthevalueofzattimet1.Unfortunately,atanytime,werarelyknowthevalueoftheexplanatoryvariablesinfuturetimeperiods.Exceptionsincludetimetrendsandseasonaldummyvariables,whichwecoverexplicitlybelow,butotherwiseknowledgeofzt1attimetisrare.Sometimes,wewishtogenerateconditionalforecastsforseveralvaluesofzt1.Anotherproblemwith(18.42)asamodelforforecastingisthatE(ut1It)0meansthat{ut}cannotcontainserialcorrelation,somethingwehaveseentobefalseinmoststaticregressionmodels.[Problem18.8asksyoutoderivetheforecastinasim-pledistributedlagmodelwithAR(1)errors.]Ifzt1isnotknownattimet,wecannotincludeitinIt.Then,wehaveE(yt1It)01E(zt1It).Thismeansthatinordertoforecastyt1,wemustfirstforecastzt1,basedonthesameinformationset.Thisisusuallycalledanunconditionalforecastbecausewedonotassumeknowledgeofzt1attimet.Unfortunately,thisissomewhatofamisnomer,asourforecastisstillconditionalontheinformationinIt.Butthenameisentrenchedinforecastingliterature.Forforecasting,unlessweareweddedtothestaticmodelin(18.42)forotherrea-sons,itmakesmoresensetospecifyamodelthatdependsonlyonlaggedvaluesofyandz.Thissavesustheextrastepofhavingtoforecastaright-handsidevariablebeforeforecastingy.Thekindofmodelwehaveinmindisyt01yt11zt1ut(18.43)E(utIt1)0,whereIt1containsyandzdatedattimet1andearlier.Now,theforecastofyt1attimetis01yt1zt;ifweknowtheparameters,wecanjustpluginthevaluesofytandzt.Ifweonlywanttousepastytopredictfuturey,thenwecandropzt1from(18.43).Naturally,wecanaddmorelagsofyorzandlagsofothervariables.Especiallyforfore-castingonestepahead,suchmodelscanbeveryuseful.595\nd7/14/998:36PMPage596Part3AdvancedTopicsOne-Step-AheadForecastingObtainingaforecastoneperiodafterthesampleendsisrelativelystraightforwardusingmodelssuchas(18.43).Asusual,letnbethesamplesize.Theforecastofyn1isfˆnˆ0ˆ1ynˆ1zn,(18.44)whereweassumethattheparametershavebeenestimatedbyOLS.Weuseahatonfntoemphasizethatwehaveestimatedtheparametersintheregressionmodel.(Ifweknewtheparameters,therewouldbenoestimationerrorintheforecast.)Theforecasterror—whichwewillnotknowuntiltimen1—iseˆn1yn1fˆn.(18.45)Ifweaddmorelagsofyorztotheforecastingequation,wesimplylosemoreobser-vationsatthebeginningofthesample.Theforecastfˆnofyn1isusuallycalledapointforecast.Wecanalsoobtainafore-castinterval.Aforecastintervalisessentiallythesameasapredictioninterval,whichwestudiedinSection6.4.Thereweshowedhow,undertheclassicallinearmodelassumptions,toobtainanexact95%predictioninterval.Aforecastintervalisobtainedinexactlythesameway.Ifthemodeldoesnotsatisfytheclassicallinearmodelassump-tions—forexample,ifitcontainslaggeddependentvariables,asin(18.44)—thefore-castintervalisstillapproximatelyvalid,providedutgivenIt1isnormallydistributedwithzeromeanandconstantvariance.(ThisensuresthattheOLSestimatorsareapprox-imatelynormallydistributedwiththeusualOLSvariancesandthatun1isindependent2oftheOLSestimatorswithmeanzeroandvariance.)Letse(fˆn)bethestandarderroroftheforecastandletˆbethestandarderroroftheregression.[FromSection6.4,wecanobtainfˆnandse(fˆn)astheinterceptanditsstandarderrorfromtheregressionofyton(yt1yn)and(zt1zn),t1,2,…,n;thatis,wesubtractthetimenvalueofyfromeachlaggedy,andsimilarlyforz,beforedoingtheregression.]Then,221/2se(eˆn1){[se(fˆn)]ˆ},(18.46)andthe(approximate)95%forecastintervalisfˆn1.96se(eˆn1).(18.47)Becausese(fˆn)isroughlyproportionalto1/n,se(fˆn)isusuallysmallrelativetotheuncertaintyintheerrorun1,asmeasuredbyˆ.[Someeconometricspackagescom-puteforecastintervalsroutinely,butothersrequiresomesimplemanipulationstoobtain(18.47).]EXAMPLE18.8(ForecastingtheU.S.UnemploymentRate)WeusethedatainPHILLIPS.RAW,whichisfortheyears1948through1996,toforecasttheU.S.civilianunemploymentratefor1997.Weusetwomodels.ThefirstisasimpleAR(1)modelforunem:596\nd7/14/998:36PMPage597Chapter18AdvancedTimeSeriesTopicsuneˆmt(1.572)(.732)unemt1uneˆmt1(.577)(.097)unemt1(18.48)2n48,R¯.544,ˆ1.049.Inasecondmodel,weaddinflationwithalagofoneyear:uneˆmt(1.304)(.647)unemt1(.184)inft1uneˆmt1(.490)(.084)unemt1(.041)inft1(18.49)2n48,R¯.677,ˆ.883.Thelaggedinflationrateisverysignificantin(18.49)(t4.5),andtheadjustedR-squaredfromthesecondequationismuchhigherthanthatfromthefirst.Nevertheless,thisdoesnotnecessarilymeanthatthesecondequationwillproduceabetterforecastfor1997.Allwecansaysofaristhat,usingthedataupthrough1996,alagofinflationhelpstoexplainvariationintheunemploymentrate.Toobtaintheforecastsfor1997,weneedtoknowunemandinfin1996.Theseare5.4and3.0,respectively.Therefore,theforecastofunem1997fromequation(18.48)is1.572.732(5.4),orabout5.52.Theforecastfromequation(18.49)is1.304.647(5.4).184(3.0),orabout5.35.Theactualcivilianunemploymentratefor1997was4.9,andsobothequationsover-predicttheactualrate.Thesecondequationdoesprovideasomewhatbetterforecast.Wecaneasilyobtaina95%forecastinterval.Whenweregressunemton(unemt15.4)and(inft13.0),weobtain5.35astheintercept—whichwealreadycomputedasthe2forecast—andse(fˆn).137.Therefore,becauseˆ.883,wehavese(eˆn1)[(.137)21/2(.883)].894.The95%forecastintervalfrom(18.47)is5.351.96(.894),orabout[3.6,7.1].Thisisawideinterval,andtherealized1997value,4.9,iswellwithintheinterval.Asexpected,thestandarderrorofun1,whichis.883,isaverylargefractionofse(eˆn1).Aprofessionalforecastermustusuallyproduceaforecastforeverytimeperiod.Forexample,attimen,sheorheproducesaforecastofyn1.Then,whenyn1andzn1becomeavailable,heorshemustforecastyn2.Eveniftheforecasterhassettledonmodel(18.43),therearetwochoicesforforecastingyn2.Thefirstistouseˆ0ˆ1yn1ˆ1zn1,wheretheparametersareestimatedusingthefirstnobservations.Thesecondpossibilityistoreestimatetheparametersusingalln1observationsandthentousethesameformulatoforecastyn2.Toforecastinsubsequenttimeperiods,wecangenerallyusetheparameterestimatesobtainedfromtheinitialnobservations,orwecanupdatetheregressionparameterseachtimeweobtainanewdatapoint.Whilethelatterapproachrequiresmorecomputation,theextraburdenisrelativelyminor,anditcan(althoughitneednot)workbetterbecausetheregressioncoefficientsadjustatleastsomewhattothenewdatapoints.Asaspecificexample,supposewewishtoforecasttheunemploymentratefor1998,usingthemodelwithasinglelagofunemandinf.Thefirstpossibilityistojustplugthe1997valuesofunemploymentandinflationintotheright-handsideof(18.49).597\nd7/14/998:36PMPage598Part3AdvancedTopicsWithunem4.9andinf2.3in1997,wehaveaforecastforunem1998ofabout4.9.(Itisjustacoincidencethatthisisthesameasthe1997unemploymentrate.)Thesec-ondpossibilityistoreestimatetheequationbyaddingthe1997observationandthenusingthisnewequation(seeExercise18.15).Themodelinequation(18.43)isoneequationinwhatisknownasavectorautore-gressive(VAR)model.WeknowwhatanautoregressivemodelisfromChapter11:wemodelasingleseries,{yt},intermsofitsownpast.Invectorautoregressivemodels,wemodelseveralseries—which,ifyouarefamiliarwithlinearalgebra,iswheretheword“vector”comesfrom—intermsoftheirownpast.Ifwehavetwoseries,ytandzt,avec-torautoregressionconsistsofequationsthatlooklikeyt01yt11zt12yt22zt2…(18.50)andzt01yt11zt12yt22zt2…,whereeachequationcontainsanerrorthathaszeroexpectedvaluegivenpastinforma-tiononyandz.Inequation(18.43)—andintheexampleestimatedin(18.49)—weassumedthatonelagofeachvariablecapturedallofthedynamics.(AnFtestforjointsignificanceofunemt2andinft2confirmsthatonlyonelagofeachisneeded.)AsExample18.8illustrates,VARequationscanbeusefulforforecasting.Inmanycases,weareinterestedinforecastingonlyonevariable,y,inwhichcaseweonlyneedtoestimateandanalyzetheequationfory.Nothingpreventsusfromaddingotherlaggedvariables,saywt1,wt2,…,toequation(18.50).Suchequationsareefficientlyesti-matedbyOLS,providedwehaveincludedenoughlagsofallvariablesandtheequationsatisfiesthehomoskedasticityassumptionfortimeseriesregressions.Equationssuchas(18.50)allowustotestwhether,aftercontrollingforpasty,pastzhelptoforecastyt.Generally,wesaythatzGrangercausesyifE(ytIt1)E(ytJt1),(18.51)whereIt1containspastinformationonyandz,andJt1containsonlyinformationonpasty.When(18.51)holds,pastzisuseful,inadditiontopasty,forpredictingyt.Theterm“causes”in“Grangercauses”shouldbeinterpretedwithcaution.Theonlysenseinwhichz“causes”yisgivenin(18.51).Inparticular,ithasnothingtosayaboutcon-temporaneouscausalitybetweenyandz,soitdoesnotallowustodeterminewhetherztisanexogenousorendogenousvariableinanequationrelatingyttozt.(ThisisalsowhythenotionofGrangercausalitydoesnotapplyinpurecross-sectionalcontexts.)OnceweassumealinearmodelanddecidehowmanylagsofyshouldbeincludedinE(ytyt1,yt2,…),wecaneasilytestthenullhypothesisthatzdoesnotGrangercausey.Tobemorespecific,supposethatE(ytyt1,yt2,…)dependsononlythreelags:yt01yt12yt23yt3utE(utyt1,yt2,…)0.598\nd7/14/998:36PMPage599Chapter18AdvancedTimeSeriesTopicsNow,underthenullhypothesisthatzdoesnotGrangercausey,anylagsofzthatweaddtotheequationshouldhavezeropopulationcoefficients.Ifweaddzt1,thenwecansimplydoattestonzt1.Ifweaddtwolagsofz,thenwecandoanFtestforjointsignificanceofzt1andzt2intheequationyt01yt12yt23yt31zt12zt2ut.(Ifthereisheteroskedasticity,wecanusearobustformofthetest.Therecannotbeser-ialcorrelationunderH0becausethemodelisdynamicallycomplete.)Asapracticalmatter,howdowedecideonwhichlagsofyandztoinclude?First,westartbyestimatinganautoregressivemodelforyandperformingtandFteststodeterminehowmanylagsofyshouldappear.Withannualdata,thenumberoflagsistypicallysmall,sayoneortwo.Withquarterlyormonthlydata,thereareusuallymanymorelags.Onceanautoregressivemodelforyhasbeenchosen,wecantestforlagsofz.Thechoiceoflagsofzislessimportantbecause,whenzdoesnotGrangercausey,nosetoflaggedz’sshouldbesignificant.Withannualdata,oneortwolagsaretypi-callyused;withquarterlydata,usuallyfouroreight;andwithmonthlydata,perhapssix,12,ormaybeeven24,givenenoughdata.WehavealreadydoneoneexampleoftestingforGrangercausalityinequation(18.49).TheautoregressivemodelthatbestfitsunemploymentisanAR(1).Inequation(18.49),weaddedasinglelagofinflation,anditwasverysignificant.Therefore,infla-tionGrangercausesunemployment.ThereisanextendeddefinitionofGrangercausalitythatisoftenuseful.Let{wt}beathirdseries(or,itcouldrepresentseveraladditionalseries).Then,zGrangercausesyconditionalonwif(18.51)holds,butnowIt1containspastinformationony,z,andw,whileJt1containspastinformationonyandw.ItiscertainlypossiblethatzGrangercausesy,butzdoesnotGrangercauseyconditionalonw.AtestofthenullthatzdoesnotGrangercauseyconditionalonwisobtainedbytestingforsignificanceoflaggedzinamodelforythatalsodependsonlaggedyandlaggedw.Forexample,totestwhethergrowthinthemoneysupplyGrangercausesgrowthinrealGDP,conditionalonthechangeininterestrates,wewouldregressgGDPtonlagsofgGDP,int,andgManddosignificancetestsonthelagsofgM.[See,forexample,StockandWatson(1989).]ComparingOne-Step-AheadForecastsInalmostanyforecastingproblem,thereareseveralcompetingmethodsforforecast-ing.Evenwhenwerestrictattentiontoregressionmodels,therearemanypossibilities.Whichvariablesshouldbeincluded,andwithhowmanylags?Shouldweuselogs,lev-elsofvariables,orfirstdifferences?Inordertodecideonaforecastingmethod,weneedawaytochoosewhichoneismostsuitable.Broadly,wecandistinguishbetweenin-samplecriteriaandout-of-samplecriteria.Inaregressioncontext,in-samplecriteriaincludeR-squaredandespeciallyadjustedR-squared.Therearemanyothermodelselectionstatistics,butwewillnotcoverthosehere[see,forexample,Ramanathan(1995,Chapter4)].Forforecasting,itisbettertouseout-of-samplecriteria,asforecastingisessentiallyanout-of-sampleproblem.Amodelmightprovideagoodfittoyinthesampleusedto599\nd7/14/998:36PMPage600Part3AdvancedTopicsestimatetheparameters.Butthisneednottranslatetogoodforecastingperformance.Anout-of-samplecomparisoninvolvesusingthefirstpartofasampletoestimatetheparametersofthemodelandsavingthelatterpartofthesampletogaugeitsforecast-ingcapabilities.Thismimicswhatwewouldhavetodoinpracticeifwedidnotyetknowthefuturevaluesofthevariables.Supposethatwehavenmobservations,whereweusethefirstnobservationstoestimatetheparametersinourmodelandsavethelastmobservationsforforecasting.Letfˆnhbetheone-step-aheadforecastofynh1forh0,1,…,m1.Themforecasterrorsareeˆnh1ynh1fˆnh.Howshouldwemeasurehowwellourmodelfore-castsywhenitisoutofsample?Twomeasuresaremostcommon.Thefirstistherootmeansquarederror(RMSE):m11/212RMSEmeˆnh1.(18.52)h0Thisisessentiallythesamplestandarddeviationoftheforecasterrors(withoutanydegreesoffreedomadjustment).IfwecomputeRMSEfortwoormoreforecastingmethods,thenwepreferthemethodwiththesmallestout-of-sampleRMSE.Asecondcommonmeasureisthemeanabsoluteerror(MAE),whichistheaver-ageoftheabsoluteforecasterrors:m11MAEmeˆnh1.(18.53)h0Again,wepreferasmallerMAE.Otherpossiblecriteriaincludeminimizingthelargestoftheabsolutevaluesoftheforecasterrors.EXAMPLE18.9(Out-of-SampleComparisonsofUnemploymentForecasts)InExample18.8,wefoundthatequation(18.49)fitbetterinoursamplethan(18.48)did,and,atleastforforecasting1997,themodelwithlaggedinflationworkedbetter.Now,weestimatebothmodelsusingdatathrough1989,saving1990through1996forout-of-samplecomparisons.Thisleavessevenout-of-sampleobservations(n41andm7,tobeprecise).FortheAR(1)model,RMSE.632,andMAE.515.Forthemodelthataddslaggedinflation,RMSE.550,andMAE.362.Thus,byeithermeasure,themodelthatincludesinft1producesbetterout-of-sampleforecastsforthe1990s.Inthiscase,thein-sampleandout-of-samplecriteriabothchoosethesamemodel.Ratherthanusingonlythefirstnobservationstoestimatetheparametersofthemodel,wecanreestimatethemodelseachtimeweaddanewobservationandusethenewmodeltoforecastthenexttimeperiod.600\nd7/14/998:36PMPage601Chapter18AdvancedTimeSeriesTopicsMultiple-Step-AheadForecastsForecastingmorethanoneperiodaheadisgenerallymoredifficultthanforecastingoneperiodahead.Wecanformalizethisasfollows.Supposeweconsiderforecastingyt1attimetandatanearliertimeperiods(sothatst).ThenVar[yt1E(yt1It)]Var[yt1E(yt1Is)],wheretheinequalityisusuallystrict.Wewillnotprovethisresultgenerally,but,intuitively,itmakessense:theforecasterrorvarianceinpredictingyt1islargerwhenwemakethatforecastbasedonlessinformation.If{yt}followsanAR(1)model(whichincludesarandomwalk,possiblywithdrift),wecaneasilyshowthattheerrorvarianceincreaseswiththeforecasthorizon.Themodelisytyt1utE(utIt1)0,It1{yt1,yt2,…},2and{ut}hasconstantvarianceconditionalonIt1.Attimeth1,ourfore-castofythisyth1,andtheforecasterrorissimplyuth.Therefore,theone-2step-aheadforecastvarianceissimply.Tofindmultiple-step-aheadforecasts,wehave,byrepeatedsubstitution,h1hyth(1…)yth1h2ut1ut2…uth.Attimet,theexpectedvalueofutj,forallj1,iszero.Soh1hE(ythIt)(1…)yt,(18.54)h1h2andtheforecasterroriset,hut1ut2…uth.Thisisasumofuncorrelatedrandomvariables,andsothevarianceofthesumisthesumofthevari-22(h1)2(h2)22ances:Var(et,h)[…1].Because0,eachtermmul-22tiplyingispositive,andsotheforecasterrorvarianceincreaseswithh.When1,22theforecastvarianceconvergesto/(1),whichisjusttheunconditionalvariance2ofyt.Inthecaseofarandomwalk(1),ft,hhyt,andVar(et,h)h:thefore-castvariancegrowswithoutboundasthehorizonhincreases.Thisdemonstratesthatitisverydifficulttoforecastarandomwalk,withorwithoutdrift,faroutintothefuture.Forexample,forecastsofinterestratesfartherintothefuturebecomelessprecise.Equation(18.54)showsthatusingtheAR(1)modelformulti-stepforecastingiseasy,oncewehaveestimatedbyOLS.Theforecastofynhattimenish1hfˆn,h(1ˆ…ˆ)ˆˆyn.(18.55)Obtainingforecastintervalsisharder,unlessh1,becauseobtainingthestandarderroroffˆn,hisdifficult.Nevertheless,thestandarderroroffˆn,hisusuallysmall,comparedwiththestandarddeviationoftheerrorterm,andthelattercanbeestimatedas2(h1)2(h2)21/2ˆ[ˆˆ…ˆ1],whereˆisthestandarderroroftheregressionfromtheAR(1)estimation.Wecanusethistoobtainanapproximateconfidenceinter-val.Forexample,whenh2,anapproximate95%confidenceinterval(forlargen)is601\nd7/14/998:36PMPage602Part3AdvancedTopics21/2fˆn,21.96ˆ(1ˆ).(18.56)Becauseweareunderestimatingthestandarddeviationofynh,thisintervalistoonar-row,butperhapsnotbymuch,especiallyifnislarge.Alesstraditional,butuseful,approachistoestimateadifferentmodelforeachfore-casthorizon.Forexample,supposewewishtoforecastytwoperiodsahead.IfItdependsonlyonyupthroughtimet,wemightassumethatE(yt2It)01yt[which,aswesawearlier,holdsif{yt}followsanAR(1)model].Wecanestimate0and1byregressingytonaninterceptandonyt2.Eventhoughtheerrorsinthisequa-tioncontainserialcorrelation—errorsinadjacentperiodsarecorrelated—wecanobtainconsistentandapproximatelynormalestimatorsof0and1.Theforecastofyn2attimenissimplyfˆn,2ˆ0ˆ1yn.Further,andveryimportantly,thestandarderroroftheregressionisjustwhatweneedforcomputingaconfidenceintervalforthefore-cast.Unfortunately,togetthestandarderroroffˆn,2,usingthetrickforaone-step-aheadforecastrequiresustoobtainaserialcorrelation-robuststandarderrorofthekinddescribedinSection12.5.Thisstandarderrorgoestozeroasngetslargewhilethevari-anceoftheerrorisconstant.Therefore,wecangetanapproximateintervalbyusing(18.56)andbyputtingtheSERfromtheregressionofytonyt2inplaceofˆ(121/2ˆ).Butweshouldrememberthatthisstillignorestheestimationerrorinˆ0andˆ1.Wecanalsocomputemulti-step-aheadforecastswithmorecomplicatedautore-gressivemodels.Forexample,suppose{yt}followsanAR(2)modelandthatattimen,wewishtoforecastyn2.Now,yn21yn12ynun2,andsoE(yn2In)1E(yn1In)2yn.Wecanwritethisasfn,21fn,12yn,sothatthetwo-step-aheadforecastattimencanbeobtained,oncewegettheone-step-aheadforecast.IftheparametersoftheAR(2)modelhavebeenestimatedbyOLS,thenweoperationalizethisasfˆn,2ˆˆ1fˆn,1ˆ2yn.(18.57)Now,fˆn,1ˆˆ1ynˆ2yn1,whichwecancomputeattimen.Then,weplugthisinto(18.57),alongwithyn,toobtainfˆn,2.Foranyh2,obtaininganyh-step-aheadforecastforanAR(2)modeliseasytofindinarecursivemanner:fˆn,hˆˆ1fˆn,h1ˆ2fˆn,h2.Similarreasoningcanbeusedtoobtainmulti-step-aheadforecastsforVARmodels.Toillustrate,supposewehaveyt01yt11zt1ut(18.58)andzt01yt11zt1vt.602\nd7/14/998:36PMPage603Chapter18AdvancedTimeSeriesTopicsNow,ifwewishtoforecastyn1attimen,wesimplyusefˆn,1ˆ0ˆ1ynˆ1zn.Likewise,theforecastofzn1attimenis(say)gˆn,1ˆ0ˆ1ynˆ1zn.Now,supposewewishtoobtainatwo-step-aheadforecastofyattimen.From(18.58),wehaveE(yn2In)01E(yn1In)1E(zn1In)[becauseE(un2In)0],andsowecanwritetheforecastasfˆn,2ˆ0ˆ1fˆn,1ˆ1gˆn,1.(18.59)Thisequationshowsthatthetwo-step-aheadforecastforydependsontheone-step-aheadforecastsforyandz.Generally,wecanbuildupmulti-step-aheadforecastsofybyusingtherecursiveformulafˆn,hˆ0ˆ1fˆn,h1ˆ1gˆn,h1,h2.EXAMPLE18.10(Two-Year-AheadForecastfortheUnemploymentRate)Touseequation(18.49)toforecastunemploymenttwoyearsout—say,the1998rateusingthedatathrough1996—weneedamodelforinflation.ThebestmodelforinfintermsoflaggedunemandinfappearstobeasimpleAR(1)model(unem1isnotsignificantwhenaddedtotheregression):inˆft(1.277)(.665)inft1inˆft0(.558)(.107)inft122n48,R.457,R¯.445.Ifweplugthe1996valueofinfintothisequation,wegettheforecastofinffor1997:inˆf19973.27.Now,wecanplugthis,alongwithuneˆm19975.35(whichweobtainedearlier)into(18.59)toforecastunem1998:uneˆm19981.304.647(5.35).184(3.27)5.37.Remember,thisforecastusesinformationonlythrough1996.Theone-step-aheadforecastofunem1998,obtainedbypluggingthe1997valuesofunemandinfinto(18.48),wasabout4.90.Youcanfindtheactualcivilianunemploymentratefor1998inarecentEconomicReportofthePresident.Youwillseethattheone-step-aheadforecastturnsouttobemuchcloserthanthetwo-step-aheadforecast.Justaswithone-step-aheadforecasting,anout-of-samplerootmeansquarederrororameanabsoluteerrorcanbeusedtochooseamongmulti-step-aheadforecastingmethods.ForecastingTrending,Seasonal,andIntegratedProcessesWenowturntoforecastingseriesthateitherexhibittrends,haveseasonality,orhaveunitroots.RecallfromChapters10and11thatoneapproachtohandlingtrending603\nd7/14/998:36PMPage604Part3AdvancedTopicsdependentorindependentvariablesinregressionmodelsistoincludetimetrends,themostpopularbeingalineartrend.Trendscanbeincludedinforecastingequationsaswell,althoughtheymustbeusedwithcaution.Inthesimplestcase,supposethat{yt}hasalineartrendbutisunpredictablearoundthattrend.Then,wecanwriteyttut,E(utIt1)0,t1,2,…,(18.60)where,asusual,It1containsinformationobservedthroughtimet1(whichincludesatleastpasty).Howdoweforecastynhattimenforanyh1?Thisissimplebecause2E(ynhIn)(nh).TheforecasterrorvarianceissimplyVar(ut)(assum-ingaconstantvarianceovertime).IfweestimateandbyOLSusingthefirstnobservations,thenourforecastforynhattimenisfˆn,hˆˆ(nh).Inotherwords,wesimplyplugthetimeperiodcorrespondingtoyintotheestimatedtrendfunction.Forexample,ifweusethen131observationsinBARIUM.RAWtoforecastmonthlyChineseimportsofbariumchloridetotheUnitedStates,weobtainˆ249.56andˆ5.15.ThesampleperiodendsinDecember1988,sotheforecastofChineseimportssixmonthslateris249.565.15(137)955.11,measuredasshorttons.Forcomparison,theDecember1988valueis1,087.81,soitisgreaterthantheforecastedvaluesixmonthslater.TheseriesanditsestimatedtrendlineareshowninFigure18.2.Figure18.2ChinesebariumchlorideimportsintotheUnitedStates(inshorttons)anditsestimatedlineartrendline,249.565.15t.bariumchloride(shorttons)150010005004013570100131t604\nd7/14/998:36PMPage605Chapter18AdvancedTimeSeriesTopicsAswediscussedinChapter10,mosteconomictimeseriesarebettercharacterizedashaving,atleastapproximately,aconstantgrowthrate,whichsuggeststhatlog(yt)followsalineartimetrend.Supposeweusenobservationstoobtaintheequationloˆg(yt)ˆˆt,t1,2,…,n.(18.61)Then,toforecastlog(y)atanyfuturetimeperiodnh,wejustplugnhintothetrendequation,asbefore.Butthisdoesnotallowustoforecasty,whichisusuallyQUESTION18.5whatwewant.ItistemptingtosimplySupposeyoumodel{yt:t1,2,…,46}asalineartimetrend,whereexponentiateˆˆ(nh)toobtainthedataareannualstartingin1950andendingin1995.Definetheforecastforynh,butthisisnotquitevariableyeartasrangingfrom50whent1to95whent46.Ifright,forthesamereasonswegaveinyouestimatetheequationyˆtˆˆyeart,howdoˆandˆcom-Section6.4.Wemustproperlyaccountforparewithˆandˆinyˆtˆˆt?Howwillforecastsfromthetwotheerrorimplicitin(18.61).Thesimplestequationscompare?waytodothisistousethenobservationstoregressytonexp(logyˆt)withoutaninter-cept.Letˆbetheslopecoefficientonexp(logyˆt).Then,theforecastofyinperiodnhissimplyfˆn,hˆexp[ˆˆ(nh)].(18.62)Asanexample,ifweusethefirst687weeksofdataontheNewYorkstockexchangeindexinNYSE.RAW,weobtainˆ3.782andˆ.0019[byregressinglog(pricet)onalineartimetrend];thisshowsthattheindexgrowsabout.2%perweek,onaverage.Whenweregresspriceontheexponentiatedfittedvalues,weobtainˆ1.018.Now,weforecastpricefourweeksout,whichisthelastweekinthesample,using(18.62):1.018exp[3.782.0019(691)]166.12.Theactualvalueturnedouttobe164.25,sowehavesomewhatover-predicted.Butthisresultismuchbetterthanifweestimatealineartimetrendforthefirst687weeks:theforecastedvalueforweek691is152.23,whichisasubstantialunder-prediction.Whiletrendmodelscanbeusefulforprediction,theymustbeusedwithcaution,especiallyforforecastingfarintothefutureintegratedseriesthathavedrift.Thepoten-tialproblemcanbeseenbyconsideringarandomwalkwithdrift.Attimeth,wecanwriteythasythhytut1…uth,whereisthedriftterm(usually0),andeachutjhaszeromeangivenItandcon-2stantvariance.Aswesawearlier,theforecastofythattimetisE(ythIt)2hyt,andtheforecasterrorvarianceish.Whathappensifweusealineartrendmodel?Lety0betheinitialvalueoftheprocessattimezero,whichwetakeasnonran-dom.Then,wecanalsowriteythy0(th)u1u2…uthy0(th)vth.605\nd7/14/998:36PMPage606Part3AdvancedTopicsThislookslikealineartrendmodelwiththeintercepty0.Buttheerror,vth,while2havingmeanzero,hasvariance(th).Therefore,ifweusethelineartrend2y0(th)toforecastythattimet,theforecasterrorvarianceis(th),ascom-2paredwithhwhenweusehyt.Theratiooftheforecastvariancesis(th)/h,whichcanbebigforlarget.Thebottomlineisthatweshouldnotusealineartrendtoforecastarandomwalkwithdrift.(Problem18.17asksyoutocompareforecastsfromacubictrendlineandthosefromthesimplerandomwalkmodelforthegeneralfertil-ityrateintheUnitedStates.)Deterministictrendscanalsoproducepoorforecastsifthetrendparametersareesti-matedusingolddataandtheprocesshasasubsequentshiftinthetrendline.Sometimes,exogenousshocks—suchastheoilcrisesofthe1970s—canchangethetra-jectoryoftrendingvariables.Ifanoldtrendlineisusedtoforecastfarintothefuture,theforecastscanbewayoff.Thisproblemcanbemitigatedbyusingthemostrecentdataavailabletoobtainthetrendlineparameters.Nothingpreventsusfromcombiningtrendswithothermodelsforforecasting.Forexample,wecanaddalineartrendtoanAR(1)model,whichcanworkwellforfore-castingserieswithlineartrendsbutwhicharealsostableARprocessesaroundthetrend.Itisalsostraightforwardtoforecastprocesseswithdeterministicseasonality(monthlyorquarterlyseries).Forexample,thefileBARIUM.RAWcontainsthemonthlyproductionofgasolineintheUnitedStatesfrom1978through1988.Thisserieshasnoobvioustrend,butitdoeshaveastrongseasonalpattern.(Gasolinepro-ductionishigherinthesummermonthsandinDecember.)Inthesimplestmodel,wewouldregressgas(measuredingallons)onelevenmonthdummies,sayforFebruarythroughDecember.Then,theforecastforanyfuturemonthissimplytheinterceptplusthecoefficientontheappropriatemonthdummy.(ForJanuary,theforecastisjusttheinterceptintheregression.)Wecanalsoaddlagsofvariablesandtimetrendstoallowforgeneralserieswithseasonality.Forecastingprocesseswithunitrootsalsodeservesspecialattention.Earlier,weobtainedtheexpectedvalueofarandomwalkconditionaloninformationthroughtimen.Toforecastarandomwalk,withpossibledrift,hperiodsintothefutureattimen,weusefˆn,hˆhyn,whereˆisthesampleaverageoftheytupthroughtn.(Ifthereisnodrift,wesetˆ0.)Thisapproachimposestheunitroot.AnalternativewouldbetoestimateanAR(1)modelfor{yt}andtousetheforecastformula(18.55).Thisapproachdoesnotimposeaunitroot,butifoneispresent,ˆconvergesinproba-bilitytooneasngetslarge.Nevertheless,ˆcanbesubstantiallydifferentthanone,especiallyifthesamplesizeisnotverylarge.Thematterofwhichapproachproducesbetterout-of-sampleforecastsisanempiricalissue.IfintheAR(1)model,islessthanone,evenslightly,theAR(1)modelwilltendtoproducebetterlong-runforecasts.Generally,therearetwoapproachestoproducingforecastsforI(1)processes.Thefirstistoimposeaunitroot.Foraone-step-aheadforecast,weobtainamodeltofore-castthechangeiny,yt1,giveninformationupthroughtimet.Then,becauseyt1yt1yt,E(yt1It)E(yt1It)yt.Therefore,ourforecastofyn1attimenisjustfˆngˆnyn,wheregˆnistheforecastofyn1attimen.Typically,anARmodel(whichisnecessar-ilystable)isusedforyt,oravectorautoregression.606\nd7/14/998:36PMPage607Chapter18AdvancedTimeSeriesTopicsThiscanbeextendedtomulti-step-aheadforecastsbywritingynhasynh(ynhynh1)(ynh1ynh2)…(yn1yn)yn,orynhynhynh1…yn1yn.Therefore,theforecastofynhattimenisfˆn,hgˆn,hgˆn,h1…gˆn,1yn,(18.63)wheregˆn,jistheforecastofynjattimen.Forexample,wemightmodelytasasta-bleAR(1),obtainthemulti-step-aheadforecastsfrom(18.55)(butwithˆandˆobtainedfromytonyt1,andynreplacedwithyn),andthenplugtheseinto(18.63).ThesecondapproachtoforecastingI(1)variablesistouseageneralARorVARmodelfor{yt}.Thisdoesnotimposetheunitroot.Forexample,ifweuseanAR(2)model,yt1yt12yt2ut,(18.64)then121.Ifweplugin112andrearrange,weobtainyt2yt1ut,whichisastableAR(1)modelinthedifferencethattakesusbacktothefirstapproachdescribedearlier.Nothingpreventsusfromestimating(18.64)directlybyOLS.Onenicethingaboutthisregressionisthatwecanusetheusualtstatisticonˆ2todetermineifyt2issignificant.(Thisassumesthatthehomoskedasticityassumptionholds;ifnot,wecanusetheheteroskedasticity-robustform.)Wewillnotshowthisformally,but,intuitively,itfollowsbyrewritingtheequationasytyt12yt1ut,where12.Evenif1,2isminusthecoefficientonasta-tionary,weaklydependentprocess{yt1}.Becausetheregressionresultswillbeiden-ticalto(18.64),wecanuseitdirectly.Asanexample,letusestimateanAR(2)modelforthegeneralfertilityrateinFER-TIL3.RAW,usingtheobservationsupthrough1979.(InExercise18.17youareaskedtousethismodelforforecasting,whichiswhywesavesomeobservationsattheendofthesample.)gfˆrt(3.22)(1.272)gfrt1(.311)gfrt2(18.65)gfˆrt(2.92)1(.120)gfrt1(.121)gfrt222n65,R.949,R¯.947.Thetstatisticonthesecondlagisabout2.57,whichisstatisticallydifferentfromzeroataboutthe1%level.(Thefirstlagalsohasaverysignificanttstatistic,whichhasanapproximatetdistributionbythesamereasoningusedforˆ2.)TheR-squared,adjustedornot,isnotespeciallyinformativeasagoodness-of-fitmeasurebecausegfrapparentlycontainsaunitroot,anditmakeslittlesensetoaskhowmuchofthevarianceingfrweareexplaining.Thecoefficientsonthetwolagsin(18.65)addupto.961,whichisclosetoandnotstatisticallydifferentfromone(ascanbeverifiedbyapplyingtheaugmentedDickey-607\nd7/14/998:36PMPage608Part3AdvancedTopicsFullertesttotheequationgfrtgfrt1gfrt1ut).Eventhoughwehavenotimposedtheunitrootrestriction,wecanstilluse(18.65)forforecasting,aswedis-cussedearlier.Beforeendingthissection,wepointoutonepotentialimprovementinforecastinginthecontextofvectorautoregressivemodelswithI(1)variables.Suppose{yt}and{zt}areeachI(1)processes.Oneapproachforobtainingforecastsofyistoestimateabivari-ateautoregressioninthevariablesytandztandthentouse(18.63)togenerateone-ormulti-step-aheadforecasts;thisisessentiallythefirstapproachwedescribedearlier.However,ifytandztarecointegrated,wehavemorestationary,stablevariablesintheinformationsetthatcanbeusedinforecastingy:namely,lagsofytzt,whereisthecointegratingparameter.Asimpleerrorcorrectionmodelisyt01yt11zt11(yt1zt1)et,(18.66)E(etIt1)0.Toforecastyn1,weuseobservationsupthroughntoestimatethecointegratingpara-meter,,andthenestimatetheparametersoftheerrorcorrectionmodelbyOLS,asdescribedinSection18.4.Forecastingyn1iseasy:wejustplugyn,zn,andynˆznintotheequation.Havingobtainedtheforecastofyn1,weaddittoyn.Byrearrangingtheerrorcorrectionmodel,wecanwriteyt01yt12yt21zt12zt2ut,(18.67)where111,21,andsoon,whichisthefirstequationinaVARmodelforytandzt.Noticethatthisdependsonfiveparameters,justasmanyasintheerrorcor-rectionmodel.Thepointisthat,forthepurposesofforecasting,theVARmodelinthelevelsandtheerrorcorrectionmodelareessentiallythesame.Thisisnotthecaseinmoregeneralerrorcorrectionmodels.Forexample,supposethat110in(18.66),butwehaveaseconderrorcorrectionterm,2(yt2zt2).Then,theerrorcorrectionmodelinvolvesonlyfourparameters,whereas(18.67)—whichhasthesameorderoflagsforyandz—containsfiveparameters.Thus,errorcorrectionmodelscaneconomizeonparameters,thatis,theyaregenerallymoreparsimoniousthanVARsinlevels.IfytandztareI(1)butnotcointegrated,theappropriatemodelis(18.66)withouttheerrorcorrectionterm.Thiscanbeusedtoforecastyn1,andwecanaddthistoyntoforecastyn1.SUMMARYThetimeseriestopicscoveredinthischapterareusedroutinelyinempiricalmacro-economics,empiricalfinance,andavarietyofotherappliedfields.Webeganbyshow-inghowinfinitedistributedlagmodelscanbeinterpretedandestimated.Thesecanprovideflexiblelagdistributionswithfewerparametersthanasimilarfinitedistributedlagmodel.Thegeometricdistributedlagand,moregenerally,rationaldistributedlagmodels,arethemostpopular.Theycanbeestimatedusingstandardeconometricpro-ceduresonsimpledynamicequations.608\nd7/14/998:36PMPage609Chapter18AdvancedTimeSeriesTopicsTestingforaunitroothasbecomeverycommonintimeserieseconometrics.Ifaserieshasaunitroot,then,inmanycases,theusuallargesamplenormalapproxima-tionsarenolongervalid.Inaddition,aunitrootprocesshasthepropertythataninno-vationhasalong-lastingeffect,whichisofinterestinitsownright.Whiletherearemanytestsforunitroots,theDickey-Fullerttest—anditsextension,theaugmentedDickey-Fullertest—isprobablythemostpopularandeasiesttoimplement.WecanallowforalineartrendwhentestingforunitrootsbyaddingatrendtotheDickey-Fullerregression.WhenanI(1)series,yt,isregressedonanotherI(1)series,xt,thereisseriouscon-cernaboutspuriousregression,eveniftheseriesdonotcontainobvioustrends.Thishasbeenstudiedthoroughlyinthecaseofarandomwalk:evenifthetworandomwalksareindependent,theusualttestforsignificanceoftheslopecoefficient,basedontheusualcriticalvalues,willrejectmuchmorethanthenominalsizeofthetest.Inaddi-2tion,theRtendstoarandomvariable,ratherthantozero(aswouldbethecaseifweregressthedifferenceinytonthedifferenceinxt).Inoneimportantcase,aregressioninvolvingI(1)variablesisnotspurious,andthatiswhentheseriesarecointegrated.ThismeansthatalinearfunctionofthetwoI(1)variablesisI(0).IfytandxtareI(1)butytxtisI(0),ytandxtcannotdriftarbitrarilyfarapart.Therearesimpletestsofthenullofnocointegrationagainstthealternativeofcointegration,oneofwhichisbasedonapplyingaDickey-Fullerunitroottesttotheresidualsfromastaticregression.Therearealsosimpleestimatorsofthecointegratingparameterthatyieldtstatisticswithapproximatestandardnormaldistributions(andasymptoticallyvalidconfidenceintervals).WecoveredtheleadsandlagsestimatorinSection18.4.Cointegrationbetweenytandxtimpliesthaterrorcorrectiontermsmayappearinamodelrelatingyttoxt;theerrorcorrectiontermsarelagsinytxt,whereisthecointegratingparameter.Asimpletwo-stepestimationprocedureisavailableforesti-matingerrorcorrectionmodels.First,isestimatedusingastaticregression(ortheleadsandlagsregression).Then,OLSisusedtoestimateasimpledynamicmodelinfirstdifferenceswhichincludestheerrorcorrectionterms.Section18.5containedanintroductiontoforecasting,withemphasisonregression-basedforecastingmethods.Staticmodelsor,moregenerally,modelsthatcontainexplanatoryvariablesdatedcontemporaneouslywiththedependentvariable,arelim-itedbecausethentheexplanatoryvariablesneedtobeforecasted.Ifwepluginhypoth-esizedvaluesofunknownfutureexplanatoryvariables,weobtainaconditionalforecast.Unconditionalforecastsaresimilartosimplymodelingytasafunctionofpastinformationwehaveobservedatthetimetheforecastisneeded.Dynamicregressionmodels,includingautoregressionsandvectorautoregressions,areusedroutinely.Inadditiontoobtainingone-step-aheadpointforecasts,wealsodiscussedtheconstructionofforecastintervals,whichareverysimilartopredictionintervals.Variouscriteriaareusedforchoosingamongforecastingmethods.Themostcom-monperformancemeasuresaretherootmeansquarederrorandthemeanabsoluteerror.Bothestimatethesizeoftheaverageforecasterror.Itismostinformativetocom-putethesemeasuresusingout-of-sampleforecasts.Multi-step-aheadforecastspresentnewchallengesandaresubjecttolargeforecasterrorvariances.Nevertheless,formodelssuchasautoregressionsandvectorautore-609\nd7/14/998:36PMPage610Part3AdvancedTopicsgressions,multi-step-aheadforecastscanbecomputed,andapproximateforecastinter-valscanbeobtained.ForecastingtrendingandI(1)seriesrequiresspecialcare.Processeswithdetermin-istictrendscanbeforecastedbyincludingtimetrendsinregressionmodels,possiblywithlagsofvariables.Apotentialdrawbackisthatdeterministictrendscanprovidepoorforecastsforlong-horizonforecasts:onceitisestimated,alineartrendcontinuestoincreaseordecrease.ThetypicalapproachtoforecastinganI(1)processistofore-castthedifferenceintheprocessandtoaddthelevelofthevariabletothatforecasteddifference.Alternatively,vectorautoregressivemodelscanbeusedinthelevelsoftheseries.Iftheseriesarecointegrated,errorcorrectionmodelscanbeusedinstead.KEYTERMSAugmentedDickey-FullerTestLeadsandLagsEstimatorCointegrationLossFunctionConditionalForecastMartingaleDickey-FullerDistributionMartingaleDifferenceSequenceDickey-Fuller(DF)TestMeanAbsoluteError(MAE)Engle-GrangerTwo-StepProcedureMultiple-Step-AheadForecastErrorCorrectionModelOne-Step-AheadForecastExponentialSmoothingOut-of-SampleCriteriaForecastErrorPointForecastForecastIntervalRationalDistributedLag(RDL)ModelGeometric(orKoyck)DistributedLagRootMeanSquaredError(RMSE)GrangerCausalitySpuriousRegressionProblemIn-SampleCriteriaUnconditionalForecastInfiniteDistributedLag(IDL)ModelUnitRootsInformationSetVectorAutoregressive(VAR)ModelPROBLEMS18.1Considerequation(18.15)withk2.UsingtheIVapproachtoestimatingthehand,whatwouldyouuseasinstrumentsforyt1?18.2Aninterestingeconomicmodelthatleadstoaneconometricmodelwithalaggeddependentvariablerelatesyttotheexpectedvalueofxt,sayx*t,wheretheexpectationisbasedonallobservedinformationattimet1:yt01x*tut.(18.68)Anaturalassumptionon{ut}isthatE(utIt1)0,whereIt1denotesallinformationonyandxobservedattimet1;thismeansthatE(ytIt1)01x*t.Tocompletethismodel,weneedanassumptionabouthowtheexpectationx*tisformed.WesawasimpleexampleofadaptiveexpectationsinSection11.2,wherex*txt1.Amorecom-plicatedadaptiveexpectationsschemeis610\nd7/14/998:36PMPage611Chapter18AdvancedTimeSeriesTopicsx*tx*t1(xt1x*t1),(18.69)where01.Thisequationimpliesthatthechangeinexpectationsreactstowhetherlastperiod’srealizedvaluewasaboveorbelowitsexpectation.Theassump-tion01impliesthatthechangeinexpectationsisafractionoflastperiod’serror.(i)Showthatthetwoequationsimplythatyt0(1)yt11xt1ut(1)ut1.[Hint:Lagequation(18.68)oneperiod,multiplyitby(1),andsub-tractthisfrom(18.68).Then,use(18.69).](ii)UnderE(utIt1)0,{ut}isseriallyuncorrelated.Whatdoesthisimplyabouttheerrors,vtut(1)ut1?(iii)Ifwewritetheequationfrompart(i)asyt01yt12xt1vt,howwouldyouconsistentlyestimatethej?(iv)Givenconsistentestimatorsofthej,howwouldyouconsistentlyesti-mateand1?18.3Supposethat{yt}and{zt}areI(1)series,butytztisI(0)forsome0.Showthatforany,ytztmustbeI(1).18.4Considertheerrorcorrectionmodelinequation(18.37).Showthatifyouaddanotherlagoftheerrorcorrectionterm,yt2xt2,theequationsuffersfromperfectcollinearity.[Hint:Showthatyt2xt2isaperfectlinearfunctionofyt1xt1,xt1,andyt1.]18.5Supposetheprocess{(xt,yt):t0,1,2,…}satisfiestheequationsytxtutandxtxt1vt,whereE(utIt1)E(vtIt1)0,It1containsinformationonxandydatedattimet1andearlier,0,and1[sothatxt,andthereforeyt,isI(1)].Showthatthesetwoequationsimplyanerrorcorrectionmodeloftheformyt1xt1(yt1xt1)et,where1,1,andetutvt.(Hint:Firstsubtractyt1frombothsidesofthefirstequation.Then,addandsubtractxt1fromtheright-handsideandrearrange.Finally,usethesecondequationtogettheerrorcorrectionmodelthatcon-tainsxt1.)18.6UsingthemonthlydatainVOLAT.RAW,thefollowingmodelwasestimated:pciˆp(1.54)(.344)pcip1(.074)pcip2(.073)pcip3(.031)pcsp1pciˆp0(.56)(.042)pcip1(.045)pcip2(.042)pcip3(.013)pcsp122n554,R.174,R¯.168,611\nd7/14/998:36PMPage612Part3AdvancedTopicswherepcipisthepercentagechangeinmonthlyindustrialproduction,atanannualizedrate,andpcspisthepercentagechangeintheStandard&Poors500Index,alsoatanannualizedrate.(i)Ifthepastthreemonthsofpciparezero,andpcsp10,whatisthepredictedgrowthinindustrialproductionforthismonth?Isitstatisti-callydifferentfromzero?(ii)Ifthepastthreemonthsofpciparezero,butpcsp110,whatisthepredictedgrowthinindustrialproduction?(iii)Whatdoyouconcludeabouttheeffectsofthestockmarketonrealeco-nomicactivity?18.7LetgMtbetheannualgrowthinthemoneysupplyandletunemtbetheunem-ploymentrate.AssumingthatunemtfollowsastableAR(1)process,explainindetailhowyouwouldtestwhethergMGrangercausesunem.18.8Supposethatytfollowsthemodelyt1zt1ututut1etE(etIt1)0,whereIt1containsyandzdatedatt1andearlier.(i)ShowthatE(yt1It)(1)yt1zt1zt1.(Hint:Writeut1yt11zt2andplugthisintothesecondequation;then,plugtheresultintothefirstequationandtaketheconditionalexpecta-tion.)(ii)Supposethatyouusenobservationstoestimate,1,and.Writetheequationforforecastingyn1.(iii)ExplainwhythemodelwithonelagofzandAR(1)serialcorrelationisaspecialcaseofthemodelyt0yt11zt12zt2et.(iv)Whatdoespart(iii)suggestaboutusingmodelswithAR(1)serialcor-relationforforecasting?18.9Let{yt}beanI(1)sequence.Supposethatgˆnistheone-step-aheadforecastofyn1andletfˆngˆnynbetheone-step-aheadforecastofyn1.Explainwhythefore-casterrorsforforecastingyn1andyn1areidentical.COMPUTEREXERCISES18.10UsethedatainWAGEPRC.RAWforthisexercise.Problem11.5givesestimatesofafinitedistributedlagmodelofgpriceongwage,where12lagsofgwageareused.(i)EstimateasimplegeometricDLmodelofgpriceongwage.Inparticu-lar,estimateequation(18.11)byOLS.WhataretheestimatedimpactpropensityandLRP?Sketchtheestimatedlagdistribution.(ii)ComparetheestimatedIPandLRPtothoseobtainedinProblem11.5.Howdotheestimatedlagdistributionscompare?612\nd7/14/998:36PMPage613Chapter18AdvancedTimeSeriesTopics(iii)Now,estimatetherationaldistributedlagmodelfrom(18.16).SketchthelagdistributionandcomparetheestimatedIPandLRPtothoseobtainedinpart(ii).18.11UsethedatainHSEINV.RAWforthisexercise.(i)Testforaunitrootinlog(invpc),includingalineartimetrendandtwolagsoflog(incpct).Usea5%significancelevel.(ii)Usetheapproachfrompart(i)totestforaunitrootinlog(price).(iii)Giventheoutcomesinparts(i)and(ii),doesitmakesensetotestforcointegrationbetweenlog(invpc)andlog(price)?18.12UsethedatainVOLAT.RAWforthisexercise.(i)EstimateanAR(3)modelforpcip.Now,addafourthlagandverifythatitisveryinsignificant.(ii)TotheAR(3)modelfrompart(i),addthreelagsofpcsptotestwhetherpcspGrangercausespcip.Carefully,stateyourconclusion.(iii)Tothemodelinpart(ii),addthreelagsofthechangeini3,thethree-monthT-billrate.DoespcspGrangercausepcipconditionalonpasti3?218.13IntestingforcointegrationbetweengfrandpeinExample18.5,addttoequa-tion(18.32)toobtaintheOLSresiduals.IncludeonelagintheaugmentedDFtest.The5%criticalvalueforthetestis4.15.18.14UseINTQRT.RAWforthisexercise.(i)Estimatetheequationhy6thy3t10hy3t1hy3t11hy3t2etandreporttheresultsinequationform.TestH0:1againstatwo-sidedalternative.Assumethattheleadandlagaresufficientsothat{hy3t1}isstrictlyexogenousinthisequationanddonotworryaboutserialcorrelation.(ii)Totheerrorcorrectionmodelin(18.39),addhy3t2and(hy6t2hy3t3).Arethesetermsjointlysignificant?Whatdoyouconcludeabouttheappropriateerrorcorrectionmodel?18.15UsethedatainPHILLIPS.RAW,addingthe1997valuesforunemandinf:4.9and2.3,respectively.(i)Estimatethemodelsin(18.48)and(18.49)usingthedataupthrough1997.Dotheparameterestimateschangemuchcomparedwith(18.48)and(18.49)?(ii)Usethenewequationstoforecastunem1998;roundtotwoplacesafterthedecimal.UsetheEconomicReportofthePresident(1999orlater)toobtainunem1998.Whichequationproducesabetterforecast?(iii)Aswediscussedinthetext,theforecastforunem1998using(18.49)is4.90.Comparethiswiththeforecastobtainedusingthedatathrough1997.Doesusingtheextrayearofdatatoobtaintheparameteresti-matesproduceabetterforecast?613\nd7/14/998:36PMPage614Part3AdvancedTopics(iv)Usethemodelestimatedin(18.48)toobtainatwo-step-aheadforecastofunem.Thatis,forecastunem1998usingequation(18.55)withˆ1.572,ˆ.732,andh2.Isthisbetterorworsethantheone-step-aheadforecastobtainedbypluggingunem19974.9into(18.48)?18.16UsethedatainBARIUM.RAWforthisexercise.(i)Estimatethelineartrendmodelchnimpttut,usingthefirst119observations(thisexcludesthelasttwelvemonthsofobservationsfor1988).Whatisthestandarderroroftheregression?(ii)Now,estimateanAR(1)modelforchnimp,againusingalldatabutthelasttwelvemonths.Comparethestandarderroroftheregressionwiththatfrompart(i).Whichmodelprovidesabetterin-samplefit?(iii)Usethemodelsfromparts(i)and(ii)tocomputetheone-step-aheadforecasterrorsforthetwelvemonthsin1988.(Youshouldobtaintwelveforecasterrorsforeachmethod.)ComputeandcomparetheRMSEsandtheMAEsforthetwomethods.Whichforecastingmethodworksbetterout-of-sampleforone-step-aheadforecasts?(iv)Addmonthlydummyvariablestotheregressionfrompart(i).Arethesejointlysignificant?(Donotworryabouttheslightserialcorrelationintheerrorsfromthisregressionwhendoingthejointtest.)18.17UsethedatainFERTIL3.RAWforthisexercise.(i)Graphgfragainsttime.Doesitcontainaclearupwardordownwardtrendovertheentiresampleperiod?(ii)Usingthedataupthrough1979,estimateacubictimetrendmodelfor23gfr(thatis,regressgfront,t,andt,alongwithanintercept).CommentontheR-squaredoftheregression.(iii)Usingthemodelinpart(ii),computethemeanabsoluteerroroftheone-step-aheadforecasterrorsfortheyears1980through1984.(iv)Usingthedatathrough1979,regressgfrtonaconstantonly.Isthecon-stantstatisticallydifferentfromzero?Doesitmakesensetoassumethatanydrifttermiszero,ifweassumethatgfrtfollowsarandomwalk?(v)Now,forecastgfrfor1980through1984,usingarandomwalkmodel:theforecastofgfrn1issimplygfrn.FindtheMAE.Howdoesitcom-parewiththeMAEfrompart(iii)?Whichmethodofforecastingdoyouprefer?(vi)Now,estimateanAR(2)modelforgfr,againusingthedataonlythrough1979.Isthesecondlagsignificant?(vii)ObtaintheMAEfor1980through1984,usingtheAR(2)model.Doesthismoregeneralmodelworkbetterout-of-samplethantherandomwalkmodel?18.18UseCONSUMP.RAWforthisexercise.(i)Letytberealpercapitadisposableincome.Usethedataupthrough1989toestimatethemodelyttyt1utandreporttheresultsintheusualform.614\nd7/14/998:36PMPage615Chapter18AdvancedTimeSeriesTopics(ii)Usetheestimatedequationfrompart(i)toforecastyin1990.Whatistheforecasterror?(iii)Computethemeanabsoluteerroroftheone-step-aheadforecastsforthe1990s,usingtheparametersestimatedinpart(i).(iv)Now,computetheMAEoverthesameperiod,butdropyt1fromtheequation.Isitbettertoincludeyt1inthemodelornot?18.19UsethedatainINTQRT.RAWforthisexercise.(i)Usingthedatafromallbutthelastfouryears(16quarters),estimateanAR(1)modelforr6t.(Weusethedifferencebecauseitappearsthatr6thasaunitroot.)FindtheRMSEoftheone-step-aheadforecastsforr6,usingthelast16quarters.(ii)Now,addtheerrorcorrectiontermsprt1r6t1r3t1totheequa-tionfrompart(i).(Thisassumesthatthecointegratingparameterisone.)ComputetheRMSEforthelast16quarters.Doestheerrorcor-rectiontermhelpwithout-of-sampleforecastinginthiscase?(iii)Now,estimatethecointegratingparameter,ratherthansettingittoone.Usethelast16quartersagaintoproducetheout-of-sampleRMSE.Howdoesthiscomparewiththeforecastsfromparts(i)and(ii)?(iv)Wouldyourconclusionschangeifyouwantedtopredictr6ratherthanr6?Explain.615\n\nd7/14/998:42PMPage616ChapterNineteenCarryingoutanEmpiricalProjectnthischapter,wediscusstheingredientsofasuccessfulempiricalanalysis,withIemphasisoncompletingatermproject.Inadditiontoremindingyouoftheimpor-tantissuesthathavearisenthroughoutthetext,weemphasizerecurringthemesthatareimportantforappliedresearch.Wealsoprovidesuggestionsfortopicsasawayofstimulatingyourimagination.Severalsourcesofeconomicresearchanddataaregivenasreferences.19.1POSINGAQUESTIONTheimportanceofposingaveryspecificquestioncannotbeoverstated.Withoutbeingexplicitaboutthegoalofyouranalysis,youcannotknowwheretoevenbegin.Thewidespreadavailabilityofrichdatasetsmakesittemptingtolaunchintodatacollectionbasedonhalf-bakedideas,butthisisoftencounterproductive.Itislikelythat,withoutcarefullyformulatingyourhypothesesandthekindofmodelyouwillneedtoestimate,youwillforgettocollectinformationonimportantvariables,obtainasamplefromthewrongpopulation,orcollectdataforthewrongtimeperiod.Thisdoesnotmeanthatyoushouldposeyourquestioninavacuum.Especiallyforaone-termproject,youcannotbetooambitious.Therefore,whenchoosingatopic,youshouldbereasonablysurethatdatasourcesexistthatwillallowyoutoansweryourquestionintheallottedtime.Youneedtodecidewhatareasofeconomicsorothersocialsciencesinterestyouwhenselectingatopic.Forexample,ifyouhavetakenacourseinlaboreconomics,youhaveprobablyseentheoriesthatcanbetestedempiricallyorrelationshipsthathavesomepolicyrelevance.Laboreconomistsareconstantlycomingupwithnewvariablesthatcanexplainwagedifferentials.Examplesincludequalityofhighschool[CardandKrueger(1992)andBetts(1995)],amountofmathandsciencetakeninhighschool[LevineandZimmerman(1995)],andphysicalappearance[HamermeshandBiddle(1994),AverettandKorenman(1996),andBiddleandHamermesh(1998)].Researchersinstateandlocalpublicfinancestudyhowlocaleconomicactivitydependsoneconomicpolicyvariables,suchaspropertytaxes,salestaxes,levelandqualityofservices(suchasschools,fire,andpolice),andsoon.[See,forexample,White(1986),Papke(1987),Bartik(1991),andNetzer(1992).]616\nd7/14/998:42PMPage617Chapter19CarryingoutanEmpiricalProjectEconomiststhatstudyeducationissuesareinterestedinhowspendingaffectsper-formance[Hanushek(1986)],whetherattendingcertainkindsofschoolsimprovesper-formance[forexample,EvansandSchwab(1995)],andindeterminingfactorsthataffectwhereprivateschoolschoosetolocate[DownesandGreenstein(1996)].Macroeconomistsareinterestedinrelationshipsbetweenvariousaggregatetimeseries,suchasthelinkbetweengrowthingrossdomesticproductandgrowthinfixedinvestmentormachinery[seeDeLongandSummers(1991)]ortheeffectoftaxesoninterestrates[forexample,Peek(1982)].Therearecertainlyreasonsforestimatingmodelsthataremostlydescriptive.Forexample,propertytaxassessorsusemodels(calledhedonicpricemodels—seeExample4.8)toestimatehousingvaluesforhomesthathavenotbeensoldrecently.Thisinvolvesaregressionmodelrelatingthepriceofahousetoitscharacteristics(size,numberofbedrooms,numberofbathrooms,andsoon).Asatopicforatermpaper,thisisnotveryexciting:weareunlikelytolearnmuchthatissurprising,andsuchananaly-sishasnoobviouspolicyimplications.Addingthecrimerateintheneighborhoodasanexplanatoryvariablewouldallowustodeterminehowimportantafactorcrimeisonhousingprices,somethingthatwouldbeusefulinestimatingthecostsofcrime.Severalrelationshipshavebeenestimatedusingmacroeconomicdatathataremostlydescriptive.Forexample,anaggregatesavingfunctioncanbeusedtoestimatetheaggregatemarginalpropensitytosave,aswellastheresponseofsavingtoassetreturns(suchasinterestrates).Suchananalysiscouldbemademoreinterestingbyusingtimeseriesdataonacountrythathasahistoryofpoliticalupheavalsanddeter-miningwhethersavingsratesdeclineduringtimesofpoliticaluncertainty.Onceyoudecideonanareaofresearch,thereareavarietyofwaystolocatespe-cificpapersonthetopic.TheJournalofEconomicLiterature(JEL)hasadetailedclas-sificationsystemsothateachpaperisgivenasetofidentifyingcodesthatplacesitwithincertainsubfieldsofeconomics.TheJELalsocontainsalistofarticlespublishedinawidevarietyofjournals,organizedbytopic,anditevencontainsshortabstractsofsomearticles.EspeciallyconvenientforfindingpublishedpapersonvarioustopicsareInternetservices,suchasEconLit,whichissubscribedtobymanyuniversities.EconLitallowsuserstodoacomprehensivesearchofalmostalleconomicsjournalsbyauthor,subject,wordsinthetitle,andsoon.TheSocialScienceCitationIndexisusefulforfindingpapersonabroadrangeoftopicsinthesocialsciences,includingpopularpapersthathavebeencitedofteninotherpublishedworks.Inthinkingaboutatopic,therearesomethingstokeepinmind.First,foraques-tiontobeinteresting,itdoesnotneedtohavebroad-basedpolicyimplications;rather,itcanbeoflocalinterest.Forexample,youmightbeinterestedinknowingwhetherliv-inginafraternityatyouruniversitycausesstudentstohavelowerorhighergradepointaverages.Thismayormaynotbeofinteresttopeopleoutsideofyouruniversity,butitisprobablyofconcerntoatleastsomepeoplewithintheuniversity.Ontheotherhand,youmightstudyaproblemthatstartsoutbeingoflocalinterestbutturnsouttohavewidespreadinterest,suchasdeterminingwhichfactorsaffect,andwhichuniversitypoliciescanstem,alcoholabuseoncollegecampuses.Second,itisverydifficult,especiallyforaquarterorsemesterproject,todotrulyoriginalresearchusingthestandardmacroeconomicaggregatesontheU.S.economy.617\nd7/14/998:42PMPage618Part3AdvancedTopicsForexample,thequestionofwhethermoneygrowth,governmentspendinggrowth,andsoon,affecteconomicgrowthhasbeenandcontinuestobestudiedbyprofessionalmacroeconomists.Thequestionofwhetherstockorotherassetreturnscanbesystem-aticallypredictedusingknowninformationhas,forobviousreasons,beenstudiedprettycarefully.Thisdoesnotmeanthatyoushouldavoidestimatingmacroeconomicorempiricalfinancemodels,asevenjustusingmorerecentdatacanaddconstructivelytoadebate.Inaddition,youcansometimesfindanewvariablethathasanimportanteffectoneconomicaggregatesorfinancialreturns;suchadiscoverycanbeexciting.Thepointisthatexercisessuchasusingafewadditionalyearstoestimateastan-dardPhillipscurveoranaggregateconsumptionfunctionfortheU.S.economy,orsomeotherlargeeconomy,areunlikelytoyieldadditionalinsights,althoughtheycanbeinstructiveforthestudent.Instead,youmightusedataonasmallercountrytoesti-mateastaticordynamicPhillipscurve,ortotesttheefficientmarketshypothesis,andsoon.Atthenonmacroeconomiclevel,therearealsoplentyofquestionsthathavebeenstudiedextensively.Forexample,laboreconomistshavepublishedmanypapersonesti-matingthereturntoeducation.Thisquestionisstillstudiedbecauseitisveryimpor-tant,andnewdatasets,aswellasneweconometricapproaches,continuetobedeveloped.Forexample,aswesawinChapter9,certaindatasetshavebetterproxyvariablesforunobservedabilitythanotherdatasets.(CompareWAGE1.RAWandWAGE2.RAW.)Inothercases,wecanobtainpaneldataordatafromanaturalexperi-ment—seeChapter13—whichallowustoapproachanoldquestionfromadifferentperspective.Asanotherexample,criminologistsareinterestedinstudyingtheeffectsofvariouslawsoncrimes.Thequestionofwhethercapitalpunishmenthasadeterrenteffecthaslongbeendebated.Similarly,economistshavebeeninterestedinwhethertaxesoncig-arettesandalcoholreduceconsumption(asalways,inaceterisparibussense).Asmoreyearsofdataatthestatelevelbecomeavailable,aricherpaneldatasetcanbecreated,andthiscanhelpusbetteranswermajorpolicyquestions.Plus,therearefairlyrecentcrime-fightinginnovations—suchastheadventofcommunitypolicing—whoseeffec-tivenesscanbeevaluatedempiricially.Whileyouareformulatingyourquestion,itishelpfultodiscussyourideaswithyourclassmates,instructor,andfriends.Youshouldbeabletoconvincepeoplethattheanswertoyourquestionisofsomeinterest.(Ofcourse,whetheryoucanpersuasivelyansweryourquestionisanotherissue,butyouneedtobeginwithaninterestingques-tion.)Ifsomeoneasksyouaboutyourpaperandyourespondwith“I’mdoingmypaperoncrime”or“I’mdoingmypaperoninterestrates,”chancesareyouhaveonlydecidedonageneralareawithoutformulatingatruequestion.Youshouldbeabletosaysome-thinglike“I’mstudyingtheeffectsofcommunitypolicingoncitycrimeratesintheUnitedStates”or“I’mlookingathowinflationvolatilityaffectsshort-terminterestratesinBrazil.”19.2LITERATUREREVIEWAllpapers,eveniftheyarerelativelyshort,shouldcontainareviewofrelevantlitera-ture.Itisrarethatoneattemptsanempiricalprojectwherethereisnotsomepublished618\nd7/14/998:42PMPage619Chapter19CarryingoutanEmpiricalProjectprecedent.Ifyousearchthroughjournalsoruseon-linesearchservicessuchasEconLittocomeupwithatopic,youarealreadywellonyourwaytoaliteraturereview.Ifyouselectatopiconyourown—suchasstudyingtheeffectsofdrugusageoncol-legeperformanceatyouruniversity—thenyouwillprobablyhavetoworkalittleharder.Buton-linesearchservicesmakethatworkaloteasier,asyoucansearchbykeywords,bywordsinthetitle,byauthor,andsoon.Youcanthenreadabstractsofpaperstoseehowrelevanttheyaretoyourownwork.Whendoingyourliteraturesearch,youshouldthinkofrelatedtopicsthatmightnotshowupinasearchusingahandfulofkeywords.Forexample,ifyouarestudyingtheeffectsofdrugusageonwagesorgradepointaverage,youshouldprobablylookattheliteratureonhowalcoholusageaffectssuchfactors.Knowinghowtodoathoroughliter-aturesearchisanacquiredskill,butyoucangetalongwaybythinkingbeforesearching.Researchersdifferonhowaliteraturereviewshouldbeincorporatedintoapaper.Someliketohaveaseparatesectioncalled“literaturereview,”whileothersliketoincludetheliteraturereviewaspartoftheintroduction.Thisislargelyamatteroftaste,althoughanextensiveliteraturereviewprobablydeservesitsownsection.Ifthetermpaperisthefocusofthecourse—say,inaseniorseminaroranadvancedeconometricscourse—yourliteraturereviewprobablywillbelengthy.Termpapersattheendofafirstcoursearetypicallyshorter,andtheliteraturereviewsarebriefer.19.3DATACOLLECTIONDecidingontheAppropriateDataSetCollectingdataforatermpapercanbeeducational,exciting,andsometimesevenfrus-trating.Youmustfirstdecideonthekindofdataneededtoansweryourposedquestion.Aswediscussedintheintroductionandhavecoveredthroughoutthistext,datasetscomeinavarietyofforms.Themostcommonkindsarecross-sectional,timeseries,pooledcrosssections,andpaneldatasets.Manyquestionscanbeaddressedusinganyofthedatastructureswehavedescribed.Forexample,tostudywhethermorelawenforcementlowerscrime,wecoulduseacrosssectionofcities,atimeseriesforagivencity,orapaneldatasetofcities—whichconsistsofdataonthesamecitiesovertwoormoreyears.Decidingonwhichkindofdatatocollectoftendependsonthenatureoftheanaly-sis.Toanswerquestionsattheindividualorfamilylevel,weoftenonlyhaveaccesstoasinglecrosssection;typically,theseareobtainedviasurveys.Then,wemustaskwhetherwecanobtainarichenoughdatasettodoaconvincingceterisparibusanaly-sis.Forexample,supposewewanttoknowwhetherfamilieswhosavethroughindi-vidualretirementaccounts(IRAs)—whichhavecertaintaxadvantages—havelessnon-IRAsavings.Inotherwords,doesIRAsavingsimplycrowdoutotherformsofsaving?Therearedatasets,suchastheSurveyofConsumerFinances,whichcontaininformationonvariouskindsofsavingforadifferentsampleoffamilieseachyear.Thereareseveralissuesthatariseinusingsuchadataset.Perhapsthemostimportantiswhetherthereareenoughcontrols—includingincome,demographics,andproxiesforsavingtastes—todoareasonableceterisparibusanalysis.Ifthesearetheonlykindsofdataavailable,wemustdowhatwecanwiththem.619\nd7/14/998:42PMPage620Part3AdvancedTopicsThesameissuesarisewithcross-sectionaldataonfirms,cities,states,andsoon.Inmostcases,itisnotobviousthatwewillbeabletodoaceterisparibusanalysiswithasinglecrosssection.Forexample,anystudyofthetheeffectsoflawenforcementoncrimemustrecognizetheendogeneityoflawenforcementexpenditures.Whenusingstandardregressionmethods,itmaybeveryhardtocompleteaconvincingceterisparibusanalysis,nomatterhowmanycontrolswehave.(SeeSection19.4formoredis-cussion.)Ifyouhavereadtheadvancedchaptersonpaneldatamethods,youknowthathav-ingthesamecross-sectionalunitsattwoormoredifferentpointsintimecanallowustocontrolfortime-constantunobservedeffectsthatwouldnormallyconfoundregres-siononasinglecrosssection.Paneldatasetsarerelativelyhardtoobtainforindividu-alsorfamilies—althoughsomeimportantonesexist,suchasthePanelStudyofIncomeDynamics—buttheycanbeusedinveryconvincingways.Paneldatasetsonfirmsalsoexist.Forexample,CompuStatandtheCenterforResearchonSecuritiesPrices(CRSP)manageverylargepaneldatasetsoffinancialinformationonfirms.Easiertoobtainarepaneldatasetsonlargerunits,suchasschools,cities,counties,andstates,asthesetendnottodisappearovertime,andgovernmentagenciesareresponsibleforcol-lectinginformationonthesamevariableseachyear.Forexample,theFederalBureauofInvestigationcollectsandreportsdetailedinformationoncrimeratesatthecitylevel.Sourcesofdataarelistedinthechapterappendix.Datacomeinavarietyofforms.Somedatasets,especiallyhistoricalones,areavailableonlyinprintedform.Forsmalldatasets,enteringthedatayourselffromtheprintedsourceismanageableandconvenient.Sometimes,articlesarepublishedwithsmalldatasets—especiallytimeseriesapplications.Thesecanbeusedinanempiricalstudy,perhapsbysupplementingthedatawithmorerecentyears.Manydatasetsareavailableoncomputerdiskettesormagnetictapes.Theformerareespeciallyeasytoworkwith.Currently,verylargedatasetscanbeputonsmalldiskettes.Variousgovernmentagenciesselldatadiskettes,asdoprivatefirms.Authorsofpapersareoftenwillingtoprovidetheirdatasetsindisketteform.Moreandmoredatasetsareavailableontheworldwideweb.Thewebisavastresourceofon-linedatabases.Numerouswebsitescontainingeconomicandrelateddatasetshaverecentlybeencreated.Severalotherwebsitescontainlinkstodatasetsthatareofinteresttoeconomists;someofthesearelistedinthechapterappendix.Generally,searchingtheInternetfordatasourcesisfairlyeasyandwillbecomeevenmoreconvenientinthefuture.EnteringandStoringYourDataOnceyouhavedecidedonadatatypeandhavelocatedadatasource,youmustputthedataintousableform.Ifthedatacameondiskette,theyarealreadyinsomeform,hope-fullyoneinwidespreaduse.Themostflexiblewaytoobtaindataindisketteformisasastandardtext(ASCII)file.Allstatisticsandeconometricssoftwarepackagesallowrawdatatobestoredthisway.Typically,itisstraightforwardtoreadatextfiledirectlyintoaneconometricspackage,providedthefileisproperlystructured.Thedatafileswehaveusedthroughoutthetextprovideseveralexamplesofhowcross-sectional,timeseries,pooledcrosssections,andpaneldatasetsareusuallystored.Asageneralrule,620\nd7/14/998:42PMPage621Chapter19CarryingoutanEmpiricalProjectthedatashouldhaveatabularform,witheachobservationrepresentingadifferentrow;thecolumnsinthedatasetrepresentdifferentvariables.Occasionally,youmightencounteradatasetstoredwitheachcolumnrepresentinganobservationandeachrowadifferentvariable.Thisisnotideal,butmostsoftwarepackagesallowdatatobereadinthisform,andthenreshaped.Naturally,itiscrucialtoknowhowthedataareorga-nizedbeforereadingthemintoyoureconometricspackage.Fortimeseriesdatasets,thereisonlyonesensiblewaytoenterandstorethedata:namely,chronologically,withtheearliesttimeperiodlistedasthefirstobservationandthemostrecenttimeperiodasthelastobservation.Itisoftenusefultoincludevariablesindicatingyearand,ifrelevant,quarterormonth.Thisfacilitatesestimationofavari-etyofmodelslateron,includingallowingforseasonalityandbreaksatdifferenttimeperiods.Forcrosssectionspooledovertime,itisusuallybesttohavethecrosssectionfortheearliestyearfillthefirstblockofobservations,followedbythecrosssectionforthesecondyear,andsoon.(SeeFERTIL1.RAWasanexample.)Thisarrangementisnotcrucial,butitisveryimportanttohaveavariablestatingtheyearattachedtoeachobservation.Forpaneldata,aswediscussedinSection13.5,itisbestifalltheyearsforeachcross-sectionalobservationareadjacentandinchronologicalorder.WiththisorderingwecanuseallofthepaneldatamethodsfromChapters13and14.Withpaneldata,itisimportanttoincludeauniqueidentifierforeachcross-sectionalunit,alongwithayearvariable.Ifyouobtainyourdatainprintedform,youhaveseveraloptionsforenteringitintoacomputer.First,youcancreateatextfileusingastandardtexteditor.(Thisishowseveraloftherawdatasetsincludedwiththetextwereinitiallycreated.)Typically,itisrequiredthateachrowstartsanewobservation,thateachrowcontainsthesameorder-ingofthevariables—inparticular,eachrowshouldhavethesamenumberofentries—andthatthevaluesareseparatedbyatleastonespace.Sometimes,adifferentseparator,suchasacomma,isbetter,butthisdependsonthesoftwareyouareusing.Ifyouhavemissingobservationsonsomevariables,youmustdecideonhowtodenotethat;sim-plyleavingablankdoesnotgenerallywork.Manyregressionpackagesacceptaperiodasthemissingvaluesymbol.Somepeopleprefertouseanumber—presumablyanimpossiblevalueforthevariableofinterest—todenotemissingvalues.Ifyouarenotcareful,thiscanbedangerous;wediscussthisfurtherlater.Ifyouhavenonnumericaldata—forexample,youwanttoincludethenamesinasampleofcollegesorthenamesofcities—thenyoushouldchecktheeconometricspackageyouwillusetoseethebestwaytoentersuchvariables(oftencalledstrings).Typically,stringsareputbetweendoubleorsinglequotations.Or,thetextfilecanfol-lowarigidformatting,whichusuallyrequiresasmallprogramtoreadinthetextfile.Butyouneedtocheckyoureconometricspackagefordetails.Anothergenerallyavailableoptionistouseaspreadsheettoenteryourdata,suchasExcel.Thishasacoupleofadvantagesoveratextfile.First,becauseeachobserva-tiononeachvariableisacell,itislesslikelythatnumberswillberuntogether(aswouldhappenifyouforgettoenteraspaceinatextfile).Secondly,spreadsheetsallowmanipulationofdata,suchassorting,computingaverages,andsoon.Thissecondben-efitislessimportantifyouuseasoftwarepackagethatallowsforsophisticateddatamanagement;manysoftwarepackages,includingEviewsandStata,fallintothiscate-621\nd7/14/998:42PMPage622Part3AdvancedTopicsgory.Ifyouuseaspreadsheetforinitialdataentry,thenyoumustoftenexportthedatainaformthatcanbereadbyyoureconometricspackage.Thisisusuallystraightfor-ward,asspreadsheetsexporttotextfilesusingavarietyofformats.Athirdalternativeistoenterthedatadirectlyintoyoureconometricspackage.Whilethisobviatestheneedforatexteditororaspreadsheet,itismoreawkwardbecauseyoucannotfreelymoveacrossdifferentobservationstomakecorrectionsoradditions.DatadownloadedfromtheInternetmaycomeinavarietyofforms.Oftendatacomeastextfiles,butdifferentconventionsareusedforseparatingvariables;forpaneldatasets,theconventionsonhowtoorderthedatamaydiffer.SomeInternetdatasetscomeasspreadsheetfiles,inwhichcaseyoumustuseanappropriatespreadsheettoreadthem.Inspecting,Cleaning,andSummarizingYourDataItisextremelyimportanttobecomefamiliarwithanydatasetyouwilluseinanempir-icalanalysis.Ifyouenterthedatayourself,youwillbeforcedtoknoweverythingaboutit.Butifyouobtaindatafromanoutsidesource,youshouldstillspendsometimeunderstandingitsstructureandconventions.Evendatasetsthatarewidelyusedandheavilydocumentedcancontainglitches.Ifyouareusingadatasetobtainedfromtheauthorofapaper,youmustbeawarethatmethodsofdatasetconstructioncanbefor-gotten.Earlier,wereviewedthestandardwaysthatvariousdatasetsarestored.Youalsoneedtoknowhowmissingvaluesarecoded.Preferably,missingvaluesareindicatedwithanonnumericcharacter,suchasaperiod.Ifanumberisusedasamissingvaluecode,suchas“999”or“1”,youmustbeverycarefulwhenusingtheseobservationsincomputinganystatistics.Youreconometricspackagewillprobablynotknowthatacertainnumberreallyrepresentsamissingvalue:itislikelythatsuchobservationswillbeusedasiftheyarevalid,andthiscanproducerathermisleadingresults.Thebestapproachistosetanynumericalcodesformissingvaluestosomeothercharacter(suchasaperiod)thatcannotbemistakenforrealdata.Youmustalsoknowthenatureofthevariablesinthedataset.Whicharebinaryvariables?Whichareordinalvariables(suchasacreditrating)?Whataretheunitsofmeasurementofthevariables?Forexample,aremonetaryvaluesexpressedindollars,thousandsofdollars,millionsofdollars,orsoon?Arevariablesrepresentingarate—suchasschooldropoutrates,inflationrates,unionizationrates,orinterestrates—measuredasapercentoraproportion?Especiallyfortimeseriesdata,itiscrucialtoknowifmonetaryvaluesareinnom-inal(current)orreal(constant)dollars.Ifthevaluesareinrealterms,whatisthebaseyearorperiod?Ifyoureceiveadatasetfromanauthor,somevariablesmayalreadybetransformedincertainways.Forexample,sometimesonlythelogofavariable(suchaswageorsalary)isreportedinthedataset.Detectingmistakesinadatasetisnecessaryforpreservingtheintegrityofanydataanalysis.Itisalwaysusefultofindminimums,maximums,means,andstandarddevia-tionsofall,oratleastthemostsignificant,variablesintheanalysis.Forexample,ifyou622\nd7/14/998:42PMPage623Chapter19CarryingoutanEmpiricalProjectfindthattheminimumvalueofeducationinyoursampleis99,youknowthatatleastoneentryoneducationneedstobesettoamissingvalue.If,uponfurtherinspection,youfindthatseveralobservationshave99asthelevelofeducation,youcanbecon-fidentthatyouhavestumbledontothemissingvaluecodeforeducation.Asanotherexample,ifyoufindthatanaveragemurderconvictionrateacrossasampleofcitiesis.632,youknowthatconvictionrateismeasuredasaproportion,notapercent.Then,ifthemaximumvalueisaboveone,thisislikelyatypographicalerror.(Itisnotuncom-montofinddatasetswheremostoftheentriesonaratevariablewereenteredasaper-cent,butwheresomewereenteredasaproportion,andviceversa.Suchdatacodingerrorscanbedifficulttodetect,butitisimportanttotry.)Wemustalsobecarefulinusingtimeseriesdata.Ifweareusingmonthlyorquar-terlydata,wemustknowwhichvariables,ifany,havebeenseasonallyadjusted.Transformingdataalsorequiresgreatcare.Supposewehaveamonthlydatasetandwewanttocreatethechangeinavariablefromonemonthtothenext.Todothis,wemustbesurethatthedataareorderedchronologically,fromearliestperiodtolatest.Ifforsomereasonthisisnotthecase,thedifferencingwillresultingarbage.Tobesurethedataareproperlyordered,itisusefultohaveatimeperiodindicator.Withannualdata,itissufficienttoknowtheyear,butthenweshouldknowwhethertheyearisenteredasfourdigitsortwodigits(forexample,1998versus98).Withmonthlyorquarterlydata,itisalsousefultohaveavariableorvariablesindicatingmonthorquarter.Withmonthlydata,wemayhaveasetofdummyvariables(11or12)oronevariableindicatingthemonth(1through12orastringvariable,suchasjan,feb,andsoon).Withorwithoutyearly,monthly,orquarterlyindicators,wecaneasilyconstructtimetrendsinalleconometricssoftwarepackages.Creatingseasonaldummyvariablesiseasyifthemonthorquarterisindicated;ataminimum,weneedtoknowthemonthorquarterofthefirstobservation.Manipulatingpaneldatacanbeevenmorechallenging.InChapter13,wediscussedpooledOLSonthedifferenceddataasonegeneralapproachtocontrollingforunob-servedeffects.Inconstructingthedifferenceddata,wemustbecarefulnottocreatephantomobservations.Supposewehaveabalancedpaneloncitiesfrom1992through1997.Evenifthedataareorderedchronologicallywithineachcross-sectionalunit—somethingthatshouldbedonebeforeproceeding—amindlessdifferencingwillcreateanobservationfor1992forallcitiesexceptthefirstinthesample.Thisobservationwillbethe1992valueforcityi,minusthe1997valueforcityi1;thisisclearlynonsense.Thus,wemustmakesurethat1992ismissingforalldifferencedvariables.Withanunbalancedpanel,thingsbecomemuchtrickierbecausenosinglecom-mandworksforallcross-sectionalunits.Itisusuallyeasiertousefixedeffectsestima-tiononunbalancedpanels.19.4ECONOMETRICANALYSISThistexthasfocusedoneconometricanalysis,andwearenotabouttoprovideareviewofeconometricmethodsinthissection.Nevertheless,wecangivesomegeneralguide-linesaboutthesortsofissuesthatneedtobeconsideredinanempiricalanalysis.623\nd7/14/998:42PMPage624Part3AdvancedTopicsAswediscussedearlier,afterdecidingonatopic,wemustcollectanappropriatedataset.Assumingthatthishasalsobeendone,wemustnextdecideontheappropri-ateeconometricmethods.Ifyourcoursehasfocusedonordinaryleastsquaresestimationofamultiplelinearregressionmodel,usingeithercross-sectionalortimeseriesdata,theeconometricapproachhasprettymuchbeendecidedforyou.Thisisnotnecessarilyaweakness,asOLSisstillthemostwidelyusedeconometricmethod.Ofcourse,youstillhavetodecidewhetheranyofthevariantsofOLS—suchasweightedleastsquaresorcorrect-ingforserialcorrelationinatimeseriesregression—arerequired.InordertojustifyOLS,youmustalsomakeaconvincingcasethatthekeyOLSassumptionsaresatisfiedforyourmodel.Aswehavediscussedatsomelength,thefirstissueiswhethertheerrortermisuncorrelatedwiththeexplanatoryvariables.Ideally,youhavebeenabletocontrolforenoughotherfactorstoassumethatthosethatareleftintheerrorareunrelatedtotheregressors.Especiallywhendealingwithindividual,family,orfirm-levelcross-sectionaldata,theself-selectionproblem—whichwedis-cussedinChapters7and15—isoftenrelevant.Forinstance,intheIRAexamplefromSection19.3,itmaybethatfamilieswithunobservedtasteforsavingarealsotheonesthatopenIRAs.Youshouldalsobeabletoarguethattheotherpotentialsourcesofendogeneity—namely,measurementerrorandsimultaneity—arenotaseriousproblem.Whenspecifyingyourmodelyoumustalsomakefunctionalformdecisions.Shouldsomevariablesappearinlogarithmicform?(Ineconometricapplications,theanswerisoftenyes.)Shouldsomevariablesbeincludedinlevelsandsquares,topossiblycaptureadiminishingeffect?Howshouldqualitativefactorsappear?Isitenoughtojustincludebinaryvariablesfordifferentattributesorgroups?Or,dotheseneedtobeinteractedwithquantitativevariables?(SeeChapter7fordetails.)Forcross-sectionalanalysis,asecondary,butneverthelessimportantissue,iswhetherthereisheteroskedasticity.InChapter8,weexplainedhowthiscanbedealtwith.Thesimplestwayistocomputeheteroskedasticity-robuststatistics.AsweemphasizedinChapters10,11,and12,timeseriesapplicationsrequireaddi-tionalcare.Shouldtheequationbeestimatedinlevels?Iflevelsareused,aretimetrendsneeded?Isdifferencingthedatamoreappropriate?Ifthedataaremonthlyorquarterly,doesseasonalityhavetobeaccountedfor?Ifyouareallowingfordynamics—forexample,distributedlagdynamics—howmanylagsshouldbeincluded?Youmuststartwithsomelagsbasedonintuitionorcommonsense,buteventuallyitisanempir-icalmatter.Ifyourmodelhassomepotentialmisspecification,suchasomittedvariables,andyouuseOLS,youshouldattemptsomesortofmisspecificationanalysisofthekindswediscussedinChapters3and5.Canyoudetermine,basedonreasonableassump-tions,thedirectionofanybiasintheestimators?Ifyouhavestudiedthemethodofinstrumentalvariables,youknowthatitcanbeusedtosolvevariousformsofendogeneity,includingomittedvariables(Chapter15),errors-in-variables(Chapter15),andsimultaneity(Chapter16).Naturally,youneedtothinkhardaboutwhethertheinstrumentalvariablesyouareconsideringarelikelytobevalid.Goodpapersintheempiricalsocialsciencescontainsensitivityanalysis.Broadly,thismeansyouestimateyouroriginalmodelandmodifyitinwaysthatseemreason-624\nd7/14/998:42PMPage625Chapter19CarryingoutanEmpiricalProjectable.Hopefully,theimportantconclusionsdonotchange.Forexample,ifyouuseasanexplanatoryvariableameasureofalcoholconsumption(say,inagradepointaverageequation),doyougetqualitativelysimilarresultsifyoureplacethequantitativemea-surewithadummyvariableindicatingalcoholusage?Ifthebinaryusagevariableissignificantbutthealcoholquantityvariableisnot,itcouldbethatusagereflectssomeunobservedattributethataffectsGPAandisalsocorrelatedwithalcoholusage.Butthisneedstobeconsideredonacase-by-casebasis.Ifsomeobservationsaremuchdifferentfromthebulkofthesample—say,youhaveafewfirmsinasamplethataremuchlargerthantheotherfirms—doyourresultschangemuchwhenthoseobservationsareexcludedfromtheestimation?Ifso,youmayhavetoalterfunctionalformstoallowfortheseobservationsorarguethattheyfollowacompletelydifferentmodel.TheissueofoutlierswasdiscussedinChapter9.Usingpaneldataraisessomeadditionaleconometricissues.Supposeyouhavecol-lectedtwoperiods.Thereareatleastfourwaystousetwoperiodsofpaneldatawith-outresortingtoinstrumentalvariables.YoucanpoolthetwoyearsinastandardOLSanalysis,asdiscussedinChapter13.Whilethismightincreasethesamplesizerelativetoasinglecrosssection,itdoesnotcontrolfortime-constantunobservables.Inaddi-tion,theerrorsinsuchanequationarealmostalwaysseriallycorrelatedbecauseofanunobservedeffect.Randomeffectsestimationcorrectstheserialcorrelationproblemandproducesasymptoticallyefficientestimators,providedtheunobservedeffecthaszeromeangivenvaluesoftheexplanatoryvariablesinalltimeperiods.Anotherpossibilityistoincludealaggeddependentvariableintheequationforthesecondyear.InChapter9,wepresentedthisasawaytoatleastmitigatetheomittedvariablesproblem,asweareinanyeventholdingfixedtheinitialoutcomeofthedepen-dentvariable.Thisoftenleadstosimilarresultsasdifferencingthedata,aswecoveredinChapter13.Withmoreyearsofpaneldata,wehavethesameoptions,plusanadditionalchoice.Wecanusethefixedeffectstransformationtoeliminatetheunobservedeffect.(Withtwoyearsofdata,thisisthesameasdifferencing.)InChapter15,weshowedhowinstrumentalvariablestechniquescanbecombinedwithpaneldatatransformationstorelaxexogeneityassumptionsevenmore.Asageneralrule,itisagoodideatoapplyseveralreasonableeconometricmethodsandcomparetheresults.Thisoftenallowsustodeterminewhichofourassumptionsarelikelytobefalse.Evenifyouareverycarefulindevisingyourtopic,postulatingyourmodel,col-lectingyourdata,andcarryingouttheeconometrics,itisquitepossiblethatyouwillobtainpuzzlingresults—atleastsomeofthetime.Whenthathappens,thenaturalincli-nationistotrydifferentmodels,differentestimationtechniques,orperhapsdifferentsubsetsofdatauntiltheresultscorrespondmorecloselytowhatwasexpected.Virtuallyallappliedresearcherssearchovervariousmodelsbeforefindingthe“best”model.Unfortunately,thispracticeofdataminingviolatestheassumptionswehavemadeinoureconometricanalysis.TheresultsonunbiasednessofOLSandotherestimators,aswellasthetandFdistributionswederivedforhypothesistesting,assumethatweobserveasamplefollowingthepopulationmodelandweestimatethatmodelonce.Estimatingmodelsthatarevariantsofouroriginalmodelviolatesthatassumptionbecauseweareusingthesamesetofdatainaspecificationsearch.Ineffect,weusethe625\nd7/14/998:42PMPage626Part3AdvancedTopicsoutcomeoftestsbyusingthedatatorespecifyourmodel.Theestimatesandtestsfromdifferentmodelspecificationsarenotindependentofoneanother.Somespecificationsearcheshavebeenprogrammedintostandardsoftwarepack-ages.Apopularoneisknownasstepwiseregression,wheredifferentcombinationsofexplanatoryvariablesareusedinmultipleregressionanalysisinanattempttocomeupwiththebestmodel.Therearevariouswaysthatstepwiseregressioncanbeused,andwehavenointentionofreviewingthemhere.Thegeneralideaistoeitherstartwithalargemodelandkeepvariableswhosep-valuesarebelowacertainsignificancelevelortostartwithasimplemodelandaddvariablesthathavesignificantp-values.Sometimes,groupsofvariablesaretestedwithanFtest.Unfortunately,thefinalmodeloftendependsontheorderinwhichvariablesweredroppedoradded.[Formoreonstepwiseregression,seeDraperandSmith(1981).]Inaddition,thisisasevereformofdatamining,anditisdifficulttointerprettandFstatisticsinthefinalmodel.Onemightarguethatstepwiseregressionsimplyautomateswhatresearchersdoanywayinsearch-ingovervariousmodels.However,inmostapplications,oneortwoexplanatoryvari-ablesareofprimaryinterest,andthenthegoalistoseehowrobustthecoefficientsonthosevariablesaretoeitheraddingordroppingothervariables,ortochangingfunc-tionalform.Inprinciple,itispossibletoincorporatetheeffectsofdataminingintoourstatisti-calinference;inpractice,thisisverydifficultandisrarelydone,especiallyinsophis-ticatedempiricalwork.[SeeLeamer(1983)foranengagingdiscussionofthisproblem.]Butwecantrytominimizedataminingbynotsearchingovernumerousmodelsorestimationmethodsuntilasignificantresultisfoundandthenreportingonlythatresult.Ifavariableisstatisticallysignificantinonlyasmallfractionofthemodelsestimated,itisquitelikelythatthevariablehasnoeffectinthepopulation.19.5WRITINGANEMPIRICALPAPERWritingapaperthatuseseconometricanalysisisverychallenging,butitcanalsoberewarding.Asuccessfulpapercombinesacareful,convincingdataanalysiswithgoodexplanationsandexposition.Therefore,youmusthaveagoodgraspofyourtopic,goodunderstandingofeconometricmethods,andsolidwritingskills.Donotbediscouragedifyoufindwritinganempiricalpaperdifficult;mostprofessionalresearchershavespentmanyyearslearninghowtocraftanempiricalanalysisandtowritetheresultsinaconvincingform.Whilewritingstylesvary,manypapersfollowthesamegeneraloutline.Thefol-lowingparagraphsincludeideasforsectionheadingsandexplanationsaboutwhateachsectionshouldcontain.Theseareonlysuggestionsandhardlyneedtobestrictlyfol-lowed.Inthefinalpaper,eachsectionwouldbegivenanumber,usuallystartingwithonefortheintroduction.IntroductionTheintroductionstatesthebasicobjectivesofthestudyandexplainswhyitisimpor-tant.Itgenerallyentailsareviewoftheliterature,indicatingwhathasbeendoneandhowpreviousworkcanbeimprovedupon.(AsdiscussedinSection19.2,anextensive626\nd7/14/998:42PMPage627Chapter19CarryingoutanEmpiricalProjectliteraturereviewcanbeputinaseparatesection.)Presentingsimplestatisticsorgraphsthatrevealaseeminglyparadoxicalrelationshipisausefulwaytointroducethepaper’stopic.Forexample,supposethatyouarewritingapaperaboutfactorsaffectingfertil-ityinadevelopingcountry,withthefocusoneducationlevelsofwomen.Anappealingwaytointroducethetopicwouldbetoproduceatableoragraphshowingthatfertilityhasbeenfalling(say)overtimeandabriefexplanationofhowyouhopetoexaminethefactorscontributingtothedecline.Atthispoint,youmayalreadyknowthat,ceterisparibus,morehighlyeducatedwomenhavefewerchildrenandthataverageeducationlevelshaverisenovertime.Mostresearchersliketosummarizethefindingsoftheirpaperintheintroduction.Thiscanbeausefuldeviceforgrabbingthereader’sattention.Forexample,youmightstatethatyourbestestimateoftheeffectofmissing10hoursoflectureduringathirty-hourtermisaboutone-halfofagradepoint.Butthesummaryshouldnotbetooinvolvedbecauseneitherthemethodsnorthedatausedtoobtaintheestimateshaveyetbeenintroduced.Conceptual(orTheoretical)FrameworkThisisthesectionwhereyoudescribethegeneralapproachtoansweringthequestionyouhaveposed.Itcanbeformaleconomictheory,butinmanycases,itisanintuitivediscussionaboutwhatconceptualproblemsariseinansweringyourquestion.Asanexample,supposeyouarestudyingtheeffectsofeconomicopportunitiesandseverityofpunishmentoncriminalbehavior.Oneapproachtoexplainingparticipationincrimeistospecifyautilitymaximizationproblemwheretheindividualchoosestheamountoftimespentinlegalandillegalactivities,givenwageratesinbothkindsofactivities,aswellasvariablemeasuringprobabilityandseverityofpunishmentforcriminalactivity.Theusefulnessofsuchanexerciseisthatitsuggestswhichvariablesshouldbeincludedintheempiricalanalysis;itgivesguidance(butrarelyspecifics)astohowthevariablesshouldappearintheeconometricmodel.Oftenthereisnoneedtowritedownaneconomictheory.Foreconometricpolicyanalysis,commonsenseusuallysufficesforspecifyingamodel.Forexample,supposeyouareinterestedinestimatingtheeffectsofparticipationinAidforFamilieswithDependentChildren(AFDC)ontheeffectsofchildperformanceinschool.AFDCpro-videssupplementalincome,butparticipationalsomakesiteasiertoreceiveMedicaidandotherbenefits.Thehardpartofsuchananalysisisdecidingonthesetofvariablesthatshouldbecontrolledfor.Inthisexample,wecouldcontrolforfamilyincome(includingAFDCandanyotherwelfareincome),mother’seducation,whetherthefam-ilylivesinanurbanarea,andothervariables.Then,theinclusionofanAFDCpartici-pationindicator(hopefully)measuresthenonincomebenefitsofAFDCparticipation.AdiscussionofwhichfactorsshouldbecontrolledforandthemechanismsthroughwhichAFDCparticipationmightimproveschoolperformancesubstituteforformaleconomictheory.EconometricModelsandEstimationMethodsItisveryusefultohaveasectionthatcontainsafewequationsofthesortyouestimateandpresentintheresultssectionofthepaper.Thisallowsyoutofixideasaboutwhat627\nd7/14/998:42PMPage628Part3AdvancedTopicsthekeyexplanatoryvariableisandwhatotherfactorsyouwillcontrolfor.WritingequationscontainingerrortermsallowsyoutodiscusswhetheramethodsuchasOLSwillbeappropriate.Thedistinctionbetweenamodelandanestimationmethodshouldbemadeinthissection.Amodelrepresentsapopulationrelationship(broadlydefinedtoallowfortimeseriesequations).Forexample,weshouldwritecolGPA01alcohol2hsGPA3SAT4femaleu(19.1)todescribetherelationshipbetweencollegeGPAandalcoholconsumption,withsomeothercontrolsintheequation.Presumably,thisequationrepresentsapopulation,suchasallundergraduatesatauniversity.Thereareno“hats”(ˆ)onthejoroncolGPAbecausethisisamodel,notanestimatedequation.Wedonotputinnumbersforthejbecausewedonotknow(andneverwillknow)thesenumbers.Later,wewillestimatethem.Inthissection,donotanticipatethepresentationofyourempiricalresults.Inotherwords,donotstartwithageneralmodelandthensaythatyouomittedcertainvariablesbecausetheyturnedouttobeinsignificant.Suchdiscussionsshouldbeleftfortheresultssection.Atimeseriesmodeltorelatecity-levelcartheftstotheunemploymentrate(andothercontrols)couldlookliketheftst01unemt2unemt13carst(19.2)4convratet5convratet1ut,wherethetsubscriptisusefulforemphasizinganydynamicsintheequation(inthiscase,allowingforunemploymentandtheautomobiletheftconvictionratetohavelaggedeffects).Afterspecifyingamodelormodels,itisappropriatetodiscussestimationmethods.Inmostcases,thiswillbeOLS,but,forexample,inatimeseriesequation,youmightusefeasibleGLStodoaserialcorrelationcorrection(asinChapter12).However,themethodforestimatingamodelisquitedistinctfromthemodelitself.Itisnotmean-ingful,forinstance,totalkabout“anOLSmodel.”Ordinaryleastsquaresisamethodofestimation,andsoareweightedleastsquares,Cochrane-Orcutt,andsoon.Thereareusuallymanywaystoestimateanymodel.Youshouldexplainwhythemethodyouarechoosingiswarranted.Anyassumptionsthatareusedinobtaininganestimableeconometricmodelfromanunderlyingeconomicmodelshouldbeclearlydiscussed.Forexample,inthequalityofhighschoolexamplementionedinSection19.1,theissueofhowtomeasureschoolqualityiscentraltotheanalysis.ShoulditbebasedonaverageSATscores,percentageofgraduatesattendingcollege,student-teacherratios,averageeducationlevelofteach-ers,somecombinationofthese,orpossiblyothermeasures?Wealwayshavetomakeassumptionsaboutfunctionalformwhetherornotatheo-reticalmodelhasbeenpresented.Asyouknow,constantelasticityandconstantsemi-elasticitymodelsareattractivebecausethecoefficientsareeasytointerpret(aspercentages).Therearenohardrulesonhowtochoosefunctionalform,buttheguide-linesdiscussedinSection6.2seemtoworkwellinpractice.Youdonotneedanexten-628\nd7/14/998:42PMPage629Chapter19CarryingoutanEmpiricalProjectsivediscussionoffunctionalform,butitisusefultomentionwhetheryouwillbeesti-matingelasticitiesorasemi-elasticity.Forexample,ifyouareestimatingtheeffectofsomevariableonwageorsalary,thedependentvariablewillalmostsurelybeinloga-rithmicform,andyoumightaswellincludethisinanyequationsfromthebeginning.Youdonothavetopresentevery,orevenmost,ofthefunctionalformvariationsthatyouwillreportlaterintheresultssection.Oftenthedatausedinempiricaleconomicsareatthecityorcountylevel.Forexam-ple,supposethatforthepopulationofsmalltomid-sizecities,youwishtotestthehypothesisthathavingaminorleaguebaseballteamcausesacitytohavealowerdivorcerate.Inthiscase,youmustaccountforthefactthatlargercitieswillhavemoredivorces.Onewaytoaccountforthesizeofthecityistoscaledivorcesbythecityoradultpopulation.Thus,areasonablemodelislog(div/pop)01mlb2perCath3log(inc/pop)(19.3)otherfactors,wheremlbisadummyvariableequaltooneifthecityhasaminorleaguebaseballteam,perCathisthepercentageofthepopulationwhichisCatholic(soitisanumbersuchas34.6tomean34.6%).Notethatdiv/popisadivorcerate,whichisgenerallyeas-iertointerpretthantheabsolutenumberofdivorces.Anotherwaytocontrolforpopulationistoestimatethemodellog(div)01mlb2perCath3log(inc)4log(pop)(19.4)otherfactors.Theparameterofinterest,1,whenmultipliedby100,givesthepercentagedifferencebetweendivorcerates,holdingpopulation,percentCatholic,income,andwhateverelseisin“otherfactors”constant.Inequation(19.3),1measuresthepercentageeffectofminorleaguebaseballondiv/pop,whichcanchangeeitherbecausethenumberofdivorcesorthepopulationchanges.Usingthefactthatlog(div/pop)log(div)log(pop)andlog(inc/pop)log(inc)log(pop),wecanrewrite(19.3)aslog(div)01mlb2perccath3log(inc)(13)log(pop)otherfactors,whichshowsthat(19.3)isaspecialcaseof(19.4)with4(13)andjj,j0,1,2,and3.Alternatively,(19.4)isequivalenttoaddinglog(pop)asanadditionalexplanatoryvariableto(19.3).Thismakesiteasytotestforaseparatepopulationeffectonthedivorcerate.Ifyouareusingamoreadvancedestimationmethod,suchastwostageleastsquares,youneedtoprovidesomereasonsforwhyyouaredoingso.Ifyouuse2SLS,youmustprovideacarefuldiscussiononwhyyourIVchoicesfortheendogenousexplanatoryvariable(orvariables)arevalid.AswementionedinChapter15,therearetworequirementsforavariabletobeconsideredagoodIV.First,itmustbeomittedfromandexogenoustotheequationofinterest(structuralequation).Thisissomethingwemustassume.Second,itmusthavesomepartialcorrelationwiththeendogenousexplanatoryvariable.Thiswecantest.Forexample,inequation(19.1),youmightuse629\nd7/14/998:42PMPage630Part3AdvancedTopicsabinaryvariableforwhetherastudentlivesinadormitory(dorm)asanIVforalcoholconsumption.ThisrequiresthatlivingsituationhasnodirectimpactoncolGPA—sothatitisomittedfrom(19.1)—andthatitisuncorrelatedwithunobservedfactorsinuthathaveaneffectoncolGPA.Wewouldalsohavetoverifythatdormispartiallycor-relatedwithalcoholbyregressingalcoholondorm,hsGPA,SAT,andfemale.(SeeChapter15fordetails.)Youmightaccountfortheomittedvariableproblem(oromittedheterogeneity)byusingpaneldata.Again,thisiseasilydescribedbywritinganequationortwo.Infact,itisusefultoshowhowtodifferencetheequationsovertimetoremovetime-constantunobservables;thisgivesanequationthatcanbeestimatedbyOLS.Or,ifyouareusingfixedeffectsestimationinstead,yousimplystateso.Asasimpleexample,supposeyouaretestingwhetherhighercountytaxratesreduceeconomicactivity,asmeasuredbypercapitamanufacturingoutput.Supposethatfortheyears1982,1987,and1992,themodelislog(manufit)01d87t2d92t1taxit…aiuit,whered87tandd92tareyeardummyvariables,andtaxitisthetaxrateforcountyiattimet(inpercentform).Wewouldhaveothervariablesthatchangeovertimeintheequation,includingmeasuresforcostsofdoingbusiness(suchasaveragewages),mea-suresofworkerproductivity(asmeasuredbyaverageeducation),andsoon.Thetermaiisthefixedeffect,containingallfactorsthatdonotvaryovertime,anduitistheidio-syncraticerrorterm.Toremoveai,wecaneitherdifferenceacrosstheyearsorusetime-demeaning(thefixedeffectstransformation).TheDataYoushouldalwayshaveasectionthatcarefullydescribesthedatausedintheempiri-calestimation.Thisisparticularlyimportantifyourdataarenonstandardorhavenotbeenwidelyusedbyotherresearchers.Enoughinformationshouldbepresentedsothatareadercould,inprinciple,obtainthedataandredoyouranalysis.Inparticular,allapplicablepublicdatasourcesshouldbeincludedinthereferences,andshortdatasetscanbelistedinanappendix.Ifyouusedyourownsurveytocollectthedata,acopyofthequestionaireshouldbepresentedinanappendix.Alongwithadiscussionofthedatasources,besuretodiscusstheunitsofeachofthevariables(forexample,isincomemeasuredinhundredsorthousandsofdollars?).Includingatableofvariabledefinitionsisveryusefultothereader.Thenamesinthetableshouldcorrespondtothenamesusedindescribingtheeconometricresultsinthefollowingsection.Itisalsoveryinformativetopresentatableofsummarystatistics,suchasmini-mumandmaximumvalues,means,andstandarddeviationsforeachvariable.Havingsuchatablemakesiteasiertointerpretthecoefficientestimatesinthenextsection,anditemphasizestheunitsofmeasurementofthevariables.Forbinaryvariables,theonlynecessarysummarystatisticisthefractionofonesinthesample(whichisthesameasthesamplemean).Fortrendingvariables,thingslikemeansarelessinterest-ing.Itisoftenusefultocomputetheaveragegrowthrateinavariableovertheyearsinyoursample.630\nd7/14/998:42PMPage631Chapter19CarryingoutanEmpiricalProjectYoushouldalwaysclearlystatehowmanyobservationsyouhave.Fortimeseriesdatasets,identifytheyearsthatyouareusingintheanalysis,includingadescriptionofanyspecialperiodsinhistory(suchasWorldWarII).Ifyouuseapooledcrosssectionorapaneldataset,besuretoreporthowmanycross-sectionalunits(people,cities,andsoon)youhaveforeachyear.ResultsTheresultssectionshouldincludeyourestimatesofanymodelsformulatedinthemod-elssection.Youmightstartwithaverysimpleanalysis.Forexample,supposethatper-centofstudentsattendingcollegefromthegraduatingclass(percoll)isusedasameasureofthequalityofthehighschoolapersonattended.Then,anequationtoestimateislog(wage)01percollu.Ofcourse,thisdoesnotcontrolforseveralotherfactorsthatmaydeterminewagesandthatmaybecorrelatedwithpercoll.Butasimpleanalysiscandrawthereaderintothemoresophisticatedanalysisandrevealtheimportanceofcontrollingforotherfactors.Ifonlyafewequationsareestimated,youcanpresenttheresultsinequationformwithstandarderrorsinparenthesesbelowestimatedcoefficients.Ifyourmodelhassev-eralexplanatoryvariablesandyouarepresentingseveralvariationsonthegeneralmodel,itisbettertoreporttheresultsintabularratherthanequationform.Mostofyoushouldhaveatleastonetable,whichshouldalwaysincludeatleasttheR-squaredandthenumberofobservationsforeachequation.Otherstatistics,suchastheadjustedR-squared,canalsobelisted.Themostimportantthingistodiscusstheinterpretationandstrengthofyourempir-icalresults.Dothecoefficientshavetheexpectedsigns?Aretheystatisticallysignifi-cant?Ifacoefficientisstatisticallysignificantbuthasacounterintuitivesign,whymightthisbetrue?Itmightberevealingaproblemwiththedataortheeconometricmethod(forexample,OLSmaybeinappropriateduetoomittedvariablesproblems).Besuretodescribethemagnitudesofthecoefficientsonthemajorexplanatoryvari-ables.Oftenthereareoneortwopolicyvariablesthatarecentraltothestudy.Theirsigns,magnitudes,andstatisticalsignificanceshouldbetreatedindetail.Remembertodistinguishbetweeneconomicandstatisticalsignificance.Ifatstatisticissmall,isitbecausethecoefficientispracticallysmallorbecauseitsstandarderrorislarge?Inadditiontodiscussingestimatesfromthemostgeneralmodel,youcanprovideinterestingspecialcases,especiallythoseneededtotestcertainmultiplehypotheses.Forexample,inastudytodeterminewagedifferentialsacrossindustries,youmightpresenttheequationwithouttheindustrydummies;thisallowsthereadertoeasilytestwhethertheindustrydifferentialsarestatisticallysignificant(usingtheR-squaredformoftheFtest).Donotworrytoomuchaboutdroppingvariousvariablestofindthe“best”combinationofexplanatoryvariables.Aswementionedearlier,thisisadifficultandnotevenverywell-definedtask.Onlyifeliminatingasetofvariablessubstantiallyaltersthemagnitudesand/orsignificanceofthecoefficientsofinterestisthisimportant.Droppingagroupofvariablestosimplifythemodel—suchasquadraticsorinterac-tions—canbejustifiedviaanFtest.Ifyouhaveusedatleasttwodifferentmethods—suchasOLSand2SLS,orlevelsanddifferencingforatimeseries,orpooledOLSversusdifferencingwithapaneldata631\nd7/14/998:42PMPage632Part3AdvancedTopicsset—thenyoushouldcommentonanycriticaldifferences.Inparticular,ifOLSgivescounterintuitiveresults,didusing2SLSorpaneldatamethodsimprovetheestimates?ConclusionsThiscanbeashortsectionthatsummarizeswhatyouhavelearned.Forexample,youmightwanttopresentthemagnitudeofacoefficientthatwasofparticularinterest.Theconclusionshouldalsodiscusscaveatstotheconclusionsdrawn,anditmightevensug-gestdirectionsforfurtherresearch.Itisusefultoimaginereadersturningfirsttotheconclusioninordertodecidewhethertoreadtherestofthepaper.StyleHintsYoushouldgiveyourpaperatitlethatreflectsitstopic.Papersshouldbetypedanddouble-spaced.Allequationsshouldbeginonanewline,andtheyshouldbecenteredandnumberedconsecutively,thatis,(1),(2),(3),andsoon.Largegraphsandtablesmaybeincludedafterthemainbody.Inthetext,refertopapersbyauthoranddate,forexample,White(1980).Thereferencesectionattheendofthepapershouldbedoneinstandardformat.Severalexamplesaregiveninthereferencesatthebackofthetext.Whenyouintroduceanequationinthe“EconometricModels”section,youshoulddescribetheimportantvariables:thedependentvariableandthekeyindependentvari-ableorvariables.Tofocusonasingleindependentvariable,youcanwriteanequation,suchasGPA01alcoholxuorlog(wage)01educxu,wherethenotationxisshorthandforseveralotherexplanatoryvariables.Atthispoint,youneedonlydescribethemgenerally;theycanbedescribedspecificallyinthedatasectioninatable.Forexample,inastudyofthefactorsaffectingchiefexecutiveoffi-cersalaries,youmightincludethefollowingtableinthedatasection:Table1:VariableDescriptionssalary:annualsalary(includingbonuses)in1990(inthousands)sales:firmsalesin1990(inmillions)roe:averagereturnonequityfrom1988–1990(inpercent)pcsal:percentagechangeinsalaryfrom1988–1990pcroe:percentagechangeinroefrom1988–1990continued632\nd7/14/998:42PMPage633Chapter19CarryingoutanEmpiricalProjectTable1:(concluded)indust:1ifanindustrialcompany,0otherwisefinance:1ifafinancialcompany,0otherwiseconsprod:1ifaconsumerproductscompany,0otherwiseutil:1ifautilitycompany,0otherwiseceoten:numberofyearsasCEOofthecompanyAtableofsummarystatisticsusingthedataset401K.RAW,whichweusedforstudyingthefactorsthataffectparticipationin401(k)pensionplans,mightbesetupasfollows:Table2:SummaryStatisticsStandardVariableMeanDeviationMinimumMaximumprate.869.167.0231mrate.746.844.0115employ4,621.0116,299.6453443,040age13.149.63476sole.415.49301NumberofObservations3,784Intheresultssection,youcaneitherwritetheestimatesinequationform,asweoftenhavedone,orinatable.Especiallywhenseveralmodelshavebeenestimatedwithdifferentsetsofexplanatoryvariables,tablesareveryuseful.Ifyouwriteouttheesti-matesasanequation,forexample,log(salˆary)(2.45)(.236)log(sales)(.008)roe(.061)ceotenlog(salˆary)(0.93)(.115)log(sales)(.003)roe(.028)ceoten2n204,R.351,besuretostatenearthefirstequationthatstandarderrorsareinparentheses.ItisacceptabletoreportthetstatisticsfortestingH0:j0,ortheirabsolutevalues,butitismostimportanttostatewhatyouaredoing.633\nd7/14/998:42PMPage634Part3AdvancedTopicsIfyoureportyourresultsintabularform,makesurethedependentandindependentvariablesareclearlyindicated.Again,statewhetherstandarderrorsortstatisticsarebelowthecoefficients(withtheformerpreferred).Someauthorsliketouseasteriskstoindicatestatisticalsignificanceatdifferentsignificancelevels(forexample,onestarmeanssignificantat5%,twostarsmeansignificantat10%butnot5%andsoon).Thisisnotnecessaryifyoucarefullydiscussthesignificanceoftheexplanatoryvariablesinthetext.Asampletableofresultsfollows:Table3:OLSResultsDependentVariable:ParticipationRateIndependentVariablesmrate.156.239.218(.012)(.042)(.342)2mrate—.087.096(.043)(.073)log(emp).112.112.098(.014)(.014)(.111)2log(emp).0057.0057.0052(.0009)(.0009)(.0007)age.0060.0059.0050(.0010)(.0010)(.0021)2age.00007.00007.00006(.00002)(.00002)(.00002)sole.0001.0008.0006(.0058)(.0058)(.0061)constant1.213.198.085(0.051)(.052)(.041)industrydummies?nonoyesObservations:3,7843,7843,784R-Squared:.143.152.152Note:Thequantitiesinparenthesesbelowtheestimatesarethestandarderrors.634\nd7/14/998:42PMPage635Chapter19CarryingoutanEmpiricalProjectYourresultswillbeeasiertoreadandinterpretifyouchoosetheunitsofbothyourdependentandindependentvariablessothatcoefficientsarenottoolargeortoosmall.Youshouldneverreportnumberssuchas1.051e-007or3.524e+006foryourcoeffi-cientsorstandarderrors,andyoushouldnotusescientificnotation.Ifcoefficientsareeitherextremelysmallorlarge,rescalethedependentorindependentvariables,aswediscussedinChapter6.Youshouldlimitthenumberofdigitsreportedafterthedecimalpoint.Forexample,ifyourregressionpackageestimatesacoefficienttobe.54821059,youshouldreportthisas.548,oreven.55,inthepaper.Asageneralrule,thecommandsthatyourparticulareconometricspackageusestoproduceresultsshouldnotappearinthepaper;onlytheresultsareimportant.Ifsomespecialcommandwasusedtocarryoutacertainestimationmethod,thiscanbegiveninanappendix.Anappendixisalsoagoodplacetoincludeextraresultsthatsupportyouranalysisbutarenotcentraltoit.SUMMARYInthischapter,wehavediscussedtheingredientsofasuccessfulempiricalstudyandhaveprovidedhintsthatcanimprovethequalityofananalysis.Ultimately,thesuccessofanystudydependscruciallyonthecareandeffortputintoit.KEYTERMSDataMiningSensitivityAnalysisInternetSpreadsheetOn-LineDataBasesTextEditorOn-LineSearchServicesText(ASCII)FileSAMPLEEMPIRICALPROJECTSThroughoutthetext,wehaveseenexamplesofeconometricanalysisthateithercamefromorweremotivatedbypublishedworks.Hopefully,thesehavegivenyouagoodideaaboutthescopeofempiricalanalysis.Weincludethefollowinglistasadditionalexamplesofquestionsthatothershavefoundorarelikelytofindinteresting.Theseareintendedtostimulateyourimagination;noattemptismadetofillinallofthedetailsofspecificmodels,datarequirements,oralternativeestimationmethods.Itshouldbepos-sibletocompletetheseprojectsinoneterm.1.Doyourowncampussurveytoansweraquestionofinterestatyouruniversity.Forexample:Whatistheeffectofworking,oncollegeGPA?Youcanaskstu-dentsabouthighschoolGPA,collegeGPA,ACTorSATscores,hoursworkedperweek,participationinathletics,major,gender,race,andsoon.Then,usethesevariablestocreateamodelthatexplainsGPA.Howmuchofaneffect,ifany,doesanotherhourworkedperweekhaveonGPA?Oneissueofconcernisthathoursworkedmightbeendogenous:itmightbecorrelatedwithunob-servedfactorsthataffectcollegeGPA,orlowerGPAsmightcausestudentstoworkmore.635\nd7/14/998:42PMPage636Part3AdvancedTopicsAbetterapproachwouldbetocollectcumulativeGPApriortothesemes-terandthentoobtainGPAforthemostrecentsemester,alongwithamountworkedduringthatsemester,andtheothervariables.Now,cumulativeGPAcouldbeusedasacontrol(explanatoryvariable)intheequation.2.Therearemanyvariantsontheprecedingtopic.Youcanstudytheeffectsofdrugoralcoholusage,oroflivinginafraternity,ongradepointaverage.Youwouldwanttocontrolformanyfamilybackgroundvariables,aswellasprevi-ousperformancevariables.3.Doguncontrollawsatthecitylevelreduceviolentcrimes?Suchquestionscanbedifficulttoanswerwithasinglecrosssectionbecausecityandstatelawsareoftenendogenous.[SeeKleckandPatterson(1993)foranexample.Theyusedcross-sectionaldataandinstrumentalvariablesmethods,buttheirIVsareques-tionable.]Paneldatacanbeveryusefulforinferringcausalityinthesecontexts.Ataminimum,youcouldcontrolforapreviousyear’sviolentcrimerate.4.LowandMcPheters(1983)usedcitycross-sectionaldataonwageratesandestimatesofriskofdeathforpoliceofficers,alongwithothercontrols.Theideaistodeterminewhetherpoliceofficersarecompensatedforworkingincitieswithahigherriskofon-the-jobinjuryordeath.5.Doparentalconsentlawsincreasetheteenagebirthrate?Youcanusestate-leveldataforthis:eitheratimeseriesforagivenstateor,evenbetter,apaneldatasetofstates.Dothesamelawsreduceabortionratesamongteenagers?TheStatisticalAbstractoftheUnitedStatescontainsallkindsofstate-leveldata.Levine,Trainor,andZimmerman(1996)studiedtheeffectsofabortionfundingrestrictionsonsimilaroutcomes.Otherfactors,suchasaccesstoabortions,mayaffectteenbirthandabortionrates.6.Dochangesintrafficlawsaffecttrafficfatalities?McCarthy(1994)containsananalysisofmonthlytimeseriesdataforthestateofCalifornia.Asetofdummyvariablescanbeusedtoindicatethemonthsinwhichcertainlawswereineffect.ThefileTRAFFIC2.RAWcontainsthedatausedbyMcCarthy.Analter-nativeistoobtainapaneldatasetonstatesintheUnitedStates,whereyoucanexploitvariationinlawsacrossstates,aswellasacrosstime.(SeethefileTRAFFIC1.RAW.)MullahyandSindelar(1994)usedindividual-leveldatamatchedwithstatelawsandtaxesonalcoholtoestimatetheeffectsoflawsandtaxesontheprob-abilityofdrivingdrunk.7.Areblacksdiscriminatedagainstinthelendingmarket?HunterandWalker(1996)lookedatthisquestion;infact,weusedtheirdatainExercises7.16and17.9.8.Isthereamarriagepremiumforprofessionalathletes?KorenmanandNeumark(1991)foundasignificantwagepremiumformarriedmenafterusingavarietyofeconometricmethods.Professionalathletes—suchasNationalBasketballAssociationplayers,majorleaguebaseballplayers,andprofessionalgolfers—provideaninterestinggroupinwhichtostudythemarriagepremiumbecausewecanobserveseveralproductivitymeasures.Withplayersinindividualsports,suchasgolfortennis,earningsdirectlyreflectproductivity.Inteamsports,salarymaynotentirelyreflectproductivity—forexample,yearsinthe636\nd7/14/998:42PMPage637Chapter19CarryingoutanEmpiricalProjectleaguemightmatter.Sowecanincludeamarriageindicatorinanequationwithsomethinglikescoringasthedependentvariable,aswellasinaregressionwherelog(salary)isthedependentvariableandseveralproductivitycontrolsareamongtheindependentvariables.9.Answerthequestion:Arecigarettesmokerslessproductive?Avariantonthisis:Doworkerswhosmoketakemoresickdays(everythingelsebeingequal)?MullahyandPortney(1990)useindividual-leveldatatoevaluatethisques-tion.Youcouldusedataat,say,themetropolitanlevel.Somethinglikeaver-ageproductivityinmanufacturingcanberelatedtopercentofmanufacturingworkerswhosmoke.Othervariables,suchasaverageworkereducation,cap-italperworker,andsizeofthecity(youcanthinkofmore)shouldbecon-trolledfor.10.Dominimumwagesalleviatepoverty?Youcanusestateorcountydatatoanswerthisquestion.Theideaisthattheminimumwagevariesacrossstatebecausesomestateshavehigherminimumsthanthefederalminimum.Further,therearechangesovertimeinthenominalminimumwithinastate,someduetochangesatthefederallevelandsomebecauseofchangesatthestatelevel.NeumarkandWascher(1995)usedapaneldatasetonstatestoestimatetheeffectsoftheminimumwageontheemploymentratesofyoungworkers,aswellasonschoolenrollmentrates.11.Whatfactorsaffectstudentperformanceatpublicschools?Itisfairlyeasytogetschool-leveloratleastdistrict-leveldatainmoststates.Doesspendingperstudentmatter?Dostudent-teacherratioshaveanyeffects?Itisdifficulttoesti-mateceterisparibuseffectsbecausespendingisrelatedtootherfactors,suchasfamilyincomesorpovertyrates.ThedatasetMEAP93.RAW,forMichiganhighschools,containsameasureofthepovertyrates.Anotherpossibilityistousepaneldata,ortoatleastcontrolforapreviousyear’sperformancemeasure(suchasaveragetestscoreorpercentageofstudentspassinganexam).Youcanlookatlessobviousfactorsthataffectstudentperformance.Forexample,aftercontrollingforincome,doesfamilystructurematter?Perhapsfamilieswithtwoparents,butonlyoneworkingforawage,haveapositiveeffectonperformance.(Therecouldbeatleasttwochannels:parentsspendmoretimewiththechildren,andtheymightalsovolunteeratschool.)Whatabouttheeffectofsingle-parenthouseholds,controllingforincomeandotherfactors?Youcanmergecensusdataforoneortwoyearswithschooldistrictdata.Dopublicschoolswithmoreprivateschoolsnearbybettereducatetheirstu-dentsbecauseofcompetition?Thereisatrickysimultaneityissueherebecauseprivateschoolsareprobablylocatedinareaswherethepublicschoolsarealreadypoor.Hoxby(1994)usedaninstrumentalvariablesapproach,wherepopulationproportionsofvariousreligionswereIVsforthenumberofprivateschools.Rouse(1998)studiedadifferentquestion:DidstudentswhowereabletoattendaprivateschoolduetotheMilwaukeevoucherprogramperformbetterthanthosewhodidnot?Sheusedpaneldataandwasabletocontrolforanunobservedstudenteffect.637\nd7/14/998:42PMPage638Part3AdvancedTopics12.Canexcessreturnsonastock,orastockindex,bepredictedbythelaggedprice/dividendratio?Or,bylaggedinterestratesorweeklymonetarypolicy?Itwouldbeinterestingtopickaforeignstockindex,oroneofthelesswell-knownU.S.indexes.Cochrane(1997)containsanicesurveyofrecenttheoriesandempiricalresultsforexplainingexcessstockreturns.13.Isthereracialdiscriminationinthemarketforbaseballcards?Thisinvolvesrelatingthepricesofbaseballcardstofactorsthatshouldaffecttheirprices,suchascareerstatistics,whethertheplayerisintheHallofFame,andsoon.Holdingotherfactorsfixed,docardsofblackorHispanicplayerssellatadis-count?14.Youcantestwhetherthemarketforgamblingonsportsisefficient.Forexam-ple,doesthespreadonfootballorbasketballgamescontainallusableinfor-mationforpickingagainstthespread?ThedatasetPNTSPRD.RAWcontainsinformationonmen’scollegebasketballgames.Theoutcomevariableisbinary.Wasthespreadcoveredornot?Then,youcantrytofindinformationthatwasknownpriortoeachgame’sbeingplayedinordertopredictwhetherthespreadiscovered.(Goodluck!)15.Whateffect,ifany,doessuccessincollegeathleticshaveonotheraspectsoftheuniversity(applications,qualityofstudents,qualityofnonathleticdepart-ments)?McCormickandTinsley(1987)lookedattheeffectsofathleticsuccessatmajorcollegesonchangesinSATscoresofenteringfreshman.Timingisimportanthere:presumably,itisrecentpastsuccessthataffectscurrentappli-cationsandstudentquality.Onemustcontrolformanyotherfactors—suchastuitionandmeasuresofschoolquality—tomaketheanalysisconvincingbecause,withoutcontrollingforotherfactors,thereisanegativecorrelationbetweenacademicsandathleticperformance.Avariantistomatchupnaturalrivalsinfootballormen’sbasketballandtolookatdifferencesacrossschoolasafunctionofwhichschoolwonthefootballgameoroneormorebasketballgames.ATHLET1.RAWandATHLET2.RAWaresmalldatasetsthatcouldbeexpandedandupdated.16.Collectmurderratesforasampleofcitiesorcounties(say,fromtheFBIuni-formcrimereports)fortwoyears.MakethelatteryearsuchthateconomicanddemographicvariablesareeasytoobtainfromtheCountyandCityDataBook.FromtheStatisticalAbstractoftheUnitedStates,youcanobtainthetotalnum-berofpeopleondeathrow,plusexecutionsforinterveningyearsatthestatelevel.Iftheyearsare1990and1985,youmightestimatemrdrte9001mrdrte852executionsotherfactors,whereinterestisinthecoefficientonexecutions.Thelaggedmurderrateandotherfactorsserveascontrols.Otherfactorsmayalsoactasadeterrenttocrime.Forexample,Cloninger(1991)presentedacross-sectionalanalysisoftheeffectsoflethalpoliceresponseoncrimerates.Asadifferenttwist,whatfactorsaffectcrimeratesoncollegecampuses?Doesthefractionofstudentslivinginfraternitiesorsororitieshaveaneffect?Doesthesizeofthepoliceforcematter,orthekindofpolicingused?(Becare-638\nd7/14/998:42PMPage639Chapter19CarryingoutanEmpiricalProjectfulaboutinferringcausalityhere.)Doeshavinganescortprogramhelpreducecrime?Whataboutcrimeratesinnearbycommunities?Recently,collegesanduniversitieshavebeenrequiredtoreportcrimestatistics;inpreviousyears,reportingwasvoluntary.17.Whatfactorsaffectmanufacturingproductivityatthestatelevel?Inadditiontolevelsofcapitalandworkereducation,youcouldlookatdegreeofunioniza-tion.Apaneldataanalysiswouldbemostconvincinghere,usingtwocensusyears(say1980and1990).Clark(1984)providesananalysisofhowunion-izationaffectsfirmperformanceandproductivity.Whatothervariablesmightexplainproductivity?Firm-leveldatacanbeobtainedfromCompustat.Forexample,otherfac-torsbeingfixed,dochangesinunionizationaffectstockpriceofafirm?18.Usestate-orcounty-leveldataor,ifpossible,schooldistrict-leveldatatolookatthefactorsthataffecteducationspendingperpupil.Aninterestingquestionis:Otherthingsbeingequal(suchasincomeandeducationlevelsofresidents),dodistrictswithalargerpercentageofelderlypeoplespendlessonschools?Censusdatacanbematchedwithschooldistrictspendingdatatoobtainaverylargecrosssection.TheU.S.DepartmentofEducationcompilessuchdata.19.Whataretheeffectsofstateregulations,suchasmotorcyclehelmetlaws,onmotorcyclefatalities?Or,dodifferencesinboatinglaws—suchasminimumoperatingage—helptoexplainboatingaccidentrates?TheU.S.DepartmentofTransportationcompilessuchinformation.ThiscanbemergedwithdatafromtheStatisticalAbstractoftheUnitedStates.Apaneldataanalysisseemstobewarrantedhere.20.Whatfactorsaffectoutputgrowth?Twofactorsofinterestareinflationandinvestment[forexample,Blomström,Lipsey,andZejan(1996)].Youmightusetimeseriesdataonacountryyoufindinteresting.Or,youcoulduseacrosssec-tionofcountries,asinDeLongandSummers(1991).FriedmanandKuttner(1992)foundevidencethat,atleastinthe1980s,thespreadbetweenthecom-mercialpaperrateandthetreasurybillrateaffectsrealoutput.21.WhatisthebehaviorofmergersintheU.S.economy(orsomeothereconomy)?ShughartandTollison(1984)characterize(thelogof)annualmergersintheU.S.economyasarandomwalkbyshowingthatthedifferenceinlogs—roughly,thegrowthrate—isunpredictablegivenpastgrowthrates.Doesthisstillhold?Doesitholdacrossvariousindustries?Whatpastmeasuresofeco-nomicactivitycanbeusedtoforecastmergers?22.Whatfactorsmightexplainracialandgenderdifferencesinemploymentandwages?Forexample,Holzer(1991)reviewedtheevidenceonthe“spatialmis-matchhypothesis”toexplaindifferencesinemploymentratesbetweenblacksandwhites.KorenmanandNeumark(1992)examinedtheeffectsofchildbear-ingonwomen’swages,whileHerschandStratton(1997)lookedattheeffectsofhouseholdresponsibilitiesonmen’sandwomen’swages.23.Obtainmonthlyorquarterlydataonteenageemploymentrates,theminimumwage,andfactorsthataffectteenemployment,toestimatetheeffectsoftheminimumwageonteenemployment.Solon(1985)usedquarterlyU.S.data,whileCastillo-FreemanandFreeman(1992)usedannualdataonPuertoRico.639\nd7/14/998:42PMPage640Part3AdvancedTopicsItmightbeinformativetoanalyzetimeseriesdataonalow-wagestateintheUnitedStates—wherechangesintheminimumwagearelikelytohavethelargesteffect.24.Atthecitylevel,estimateatimeseriesmodelforcrime.AnexampleisCloningerandSartorius(1979).Asarecenttwist,youmightestimatetheeffectsofcommunitypolicingormidnightbasketballprograms,relativelynewinnovationsinfightingcrime.Inferringcausalityistricky.Includingalaggeddependentvariablemightbehelpful.Becauseyouareusingtimeseriesdata,youshouldbeawareofthespuriousregressionproblem.Grogger(1990)useddataondailyhomicidecountstoestimatethedeter-renteffectsofcapitalpunishment.Mighttherebeotherfactors—suchasnewsonlethalresponsebypolice—thathaveaneffectondailycrimecounts?25.Arethereaggregateproductivityeffectsofcomputerusage?Youwouldneedtoobtaintimeseriesdata,perhapsatthenationallevel,onproductivity,percent-ageofemployeesusingcomputers,andotherfactors.Whataboutspending(probablyasafractionoftotalsales)onresearchanddevelopment?Whatsoci-ologicalfactorsmightaffectproductivity?alcoholusage?divorcerates?26.Whatfactorsaffectchiefexecutiveofficersalaries?ThefilesCEOSAL1.RAWandCEOSAL2.RAWaredatasetsthathavevariousfirmperformancemea-sures,aswellasinformationsuchastenureandeducation.Youcancertainlyupdatethesedatafilesandlookforotherinterestingfactors.RoseandShepard(1997)consideredfirmdiversificationasoneimportantdeterminantofCEOcompensation.27.Dodifferencesintaxcodesacrossstatesaffecttheamountofforeigndirectinvestment?Hines(1996)studiedtheeffectsofstatecorporatetaxes,alongwiththeabilitytoapplyforeigntaxcredits,oninvestmentfromoutsidetheUnitedStates.28.Whatfactorsaffectelectionoutcomes?Doesspendingmatter?Dovotesonspe-cificissuesmatter?Doesthestateofthelocaleconomymatter?See,forexam-ple,Levitt(1994)andthedatasetsVOTE1.RAWandVOTE2.RAW.Fair(1996)performedatimeseriesanalysisofU.S.presidentialelections.LISTOFJOURNALSThefollowingisapartiallistofpopularjournalscontainingresearchinempiricalbusi-ness,economics,andothersocialsciences.AcompletesetofjournalscanbefoundontheInternet.AmericanEconomicReviewAmericanJournalofAgriculturalEconomicsAmericanPoliticalScienceReviewAppliedEconomicsBrookingsPapersonEconomicActivityCanadianJournalofEconomicsDemographyEconomicInquiry640\nd7/14/998:42PMPage641Chapter19CarryingoutanEmpiricalProjectEconomicaEconomicsLettersEmpiricalEconomicsFederalReserveBulletinInternationalEconomicReviewJournalofAppliedEconometricsJournalofBusinessandEconomicStatisticsJournalofDevelopmentEconomicsJournalofEconomicEducationJournalofEmpiricalFinanceJournalofEnvironmentalEconomicsandManagementJournalofFinanceJournalofHealthEconomicsJournalofHumanResourcesJournalofIndustrialEconomicsJournalofInternationalEconomicsJournalofLaborEconomicsJournalofPoliticalEconomyJournalofPublicEconomicsJournalofMonetaryEconomicsJournalofMoney,Credit,andBankingJournalofQuantitativeCriminologyJournalofUrbanEconomicsNationalBureauofEconomicResearchWorkingPaperSeriesNationalTaxJournalPublicFinanceQuarterlyQuarterlyJournalofEconomicsRegionalScience&UrbanEconomicsReviewofEconomicStudiesReviewofEconomicsandStatisticsDATASOURCESTherearenumerousdatasourcesavailablethroughouttheworld.Governmentsofmostcountriescompileawealthofdata;somegeneralandeasilyaccessibledatasourcesfortheUnitedStates,suchastheEconomicReportofthePresident,theStatisticalAbstractoftheUnitedStates,andtheCountyandCityDataBook,havealreadybeenmentioned.InternationalfinancialdataonmanycountriesarepublishedannuallyinInternationalFinancialStatistics.Variousmagazines,likeBusinessWeekandU.S.NewsandWorldReport,oftenpublishstatistics—suchasCEOsalariesandfirmperformance,orrank-ingofacademicprograms—thatarenovelandcanbeusedinaneconometricanalysis.Ratherthanattemptingtoprovidealisthere,weinsteadgivesomeInternetaddressesthatarecomprehensivesourcesforeconomists.Averyusefulsiteforecono-mists,calledResourcesforEconomistsontheInternet,ismaintainedbyBillGoffeattheUniversityofSouthernMississippi.Theaddressis641\nd7/14/998:42PMPage642Part3AdvancedTopicshttp://econwpa.wustl.edu/EconFAQ/EconFAQ.html.Thissiteprovideslinkstojournals,datasources,andlistsofprofessionalandacademiceconomists.Itisquitesimpletouse.TheBusinessandEconomicStatisticssectionoftheAmericanStatisticalAssociationcontainsanextremelydetailedlistofdatasourcesandprovideslinkstothem.Theaddressishttp://www.econ-datalinks.org.Inaddition,theJournalofAppliedEconometricsandtheJournalofBusinessandEconomicsStatisticshavedataarchivesthatcontaindatasetsusedinmostpaperspub-lishedinthejournalsoverthepastseveralyears.Ifyoufindadatasetthatinterestsyou,thisisagoodwaytogo,asmuchofthecleaningandformattingofthedatahavealreadybeendone.Thedownsideisthatsomeofthesedatasetsareusedineconometricanaly-sesthataremoreadvancedthanwehavelearnedaboutinthistext.Ontheotherhand,itisoftenusefultoestimatesimplermodelsusingstandardeconometricmethodsforcomparison.Manyuniversities,suchastheUniversityofCalifornia,Berkeley,theUniversityofMichigan,andtheUniversityofMaryland,maintainveryextensivedatasetsaswellaslinkstoavarietyofdatasets.Yourownlibrarypossiblycontainsanextensivesetoflinkstodatabasesinbusiness,economics,andtheothersocialsciences.Theregionalfederalreservebanks,suchastheoneinSt.Louis,manageavarietyofdata.TheNationalBureauofEconomicResearchpostsdatasetsusedbysomeofitsresearchers.Naturally,stateandfederalgovernmentsnowpublishawealthofdatathatcanbeaccessedviatheInternet.CensusdataarepubliclyavailablefromtheDepartmentofCensus.(TwousefulpublicationsaretheCensusofManufacturing,publishedinyearsendingwithtwoandseven,andtheCensusofthePopulation,publishedatthebegin-ningofeachdecade.)Otheragencies,suchastheDepartmentofJustice,alsomakedataavailabletothepublic.642\n\nxd7/14/998:51PMPage643AppendixABasicMathematicalToolshisappendixcoverssomebasicmathematicsthatareusedineconometricanaly-Tsis.Wesummarizevariouspropertiesofthesummationoperator,studypropertiesoflinearandcertainnonlinearequations,andreviewproportionsandpercents.Wealsopresentsomespecialfunctionsthatoftenariseinappliedeconometrics,includ-ingquadraticfunctionsandthenaturallogarithm.Thefirstfoursectionsrequireonlybasicalgebraskills.SectionA.5containsabriefreviewofdifferentialcalculus;whileaknowledgeofcalculusisnotnecessarytounderstandmostofthetext,itisusedinsomeend-of-chapterappendicesandinseveralofthemoreadvancedchaptersinPartIII.A.1THESUMMATIONOPERATORANDDESCRIPTIVESTATISTICSThesummationoperatorisausefulshorthandformanipulatingexpressionsinvolvingthesumsofmanynumbers,anditplaysakeyroleinstatisticsandeconometricanaly-sis.If{xi:i1,…,n}denotesasequenceofnnumbers,thenwewritethesumofthesenumbersasnxix1x2…xn.(A.1)i1Withthisdefinition,thesummationoperatoriseasilyshowntohavethefollowingprop-erties:PROPERTYSUM.1:Foranyconstantc,ncnc.(A.2)i1PROPERTYSUM.2:Foranyconstantc,nncxicxi.(A.3)i1i1643\nxd7/14/998:51PMPage644AppendixABasicMathematicalToolsPROPERTYSUM.3:If{(xi,yi):i1,2,…,n}isasetofnpairsofnumbers,andaandbareconstants,thennnn(axibyi)axibyi.(A.4)i1i1i1Itisalsoimportanttobeawareofsomethingsthatcannotbedonewiththesum-mationoperator.Let{(xi,yi):i1,2,…,n}againbeasetofnpairsofnumberswithyi0foreachi.Then,nnn(xi/yi)xiyi.i1i1i1Inotherwords,thesumoftheratiosisnottheratioofthesums.Inthen2case,theapplicationoffamiliarelementaryalgebraalsorevealsthislackofequality:x1/y1x2/y2(x1x2)/(y1y2).Similarly,thesumofthesquaresisnotthesquareofthenn22sum:xixi,exceptinspecialcases.Thatthesetwoquantitiesarenotgener-i1i122222allyequaliseasiesttoseewhenn2:x1x2(x1x2)x12x1x2x2.Givennnumbers{xi:i1,…,n},wecomputetheiraverageormeanbyaddingthemupanddividingbyn:nx¯(1/n)xi.(A.5)i1Whenthexiareasampleofdataonaparticularvariable(suchasyearsofeducation),weoftencallthisthesampleaverage(orsamplemean)toemphasizethatitiscom-putedfromaparticularsetofdata.Thesampleaverageisanexampleofadescriptivestatistic;inthiscase,thestatisticdescribesthecentraltendencyofthesetofpointsxi.Therearesomebasicpropertiesaboutaveragesthatareimportanttounderstand.First,supposewetakeeachobservationonxandsubtractofftheaverage:dixix¯(the“d”herestandsfordeviationfromtheaverage).Thenthesumofthesedeviationsisalwayszero:nnnnndi(xix¯)xix¯xinx¯nx¯nx¯0.i1i1i1i1i1Wesummarizethisasn(xix¯)0.(A.6)i1Asimplenumericalexampleshowshowthisworks.Supposen5andx16,x21,x32,x40,andx55.Thenx¯2,andthedemeanedsampleis{4,1,4,2,3}.Addingtheseupgiveszero,whichisjustwhatequation(A.6)says.InourtreatmentofregressionanalysisinChapter2,weneedtoknowsomeaddi-tionalalgebraicfactsinvolvingdeviationsfromsampleaverages.Animportantoneis644\nxd7/14/998:51PMPage645AppendixABasicMathematicalToolsthatthesumofsquareddeviationsisthesumofthesquaredximinusntimesthesquareofx¯:nn222(xix¯)xin(x¯).(A.7)i1i1Thiscanbeshownusingbasicpropertiesofthesummationoperator:nn222(xix¯)(xi2xix¯x¯)i1i1nn22xi2x¯xin(x¯)i1i1nn22222xi2n(x¯)n(x¯)xin(x¯).i1i1Givenadatasetontwovariables,{(xi,yi):i1,2,…,n},itcanalsobeshownthatnn(xix¯)(yiy¯)xi(yiy¯)i1i1nn(A.8)(xix¯)yixiyin(x¯y¯);i1i1thisisageneralizationofequation(A.7)(there,yixiforalli).Theaverageisthemeasureofcentraltendencythatwewillfocusoninmostofthistext.However,itissometimesinformativetousethemedian(orsamplemedian)todescribethecentralvalue.Toobtainthemedianofthennumbers{x1,…,xn},wefirstorderthevaluesofthexifromsmallesttolargest.Then,ifnisodd,thesamplemedianisthemiddlenumberoftheorderedobservations.Forexample,giventhenumbers{4,8,2,0,21,10,18},themedianvalueis2(sincetheorderedsequenceis{10,4,0,2,8,18,21}).Ifwechangethelargestnumberinthislist,21,totwiceitsvalue,42,themedianisstill2.Bycontrast,thesampleaveragewouldincreasefrom5to8,asizablechange.Generally,themedianislesssensitivethantheaveragetochangesintheextremevalues(largeorsmall)inalistofnumbers.Thisiswhy“medianincomes”or“medianhousingvalues”areoftenreported,ratherthanaverages,whensummarizingincomeorhousingvaluesinacityorcounty.Ifniseven,thereisnouniquewaytodefinethemedianbecausetherearetwonum-bersatthecenter.Usuallythemedianisdefinedtobetheaverageofthetwomiddleval-ues(again,afterorderingthenumbersfromsmallesttolargest).Usingthisrule,themedianforthesetofnumbers{4,12,2,6}wouldbe(46)/25.A.2PROPERTIESOFLINEARFUNCTIONSLinearfunctionsplayanimportantroleineconometricsbecausetheyaresimpletointerpretandmanipulate.Ifxandyaretwovariablesrelatedby645\nxd7/14/998:51PMPage646AppendixABasicMathematicalToolsy01x,(A.9)thenwesaythatyisalinearfunctionofx,and0and1aretwoparameters(num-bers)describingthisrelationship.Theinterceptis0,andtheslopeis1.Thedefiningfeatureofalinearfunctionisthatthechangeinyisalways1timesthechangeinx:y1x,(A.10)wheredenotes“change.”Inotherwords,themarginaleffectofxonyisconstantandequalto1.EXAMPLEA.1(LinearHousingExpenditureFunction)Supposethattherelationshipbetweenmonthlyhousingexpenditureandmonthlyin-comeishousing164.27income.(A.11)Then,foreachadditionaldollarofincome,27centsisspentonhousing.Iffamilyincomeincreasesby$200,thenhousingexpenditureincreasesby(.27)200$54.ThisfunctionisgraphedinFigureA.1.Accordingtoequation(A.11),afamilywithnoincomespends$164onhousing,whichofcoursecannotbeliterallytrue.Forlowlevelsofincome,thislinearfunctionwouldnotdescribetherelationshipbetweenhousingandincomeverywell,whichiswhywewilleventuallyhavetouseothertypesoffunctionstodescribesuchrelationships.In(A.11),themarginalpropensitytoconsume(MPC)housingoutofincomeis.27.Thisisdifferentfromtheaveragepropensitytoconsume(APC),whichishousing164/income.27.incomeTheAPCisnotconstant,itisalwayslargerthantheMPC,anditgetsclosertotheMPCasincomeincreases.Linearfunctionsareeasilydefinedformorethantwovariables.Supposethatyisrelatedtotwovariables,x1andx2,inthegeneralformy01x12x2.(A.12)Itisratherdifficulttoenvisionthisfunctionbecauseitsgraphisthree-dimensional.Nevertheless,0isstilltheintercept(thevalueofywhenx10andx20),and1and2measureparticularslopes.From(A.12),thechangeiny,forgivenchangesinx1andx2,is646\nxd7/14/998:51PMPage647AppendixABasicMathematicalToolsFigureA.1Graphofhousing164.27income.housinghousing=.271,514income1645,000incomey1x12x2.(A.13)Ifx2doesnotchange,thatis,x20,thenwehavey1x1ifx20,sothat1istheslopeoftherelationshipinthedirectionofx1:y1ifx20.x1Becauseitmeasureshowychangeswithx1,holdingx2fixed,1isoftencalledthepar-tialeffectofx1ony.Sincethepartialeffectinvolvesholdingotherfactorsfixed,itiscloselylinkedtothenotionofceterisparibus.Theparameter2hasasimilarinterpre-tation:2y/x2ifx10,sothat2isthepartialeffectofx2ony.EXAMPLEA.2(DemandforCompactDiscs)Forcollegestudents,supposethatthemonthlyquantitydemandedofcompactdiscsisrelatedtothepriceofcompactdiscsandmonthlydiscretionaryincomeby647\nxd7/14/998:51PMPage648AppendixABasicMathematicalToolsFigureA.2Graphofquantity1209.8price.03income,withincomefixedat$900.quantity147quantity=–9.8price15pricequantity1209.8price.03income,wherepriceisdollarsperdiskandincomeismeasuredindollars.Thedemandcurveistherelationshipbetweenquantityandprice,holdingincome(andotherfactors)fixed.ThisisgraphedintwodimensionsinFigureA.2atanincomelevelof$900.Theslopeofthedemandcurve,9.8,isthepartialeffectofpriceonquantity:holdingincomefixed,ifthepriceofcompactdiscsincreasesbyonedollar,thenthequantitydemandedfallsby9.8.(WeabstractfromthefactthatCDscanonlybepurchasedindiscreteunits.)Anincreaseinincomesimplyshiftsthedemandcurveup(changestheintercept),butthesloperemainsthesame.A.3PROPORTIONSANDPERCENTAGESProportionsandpercentagesplaysuchanimportantroleinappliedeconomicsthatitisnecessarytobecomeverycomfortableinworkingwiththem.Manyquantitiesreportedinthepopularpressareintheformofpercentages;afewexamplesincludeinterestrates,unemploymentrates,andhighschoolgraduationrates.648\nxd7/14/998:51PMPage649AppendixABasicMathematicalToolsAnimportantskillisbeingabletoconvertbetweenproportionsandpercentages.Apercentageiseasilyobtainedbymultiplyingaproportionby100.Forexample,iftheproportionofadultsinacountywithahighschooldegreeis.82,thenwesaythat82%(82percent)ofadultshaveahighschooldegree.Anotherwaytothinkofpercentsandproportionsisthataproportionisthedecimalformofapercent.Forexample,ifthemarginaltaxrateforafamilyearning$30,000peryearisreportedas28%,thenthepro-portionofthenextdollarofincomethatispaidinincometaxesis.28(or28cents).Whenusingpercentages,weoftenneedtoconvertthemtodecimalform.Forexam-ple,ifastatesalestaxis6%and$200isspentonataxableitem,thenthesalestaxpaidis200(.06)12dollars.Iftheannualreturnonacertificateofdeposit(CD)is7.6%andweinvest$3,000insuchaCDatthebeginningoftheyear,thenourinterestincomeis3,000(.076)228dollars.Asmuchaswewouldlikeit,theinterestincomeisnotobtainedbymultiplying3,000by7.6.Wemustbewaryofproportionsthataresometimesincorrectlyreportedasper-centagesinthepopularmedia.Ifweread,“Thepercentageofhighschoolstudentswhodrinkalcoholis.57,”weknowthatthisreallymeans57%(notjustoverone-halfofapercent,asthestatementliterallyimplies).Collegevolleyballfansareprobablyfamil-iarwithpressclipscontainingstatementssuchas“Herhittingpercentagewas.372.”Thisreallymeansthatherhittingpercentagewas37.2%.Ineconometrics,weareofteninterestedinmeasuringthechangesinvariousquan-tities.Letxdenotesomevariable,suchasanindividual’sincome,thenumberofcrimescommittedinacommunity,ortheprofitsofafirm.Letx0andx1denotetwovaluesforx:x0istheinitialvalue,andx1isthesubsequentvalue.Forexample,x0couldbetheannualincomeofanindividualin1994andx1theincomeofthesameindividualin1995.Theproportionatechangeinxinmovingfromx0tox1issimply(x1x0)/x0x/x0,(A.14)assuming,ofcourse,thatx00.Inotherwords,togettheproportionatechange,wesimplydividethechangeinxbyitsinitialvalue.Thisisawayofstandardizingthechangesothatitisfreeofunits.Forexample,ifanindividual’sincomegoesfrom$30,000peryearto$36,000peryear,thentheproportionatechangeis6,000/30,000.20.Itismorecommontostatechangesintermsofpercentages.Thepercentagechangeinxingoingfromx0tox1issimply100timestheproportionatechange:%x100(x/x0);(A.15)thenotation“%x”isreadas“thepercentagechangeinx.”Forexample,whenincomegoesfrom$30,000to$33,750,incomehasincreasedby12.5%;togetthis,wesimplymultiplytheproportionatechange,.125,by100.Again,wemustbeonguardforproportionatechangesthatarereportedaspercent-agechanges.Inthepreviousexample,forinstance,reportingthepercentagechangeinincomeas.125isincorrectandcouldleadtoconfusion.Whenwelookatchangesinthingslikedollaramountsorpopulation,thereisnoambiguityaboutwhatismeantbyapercentagechange.Bycontrast,interpretingper-649\nxd7/14/998:51PMPage650AppendixABasicMathematicalToolscentagechangecalculationscanbetrickywhenthevariableofinterestisitselfaper-centage,somethingthathappensoftenineconomicsandothersocialsciences.Toillus-trate,letxdenotethepercentageofadultsinaparticularcityhavingacollegeeducation.Supposetheinitialvalueisx024(24%haveacollegeeducation),andthenewvalueisx130.Therearetwoquantitieswecancomputetodescribehowtheper-centageofcollege-educatedpeoplehaschanged.Thefirstisthechangeinx,x.Inthiscase,xx1x06:thepercentageofpeoplewithacollegeeducationhasincreasedbysixpercentagepoints.Ontheotherhand,wecancomputethepercentagechangeinxusingequation(A.15):%x100[(3024)/24]25.Inthisexample,thepercentagepointchangeandthepercentagechangeareverydifferent.Thepercentagepointchangeisjustthechangeinthepercentages.Theper-centagechangeisthechangerelativetotheinitialvalue.Generally,wemustpaycloseattentiontowhichnumberisbeingcomputed.Thecarefulresearchermakesthisdis-tinctionperfectlyclear;unfortunately,inthepopularpressaswellasinacademicresearch,thetypeofreportedchangeisoftenunclear.EXAMPLEA.3(MichiganSalesTaxIncrease)InMarch1994,Michiganvotersapprovedasalestaxincreasefrom4%to6%.Inpoliticaladvertisements,supportersofthemeasurereferredtothisasatwopercentagepointincrease,oranincreaseoftwocentsonthedollar.Opponentstothetaxincreasecalledita50%increaseinthesalestaxrate.Bothclaimsarecorrect;theyaresimplydifferentwaysofmeasuringtheincreaseinthesalestax.Naturally,eachgroupreportedthemeasurethatmadetheirpositionmostfavorable.Foravariablesuchassalary,itmakesnosensetotalkofa“percentagepointchangeinsalary”becausesalaryisnotmeasuredasapercentage.Wecandescribeachangeinsalaryeitherindollarorpercentageterms.A.4SOMESPECIALFUNCTIONSANDTHEIRPROPERTIESInSectionA.2,wereviewedthebasicpropertiesoflinearfunctions.Wealreadyindi-catedoneimportantfeatureoffunctionslikey01x:aone-unitchangeinxresultsinthesamechangeiny,regardlessoftheinitialvalueofx.Aswenotedearlier,thisisthesameassayingthemarginaleffectofxonyisconstant,somethingthatisnotrealisticformanyeconomicrelationships.Forexample,theimportanteconomicnotionofdiminishingmarginalreturnsisnotconsistentwithalinearrelationship.Inordertomodelavarietyofeconomicphenomena,weneedtostudyseveralnon-linearfunctions.Anonlinearfunctionischaracterizedbythefactthatthechangeinyforagivenchangeinxdependsonthestartingvalueofx.Certainnonlinearfunctionsappearfrequentlyinempiricaleconomics,soitisimportanttoknowhowtointerpretthem.Acompleteunderstandingofnonlinearfunctionstakesusintotherealmofcal-650\nxd7/14/998:51PMPage651AppendixABasicMathematicalToolsculus.Here,wesimplysummarizethemostsignificantaspectsofthefunctions,leav-ingthedetailsofsomederivationsforSectionA.5.QuadraticFunctionsOnesimplewaytocapturediminishingreturnsistoaddaquadratictermtoalinearrelationship.Considertheequation2y01x2x,(A.16)where0,1,and2areparameters.When10and20,therelationshipbetweenyandxhastheparabolicshapegiveninFigureA.3,where06,18,and22.When10and20,itcanbeshown(usingcalculusinthenextsection)thatthemaximumofthefunctionoccursatthepointx*1/(22).(A.17)2Forexample,ify68x2x(so18,22),thenthelargestvalueofy2occursatx*8/42,andthisvalueis68(2)2(2)14(seeFigureA.3).FigureA.32Graphofy68x2x.y1412108642001234xx*651\nxd7/14/998:51PMPage652AppendixABasicMathematicalToolsThefactthatequation(A.16)impliesadiminishingmarginaleffectofxonyiseasilyseenfromitsgraph.Supposewestartatalowvalueofxandthenincreasexbysomeamount,sayc.Thishasalargereffectonythanifwestartatahighervalueofxandincreasexbythesameamountc.Infact,oncexx*,anincreaseinxactuallydecreasesy.ThestatementthatxhasadiminishingmarginaleffectonyisthesameassayingthattheslopeofthefunctioninFigureA.3decreasesasxincreases.Whilethisisclearfromlookingatthegraph,weusuallywanttoquantifyhowquicklytheslopeischang-ing.Anapplicationofcalculusgivestheapproximateslopeofthequadraticfunctionasyslope122x,(A.18)xfor“small”changesinx.[Theright-handsideofequation(A.18)isthederivativeofthefunctioninequation(A.16)withrespecttox.]Anotherwaytowritethisisy(122x)xfor“small”x.(A.19)Toseehowwellthisapproximationworks,consideragainthefunctiony68x22x.Then,accordingtoequation(A.19),y(84x)x.Now,supposewestartatx1andchangexbyx.1.Using(A.19),y(84)(.1).4.Ofcourse,wecancomputethechangeexactlybyfindingthevaluesofywhenx1andx1.1:22y068(1)2(1)12andy168(1.1)2(1.1)12.38,andsotheexactchangeinyis.38.Theapproximationisprettycloseinthiscase.Now,supposewestartatx1butchangexbyalargeramount:x.5.Then,theapproximationgivesy4(.5)2.Theexactchangeisdeterminedbyfindingthedif-ferenceinywhenx1andx1.5.Theformervalueofywas12,andthelattervalue2is68(1.5)2(1.5)13.5,sotheactualchangeis1.5(not2).Theapproximationisworseinthiscasebecausethechangeinxislarger.Formanyapplications,equation(A.19)canbeusedtocomputetheapproximatemarginaleffectofxonyforanyinitialvalueofxandsmallchanges.And,wecanalwayscomputetheexactchangeifnecessary.EXAMPLEA.4(AQuadraticWageFunction)Supposetherelationshipbetweenhourlywagesandyearsintheworkforce(exper)isgivenby2wage5.25.48exper.008exper.(A.20)ThisfunctionhasthesamegeneralshapeastheoneinFigureA.3.Usingequation(A.17),experhasapositiveeffectonwageuptotheturningpoint,exper*.48/[2(.008)]30.Thefirstyearofexperienceisworthapproximately.48,or48cents[see(A.19)withx0,x1].Eachadditionalyearofexperienceincreaseswagebylessthantheprevious652\nxd7/14/998:51PMPage653AppendixABasicMathematicalToolsyear—reflectingadiminishingmarginalreturntoexperience.At30years,anadditionalyearofexperiencewouldactuallylowerthewage.Thisisnotveryrealistic,butitisoneoftheconsequencesofusingaquadraticfunctiontocaptureadiminishingmarginaleffect:atsomepoint,thefunctionmustreachamaximumandcurvedownward.Forpracticalpurposes,thepointatwhichthishappensisoftenlargeenoughtobeinconse-quential,butnotalways.Thegraphofthequadraticfunctionin(A.16)hasaU-shapeif10and20,inwhichcasethereisanincreasingmarginalreturn.Theminimumofthefunctionisatthepoint1/(22).TheNaturalLogarithmThenonlinearfunctionthatplaysthemostimportantroleineconometricanalysisisthenaturallogarithm.Inthistext,wedenotethenaturallogarithm,whichweoftenrefertosimplyasthelogfunction,asylog(x).(A.21)Youmightrememberlearningdifferentsymbolsforthenaturallog;ln(x)orloge(x)arethemostcommon.Thesedifferentnotationsareusefulwhenlogarithmswithseveraldifferentbasesarebeingused.Forourpurposes,onlythenaturallogarithmisimpor-tant,andsolog(x)denotesthenaturallogarithmthroughoutthistext.Thiscorrespondstothenotationusageinmanystatisticalpackages,althoughsomeuseln(x)[andmostcalculatorsuseln(x)].Economistsusebothlog(x)andln(x),whichisusefultoknowwhenyouarereadingpapersinappliedeconomics.Thefunctionylog(x)isdefinedonlyforx0,anditisplottedinFigureA.4.Itisnotveryimportanttoknowhowthevaluesoflog(x)areobtained.Forourpurposes,thefunctioncanbethoughtofasablackbox:wecanpluginanyx0andobtainlog(x)fromacalculatororacomputer.SeveralthingsareapparentfromFigureA.4.First,whenylog(x),therelationshipbetweenyandxdisplaysdiminishingmarginalreturns.OneimportantdifferencebetweenthelogandthequadraticfunctioninFigureA.3isthatwhenylog(x),theeffectofxonyneverbecomesnegative:theslopeofthefunctiongetscloserandclosertozeroasxgetslarge,buttheslopeneverquitereacheszeroandcertainlyneverbecomesnegative.ThefollowingarealsoapparentfromFigureA.4:log(x)0for0x1log(1)0log(x)0forx1.Inparticular,log(x)canbepositiveornegative.Someusefulalgebraicfactsaboutthelogfunctionare653\nxd7/14/998:51PMPage654AppendixABasicMathematicalToolsFigureA.4Graphofylog(x).yy=log(x)01xlog(x1x2)log(x1)log(x2),x1,x20log(x1/x2)log(x1)log(x2),x1,x20clog(x)clog(x),x0,canynumber.Occasionally,wewillneedtorelyontheseproperties.Thelogarithmcanbeusedforvariousapproximationsthatariseineconometricapplications.First,log(1x)xforx0.Youcantrythiswithx.02,.1,and.5toseehowthequalityoftheapproximationdeterioratesasxgetslarger.Evenmoreuse-fulisthefactthatthedifferenceinlogscanbeusedtoapproximateproportionatechanges.Letx0andx1bepositivevalues.Then,itcanbeshown(usingcalculus)thatlog(x1)log(x0)(x1x0)/x0x/x0(A.22)forsmallchangesinx.Ifwemultiplyequation(A.22)by100andwritelog(x)log(x1)log(x0),then100log(x)%x(A.23)forsmallchangesinx.Themeaningofsmalldependsonthecontext,andwewillencounterseveralexamplesthroughoutthistext.Whyshouldweapproximatethepercentagechangeusing(A.23)whentheexactpercentagechangeissoeasytocompute?Momentarily,wewillseewhytheapproxi-654\nxd7/14/998:51PMPage655AppendixABasicMathematicalToolsmationin(A.23)isusefulineconometrics.First,letusseehowgoodtheapproxima-tionisintwoexamples.First,supposex040andx141.Then,thepercentagechangeinxinmovingfromx0tox1is2.5%,using100(x1x0)/x0.Now,log(41)log(40).0247tofourdigits,whichwhenmultipliedby100isverycloseto2.5.Theapproximationworksprettywell.Now,consideramuchbiggerchange:x040andx160.Theexactper-centagechangeis50%.However,log(60)log(40).4055,sotheapproximationgives40.55%,whichismuchfartheroff.Whyistheapproximationin(A.23)usefulifitisonlysatisfactoryforsmallchanges?Tobuilduptotheanswer,wefirstdefinetheelasticityofywithrespecttoxasyx%y.(A.24)xy%xInotherwords,theelasticityofywithrespecttoxisthepercentagechangeiny,whenxincreasesby1%.Thisnotionshouldbefamiliarfromintroductoryeconomics.Ifyisalinearfunctionofx,y01x,thentheelasticityisyxxx11,(A.25)xyy01xwhichclearlydependsonthevalueofx.(Thisisageneralizationofthewell-knownresultfrombasicdemandtheory:theelasticityisnotconstantalongastraight-linedemandcurve.)Elasticitiesareofcriticalimportanceinmanyareasofappliedeconomics—notjustindemandtheory.Itisconvenientinmanysituationstohaveconstantelasticitymod-els,andthelogfunctionallowsustospecifysuchmodels.Ifweusetheapproximation(A.23)forbothxandy,thentheelasticityisapproximatelyequaltolog(y)/log(x).Thus,aconstantelasticitymodelisapproximatedbytheequationlog(y)01log(x),(A.26)and1istheelasticityofywithrespecttox(assumingthatx,y0).EXAMPLEA.5(ConstantElasticityDemandFunction)Ifqisquantitydemandedandpisprice,andthesevariablesarerelatedbylog(q)4.71.25log(p),thenthepriceelasticityofdemandis1.25.Roughly,a1%increaseinpriceleadstoa1.25%fallinthequantitydemanded.655\nxd7/14/998:51PMPage656AppendixABasicMathematicalToolsForourpurposes,thefactthat1in(A.26)isonlyclosetotheelasticityisnotimpor-tant.Infact,whentheelasticityisdefinedusingcalculus—asinSectionA.5—thedefi-nitionisexact.Forthepurposesofeconometricanalysis,(A.26)definesaconstantelasticitymodel.Suchmodelsplayalargeroleinempiricaleconomics.Thereareotherpossibilitiesforusingthelogfunctionthatoftenariseinempiricalwork.Supposethaty0,andlog(y)01x.(A.27)Thenlog(y)1x,so100log(y)(1001)x.Itfollowsthat,whenyandxarerelatedbyequation(A.27),%y(1001)x.(A.28)EXAMPLEA.6(LogarithmicWageEquation)Supposethathourlywageandyearsofeducationarerelatedbylog(wage)2.78.094educ.Then,usingequation(A.28),%wage100(.094)educ9.4educ.Itfollowsthatonemoreyearofeducationincreaseshourlywagebyabout9.4%.Generally,thequantity%y/xiscalledthesemi-elasticityofywithrespecttox.Thesemi-elasticityisthepercentagechangeinywhenxincreasesbyoneunit.Whatwehavejustshownisthat,inmodel(A.27),thesemi-elasticityisconstantandequalto1001.InExampleA.6,wecanconvenientlysummarizetherelationshipbetweenwagesandeducationbysayingthatonemoreyearofeducation—startingfromanyamountofeducation—increasesthewagebyabout9.4%.Thisiswhysuchmodelsplayanimportantroleineconomics.Anotherrelationshipofsomeinterestinappliedeconomicsis:y01log(x),(A.29)wherex0.Howcanweinterpretthisequation?Ifwetakethechangeiny,wegety1log(x),whichcanberewrittenasy(1/100)[100log(x)].Thus,usingtheapproximationin(A.23),wehavey(1/100)(%x).(A.30)Inotherwords,1/100istheunitchangeinywhenxincreasesby1%.656\nxd7/14/998:51PMPage657AppendixABasicMathematicalToolsEXAMPLEA.7(LaborSupplyFunction)Assumethatthelaborsupplyofaworkercanbedescribedbyhours3345.1log(wage),wherewageishourlywageandhoursishoursworkedperweek.Then,from(A.30),hours(45.1/100)(%wage).451%wage.Inotherwords,a1%increaseinwageincreasestheweeklyhoursworkedbyabout.45,orslightlylessthanone-halfofanhour.Ifthewageincreasesby10%,thenhours.451(10)4.51,oraboutfourandone-halfhours.Wewouldnotwanttousethisapprox-imationformuchlargerpercentagechangesinwages.TheExponentialFunctionBeforeleavingthissection,weneedtodiscussonemorespecialfunction,onethatisrelatedtothelog.Asmotivation,considerequation(A.27).There,log(y)isalinearfunctionofx.Buthowdowefindyitselfasafunctionofx?Theanswerisgivenbytheexponentialfunction.Wewillwritetheexponentialfunctionasyexp(x),whichisgraphedinFigureA.5.FigureA.5Graphofyexp(x).yy=exp(x)0x657\nxd7/14/998:51PMPage658AppendixABasicMathematicalToolsFromFigureA.5,weseethatexp(x)isdefinedforanyvalueofxandisalwaysxgreaterthanzero.Sometimestheexponentialfunctioniswrittenasye,butwewillnotusethisnotation.Twoimportantvaluesoftheexponentialfunctionareexp(0)1andexp(1)2.7183(tofourdecimals).Theexponentialfunctionistheinverseofthelogfunctioninthefollowingsense:log[exp(x)]xforallx,andexp[log(x)]xforx0.Inotherwords,thelog“undoes”theexponential,andviceversa.(Thisiswhytheexponentialfunctionissometimescalledtheanti-logfunction.)Inparticular,notethatlog(y)01xisequivalenttoyexp(01x).If10,therelationshipbetweenxandyhasthesameshapeasinFigureA.5.Thus,iflog(y)01xwith10,thenxhasanincreasingmarginaleffectony.InExampleA.6,thismeansthatanotheryearofeducationleadstoalargerchangeinwagethanthepreviousyearofeducation.Twousefulfactsabouttheexponentialfunctionareexp(x1x2)exp(x1)exp(x2)candexp[clog(x)]x.A.5DIFFERENTIALCALCULUSIntheprevioussection,weassertedseveralapproximationsthathavefoundationsincalculus.Letyf(x)forsomefunctionf.Then,forsmallchangesinx,dfyx,(A.31)dxwheredf/dxisthederivativeofthefunctionf,evaluatedattheinitialpointx0.Wealsowritethederivativeasdy/dx.Forexample,ifylog(x),thendy/dx1/x.Using(A.31),withdy/dxevaluatedatx0,wehavey(1/x0)x,orlog(x)x/x0,whichistheapproximationgivenin(A.22).Inapplyingeconometrics,ithelpstorecallthederivativesofahandfuloffunctionsbecauseweusethederivativetodefinetheslopeofafunctionatagivenpoint.Wecanthenuse(A.31)tofindtheapproximatechangeinyforsmallchangesinx.Inthelin-earcase,thederivativeissimplytheslopeoftheline,aswewouldhope:ify01x,thendy/dx1.cc1Ifyx,thendy/dxcx.Thederivativeofasumoftwofunctionsisthesumofthederivatives:d[f(x)g(x)]/dxdf(x)/dxdg(x)/dx.Thederivativeofaconstanttimesanyfunctionisthatsameconstanttimesthederivativeofthefunction:d[cf(x)]/dxc[df(x)/dx].Thesesimplerulesallowustofindderivativesofmorecom-plicatedfunctions.Otherrules,suchastheproduct,quotient,andchainruleswillbefamiliartothosewhohavetakencalculus,butwewillnotreviewthosehere.Somefunctionsthatareoftenusedineconomics,alongwiththeirderiva-tives,are658\nxd7/14/998:51PMPage659AppendixABasicMathematicalTools2y01x2x;dy/dx122x2y01/x;dy/dx1/(x)1/2y01x;dy/dx(1/2)xy01log(x);dy/dx1/xyexp(01x);dy/dx1exp(01x).If00and11inthislastexpression,wegetdy/dxexp(x),whenyexp(x).InSectionA.4,wenotedthatequation(A.26)definesaconstantelasticitymodeldyxwhencalculusisused.Thecalculusdefinitionofelasticityis.Itcanbeshowndxydyxusingpropertiesoflogsandexponentialsthat,when(A.26)holds,1.dxyWhenyisafunctionofmultiplevariables,thenotionofapartialderivativebecomesimportant.Supposethatyf(x1,x2).(A.32)Then,therearetwopartialderivatives,onewithrespecttox1andonewithrespecttox2.yThepartialderivativeofywithrespecttox1,denotedhereby,isjusttheusualderiv-x1yativeof(A.32)withrespecttox1,wherex2istreatedasaconstant.Similarly,isjustx2thederivativeof(A.32)withrespecttox2,holdingx1fixed.Partialderivativesareusefulformuchthesamereasonasordinaryderivatives.Wecanapproximatethechangeinyasyyx1,holdingx2fixed.(A.33)x1Thus,calculusallowsustodefinepartialeffectsinnonlinearmodelsjustaswecouldinlinearmodels.Infact,ify01x12x2,thenyy1,2.x1x2ThesecanberecognizedasthepartialeffectsdefinedinSectionA.2.Amorecomplicatedexampleis2y54x1x13x27x1x2.(A.34)Now,thederivativeof(A.34),withrespecttox1(treatingx2asaconstant),issimplyy42x17x2;x1659\nxd7/14/998:51PMPage660AppendixABasicMathematicalToolsynotehowthisdependsonx1andx2.Thederivativeof(A.34),withrespecttox2,isx237x1,sothisdependsonlyonx1.EXAMPLEA.8(WageFunctionwithInteraction)Afunctionrelatingwagestoyearsofeducationandexperienceis2wage3.10.41educ.19exper.004exper(A.35).007educexper.Thepartialeffectofexperonwageisthepartialderivativeof(A.35):wage.19.008exper.007educ.experThisistheapproximatechangeinwageduetoincreasingexperiencebyoneyear.Noticethatthispartialeffectdependsontheinitiallevelofexperandeduc.Forexample,foraworkerwhoisstartingwitheduc12andexper5,thenextyearofexperienceincreaseswagebyabout.19.008(5).007(12).234,or23.4centsperhour.Theexactchangecanbecal-culatedbycomputing(A.35)atexper5,educ12andatexper6,educ12,andthentakingthedifference.Thisturnsouttobe.23,whichisveryclosetotheapproximation.Differentialcalculusplaysanimportantroleinminimizingandmaximizingfunc-tionsofoneormorevariables.Iff(x1,x2,…,xk)isadifferentiablefunctionofkvari-ables,thenanecessaryconditionforx*1,x*2,…,x*ktoeitherminimizeormaximizefoverallpossiblevaluesofxjisf(x*1,x*2,…,x*k)0,j1,2,…,k.(A.36)xjInotherwords,allofthepartialderivativesoffmustbezerowhentheyareevaluatedatthex*h.Thesearecalledthefirstorderconditionsforminimizingormaximizingafunction.Practically,wehopetosolveequation(A.36)forthex*h.Then,wecanuseothercriteriatodeterminewhetherwehaveminimizedormaximizedthefunction.Wewillnotneedthosehere.[SeeSydsaeterandHammond(1995)foradiscussionofmul-tivariablecalculusanditsuseinoptimizingfunctions.]SUMMARYThemathtoolsreviewedherearecrucialforunderstandingregressionanalysisandtheprobabilityandstatisticsthatarecoveredinAppendicesBandC.Thematerialonnon-linearfunctions—especiallyquadratic,logarithmic,andexponentialfunctions—iscrit-icalforunderstandingmodernappliedeconomicresearch.Thelevelofcomprehension660\nxd7/14/998:51PMPage661AppendixABasicMathematicalToolsrequiredofthesefunctionsdoesnotincludeadeepknowledgeofcalculus,althoughcalculusisneededforcertainderivations.KEYTERMSAverageMedianCeterisParibusNaturalLogarithmConstantElasticityModelNonlinearFunctionDerivativePartialDerivativeDescriptiveStatisticPartialEffectDiminishingMarginalEffectPercentageChangeElasticityPercentagePointChangeExponentialFunctionProportionateChangeInterceptSemi-ElasticityLinearFunctionSlopeLogFunctionSummationOperatorMarginalEffectPROBLEMSA.1Thefollowingtablecontainsmonthlyhousingexpendituresfor10families.MonthlyHousingFamilyExpenditures(Dollars)13002440335041,1005640648074508700967010530661\nxd7/14/998:51PMPage662AppendixABasicMathematicalTools(i)Findtheaveragemonthlyhousingexpenditure.(ii)Findthemedianmonthlyhousingexpenditure.(iii)Ifmonthlyhousingexpendituresweremeasuredinhundredsofdollars,ratherthanindollars,whatwouldbetheaverageandmedianexpendi-tures?(iv)Supposethatfamilynumber8increasesitsmonthlyhousingexpendi-tureto$900dollars,buttheexpendituresofallotherfamiliesremainthesame.Computetheaverageandmedianhousingexpenditures.A.2Supposethefollowingequationdescribestherelationshipbetweentheaveragenumberofclassesmissedduringasemester(missed)andthedistancefromschool(dis-tance,measuredinmiles):missed30.2distance.(i)Sketchthisline,beingsuretolabeltheaxes.Howdoyouinterprettheinterceptinthisequation?(ii)Whatistheaveragenumberofclassesmissedforsomeonewholivesfivemilesaway?(iii)Whatisthedifferenceintheaveragenumberofclassesmissedforsomeonewholives10milesawayandsomeonewholives20milesaway?A.3InExampleA.2,quantityofcompactdiskswasrelatedtopriceandincomebyquantity1209.8price.03income.WhatisthedemandforCDsifprice15andincome200?Whatdoesthissuggestaboutusinglinearfunctionstodescribedemandcurves?A.4SupposetheunemploymentrateintheUnitedStatesgoesfrom6.4%inoneyearto5.6%inthenext.(i)Whatisthepercentagepointdecreaseintheunemploymentrate?(ii)Bywhatpercenthastheunemploymentratefallen?A.5Supposethatthereturnfromholdingaparticularfirm’sstockgoesfrom15%inoneyearto18%inthefollowingyear.Themajorityshareholderclaimsthat“thestockreturnonlyincreasedby3%,”whilethechiefexecutiveofficerclaimsthat“thereturnonthefirm’sstockhasincreasedby20%.”Reconciletheirdisagreement.A.6SupposethatPersonAearns$35,000peryearandPersonBearns$42,000.(i)FindtheexactpercentbywhichPersonB’ssalaryexceedsPersonA’s.(ii)Nowusethedifferenceinnaturallogstofindtheapproximatepercent-agedifference.A.7Supposethefollowingmodeldescribestherelationshipbetweenannualsalary(salary)andthenumberofpreviousyearsoflabormarketexperience(exper):log(salary)10.6.027exper.(i)Whatissalarywhenexper0?whenexper5?(Hint:Youwillneedtoexponentiate.)662\nxd7/14/998:51PMPage663AppendixABasicMathematicalTools(ii)Useequation(A.28)toapproximatethepercentageincreaseinsalarywhenexperincreasesbyfiveyears.(iii)Usetheresultsofpart(i)tocomputetheexactpercentagedifferenceinsalarywhenexper5andexper0.Commentonhowthiscompareswiththeapproximationinpart(ii).A.8Letgrthempdenotetheproportionategrowthinemployment,atthecountylevel,from1990to1995,andletsalestaxdenotethecountysalestaxrate,statedasapro-portion.Interprettheinterceptandslopeintheequationgrthemp.043.78salestax.A.9Supposetheyieldofacertaincrop(inbushelsperacre)isrelatedtofertilizeramount(inpoundsperacre)asyield120.19fertilizer.(i)Graphthisrelationshipbyplugginginseveralvaluesforfertilizer.(ii)Describehowtheshapeofthisrelationshipcompareswithalinearfunctionbetweenyieldandfertilizer.663\n\nxd7/14/998:57PMPage664AppendixBFundamentalsofProbabilityhisappendixcoverskeyconceptsfrombasicprobability.AppendicesBandCTareprimarilyforreview;theyarenotintendedtoreplaceacourseinprobabilityandstatistics.Nevertheless,alloftheprobabilityandstatisticsconceptsthatweuseinthetextarecoveredintheseappendices.Probabilityisofinterestinitsownrightforstudentsinbusiness,economics,andothersocialsciences.Forexample,considertheproblemofanairlinetryingtodecidehowmanyreservationstoacceptforaflightthathas100availableseats.Iffewerthan100peoplewantreservations,thentheseshouldallbeaccepted.Butwhatifmorethan100peoplerequestreservations?Asafesolutionistoacceptatmost100reservations.However,sincesomepeoplebookreservationsandthendonotshowupfortheflight,thereissomechancethattheplanewillnotbefullevenif100reservationsarebooked.Thisresultsinlostrevenuetotheairline.Adifferentstrategyistobookmorethan100reservationsandtohopethatsomepeopledonotshowup,andsothefinalnumberofpassengersisascloseto100aspossible.Thispolicyrunstheriskoftheairlinehavingtocompensatepeoplewhoarenecessarilybumpedfromanoverbookedflight.Anaturalquestioninthiscontextis:Canwedecideontheoptimal(orbest)num-berofreservationstheairlineshouldmake?Thisisanontrivialproblem.Nevertheless,givencertaininformation(onairlinecostsandhowfrequentlypeopleshowupforreser-vations),wecanusebasicprobabilitytoarriveatasolution.B.1RANDOMVARIABLESANDTHEIRPROBABILITYDISTRIBUTIONSSupposethatweflipacoin10timesandcountthenumberoftimesthecointurnsupheads.Thisisanexampleofanexperiment.Generally,anexperimentisanyprocedurethatcan,atleastintheory,beinfinitelyrepeated,andhasawell-definedsetofout-comes.Wecould,inprinciple,carryoutthecoin-flippingprocedureagainandagain.Beforeweflipthecoin,weknowthatthenumberofheadsappearingisanintegerfrom0to10,sotheoutcomesoftheexperimentarewell-defined.Arandomvariableisonethattakesonnumericalvaluesandhasanoutcomethatisdeterminedbyanexperiment.Inthecoin-flippingexample,thenumberofheadsappearingin10flipsofacoinisanexampleofarandomvariable.Beforeweflipthe664\nxd7/14/998:57PMPage665AppendixBFundamentalsofProbabilitycoin10times,wedonotknowhowmanytimesthecoinwillcomeupheads.Onceweflipthecoin10timesandcountthenumberofheads,weobtaintheoutcomeoftheran-domvariableforthisparticulartrialoftheexperiment.Anothertrialcanproduceadif-ferentoutcome.Intheairlinereservationexamplementionedearlier,thenumberofpeopleshowingupfortheirflightisarandomvariable:beforeanyparticularflight,wedonotknowhowmanypeoplewillshowup.Toanalyzedatacollectedinbusinessandthesocialsciences,itisimportanttohaveabasicunderstandingofrandomvariablesandtheirproperties.FollowingtheusualconventionsinprobabilityandstatisticsthroughoutAppendicesBandC,wedenoterandomvariablesbyuppercaseletters,usuallyW,X,Y,andZ;particularoutcomesofrandomvariablesaredenotedbythecorrespondinglowercaseletters,w,x,y,andz.Forexample,inthecoin-flippingexperiment,letXdenotethenumberofheadsappearingin10flipsofacoin.Then,Xisnotassociatedwithanyparticularvalue,butweknowXwilltakeonavalueintheset{0,1,2,…,10}.Aparticularoutcomeis,say,x6.Weindicatelargecollectionsofrandomvariablesbyusingsubscripts.Forexample,ifwerecordlastyear’sincomeof20randomlychosenhouseholdsintheUnitedStates,wemightdenotetheserandomvariablesbyX1,X2,…,X20;theparticularoutcomeswouldbedenotedx1,x2,…,x20.Asstatedinthedefinition,randomvariablesarealwaysdefinedtotakeonnumeri-calvalues,evenwhentheydescribequalitativeevents.Forexample,considertossingasinglecoin,wherethetwooutcomesareheadsandtails.Wecandefinearandomvari-ableasfollows:X1ifthecointurnsupheads,andX0ifthecointurnsuptails.ArandomvariablethatcanonlytakeonthevalueszeroandoneiscalledaBernoulli(orbinary)randomvariable.Inbasicprobability,itistraditionaltocalltheeventX1a“success”andtheeventX0a“failure.”Foraparticularapplication,thesuccess-failurenomenclaturemightnotcorrespondtoournotionofasuccessorfailure,butitisausefulterminologythatwewilladopt.DiscreteRandomVariablesAdiscreterandomvariableisonethattakesononlyafiniteorcountablyinfinitenumberofvalues.Thenotionof“countablyinfinite”meansthateventhoughaninfinitenumberofvaluescanbetakenonbyarandomvariable,thosevaluescanbeputinaone-to-onecorrespondencewiththepositiveintegers.Becausethedistinctionbetween“countablyinfinite”and“uncountablyinfinite”issomewhatsubtle,wewillconcentrateondiscreterandomvariablesthattakeononlyafinitenumberofvalues.LarsenandMarx(1986,Chapter3)containsadetailedtreatment.ABernoullirandomvariableisthesimplestexampleofadiscreterandomvariable.TheonlythingweneedtocompletelydescribethebehaviorofaBernoullirandomvari-ableistheprobabilitythatittakesonthevalueone.Inthecoin-flippingexample,ifthecoinis“fair,”thenP(X1)1/2(readas“theprobabilitythatXequalsoneisone-half”).Becauseprobabilitiesmustsumtoone,P(X0)1/2,also.Socialscientistsareinterestedinmorethanflippingcoins,sowemustallowformoregeneralsituations.Again,considertheexamplewheretheairlinemustdecidehowmanypeopletobookforaflightwith100availableseats.Thisproblemcanbeanalyzed665\nxd7/14/998:57PMPage666AppendixBFundamentalsofProbabilityinthecontextofseveralBernoullirandomvariablesasfollows:forarandomlyselectedcustomer,defineaBernoullirandomvariableasX1ifthepersonshowsupforthereservation,andX0ifnot.Thereisnoreasontothinkthattheprobabilityofanyparticularcustomershowingupis1/2;inprinciple,theprobabilitycanbeanynumberbetweenzeroandone.Callthisnumber,sothatP(X1)(B.1)P(X0)1.(B.2)Forexample,if.75,thenthereisa75%chancethatacustomershowsupaftermak-ingareservation,anda25%chancethatthecustomerdoesnotshowup.Intuitively,thevalueofiscrucialindeterminingtheairline’sstrategyforbookingreservations.Methodsforestimating,givenhistoricaldataonairlinereservations,isasubjectofmathematicalstatistics,somethingweturntoinAppendixC.Moregenerally,anydiscreterandomvariableiscompletelydescribedbylistingitspossiblevaluesandtheassociatedprobabilitythatittakesoneachvalue.IfXtakesonthekpossiblevalues{x1,…,xk},thentheprobabilitiesp1,p2,…,pkaredefinedbypjP(Xxj),j1,2,…,k,(B.3)whereeachpjisbetween0and1,andp1p2…pk1.(B.4)Equation(B.3)isreadas:“TheprobabilitythatXtakesonthevaluexjisequaltopj.”Equations(B.1)and(B.2)showthattheprobabilitiesofsuccessandfailureforaBernoullirandomvariablearedeterminedentirelybythevalueof.BecauseBernoullirandomvariablesaresoprevalent,wehaveaspecialnotationforthem:X~Bernoulli()isreadas“XhasaBernoullidistributionwithprobabilityofsuccessequalto.”Theprobabilitydensityfunction(pdf)ofXsummarizestheinformationconcern-ingthepossibleoutcomesofXandthecorrespondingprobabilities:f(xj)pj,j1,2,…,k,(B.5)withf(x)0foranyxnotequaltoxjforsomej.Inotherwords,foranyrealnumberx,f(x)istheprobabilitythattherandomvariableXtakesontheparticularvaluex.Whendealingwithmorethanonerandomvariable,itissometimesusefultosubscriptthepdfinquestion:fXisthepdfofX,fYisthepdfofY,andsoon.Giventhepdfofanydiscreterandomvariable,itissimpletocomputetheproba-bilityofanyeventinvolvingthatrandomvariable.Forexample,supposethatXisthenumberoffreethrowsmadebyabasketballplayeroutoftwoattempts,sothatXcantakeonthethreevalues{0,1,2}.AssumethatthepdfofXisgivenbyf(0).20,f(1).44,andf(2).36.666\nxd7/14/998:57PMPage667AppendixBFundamentalsofProbabilityThethreeprobabilitiessumtoone,astheymust.Usingthispdf,wecancalculatetheprobabilitythattheplayermakesatleastonefreethrow:P(X1)P(X1)P(X2).44.36.80.ThepdfofXisshowninFigureB.1.ContinuousRandomVariablesAvariableXisacontinuousrandomvariableifittakesonanyrealvaluewithzeroprobability.Thisdefinitionissomewhatcounterintuitive,sinceinanyapplication,weeventuallyobservesomeoutcomeforarandomvariable.TheideaisthatacontinuousrandomvariableXcantakeonsomanypossiblevaluesthatwecannotcountthemormatchthemupwiththepositiveintegers,sologicalconsistencydictatesthatXcantakeoneachvaluewithprobabilityzero.Whilemeasurementsarealwaysdiscreteinprac-tice,randomvariablesthattakeonnumerousvaluesarebesttreatedascontinuous.Forexample,themostrefinedmeasureofthepriceofagoodisintermsofcents.Wecanimaginelistingallpossiblevaluesofpriceinorder(eventhoughthelistmaycontinueindefinitely),whichtechnicallymakespriceadiscreterandomvariable.However,therearesomanypossiblevaluesofpricethatusingthemechanicsofdiscreterandomvari-ablesisnotfeasible.Wecandefineaprobabilitydensityfunctionforcontinuousrandomvariables,and,aswithdiscreterandomvariables,thepdfprovidesinformationonthelikelyoutcomesoftherandomvariable.However,becauseitmakesnosensetodiscusstheprobabilitythatacontinuousrandomvariabletakesonaparticularvalue,weusethepdfofacon-FigureB.1Thepdfofthenumberoffreethrowsmadeoutoftwoattempts.f(x).44.36.20012x667\nxd7/14/998:57PMPage668AppendixBFundamentalsofProbabilitytinuousrvonlytocomputeeventsinvolvingarangeofvalues.Forexample,ifaandbareconstantswhereab,theprobabilitythatXliesbetweenthenumbersaandb,P(aXb),istheareaunderthepdfbetweenpointsaandb,asshowninFigureB.2.Ifyouarefamiliarwithcalculus,yourecognizethisastheintegralofthefunctionfbetweenthepointsaandb.Theentireareaunderthepdfmustalwaysequalone.Whencomputingprobabilitiesforcontinuousrandomvariables,itiseasiesttoworkwiththecumulativedistributionfunction(cdf).IfXisanyrandomvariable,thenitscdfisdefinedforanyrealnumberxbyF(x)P(Xx).(B.6)Fordiscreterandomvariables,(B.6)isobtainedbysummingthepdfoverallvaluesxjsuchthatxjx.Foracontinuousrandomvariable,F(x)istheareaunderthepdf,f,totheleftofthepointx.SinceF(x)issimplyaprobability,itisalwaysbetween0and1.Further,ifx1x2,thenP(Xx1)P(Xx2),thatis,F(x1)F(x2).Thismeansthatacdfisanincreasing(oratleastnondecreasing)functionofx.Twoimportantpropertiesofcdfsthatareusefulforcomputingprobabilitiesarethefollowing:FigureB.2TheprobabilitythatXliesbetweenthepointsaandb.f(x)abx668\nxd7/14/998:57PMPage669AppendixBFundamentalsofProbabilityForanynumberc,P(Xc)1F(c).(B.7)Foranynumbersab,P(aXb)F(b)F(a).(B.8)Inourstudyofeconometrics,wewillusecdfstocomputeprobabilitiesonlyforcon-tinuousrandomvariables,inwhichcaseitdoesnotmatterwhetherinequalitiesinprob-abilitystatementsarestrictornot.Thatis,foracontinuousrandomvariableX,P(Xc)P(Xc),(B.9)andP(aXb)P(aXb)P(aXb)P(aXb).(B.10)Combinedwith(B.7)and(B.8),equations(B.9)and(B.10)greatlyexpandtheproba-bilitycalculationsthatcanbedoneusingcontinuouscdfs.Cumulativedistributionfunctionshavebeentabulatedforalloftheimportantcontinuousdistributionsinprobabilityandstatistics.Themostwell-knownoftheseisthenormaldistribution,whichwecoveralongwithsomerelateddistributionsinSectionB.5.B.2JOINTDISTRIBUTIONS,CONDITIONALDISTRIBUTIONS,ANDINDEPENDENCEIneconomics,weareusuallyinterestedintheoccurrenceofeventsinvolvingmorethanonerandomvariable.Forexample,intheairlinereservationexamplereferredtoearlier,theairlinemightbeinterestedintheprobabilitythatapersonwhomakesareservationshowsupandisabusinesstraveler;thisisanexampleofajointprobability.Or,theair-linemightbeinterestedinthefollowingconditionalprobability:conditionalontheper-sonbeingabusinesstraveler,whatistheprobabilityofheorsheshowingup?Inthenexttwosubsections,weformalizethenotionsofjointandconditionaldistributionsandtheimportantnotionofindependenceofrandomvariables.JointDistributionsandIndependenceLetXandYbediscreterandomvariables.Then,(X,Y)haveajointdistribution,whichisfullydescribedbythejointprobabilitydensityfunctionof(X,Y):fX,Y(x,y)P(Xx,Yy),(B.11)wheretheright-handsideistheprobabilitythatXxandYy.WhenXandYarecontinuous,ajointpdfcanalsobedefined,butwewillnotcoversuchdetailsbecausejointpdfsforcontinuousrandomvariablesarenotusedexplicitlyinthistext.Inonecase,itiseasytoobtainthejointpdfifwearegiventhepdfsofXandY.Inparticular,randomvariablesXandYaresaidtobeindependentifandonlyif669\nxd7/14/998:57PMPage670AppendixBFundamentalsofProbabilityfX,Y(x,y)fX(x)fY(y)(B.12)forallxandy,wherefXisthepdfofX,andfYisthepdfofY.Inthecontextofmorethanonerandomvariable,thepdfsfXandfYareoftencalledmarginalprobabilityden-sityfunctionstodistinguishthemfromthejointpdffX,Y.Thisdefinitionofindepen-denceisvalidfordiscreteandcontinuousrandomvariables.Tounderstandthemeaningof(B.12),itiseasiesttodealwiththediscretecase.IfXandYarediscrete,then(B.12)isthesameasP(Xx,Yy)P(Xx)P(Yy);(B.13)inotherwords,theprobabilitythatXxandYyistheproductofthetwoproba-bilitiesP(Xx)andP(Yy).Oneimplicationof(B.13)isthatjointprobabilitiesarefairlyeasytocompute,sincetheyonlyrequireknowledgeofP(Xx)andP(Yy).Ifrandomvariablesarenotindependent,thentheyaresaidtobedependent.EXAMPLEB.1(FreeThrowShooting)Considerabasketballplayershootingtwofreethrows.LetXbetheBernoullirandomvari-ableequaltooneifsheorhemakesthefirstfreethrow,andzerootherwise.LetYbeaBernoullirandomvariableequaltooneifheorshemakesthesecondfreethrow.Supposethatsheorheisan80%free-throwshooter,sothatP(X1)P(Y1).8.Whatistheprobabilityoftheplayermakingbothfreethrows?IfXandYareindependent,wecaneasilyanswerthisquestion:P(X1,Y1)P(X1)P(Y1)(.8)(.8).64.Thus,thereisa64%chanceofmakingbothfreethrows.Ifthechanceofmakingthesecondfreethrowdependsonwhetherthefirstwasmade—thatis,XandYarenotindependent—thenthissimplecalculationisnotvalid.Independenceofrandomvariablesisaveryimportantconcept.Inthenextsubsec-tion,wewillshowthatifXandYareindependent,thenknowingtheoutcomeofXdoesnotchangetheprobabilitiesofthepossibleoutcomesofY,andviceversa.OneusefulfactaboutindependenceisthatifXandYareindependentandwedefinenewrandomvariablesg(X)andh(Y)foranyfunctionsgandh,thenthesenewrandomvariablesarealsoindependent.Thereisnoneedtostopattworandomvariables.IfX1,X2,…,Xnarediscreteran-domvariables,thentheirjointpdfisf(x1,x2,…,xn)P(X1x1,X2x2,…,Xnxn).TherandomvariablesX1,X2,…,Xnareindependentrandomvariablesifandonlyiftheirjointpdfistheproductoftheindividualpdfsforany(x1,x2,…,xn).Thisdefinitionofindependencealsoholdsforcontinuousrandomvariables.Thenotionofindependenceplaysanimportantroleinobtainingsomeoftheclas-sicdistributionsinprobabilityandstatistics.EarlierwedefinedaBernoullirandomvariableasazero-onerandomvariableindicatingwhetherornotsomeeventoccurs.Often,weareinterestedinthenumberofsuccessesinasequenceofindependent670\nxd7/14/998:57PMPage671AppendixBFundamentalsofProbabilityBernoullitrials.AstandardexampleofindependentBernoullitrialsisflippingacoinagainandagain.Sincetheoutcomeonanyparticularfliphasnothingtodowiththeoutcomesonotherflips,independenceisanappropriateassumption.Independenceisoftenareasonableapproximationinmorecomplicatedsituations.Intheairlinereservationexample,supposethattheairlineacceptsnreservationsforaparticularflight.Foreachi1,2,…,n,letYidenotetheBernollirandomvariableindi-catingwhethercustomerishowsup:Yi1ifcustomeriappears,andYi0other-wise.Lettingagaindenotetheprobabilityofsuccess(usingreservation),eachYihasaBernoulli()distribution.Asanapproximation,wemightassumethattheYiareinde-pendentofoneanother,althoughthisisnotexactlytrueinreality:somepeopletravelingroups,whichmeansthatwhetherornotapersonshowsupisnottrulyindependentofwhetherallothersshowup.Modelingthiskindofdependenceiscomplex,however,sowemightbewillingtouseindependenceasanapproximation.Thevariableofprimaryinterestisthetotalnumberofcustomersshowingupoutofthenreservations;callthisvariableX.SinceeachYiisunitywhenapersonshowsup,wecanwriteXY1Y2…Yn.Now,assumingthateachYihasprobabilityofsuccessandthattheYiareindependent,Xcanbeshowntohaveabinomialdistri-bution.Thatis,theprobabilitydensityfunctionofXisnxnxf(x)(1),x0,1,2,…,n,(B.14)xnn!where,andforanyintegern,n!(read“nfactorial”)isdefinedasxx!(nx)!n!n(n1)(n2)1.Byconvention,0!1.WhenarandomvariableXhasthepdfgivenin(B.14),wewriteX~Binomial(n,).Equation(B.14)canbeusedtocom-puteP(Xx)foranyvalueofxfrom0ton.Iftheflighthas100availableseats,theairlineisinterestedinP(X100).Suppose,initially,thatn120,sothattheairlineaccepts120reservations,andtheprobabilitythateachpersonshowsupis.80.Then,P(X100)P(X101)P(X102)…P(X120),andeachoftheprobabilitiesinthesumcanbefoundfromequa-tion(B.14)withn120,.80,andtheappropriatevalueofx(101to120).Thisisadifficulthandcalculation,butmanystatisticalpackageshavecommandsforcomput-ingthiskindofprobability.Inthiscase,theprobabilitythatmorethan100peoplewillshowupisabout.659,whichisprobablymoreriskofoverbookingthantheairlinewantstotolerate.If,instead,thenumberofreservationsis110,theprobabilityofmorethan100passengersshowingupisonlyabout.024.ConditionalDistributionsIneconometrics,weareusuallyinterestedinhowonerandomvariable,callitY,isrelatedtooneormoreothervariables.Fornow,supposethatthereisonlyvariablewhoseeffectsweareinterestedin,callitX.ThemostwecanknowabouthowXaffectsYiscontainedintheconditionaldistributionofYgivenX.Thisinformationissum-marizedbytheconditionalprobabilitydensityfunction,definedby671\nxd7/14/998:57PMPage672AppendixBFundamentalsofProbabilityfYX(yx)fX,Y(x,y)/fX(x)(B.15)forallvaluesofxsuchthatfX(x)0.Theinterpretationof(B.15)ismosteasilyseenwhenXandYarediscrete.Then,fYX(yx)P(YyXx),(B.16)wheretheright-handsideisreadas“theprobabilitythatYygiventhatXx.”WhenYiscontinuous,fYX(yx)isnotinterpretabledirectlyasaprobability,forthereasonsdis-cussedearlier,butconditionalprobabilitiesarefoundbycomputingareasundertheconditionalpdf.Animportantfeatureofconditionaldistributionsisthat,ifXandYareindependentrandomvariables,knowledgeofthevaluetakenonbyXtellsusnothingabouttheprob-abilitythatYtakesonvariousvalues(andviceversa).Thatis,fYX(yx)fY(y),andfXY(xy)fX(x).EXAMPLEB.2(FreeThrowShooting)Consideragainthebasketball-shootingexample,wheretwofreethrowsaretobeattempted.AssumethattheconditionaldensityisfYX(11).85,fYX(01).15fYX(10).70,fYX(00).30.Thismeansthattheprobabilityoftheplayermakingthesecondfreethrowdependsonwhetherthefirstfreethrowwasmade:ifthefirstfreethrowismade,thechanceofmak-ingthesecondis.85;ifthefirstfreethrowismissed,thechanceofmakingthesecondis.70.ThisimpliesthatXandYarenotindependent;theyaredependent.WecanstillcomputeP(X1,Y1),providedweknowP(X1).Assumethattheprobabilityofmakingthefirstfreethrowis.8,thatis,P(X1).8.Then,from(B.15),wehaveP(X1,Y1)P(Y1X1)P(X1)(.85)(.8).68.B.3FEATURESOFPROBABILITYDISTRIBUTIONSFormanypurposes,wewillbeinterestedinonlyafewaspectsofthedistributionsofrandomvariables.Thefeaturesofinterestcanbeputintothreecategories:measuresofcentraltendency,measuresofvariabilityorspread,andmeasuresofassociationbetweentworandomvariables.WecoverthelastoftheseinSectionB.4.AMeasureofCentralTendency:TheExpectedValueTheexpectedvalueisoneofthemostimportantprobabilisticconceptsthatwewillencounterinourstudyofeconometrics.IfXisarandomvariable,theexpectedvalue672\nxd7/14/998:57PMPage673AppendixBFundamentalsofProbability(orexpectation)ofX,denotedE(X)andsometimesXorsimply,isaweightedaver-ageofallpossiblevaluesofX.Theweightsaredeterminedbytheprobabilitydensityfunction.Sometimes,theexpectedvalueiscalledthepopulationmean,especiallywhenwewanttoemphasizethatXrepresentssomevariableinapopulation.TheprecisedefinitionofexpectedvalueissimplestinthecasethatXisadiscreterandomvariabletakingonafinitenumberofvalues,say{x1,…,xk}.Letf(x)denotetheprobabilitydensityfunctionofX.TheexpectedvalueofXistheweightedaveragekE(X)x1f(x1)x2f(x2)…xkf(xk)xjf(xj).(B.17)j1ThisiseasilycomputedgiventhevaluesofthepdfateachpossibleoutcomeofX.EXAMPLEB.3(ComputinganExpectedValue)SupposethatXtakesonthevalues1,0,and2withprobabilities1/8,1/2,and3/8,respec-tively.Then,E(X)(1)(1/8)0(1/2)2(3/8)5/8.Thisexampleillustratessomethingcuriousaboutexpectedvalues:theexpectedvalueofXcanbeanumberthatisnotevenapossibleoutcomeofX.WeknowthatXtakesonthevalue1,0,or2,yetitsexpectedvalueis5/8.Thismakestheexpectedvaluedeficientforsummarizingthecentraltendencyofcertaindiscreterandomvariables,butcalculationssuchasthosejustmentionedcanbeuseful,aswewillseelater.IfXisacontinuousrandomvariable,thenE(X)isdefinedasanintegral:E(X)xf(x)dx,(B.18)whichweassumeiswell-defined.Thiscanstillbeinterpretedasaweightedaverage.Unlikeinthediscretecase,E(X)isalwaysanumberthatisapossibleoutcomeofX.Inthistext,wewillnotneedtocomputeexpectedvaluesusingintegration,althoughwewilldrawonsomewell-knownresultsfromprobabilityforexpectedvaluesofspecialrandomvariables.GivenarandomvariableXandafunctiong(),wecancreateanewrandomvari-2ableg(X).Forexample,ifXisarandomvariable,thensoisXandlog(X)(ifX0).Theexpectedvalueofg(X)is,again,simplyaweightedaverage:kE[g(X)]g(xj)fX(xj)(B.19)j1or,foracontinuousrandomvariable,673\nxd7/14/998:57PMPage674AppendixBFundamentalsofProbabilityE[g(X)]g(x)fX(x)dx.(B.20)EXAMPLEB.42(ExpectedValueofX)2FortherandomvariableinExampleB.3,letg(X)X.Then,2222E(X)(1)(1/8)(0)(1/2)(2)(3/8)13/8.2InExampleB.3,wecomputedE(X)5/8,sothat[E(X)]25/64.Thisshowsthat22E(X)isnotthesameas[E(X)].Infact,foranonlinearfunctiong(X),E[g(X)]g[E(X)](exceptinveryspecialcases).IfXandYarerandomvariables,theng(X,Y)isarandomvariableforanyfunctiong,andsowecandefineitsexpectation.WhenXandYarebothdiscrete,takingonval-ues{x1,x2,…,xk}and{y1,y2,…,ym},respectively,theexpectedvalueiskmE[g(X,Y)]g(xh,yj)fX,Y(xh,yj),h1j1wherefX,Yisthejointpdfof(X,Y).Thedefinitionismorecomplicatedforcontinuousrandomvariablessinceitinvolvesintegration;wedonotneedithere.Theextensiontomorethantworandomvariablesisstraightforward.PropertiesofExpectedValueIneconometrics,wearenotsoconcernedwithcomputingexpectedvaluesfromvari-ousdistributions;themajorcalculationshavebeendonemanytimes,andwewilllargelytaketheseonfaith.Wewillneedtomanipulatesomeexpectedvaluesusingafewsimplerules.Thesearesoimportantthatwegivethemlabels:PROPERTYE.1Foranyconstantc,E(c)c.PROPERTYE.2Foranyconstantsaandb,E(aXb)aE(X)b.OneusefulimplicationofE.2isthat,ifE(X),andwedefineanewrandomvari-ableasYX,thenE(Y)0;inE.2,takea1andb.AsanexampleofPropertyE.2,letXbethetemperaturemeasuredinCelsius,atnoononaparticulardayatagivenlocation;supposetheexpectedtemperatureisE(X)25.IfYisthetemperaturemeasuredinFahrenheit,thenY32(9/5)X.FromPropertyE.2,theexpectedtemperatureinFahrenheitisE(Y)32(9/5)E(X)32(9/5)2577.Generally,itiseasytocomputetheexpectedvalueofalinearfunctionofmanyran-domvariables.674\nxd7/14/998:57PMPage675AppendixBFundamentalsofProbabilityPROPERTYE.3If{a1,a2,…,an}areconstantsand{X1,X2,…,Xn}arerandomvariables,thenE(a1X1a2X2…anXn)a1E(X1)a2E(X2)…anE(Xn).Or,usingsummationnotation,nnE(aiXi)aiE(Xi).(B.21)i1i1Asaspecialcaseofthis,wehave(witheachai1)nnE(Xi)E(Xi),(B.22)i1i1sothattheexpectedvalueofthesumisthesumofexpectedvalues.Thispropertyisusedoftenforderivationsinmathematicalstatistics.EXAMPLEB.5(FindingExpectedRevenue)LetX1,X2,andX3bethenumbersofsmall,medium,andlargepizzas,respectively,solddur-ingthedayatapizzaparlor.ThesearerandomvariableswithexpectedvaluesE(X1)25,E(X2)57,andE(X3)40.Thepricesofsmall,medium,andlargepizzasare$5.50,$7.60,and$9.15.Therefore,theexpectedrevenuefrompizzasalesonagivendayisE(5.50X17.60X29.15X3)5.50E(X1)7.60E(X2)9.15E(X3)5.50(25)7.60(57)9.15(40)936.70,thatis,$936.70.Theactualrevenueonanyparticulardaywillgenerallydifferfromthisvalue,butthisistheexpectedrevenue.WecanalsousePropertyE.3toshowshowthatifX~Binomial(n,),thenE(X)n.Thatis,theexpectednumberofsuccessesinnBernoullitrialsissimplythenum-beroftrialstimestheprobabilityofsuccessonanyparticulartrial.ThisiseasilyseenbywritingXasXY1Y2…Yn,whereeachYi~Bernoulli().Then,nnE(X)E(Yi)n.i1i1Wecanapplythistotheairlinereservationexample,wheretheairlinemakesn120reservations,andtheprobabilityofshowingupis.85.Theexpectednumberofpeo-pleshowingupis120(.85)102.Therefore,ifthereare100seatsavailable,theexpectednumberofpeopleshowingupistoolarge;thishassomebearingonwhetheritisagoodideafortheairlinetomake120reservations.Actually,whattheairlineshoulddoisdefineaprofitfunctionthataccountsforthenetrevenueearnedperseatsoldandthecostperpassengerbumpedfromtheflight.This675\nxd7/14/998:57PMPage676AppendixBFundamentalsofProbabilityprofitfunctionisrandombecausetheactualnumberofpeopleshowingupisrandom.Letrbethenetrevenuefromeachpassenger.(Youcanthinkofthisasthepriceoftheticketforsimplicity.)Letcbethecompensationowedtoanypassengerbumpedfromtheflight.Neitherrnorcisrandom;theseareassumedtobeknowntotheairline.LetYdenoteprofitsfortheflight.Then,with100seatsavailable,YrXifX100100rc(X100)ifX100.Thefirstequationgivesprofitifnomorethan100peopleshowupfortheflight;thesecondequationisprofitifmorethan100peopleshowup.(Inthelattercase,thenetrevenuefromticketsalesis100r,sinceall100seatsaresold,andthenc(X100)isthecostofmakingmorethan100reservations.)UsingthefactthatXhasaBinomial(n,.85)distribution,wherenisthenumberofreservationsmade,expectedprofits,E(Y),canbefoundasafunctionofn(andrandc).ComputingE(Y)directlywouldbequitedifficult,butitcanbefoundquicklyusingacomputer.Oncevaluesforrandcaregiven,thevalueofnthatmaximizesexpectedprofitscanbefoundbysearch-ingoverdifferentvaluesofn.AnotherMeasureofCentralTendency:TheMedianTheexpectedvalueisonlyonepossibilityfordefiningthecentraltendencyofarandomvariable.Anothermeasureofcentraltendencyisthemedian.Ageneraldefinitionofmedianistoocomplicatedforourpurposes.IfXiscontinuous,thenthemedianofX,saym,isthevaluesuchthatone-halfoftheareaunderpdfistotheleftofm,andone-halfoftheareaistotherightofm.WhenXisdiscreteandtakesonafinitenumberofoddvalues,themedianisobtainedbyorderingthepossiblevaluesofXandthenselectingthevalueinthemiddle.Forexample,ifXcantakeonthevalues{4,0,2,8,10,13,17},thenthemedianvalueofXis8.IfXtakesonanevennumberofvalues,therearereallytwomedianvalues;sometimestheseareaveragedtogetauniquemedianvalue.Thus,ifXtakesontheval-ues{5,3,9,17},thenthemedianvaluesare3and9;ifweaveragethese,wegetamedianequalto6.Ingeneral,themedian,sometimesdenotedMed(X),andtheexpectedvalue,E(X),aredifferent.Neitheris“better”thantheotherasameasureofcentraltendency;theyarebothvalidwaystomeasurethecenterofthedistributionofX.Inonespecialcase,themedianandexpectedvalue(ormean)arethesame.IftheprobabilitydistributionofXissymmetricallydistributedaboutthevalue,thenisboththeexpectedvalueandthemedian.Mathematically,theconditionisf(x)f(x)forallx.ThiscaseisillustratedinFigureB.3.MeasuresofVariability:VarianceandStandardDeviationWhilethecentraltendencyofarandomvariableisvaluable,itdoesnottellusevery-thingwewanttoknowaboutthedistributionofarandomvariable.FigureB.4showsthepdfsoftworandomvariableswiththesamemean.Clearly,thedistributionofXis676\nxd7/14/998:57PMPage677AppendixBFundamentalsofProbabilityFigureB.3Asymmetricprobabilitydistribution.f(x)xmoretightlycenteredaboutitsmeanthanisthedistributionofY.Wewouldliketohaveasimplewayofsummarizingthis.VarianceForarandomvariableX,letE(X).TherearevariouswaystomeasurehowfarXisfromitsexpectedvalue,butthesimplestonetoworkwithalgebraicallyisthesquared2difference,(X).(Thesquaringservestoeliminatethesignfromthedistancemea-sure;theresultingpositivevaluecorrespondstoourintuitivenotionofdistance.)ThisdistanceisitselfarandomvariablesinceitcanchangewitheveryoutcomeofX.JustasweneededanumbertosummarizethecentraltendencyofX,weneedanumberthattellsushowfarXisfrom,onaverage.Onesuchnumberisthevariance,whichtellsustheexpecteddistancefromXtoitsmean:2Var(X)E[(X)].(B.23)22VarianceissometimesdenotedX,orsimply,whenthecontextisclear.From(B.23),itfollowsthatthevarianceisalwaysnonnegative.Asacomputationaldevice,itisusefultoobservethat677\nxd7/14/998:57PMPage678AppendixBFundamentalsofProbabilityFigureB.4Randomvariableswiththesamemeanbutdifferentdistributions.pdffXfYx,y22222222E(X2X)E(X)2E(X).(B.24)Inusingeither(B.23)or(B.24),weneednotdistinguishbetweendiscreteandcontinu-ousrandomvariables:thedefinitionofvarianceisthesameineithercase.Mostoften,2wefirstcomputeE(X),thenE(X),andthenweusetheformulain(B.24).Forexam-22ple,ifX~Bernoulli(),thenE(X),and,sinceXX,E(X).Itfollowsfrom222equation(B.24)thatVar(X)E(X)(1).Twoimportantpropertiesofthevariancefollow.PROPERTYVAR.1Var(X)0ifandonlyifthereisaconstantc,suchthatP(Xc)1,inwhichcase,E(X)c.Thisfirstpropertysaysthatthevarianceofanyconstantiszeroandifarandomvari-ablehaszerovariance,thenitisessentiallyconstant.PROPERTYVAR.22Foranyconstantsaandb,Var(aXb)aVar(X).678\nxd7/14/998:57PMPage679AppendixBFundamentalsofProbabilityThismeansthataddingaconstanttoarandomvariabledoesnotchangethevariance,butmultiplyingarandomvariablebyaconstantincreasesthevariancebyafactorequaltothesquareofthatconstant.Forexample,ifXdenotestemperatureinCelsiusand2Y32(9/5)XistemperatureinFahrenheit,thenVar(Y)(9/5)Var(X)(81/25)Var(X).StandardDeviationThestandarddeviationofarandomvariable,denotedsd(X),issimplythepositivesquarerootofthevariance:sd(X)Var(X).ThestandarddeviationissometimesdenotedX,orsimply,whentherandomvariableisunderstood.Twostandarddevi-ationpropertiesimmediatelyfollowfromPropertiesVAR.1andVAR.2.PROPERTYSD.1Foranyconstantc,sd(c)0.PROPERTYSD.2Foranyconstantsaandb,sd(aXb)asd(X).Inparticular,ifa0,thensd(aX)asd(X).Thislastpropertymakesthestandarddeviationmorenaturaltoworkwiththanthevariance.Forexample,supposethatXisarandomvariablemeasuredinthousandsofdollars,sayincome.IfwedefineY1,000X,thenYisincomemeasuredindol-lars.SupposethatE(X)20,andsd(X)6.ThenE(Y)1,000E(X)20,000,andsd(Y)1,000sd(X)6,000,sothattheexpectedvalueandstandarddeviationbothincreasebythesamefactor,1,000.Ifweworkedwithvariance,wewouldhaveVar(Y)2(1,000)Var(X),sothatthevarianceofYisonemilliontimeslargerthanthevari-anceofX.StandardizingaRandomVariableAsanapplicationofthepropertiesofvarianceandstandarddeviation—andatopicofpracticalinterestinitsownright—supposethatgivenarandomvariableX,wedefineanewrandomvariablebysubtractingoffitsmeananddividingbyitsstandarddevia-tion:XZ,(B.25)whichwecanwriteasZaXb,wherea(1/),andb(/).Then,fromPropertyE.2,E(Z)aE(X)b(/)(/)0.FromPropertyVAR.2,679\nxd7/14/998:57PMPage680AppendixBFundamentalsofProbability222Var(Z)aVar(X)(/)1.Thus,therandomvariableZhasameanofzeroandavariance(andthereforeastan-darddeviation)equaltoone.ThisprocedureissometimesknownasstandardizingtherandomvariableX,andZiscalledastandardizedrandomvariable.(Inintroductorystatisticscourses,itissometimescalledthez-transformofX.)Itisimportanttoremem-berthatthestandarddeviation,notthevariance,appearsinthedenominatorof(B.25).Aswewillsee,thistransformationisfrequentlyusedinstatisticalinference.Asaspecificexample,supposethatE(X)2,andVar(X)9.ThenZ(X2)/3hasexpectedvaluezeroandvarianceone.B.4FEATURESOFJOINTANDCONDITIONALDISTRIBUTIONSMeasuresofAssociation:CovarianceandCorrelationWhilethejointpdfoftworandomvariablescompletelydescribestherelationshipbetweenthem,itisusefultohavesummarymeasuresofhow,onaverage,tworandomvariablesvarywithoneanother.Aswiththeexpectedvalueandvariance,thisissimi-lartousingasinglenumbertosummarizesomethingaboutanentiredistribution,whichinthiscaseisajointdistributionoftworandomvariables.CovarianceLetXE(X)andYE(Y)andconsidertherandomvariable(XX)(YY).Now,ifXisaboveitsmeanandYisaboveitsmean,then(XX)(YY)0.ThisisalsotrueifXXandYY.Ontheotherhand,ifXXandYY,orviceversa,then(XX)(YY)0.How,then,canthisproducttellusanythingabouttherelationshipbetweenXandY?ThecovariancebetweentworandomvariablesXandY,sometimescalledthepopulationcovariancetoemphasizethatitconcernstherelationshipbetweentwovari-ablesdescribingapopulation,isdefinedastheexpectedvalueoftheproduct(XX)(YY):Cov(X,Y)E[(XX)(YY)],(B.26)whichissometimesdenotedXY.IfXY0,then,onaverage,whenXisaboveitsmean,Yisalsoaboveitsmean.IfXY0,then,onaverage,whenXisaboveitsmean,Yisbelowitsmean.SeveralexpressionsusefulforcomputingCov(X,Y)areasfollows:Cov(X,Y)E[(XX)(YY)]E[(XX)Y](B.27)E[X(YY)]E(XY)XY.Itfollowsfrom(B.27),thatifE(X)0orE(Y)0,thenCov(X,Y)E(XY).Covariancemeasurestheamountoflineardependencebetweentworandomvari-ables.Apositivecovarianceindicatesthattworandomvariablesmoveinthesame680\nxd7/14/998:57PMPage681AppendixBFundamentalsofProbabilitydirection,whileanegativecovarianceindicatestheymoveinoppositedirections.Interpretingthemagnitudeofacovariancecanbealittletricky,aswewillseeshortly.Sincecovarianceisameasureofhowtworandomvariablesarerelated,itisnaturaltoaskhowcovarianceisrelatedtothenotionofindependence.Thisisgivenbythefol-lowingproperty.PROPERTYCOV.1IfXandYareindependent,thenCov(X,Y)0.Thispropertyfollowsfromequation(B.27)andthefactthatE(XY)E(X)E(Y)whenXandYareindependent.ItisimportanttorememberthattheconverseofCOV.1isnottrue:zerocovariancebetweenXandYdoesnotimplythatXandYareindependent.In2fact,therearerandomvariablesXsuchthat,ifYX,Cov(X,Y)0.(Anyrandom32variablewithE(X)0andE(X)0hasthisproperty.)IfYX,thenXandYareclearlynotindependent:onceweknowX,weknowY.ItseemsratherstrangethatXand2Xcouldhavezerocovariance,andthisrevealsaweaknessofcovarianceasageneralmeasureofassociationbetweenrandomvariables.Thecovarianceisusefulincontextswhenrelationshipsareatleastapproximatelylinear.Thesecondmajorpropertyofcovarianceinvolvescovariancesbetweenlinearfunc-tions.PROPERTYCOV.2Foranyconstantsa1,b1,a2,andb2,Cov(a1Xb1,a2Yb2)a1a2Cov(X,Y).(B.28)AnimportantimplicationofCOV.2isthatthecovariancebetweentworandomvari-ablescanbealteredsimplybymultiplyingoneorbothoftherandomvariablesbyaconstant.Thisisimportantineconomicssincemonetaryvariables,inflationrates,andsoon,canbedefinedwithdifferentunitsofmeasurementwithoutchangingtheirmeaning.Finally,itisusefultoknowthattheabsolutevalueofthecovariancebetweenanytworandomvariablesisboundedbytheproductoftheirstandarddeviations;thisisknownastheCauchy-Schwartzinequality.PROPERTYCOV.3Cov(X,Y)sd(X)sd(Y).CorrelationCoefficientSupposewewanttoknowtherelationshipbetweenamountofeducationandannualearningsintheworkingpopulation.WecouldletXdenoteeducationandYdenoteearn-ingsandthencomputetheircovariance.Buttheanswerwegetwilldependonhowwechoosetomeasureeducationandearnings.PropertyCOV.2impliesthatthecovariancebetweeneducationandearningsdependsonwhetherearningsaremeasuredindollarsorthousandsofdollars,orwhethereducationismeasuredinmonthsoryears.Itispretty681\nxd7/14/998:57PMPage682AppendixBFundamentalsofProbabilityclearthathowwemeasurethesevariableshasnobearingonhowstronglytheyarerelated.Butthecovariancebetweenthemdoesdependontheunitsofmeasurement.ThefactthatthecovariancedependsonunitsofmeasurementisadeficiencythatisovercomebythecorrelationcoefficientbetweenXandY:Cov(X,Y)XYCorr(X,Y);(B.29)sd(X)sd(Y)XYthecorrelationcoefficientbetweenXandYissometimesdenotedXY(andissometimescalledthepopulationcorrelation).BecauseXandYarepositive,Cov(X,Y)andCorr(X,Y)alwayshavethesamesign,andCorr(X,Y)0ifandonlyifCov(X,Y)0.Someofthepropertiesofcovari-ancecarryovertocorrelation.IfXandYareindependent,thenCorr(X,Y)0,butzerocorrelationdoesnotimplylackofindependence.(Thecorrelationcoefficientisalsoameasureoflineardependence.)However,themagnitudeofthecorrelationcoefficientiseasiertointerpretthanthesizeofthecovarianceduetothefollowingproperty.PROPERTYCORR.11Corr(X,Y)1.IfCorr(X,Y)0,orequivalentlyCov(X,Y)0,thenthereisnolinearrelationshipbetweenXandY,andXandYaresaidtobeuncorrelated;otherwise,XandYarecor-related.Corr(X,Y)1impliesaperfectpositivelinearrelationship,whichmeansthatwecanwriteYabX,forsomeconstantaandsomeconstantb0.Corr(X,Y)1impliesaperfectnegativerelationship,sothatYabX,forsomeb0.Theextremecasesofpositiveornegativeonerarelyoccur.ValuesofXYcloserto1or1indicatestrongerlinearrelationships.Asmentionedearlier,thecorrelationbetweenXandYisinvarianttotheunitsofmeasurementofeitherXorY.Thisisstatedmoregenerallyasfollows.PROPERTYCORR.2Forconstantsa1,b1,a2,andb2,witha1a20,Corr(a1Xb1,a2Yb2)Corr(X,Y).Ifa1a20,thenCorr(a1Xb1,a2Yb2)Corr(X,Y).Asanexample,supposethatthecorrelationbetweenearningsandeducationintheworkingpopulationis.15.Thismeasuredoesnotdependonwhetherearningsaremea-suredindollars,thousandsofdollars,oranyotherunit;italsodoesnotdependonwhethereducationismeasuredinyears,quarters,months,andsoon.VarianceofSumsofRandomVariablesNowthatwehavedefinedcovarianceandcorrelation,wecancompleteourlistofmajorpropertiesofthevariance.682\nxd7/14/998:57PMPage683AppendixBFundamentalsofProbabilityPROPERTYVAR.3Forconstantsaandb,22Var(aXbY)aVar(X)bVar(Y)2abCov(X,Y).Itfollowsimmediatelythat,ifXandYareuncorrelated—sothatCov(X,Y)0—thenVar(XY)Var(X)Var(Y)(B.30)andVar(XY)Var(X)Var(Y).(B.31)Inthelattercase,notehowthevarianceofthedifferenceisthesum,notthedifference,inthevariances.Asanexampleof(B.30),letXdenoteprofitsearnedbyarestaurantduringaFridaynightandletYbeprofitsearnedonthefollowingSaturdaynight.Then,ZXYisprofitsforthetwonights.SupposeXandYeachhaveanexpectedvalueof$300andastandarddeviationof$15(sothatthevarianceis225).ExpectedprofitsforthetwonightsisE(Z)E(X)E(Y)2(300)600dollars.IfXandYareindependent,andthereforeuncorrelated,thenthevarianceoftotalprofitsisthesumofthevariances:Var(Z)Var(X)Var(Y)2(225)450.Itfollowsthatthestandarddeviationoftotalprofitsis450orabout$21.21.Expressions(B.30)and(B.31)extendtomorethantworandomvariables.Tostatethisextension,weneedadefinition.Therandomvariables{X1,…,Xn}arepairwiseuncorrelatedrandomvariablesifeachvariableinthesetisuncorrelatedwitheveryothervariableintheset.Thatis,Cov(Xi,Xj)0,forallij.PROPERTYVAR.4If{X1,…,Xn}arepairwiseuncorrelatedrandomvariablesand{ai:i1,…,n}areconstants,then22Var(a1X1…anXn)a1Var(X1)…anVar(Xn).Insummationnotation,wecanwritenn2Var(aiXi)aiVar(Xi).(B.32)i1i1AspecialcaseofPropertyVAR.4occurswhenwetakeai1foralli.Then,forpair-wiseuncorrelatedrandomvariables,thevarianceofthesumisthesumofthevariances:nnVar(Xi)Var(Xi).(B.33)i1i1Sinceindependentrandomvariablesareuncorrelated(seePropertyCOV.1),thevari-anceofasumofindependentrandomvariablesisthesumofthevariances.683\nxd7/14/998:57PMPage684AppendixBFundamentalsofProbabilitynIftheXiarenotpairwiseuncorrelated,thentheexpressionforVar(aiXi)ismuchi1morecomplicated;itdependsoneachcovariance,aswellasoneachvariance.Wewillnotneedthemoregeneralformulaforourpurposes.Wecanuse(B.33)toderivethevarianceforabinomialrandomvariable.LetX~Binomial(n,)andwriteXY1…Yn,wheretheYiareindependentBernoulli()randomvariables.Then,by(B.33),Var(X)Var(Y1)…Var(Yn)n(1).Intheairlinereservationsexamplewithn120and.85,thevarianceofthenumberofpassengersarrivingfortheirreservationsis120(.85)(.15)15.3,andsothestandarddeviationisabout3.9.ConditionalExpectationCovarianceandcorrelationmeasurethelinearrelationshipbetweentworandomvari-ablesandtreatthemsymmetrically.Moreofteninthesocialsciences,wewouldliketoexplainonevariable,calledY,intermsofanothervariable,sayX.Further,ifYisrelatedtoXinanonlinearfashion,wewouldliketoknowthis.CallYtheexplainedvariableandXtheexplanatoryvariable.Forexample,Ymightbehourlywage,andXmightbeyearsofformaleducation.Wehavealreadyintroducedthenotionoftheconditionalprobabilitydensityfunc-tionofYgivenX.Thus,wemightwanttoseehowthedistributionofwageschangeswitheducationlevel.However,weusuallywanttohaveasimplewayofsummarizingthisdistribution.Asinglenumberwillnolongersuffice,sincethedistributionofY,givenXx,generallydependsonthevalueofx.Nevertheless,wecansummarizetherelationshipbetweenYandXbylookingattheconditionalexpectationofYgivenX,sometimescalledtheconditionalmean.Theideaisthis.SupposeweknowthatXhastakenonaparticularvalue,sayx.Then,wecancomputetheexpectedvalueofY,giventhatweknowthisoutcomeofX.WedenotethisexpectedvaluebyE(YXx),orsome-timesE(Yx)forshorthand.Generally,asxchanges,sodoesE(Yx).WhenYisadiscreterandomvariabletakingonvalues{y1,…,ym},thenmE(Yx)yjfYX(yjx).j1WhenYiscontinuous,E(Yx)isdefinedbyintegratingyfYX(yx)overallpossibleval-uesofy.Aswithunconditionalexpectations,theconditionalexpectationisaweightedaverageofpossiblevaluesofY,butnowtheweightsreflectthefactthatXhastakenonaspecificvalue.Thus,E(Yx)isjustsomefunctionofx,whichtellsushowtheexpectedvalueofYvarieswithx.Asanexample,let(X,Y)representthepopulationofallworkingindividuals,whereXisyearsofeducation,andYishourlywage.Then,E(YX12)istheaveragehourlywageforallpeopleinthepopulationwith12yearsofeducation(roughlyahighschooleducation).E(YX16)istheaveragehourlywageforallpeoplewith16yearsofedu-cation.Tracingouttheexpectedvalueforvariouslevelsofeducationprovidesimpor-tantinformationonhowwagesandeducationarerelated.SeeFigureB.5foranillustration.684\nxd7/14/998:57PMPage685AppendixBFundamentalsofProbabilityFigureB.5Theexpectedvalueofhourlywagegivenvariouslevelsofeducation.E(WAGE|EDUC)48121620EDUCInprinciple,theexpectedvalueofhourlywagecanbefoundateachlevelofedu-cation,andtheseexpectationscanbesummarizedinatable.Sinceeducationcanvarywidely—andcanevenbemeasuredinfractionsofayear—thisisacumbersomewaytoshowtherelationshipbetweenaveragewageandamountofeducation.Ineconomet-rics,wetypicallyspecifysimplefunctionsthatcapturethisrelationship.Asanexam-ple,supposethattheexpectedvalueofWAGEgivenEDUCisthelinearfunctionE(WAGEEDUC)1.05.45EDUC.Ifthisrelationshipholdsinthepopulationofworkingpeople,theaveragewageforpeo-plewitheightyearsofeducationis1.05.45(8)4.65,or$4.65.Theaveragewageforpeoplewith16yearsofeducationis8.25,or$8.25.ThecoefficientonEDUCimpliesthateachyearofeducationincreasestheexpectedhourlywageby.45,or45cents.Conditionalexpectationscanalsobenonlinearfunctions.Forexample,supposethatE(Yx)10/x,whereXisarandomvariablethatisalwaysgreaterthanzero.Thisfunc-tionisgraphedinFigureB.6.Thiscouldrepresentademandfunction,whereYisquan-titydemanded,andXisprice.IfYandXarerelatedinthisway,ananalysisoflinearassociation,suchascorrelationanalysis,wouldbeinadequate.685\nxd7/14/998:57PMPage686AppendixBFundamentalsofProbabilityFigureB.6GraphofE(Yx)10/x.E(Y|x)10E(Y|x)=10/x211510xPropertiesofConditionalExpectationSeveralbasicpropertiesofconditionalexpectationsareusefulforderivationsinecono-metricanalysis.PROPERTYCE.1:E[c(X)X]c(X),foranyfunctionc(X).ThisfirstpropertymeansthatfunctionsofXbehaveasconstantswhenwecompute22expectationsconditionalonX.Forexample,E(XX)X.Intuitively,thissimply2meansthatifweknowX,thenwealsoknowX.PROPERTYCE.2Forfunctionsa(X)andb(X),E[a(X)Yb(X)X]a(X)E(YX)b(X).Forexample,wecaneasilycomputetheconditionalexpectationofafunctionsuchas222XY2X:E(XY2XX)XE(YX)2X.Thenextpropertytiestogetherthenotionsofindependenceandconditionalexpec-tations.PROPERTYCE.3IfXandYareindependent,thenE(YX)E(Y).686\nxd7/14/998:57PMPage687AppendixBFundamentalsofProbabilityThispropertymeansthat,ifXandYareindependent,thentheexpectedvalueofYgivenXdoesnotdependonX,inwhichcase,E(YX)alwaysequalsthe(unconditional)expectedvalueofY.Inthewageandeducationexample,ifwageswereindependentofeducation,thentheaveragewagesofhighschoolandcollegegraduateswouldbethesame.Sincethisisalmostcertainlyfalse,wecannotassumethatwageandeducationareindependent.AspecialcaseofPropertyCE.3isthefollowing:ifUandXareindependentandE(U)0,thenE(UX)0.TherearealsopropertiesoftheconditionalexpectationthathavetodowiththefactthatE(YX)isafunctionofX,sayE(YX)(X).SinceXisarandomvariable,(X)isalsoarandomvariable.Furthermore,(X)hasaprobabilitydistributionandthere-foreanexpectedvalue.Generally,theexpectedvalueof(X)couldbeverydifficulttocomputedirectly.Thelawofiteratedexpectationssaysthattheexpectedvalueof(X)issimplyequaltotheexpectedvalueofY.Wewritethisasfollows.PROPERTYCE.4E[E(YX)]E(Y).Thispropertyisalittlehardtograspatfirst.Itmeansthat,ifwefirstobtainE(YX)asafunctionofXandtaketheexpectedvalueofthis(withrespecttothedistributionofX,ofcourse),thenweendupwithE(Y).Thisishardlyobvious,butitcanbederivedusingthedefinitionofexpectedvalues.SupposeYWAGEandXEDUC,whereWAGEismeasuredinhours,andEDUCismeasuredinyears.SupposetheexpectedvalueofWAGEgivenEDUCisE(WAGEEDUC)4.60EDUC.Further,E(EDUC)11.5.Then,thelawofiter-atedexpectationsimpliesthatE(WAGE)E(4.60EDUC)4.60E(EDUC)4.60(11.5)10.90,or$10.90anhour.Thenextpropertystatesamoregeneralversionofthelawofiteratedexpectations.PROPERTYCE.4E(YX)E[E(YX,Z)X].Inotherwords,wecanfindE(YX)intwosteps.First,findE(YX,Z)foranyotherran-domvariableZ.Then,findtheexpectedvalueofE(YX,Z),conditionalonX.PROPERTYCE.5IfE(YX)E(Y),thenCov(X,Y)0(andsoCorr(X,Y)0).Infact,everyfunctionofXisuncorrelatedwithY.Thispropertymeansthat,ifknowledgeofXdoesnotchangetheexpectedvalueofY,thenXandYmustbeuncorrelated,whichimpliesthatifXandYarecorrelated,thenE(YX)mustdependonX.TheconverseofPropertyCE.5isnottrue:ifXandYare2uncorrelated,E(YX)couldstilldependonX.Forexample,supposeYX.Then,2E(YX)X,whichisclearlyafunctionofX.However,aswementionedinourdis-2cussionofcovarianceandcorrelation,itispossiblethatXandXareuncorrelated.The687\nxd7/14/998:57PMPage688AppendixBFundamentalsofProbabilityconditionalexpectationcapturesthenonlinearrelationshipbetweenXandYthatcorre-lationanalysiswouldmissentirely.PropertiesCE.4andCE.5havetwomajorimplications:ifUandXarerandomvari-ablessuchthatE(UX)0,thenE(U)0,andUandXareuncorrelated.PROPERTYCE.6222IfE(Y)andE[g(X)]forsomefunctiong,thenE{[Y(X)]X}222E{[Yg(X)]X}andE{[Y(X)]}E{[Yg(X)]}.Thislastpropertyisveryusefulinpredictingorforecastingcontexts.Thefirstinequal-itysaysthat,ifwemeasurepredictioninaccuracyastheexpectedsquaredpredictionerror,conditionalonX,thentheconditionalmeanisbetterthananyotherfunctionofXforpredictingY.Theconditionalmeanalsominimizestheunconditionalexpectedsquaredpredictionerror.ConditionalVarianceGivenrandomvariablesXandY,thevarianceofY,conditionalonXx,issimplythevarianceassociatedwiththeconditionaldistributionofY,givenXx:E{[Y2E(Yx)]x}.Theformula22Var(YXx)E(Yx)[E(Yx)]isoftenusefulforcalculations.Onlyoccasionallywillwehavetocomputeacondi-tionalvariance.Butwewillhavetomakeassumptionsaboutandmanipulatecon-ditionalvariancesforcertaintopicsinregressionanalysis.Asanexample,letYSAVINGandXINCOME(bothofthesemeasuredannu-allyforthepopulationofallfamilies).SupposethatVar(SAVINGINCOME)400.25INCOME.Thissaysthat,asincomeincreases,thevarianceinsavinglevelsalsoincreases.ItisimportanttoseethattherelationshipbetweenthevarianceofSAVINGandINCOMEistotallyseparatefromthatbetweentheexpectedvalueofSAVINGandINCOME.Westateoneusefulpropertyabouttheconditionalvariance.PROPERTYCV.1IfXandYareindependent,thenVar(YX)Var(Y).Thispropertyisprettyclear,sincethedistributionofYgivenXdoesnotdependonX,andVar(YX)isjustonefeatureofthisdistribution.B.5THENORMALANDRELATEDDISTRIBUTIONSTheNormalDistributionThenormaldistribution,andthosederivedfromit,arethemostwidelyuseddistribu-tionsinstatisticsandeconometrics.Assumingthatrandomvariablesdefinedoverpop-ulationsarenormallydistributedsimplifiesprobabilitycalculations.Inaddition,wewill688\nxd7/14/998:57PMPage689AppendixBFundamentalsofProbabilityrelyheavilyonthenormalandrelateddistributionstoconductinferenceinstatisticsandeconometrics—evenwhentheunderlyingpopulationisnotnecessarilynormal.Wemustpostponethedetails,butbeassuredthatthesedistributionswillarisemanytimesthroughoutthistext.Anormalrandomvariableisacontinuousrandomvariablethatcantakeonanyvalue.ItsprobabilitydensityfunctionhasthefamiliarbellshapegraphedinFigureB.7.Mathematically,thepdfofXcanbewrittenas122f(x)exp[(x)/2],x,(B.34)22whereE(X),andVar(X).WesaythatXhasanormaldistributionwith22expectedvalueandvariance,writtenasX~Normal(,).Becausethenormaldistributionissymmetricabout,isalsothemedianofX.ThenormaldistributionissometimescalledtheGaussiandistributionafterthefamousstatisticianC.F.Gauss.Certainrandomvariablesappeartoroughlyfollowanormaldistribution.Humanheightsandweights,testscores,andcountyunemploymentrateshavepdfsroughlytheshapeinFigureB.7.Otherdistributions,suchasincomedistributions,donotappeartofollowthenormalprobabilityfunction.Inmostcountries,incomeisnotsymmetricallydistributedaboutanyvalue;thedistributionisskewedtowardstheuppertail.InsomeFigureB.7Thegeneralshapeofthenormalprobabilitydensityfunction.fforanormalXrandomvariablex689\nxd7/14/998:57PMPage690AppendixBFundamentalsofProbabilitycases,avariablecanbetransformedtoachievenormality.Apopulartransformationisthenaturallog,whichmakessenseforpositiverandomvariables.IfXisapositiveran-domvariable,suchasincome,andYlog(X)hasanormaldistribution,thenwesaythatXhasalognormaldistribution.Itturnsoutthatthelognormaldistributionfitsincomedistributionprettywellinmanycountries.Othervariables,suchaspricesofgoods,appeartobewell-describedaslognormallydistributed.TheStandardNormalDistributionOnespecialcaseofthenormaldistributionoccurswhenthemeaniszeroandthevari-ance(and,therefore,thestandarddeviation)isunity.IfarandomvariableZhasaNormal(0,1)distribution,thenwesayithasastandardnormaldistribution.Thepdfofastandardnormalrandomvariableisdenoted(z);from(B.34),with0and21,itisgivenby12(z)exp(z/2),z.(B.35)2Thestandardnormalcumulativedistributionfunctionisdenoted(z)andisobtainedastheareaunder,totheleftofz;seeFigureB.8.Recallthat(z)P(Zz);sinceZiscontinuous,(z)P(Zz),aswell.Thereisnosimpleformulathatcanbeusedtoobtainthevaluesof(z)[because(z)istheintegralofthefunctionin(B.35),andthisintregralhasnoclosedform].FigureB.8Thestandardnormalcumulativedistributionfunction.1.50–303z690\nxd7/14/998:57PMPage691AppendixBFundamentalsofProbabilityNevertheless,thevaluesfor(z)areeasilytabulated;theyaregivenforzbetween3.1and3.1inTableG.1.Forz3.1,(z)islessthan.001,andforz3.1,(z)isgreaterthan.999.Moststatisticsandeconometricssoftwarepackagesincludesimplecommandsforcomputingvaluesofthestandardnormalcdf,sowecanoftenavoidprintedtablesentirelyandobtaintheprobabilitiesforanyvalueofz.Usingbasicfactsfromprobability—and,inparticular,properties(B.7)and(B.8)concerningcdfs—wecanusethestandardnormalcdfforcomputingtheprobabilityofanyeventinvolvingastandardnormalrandomvariable.ThemostimportantformulasareP(Zz)1(z),(B.36)P(Zz)P(Zz),(B.37)andP(aZb)(b)(a).(B.38)BecauseZisacontinuousrandomvariable,allthreeformulasholdwhetherornottheinequalitiesarestrict.SomeexamplesincludeP(Z.44)1.67.33,P(Z.92)P(Z.92)1.821.179,andP(1Z.5).692.159.533.Anotherusefulexpressionisthat,foranyc0,P(Zc)P(Zc)P(Zc)(B.39)2P(Zc)2[1(c)].Thus,theprobabilitythattheabsolutevalueofZisbiggerthansomepositiveconstantcissimplytwicetheprobabilityP(Zc);thisreflectsthesymmetryofthestandardnormaldistribution.Inmostapplications,westartwithanormallydistributedrandomvariable,X~22Normal(,),whereisdifferentfromzero,and1.Anynormalrandomvari-ablecanbeturnedintoastandardnormalusingthefollowingproperty.PROPERTYNORMAL.12IfX~Normal(,),then(X)/~Normal(0,1).PropertyNormal.1showshowtoturnanynormalrandomvariableintoastandardnor-mal.Thus,supposeX~Normal(3,4),andwewouldliketocomputeP(X1).ThestepsalwaysinvolvethenormalizationofXtoastandardnormal:X3P(X1)P(X313)P12P(Z1)(1).159.691\nxd7/14/998:57PMPage692AppendixBFundamentalsofProbabilityEXAMPLEB.6(ProbabilitiesforaNormalRandomVariable)First,letuscomputeP(2X6)whenX~Normal(4,9)(whetherweuseorisirrel-evantbecauseXisacontinuousrandomvariable).Now,24X464P(2X6)PP(2/3Z2/3)333(.67)(.67).749.251.498.Now,letuscomputeP(X2):P(X2)P(X2)P(X2)2P(X2)X4242P2P(Z.67)332[1(.67)].772.AdditionalPropertiesoftheNormalDistributionWeendthissubsectionbycollectingseveralotherfactsaboutnormaldistributionsthatwewilllateruse.PROPERTYNORMAL.2222IfX~Normal(,),thenaXb~Normal(ab,a).Thus,ifX~Normal(1,9),thenY2X3isdistributedasnormalwithmean22E(X)35andvariance2936;sd(Y)2sd(X)236.Earlierwediscussedhow,ingeneral,zerocorrelationandindependencearenotthesame.Inthecaseofnormallydistributedrandomvariables,itturnsoutthatzerocorre-lationsufficesforindependence.PROPERTYNORMAL.3IfXandYarejointlynormallydistributed,thentheyareindependentifandonlyifCov(X,Y)0.PROPERTYNORMAL.4Anylinearcombinationofindependent,identicallydistributednormalrandomvari-ableshasanormaldistribution.Forexample,letXi,i1,2,and3,beindependentrandomvariablesdistributedas2Normal(,).DefineWX12X23X3.Then,Wisnormallydistributed;wemustsimplyfinditsmeanandvariance.Now,E(W)E(X1)2E(X2)3E(X3)230.692\nxd7/14/998:57PMPage693AppendixBFundamentalsofProbabilityAlso,2Var(W)Var(X1)4Var(X2)9Var(X3)14.PropertyNormal.4alsoimpliesthattheaverageofindependent,normallydistrib-utedrandomvariableshasanormaldistribution.IfY1,Y2,…,Ynareindependentran-2domvariablesandeachisdistributedasNormal(,),then2Y~Normal(,/n).(B.40)Thisresultiscriticalforstatisticalinferenceaboutthemeaninanormalpopulation.TheChi-SquareDistributionThechi-squaredistributionisobtaineddirectlyfromindependent,standardnormalran-domvariables.LetZi,i1,2,…,n,beindependentrandomvariables,eachdistributedasstandardnormal.DefineanewrandomvariableasthesumofthesquaresoftheZi:n2XZi.(B.41)i1Then,Xhaswhatisknownasachi-squaredistributionwithndegreesoffreedom(or2dfforshort).WewritethisasX~n.Thedfinachi-squaredistributioncorrespondstothenumberoftermsinthesum(B.41).Theconceptofdegreesoffreedomwillplayanimportantroleinourstatisticalandeconometricanalyses.Thepdfforchi-squaredistributionswithvaryingdegreesoffreedomisgiveninFigureB.9;wewillnotneedtheformulaforthispdf,andsowedonotreproduceithere.Fromequation(B.41),itisclearthatachi-squarerandomvariableisalwaysnon-negative,andthat,unlikethenormaldistribution,thechi-squaredistributionisnotsym-2metricaboutanypoint.ItcanbeshownthatifX~n,thentheexpectedvalueofXisn[thenumberoftermsin(B.41)],andthevarianceofXis2n.ThetDistributionThetdistributionistheworkhorseinclassicalstatisticsandmultipleregressionanaly-sis.Weobtainatdistributionfromastandardnormalandachi-squarerandomvariable.LetZhaveastandardnormaldistributionandletXhaveachi-squaredistributionwithndegreesoffreedom.Further,assumethatZandXareindependent.Then,theran-domvariableZT(B.42)X/nhasatdistributionwithndegreesoffreedom.WewilldenotethisbyT~tn.Thetdis-tributiongetsitsdegreesoffreedomfromthechi-squarerandomvariableinthedenom-inatorof(B.42).Thepdfofthetdistributionhasashapesimilartothatofthestandardnormaldis-tribution,exceptthatitismorespreadoutandthereforehasmoreareainthetails.The693\nxd7/14/998:57PMPage694AppendixBFundamentalsofProbabilityFigureB.9Thechi-squaredistributionwithvariousdegreesoffreedom.f(x)df=2df=4df=8xexpectedvalueofatdistributedrandomvariableiszero(strictlyspeaking,theexpectedvalueexistsonlyforn1),andthevarianceisn/(n2)forn2.(Thevariancedoesnotexistforn2becausethedistributionissospreadout.)Thepdfofthetdistribu-tionisplottedinFigureB.10forvariousdegreesoffreedom.Asthedegreesoffreedomgetslarge,thetdistributionapproachesthestandardnormaldistribution.TheFDistributionAnotherimportantdistributionforstatisticsandeconometricsistheFdistribution.Inparticular,theFdistributionwillbeusedfortestinghypothesesinthecontextofmul-tipleregressionanalysis.22TodefineanFrandomvariable,letX1~k1andX2~k2andassumethatX1andX2areindependent.Then,therandomvariable(X1/k1)F(B.43)(X2/k2)hasanFdistributionwith(k1,k2)degreesoffreedom.WedenotethisasF~Fk1,k2.ThepdfoftheFdistributionwithdifferentdegreesoffreedomisgiveninFigureB.11.694\nxd7/14/998:57PMPage695AppendixBFundamentalsofProbabilityFigureB.10Thetdistributionwithvariousdegreesoffreedom.df=24df=2df=1–303TheorderofthedegreesoffreedominFk1,k2iscritical.Theintegerk1isoftencalledthenumeratordegreesoffreedombecauseitisassociatedwiththechi-squarevariableinthenumerator.Likewise,theintegerk2iscalledthedenominatordegreesoffreedombecauseitisassociatedwiththechi-squarevariableinthedenominator.Thiscanbealittletrickysince(B.43)canalsobewrittenas(X1k2)/(X2k1),sothatk1appearsinthedenominator.Justrememberthatthenumeratordfistheintegerassociatedwiththechi-squarevariableinthenumeratorof(B.43),andsimilarlyforthedenominatordf.SUMMARYInthisappendix,wehavereviewedtheprobabilityconceptsthatareneededinecono-metrics.Mostoftheconceptsshouldbefamiliarfromyourintroductorycourseinprob-abilityandstatistics.Someofthemoreadvancedtopics,suchasfeaturesofconditionalexpectations,donotneedtobemasterednow—thereistimeforthatwhenthesecon-ceptsariseinthecontextofregressionanalysisinPart1.Inanintroductorystatisticscourse,thefocusisoncalculatingmeans,variances,covariances,andsoon,forparticulardistributions.InPart1,wewillnotneedsuchcal-695\nxd7/14/998:57PMPage696AppendixBFundamentalsofProbabilityFigureB.11TheFk,kdistributionforvariousdegreesoffreedom,k1andk2.12f(x)df=2,8df=6,8df=6,200xculations:wemostlyrelyonthepropertiesofexpectations,variances,andsoon,thathavebeenstatedinthisappendix.KEYTERMSBernoulli(orBinary)RandomVariableIndependentRandomVariablesBinomialDistributionJointDistributionChi-SquareDistributionLawofIteratedExpectationsConditionalDistributionMedianConditionalExpectationNormalDistributionContinuousRandomVariablePairwiseUncorrelatedRandomVariablesCorrelationCoefficientProbabilityDensityFunction(pdf)CovarianceRandomVariableCumulativeDistributionFunction(cdf)StandardDeviationDegreesofFreedomStandardNormalDistributionDiscreteRandomVariableStandardizedRandomVariableExpectedValuetDistributionExperimentVarianceFDistribution696\nxd7/14/998:57PMPage697AppendixBFundamentalsofProbabilityPROBLEMSB.1SupposethatahighschoolstudentispreparingtotaketheSATexam.ExplainwhyhisorhereventualSATscoreisproperlyviewedasarandomvariable.B.2LetXbearandomvariabledistributedasNormal(5,4).Findtheprobabilitiesofthefollowingevents:(i)P(X6)(ii)P(X4)(iii)P(X51)B.3Muchismadeofthefactthatcertainmutualfundsoutperformthemarketyearafteryear(thatis,thereturnfromholdingsharesinthemutualfundishigherthanthereturnfromholdingaportfoliosuchastheS&P500).Forconcreteness,consideraten-yearperiodandletthepopulationbethe4,170mutualfundsreportedintheWallStreetJournalon1/6/95.Bysayingthatperformancerelativetothemarketisrandom,wemeanthateachfundhasa50–50chanceofoutperformingthemarketinanyyearandthatperformanceisindependentfromyeartoyear.(i)Ifperformancerelativetothemarketistrulyrandom,whatistheprobabilitythatanyparticularfundoutperformsthemarketinall10years?(ii)Findtheprobabilitythatatleastonefundoutof4,170fundsoutper-formsthemarketinall10years.Whatdoyoumakeofyouranswer?(iii)Ifyouhaveastatisticalpackagethatcomputesbinomialprobabilities,findtheprobabilitythatatleastfivefundsoutperformthemarketinall10years.B.4ForarandomlyselectedcountyintheUnitedStates,letXrepresenttheproportionofadultsoverage65whoareemployed,ortheelderlyemploymentrate.Then,Xisrestrictedtoavaluebetweenzeroandone.Supposethatthecumulativedistribution23functionforXisgivenbyF(x)3x2xfor0x1.Findtheprobabilitythattheelderlyemploymentrateisatleast.6(60%).B.5JustpriortojuryselectionforO.J.Simpson’smurdertrialin1995,apollfoundthatabout20%oftheadultpopulationbelievedSimpsonwasinnocent(aftermuchofthephysicalevidenceinthecasehadbeenrevealedtothepublic).Ignorethefactthatthis20%isanestimatebasedonasubsamplefromthepopulation;forillustration,takeitasthetruepercentageofpeoplewhothoughtSimpsonwasinnocentpriortojuryselection.Assumethatthe12jurorswereselectedrandomlyandindependentlyfromthepopulation(althoughthisturnedoutnottobetrue).(i)FindtheprobabilitythatthejuryhadatleastonememberwhobelievedinSimpson’sinnocencepriortojuryselection.(Hint:DefinetheBinomial(12,.20)randomvariableXtobethenumberofjurorsbeliev-inginSimpson’sinnocence.)(ii)FindtheprobabilitythatthejuryhadatleasttwomemberswhobelievedinSimpson’sinnocence.[Hint:P(X2)1P(X1),andP(X1)P(X0)P(X1).]697\nxd7/14/998:57PMPage698AppendixBFundamentalsofProbabilityB.6(Requirescalculus)LetXdenotetheprisonsentence,inyears,forpeoplecon-victedofautotheftinaparticularstateintheUnitedStates.SupposethatthepdfofXisgivenby2f(x)(1/9)x,0x3.Useintegrationtofindtheexpectedprisonsentence.B.7Ifabasketballplayerisa74%free-throwshooter,then,onaverage,howmanyfreethrowswillheorshemakeinagamewitheightfree-throwattempts?B.8Supposethatacollegestudentistakingthreecourses:atwo-creditcourse,athree-creditcourse,andafour-creditcourse.Theexpectedgradeinthetwo-creditcourseis3.5,whiletheexpectedgradeinthethree-andfour-creditcoursesis3.0.Whatistheexpectedoverallgradepointaverageforthesemester?(Rememberthateachcoursegradeisweightedbyitsshareofthetotalnumberofunits.)B.9LetXdenotetheannualsalaryofuniversityprofessorsintheUnitedStates,mea-suredinthousandsofdollars.Supposethattheaveragesalaryis52.3,withastandarddeviationof14.6.Findthemeanandstandarddeviationwhensalaryismeasuredindollars.B.10Supposethatatalargeuniversity,collegegradepointaverage,GPA,andSATscore,SAT,arerelatedbytheconditionalexpectationE(GPASAT).70.002SAT.(i)FindtheexpectedGPAwhenSAT800.FindE(GPASAT1,400).Commentonthedifference.(ii)IftheaverageSATintheuniversityis1,100,whatistheaverageGPA?(Hint:UsePropertyCE.4.)698\n\nxd7/14/999:21PMPage699AppendixCFundamentalsofMathematicalStatisticsC.1POPULATIONS,PARAMETERS,ANDRANDOMSAMPLINGStatisticalinferenceinvolveslearningsomethingaboutapopulationgiventheavail-abilityofasamplefromthatpopulation.Bypopulation,wemeananywell-definedgroupofsubjects,whichcouldbeindividuals,firms,cities,ormanyotherpossibilities.By“learning,”wecanmeanseveralthings,whicharebroadlydividedintothecate-goriesofestimationandhypothesistesting.Acoupleofexamplesmayhelpyouunderstandtheseterms.InthepopulationofallworkingadultsintheUnitedStates,laboreconomistsareinterestedinlearningaboutthereturntoeducation,asmeasuredbytheaveragepercentageincreaseinearningsgivenanotheryearofeducation.Itwouldbeimpracticalandcostlytoobtaininforma-tiononearningsandeducationfortheentireworkingpopulationintheUnitedStates,butwecanobtaindataonasubsetofthepopulation.Usingthedatacollected,alaboreconomistmayreportthathisorherbestestimateofthereturntoanotheryearofedu-cationis7.5%.Thisisanexampleofapointestimate.Or,sheorhemayreportarange,suchas“thereturntoeducationisbetween5.6%and9.4%.”Thisisanexampleofanintervalestimate.Anurbaneconomistmightwanttoknowwhetherneighborhoodcrimewatchpro-gramsareassociatedwithlowercrimerates.Aftercomparingcrimeratesofneighbor-hoodswithandwithoutsuchprogramsinasamplefromthepopulation,heorshecandrawoneoftwoconclusions:neighborhoodwatchprogramsdoaffectcrime,ortheydonot.Thisexamplefallsundertherubricofhypothesistesting.Thefirststepinstatisticalinferenceistoidentifythepopulationofinterest.Thismayseemobvious,butitisimportanttobeveryspecific.Oncewehaveidentifiedthepopulation,wecanspecifyamodelforthepopulationrelationshipofinterest.Suchmodelsinvolveprobabilitydistributionsorfeaturesofprobabilitydistribu-tions,andthesedependonunknownparameters.Parametersaresimplyconstantsthatdeterminethedirectionsandstrengthsofrelationshipsamongvariables.Inthelaboreconomicsexampleabove,theparameterofinterestisthereturntoeducationinthepopulation.699\nxd7/14/999:21PMPage700AppendixCFundamentalsofMathematicalStatisticsSamplingForreviewingstatisticalinference,wefocusonthesimplestpossiblesetting.LetYbearandomvariablerepresentingapopulationwithaprobabilitydensityfunctionf(y;),whichdependsonthesingleparameter.Theprobabilitydensityfunction(pdf)ofYisassumedtobeknownexceptforthevalueof;differentvaluesofimplydifferentpopulationdistributions,andthereforeweareinterestedinthevalueof.Ifwecanobtaincertainkindsofsamplesfromthepopulation,thenwecanlearnsomethingabout.Theeasiestsamplingschemetodealwithisrandomsampling.RANDOMSAMPLINGIfY1,Y2,…,Ynareindependentrandomvariableswithacommonprobabilitydensityfunctionf(y;),then{Y1,…,Yn}issaidtobearandomsamplefromf(y;)[oraran-domsamplefromthepopulationrepresentedbyf(y;)].When{Y1,…,Yn}isarandomsamplefromthedensityf(y;),wealsosaythattheYiareindependent,identicallydistributed(ori.i.d.)samplesfromf(y;).Insomecases,wewillnotneedtoentirelyspecifywhatthecommondistributionis.TherandomnatureofY1,Y2,…,Yninthedefinitionofrandomsamplingreflectsthefactthatmanydifferentoutcomesarepossiblebeforethesamplingisactuallycar-riedout.Forexample,iffamilyincomeisobtainedforasampleofn100familiesintheUnitedStates,theincomesweobservewillusuallydifferforeachdifferentsampleof100families.Onceasampleisobtained,wehaveasetofnumbers,say{y1,y2,…,yn},whichconstitutethedatathatweworkwith.Whetherornotitisappro-priatetoassumethesamplecamefromarandomsamplingschemerequiresknowledgeabouttheactualsamplingprocess.RandomsamplesfromaBernoullidistributionareoftenusedtoillustratestatisticalconcepts,andtheyalsoariseinempiricalapplications.IfY1,Y2,…,YnareindependentrandomvariablesandeachisdistributedasBernoulli(),sothatP(Yi1)andP(Yi0)1,then{Y1,Y2,…,Yn}constitutesarandomsamplefromtheBernoulli()distribution.Asanillustration,considertheairlinereservationexamplecarriedalonginAppendixB.EachYidenoteswhethercustomerishowsupforhisorherreservation;Yi1ifpassengerishowsup,andYi0otherwise.Here,istheprobabilitythatarandomlydrawnpersonfromthepopulationofallpeoplewhomakeairlinereservationsshowsupforhisorherreservation.Formanyotherapplications,randomsamplescanbeassumedtobedrawnfroma2normaldistribution.If{Y1,…,Yn}isarandomsamplefromtheNormal(,)popula-tion,thenthepopulationischaracterizedbytwoparameters,themeanandthevari-22ance.Primaryinterestusuallyliesin,butisofinterestinitsownrightbecause2makinginferencesaboutoftenrequireslearningabout.C.2FINITESAMPLEPROPERTIESOFESTIMATORSInthissection,westudywhatarecalledfinitesamplepropertiesofestimators.Theterm“finitesample”comesfromthefactthatthepropertiesholdforasampleofanysize,nomatterhowlargeorsmall.Sometimes,thesearecalledsmallsampleproperties.In700\nxd7/14/999:21PMPage701AppendixCFundamentalsofMathematicalStatisticsSectionC.3,wecover“asymptoticproperties,”whichhavetodowiththebehaviorofestimatorsasthesamplesizegrowswithoutbound.EstimatorsandEstimatesTostudypropertiesofestimators,wemustdefinewhatwemeanbyanestimator.Givenarandomsample{Y1,Y2,…,Yn}drawnfromapopulationdistributionthatdependsonanunknownparameter,anestimatorofisarulethatassignseachpossibleoutcomeofthesampleavalueof.Theruleisspecifiedbeforeanysamplingiscarriedout;inparticular,theruleisthesame,regardlessofthedataactuallyobtained.Asanexampleofanestimator,let{Y1,…,Yn}bearandomsamplefromapopula-tionwithmean.Anaturalestimatorofistheaverageoftherandomsample:n1Y¯nYi.(C.1)i1Y¯iscalledthesampleaveragebut,unlikeinAppendixAwherewedefinedthesam-pleaverageofasetofnumbersasadescriptivestatistic,Y¯isnowviewedasanestima-tor.GivenanyoutcomeoftherandomvariablesY1,…,Yn,weusethesameruletoestimate:wesimplyaveragethem.Foractualdataoutcomes{y1,…,yn},theestimateisjusttheaverageinthesample:y¯(y1y2…yn)/n.EXAMPLEC.1(CityUnemploymentRates)Supposeweobtainthefollowingsampleofunemploymentratesfor10citiesintheUnitedStates:CityUnemploymentRate15.126.439.244.157.568.372.6continued701\nxd7/14/999:21PMPage702AppendixCFundamentalsofMathematicalStatisticsCityUnemploymentRate83.595.8107.5OurestimateoftheaveragecityunemploymentrateintheUnitedStatesisy¯6.0.Eachsamplegenerallyresultsinadifferentestimate.Buttheruleforobtainingtheestimateisthesame,regardlessofwhichcitiesappearinthesample,orhowmany.Moregenerally,anestimatorWofaparametercanbeexpressedasanabstractmathematicalformula:Wh(Y1,Y2,…,Yn),(C.2)forsomeknownfunctionhoftherandomvariablesY1,Y2,…,Yn.Aswiththespecialcaseofthesampleaverage,Wisarandomvariablebecauseitdependsontherandomsample:asweobtaindifferentrandomsamplesfromthepopulation,thevalueofWcanchange.Whenaparticularsetofnumbers,say{y1,y2,…,yn},ispluggedintothefunc-tionh,weobtainanestimateof,denotedwh(y1,…,yn).Sometimes,Wiscalledapointestimatorandwapointestimatetodistinguishthesefromintervalestimatorsandestimates,whichwewillcometoinSectionC.4.Forevaluatingestimationprocedures,westudyvariouspropertiesoftheprobabil-itydistributionoftherandomvariableW.Thedistributionofanestimatorisoftencalleditssamplingdistribution,sincethisdistributiondescribesthelikelihoodofvariousoutcomesofWacrossdifferentrandomsamples.Becausethereareunlimitedrulesforcombiningdatatoestimateparameters,weneedsomesensiblecriteriaforchoosingamongestimators,oratleastforeliminatingsomeestimatorsfromconsideration.Therefore,wemustleavetherealmofdescriptivestatistics,wherewecomputethingssuchassampleaveragetosimplysummarizeabodyofdata.Inmathematicalstatistics,westudythesamplingdistributionsofestimators.UnbiasednessInprinciple,theentiresamplingdistributionofWcanbeobtainedgiventheprobabil-itydistributionofYiandthefunctionh.ItisusuallyeasiertofocusonafewfeaturesofthedistributionofWinevaluatingitasanestimatorof.Thefirstimportantpropertyofanestimatorinvolvesitsexpectedvalue.UNBIASEDESTIMATORAnestimator,Wof,isunbiasedif702\nxd7/14/999:21PMPage703AppendixCFundamentalsofMathematicalStatisticsE(W),(C.3)forallpossiblevaluesof.Ifanestimatorisunbiased,thenitsprobabilitydistributionhasanexpectedvalueequaltotheparameteritissupposedtobeestimating.Unbiasednessdoesnotmeanthattheestimatewegetwithanyparticularsampleisequalto,orevenverycloseto.Rather,ifwecouldindefinitelydrawrandomsamplesonYfromthepopulation,computeanestimateeachtime,andthenaveragetheseestimatesoverallrandomsamples,wewouldobtain.Thisthoughtexperimentisabstract,becauseinmostapplications,wejusthaveonerandomsampletoworkwith.Foranestimatorthatisnotunbiased,wedefineitsbiasasfollows.BIASOFANESTIMATORIfWisanestimatorof,itsbiasisdefinedasBias(W)E(W).(C.4)FigureC.1showstwoestimators,thefirstofwhichisunbiasedandthesecondofwhichhasapositivebias.FigureC.1Anunbiasedestimator,W1,andanestimatorwithpositivebias,W2.f(w)pdfofWpdfofW21=E(W1)E(W2)w703\nxd7/14/999:21PMPage704AppendixCFundamentalsofMathematicalStatisticsTheunbiasednessofanestimatorandthesizeofanypossiblebiasdependonthedistributionofYandonthefunctionh.ThedistributionofYisusuallybeyondourcon-trol(althoughweoftenchooseamodelforthisdistribution):itmaybedeterminedbynatureorsocialforces.Butthechoiceoftherulehisours,andifwewantanunbiasedestimator,thenwemustchoosehaccordingly.Someestimatorscanbeshowntobeunbiasedquitegenerally.WenowshowthatthesampleaverageY¯isanunbiasedestimatorofthepopulationmean,regardlessoftheunderlyingpopulationdistribution.Weusethepropertiesofexpectedvalues(E.1andE.2)thatwecoveredinSectionB.3:nnnE(Y¯)E(1/n)Yi(1/n)EYi(1/n)E(Yi)i1i1i1n(1/n)(1/n)(n).i12Forhypothesistesting,wewillneedtoestimatethevariancefromapopulationwithmean.Letting{Y1,…,Yn}denotetherandomsamplefromthepopulationwith2E(Y)andVar(Y),definetheestimatorasn122S(YiY¯),(C.5)n1i122whichisusuallycalledthesamplevariance.ItcanbeshownthatSisunbiasedfor:22E(S).Thedivisionbyn1,ratherthann,accountsforthefactthatthemean2isestimatedratherthanknown.Ifwereknown,anunbiasedestimatorofwouldben12n(Yi),butisrarelyknowninpractice.i1Althoughunbiasednesshasacertainappealasapropertyforanestimator—indeed,itsantonym,“biased”,hasdecidedlynegativeconnotations—itisnotwithoutitsprob-lems.Oneweaknessofunbiasednessisthatsomereasonable,andevensomeverygoodestimators,arenotunbiased.Wewillseeanexampleshortly.Anotherimportantweaknessofunbiasednessisthatunbiasedestimatorsexistthatareactuallyquitepoorestimators.Considerestimatingthemeanfromapopulation.RatherthanusingthesampleaverageY¯toestimate,supposethat,aftercollectingasampleofsizen,wediscardalloftheobservationsexceptthefirst.Thatis,ouresti-matorofissimplyWY1.ThisestimatorisunbiasedsinceE(Y1).Hopefully,yousensethatignoringallbutthefirstobservationisnotaprudentapproachtoesti-mation:itthrowsoutmostoftheinformationinthesample.Forexample,withn100,weobtain100outcomesoftherandomvariableY,butthenweuseonlythefirstofthesetoestimateE(Y).TheSamplingVarianceofEstimatorsTheexampleattheendoftheprevioussubsectionshowsthatweneedadditionalcrite-riainordertoevaluateestimators.Unbiasednessonlyensuresthattheprobabilitydis-tributionofanestimatorhasameanvalueequaltotheparameteritissupposedtobeestimating.Thisisfine,butwealsoneedtoknowhowspreadoutthedistributionofan704\nxd7/14/999:21PMPage705AppendixCFundamentalsofMathematicalStatisticsestimatoris.Anestimatorcanbeequalto,onaverage,butitcanalsobeveryfarawaywithlargeprobability.InFigureC.2,W1andW2arebothunbiasedestimatorsof.ButthedistributionofW1ismoretightlycenteredabout:theprobabilitythatW1isgreaterthananygivendistancefromislessthantheprobabilitythatW2isgreaterthanthatsamedistancefrom.UsingW1asourestimatormeansthatitislesslikelythatwewillobtainarandomsamplethatyieldsanestimateveryfarfrom.TosummarizethesituationshowninFigureC.2,werelyonthevariance(orstan-darddeviation)ofanestimator.Recallthatthisgivesasinglemeasureofthedisper-sioninthedistribution.Thevarianceofanestimatorisoftencalleditssamplingvariance,sinceitisthevarianceassociatedwithasamplingdistribution.Remember,thesamplingvarianceisnotarandomvariable;itisaconstant,butitmightbeunknown.Wenowobtainthevarianceofthesampleaverageforestimatingthemeanfromapopulation:nnn22Var(Y¯)Var(1/n)Yi(1/n)VarYi(1/n)Var(Yi)i1i1i1(C.6)n22222(1/n)(1/n)(n)/n.i1FigureC.2Thesamplingdistributionsoftwounbiasedestimatorsof.f(w)pdfofW1pdfofW2w705\nxd7/14/999:21PMPage706AppendixCFundamentalsofMathematicalStatisticsNoticehowweusedthepropertiesofvariancefromSectionsB.3andB.4(VAR.2andVAR.4),aswellastheindependenceoftheYi.Tosummarize:If{Yi:i1,2,…,n}isa2randomsamplefromapopulationwithmeanandvariance,thenY¯hasthesame2meanasthepopulation,butitssamplingvarianceequalsthepopulationvariance,,overthesamplesize.2AnimportantimplicationofVar(Y¯)/nisthatitcanbemadeveryclosetozerobyincreasingthesamplesizen.Thisisakeyfeatureofareasonableestimator,andwereturntoitinSectionC.3.AssuggestedbyFigureC.2,amongunbiasedestimators,weprefertheestimatorwiththesmallestvariance.Thisallowsustoeliminatecertainestimatorsfromconsid-2eration.Forarandomsamplefromapopulationwithmeanandvariance,weknow2thatY¯isunbiased,andVar(Y¯)/n.WhatabouttheestimatorY1,whichisjustthe2firstobservationdrawn?SinceY1isarandomdrawfromthepopulation,Var(Y1).Thus,thedifferencebetweenVar(Y1)andVar(Y¯)canbelargeevenforsmallsample2sizes.Ifn10,thenVar(Y1)istentimesaslargeasVar(Y¯)/10.ThisgivesusaformalwayofexcludingY1asanestimatorof.Toemphasizethispoint,TableC.1containstheoutcomeofasmallsimulationstudy.UsingthestatisticalpackageStata,20randomsamplesofsize10weregenerated2fromanormaldistribution,with2and1;weareinterestedinestimatinghere.Foreachofthe20randomsamples,wecomputetwoestimates,y1andy¯;thesevaluesarelistedinTableC.1.Ascanbeseenfromthetable,thevaluesfory1aremuchmorespreadoutthanthosefory¯:y1rangesfrom0.64to4.27,whiley¯rangesonlyfrom1.16to2.58.Further,in16outof20cases,y¯iscloserthany1to2.Theaver-ageofy1acrossthesimulationsisabout1.89,whilethatfory¯is1.96.Thefactthattheseaveragesarecloseto2illustratestheunbiasednessofbothestimators(andwecouldgettheseaveragescloserto2bydoingmorethan20replications).ButcomparingjusttheaverageoutcomesacrossrandomdrawsmasksthefactthatthesampleaverageY¯isfarsuperiortoY1asanestimatorof.TableC.1SimulationofEstimatorsforaNormal(,1)Distributionwith2Replicationy1y¯10.641.9821.061.4334.271.6541.031.8853.162.34continued706\nxd7/14/999:21PMPage707AppendixCFundamentalsofMathematicalStatisticsTableC.1(concluded)Replicationy1y¯62.772.5871.681.5882.982.2392.251.96102.042.11110.952.15121.361.93132.622.02142.972.10151.932.18161.142.10172.081.94181.522.21191.331.16201.211.75EfficiencyComparingthevariancesofY¯andY1intheprevioussubsectionisanexampleofagen-eralapproachtocomparingdifferentunbiasedestimators.RELATIVEEFFICIENCYIfW1andW2aretwounbiasedestimatorsof,W1isefficientrelativetoW2whenVar(W1)Var(W2)forall,withstrictinequalityforatleastonevalueof.Earlier,weshowedthat,forestimatingthepopulationmean,Var(Y¯)Var(Y1)forany2valueofwhenevern1.Thus,Y¯isefficientrelativetoY1forestimating.Wecan-707\nxd7/14/999:21PMPage708AppendixCFundamentalsofMathematicalStatisticsnotalwayschoosebetweenunbiasedestimatorsbasedonthesmallestvariancecrite-rion:giventwounbiasedestimatorsof,onecanhavesmallervariancefromsomeval-uesof,whiletheothercanhavesmallervarianceforothervaluesof.Ifwerestrictourattentiontoacertainclassofestimators,wecanshowthatthesam-pleaveragehasthesmallestvariance.ProblemC.2asksyoutoshowthatY¯hasthesmallestvarianceamongallunbiasedestimatorsthatarealsolinearfunctionsofY1,Y2,…,Yn.TheassumptionsarethattheYihavecommonmeanandvariance,andtheyarepairwiseuncorrelated.Ifwedonotrestrictourattentiontounbiasedestimators,thencomparingvariancesismeaningless.Forexample,whenestimatingthepopulationmean,wecanuseatriv-ialestimatorthatisequaltozero,regardlessofthesamplethatwedraw.Naturally,thevarianceofthisestimatoriszero(sinceitisthesamevalueforeveryrandomsample).Butthebiasofthisestimatoris,andsoitisaverypoorestimatorwhenislarge.Onewaytocompareestimatorsthatarenotnecessarilyunbiasedistocomputethemeansquarederror(MSE)oftheestimators.IfWisanestimatorof,thentheMSE2ofWisdefinedasMSE(W)E[(W)].TheMSEmeasureshowfar,onaverage,2theestimatorisawayfrom.ItcanbeshownthatMSE(W)Var(W)[Bias(W)],sothatMSE(W)dependsonthevarianceandbias(ifanyispresent).Thisallowsustocomparetwoestimatorswhenoneorbotharebiased.C.3ASYMPTOTICORLARGESAMPLEPROPERTIESOFESTIMATORSInSectionC.2,weencounteredtheestimatorY1forthepopulationmean,andwesawthat,eventhoughitisunbiased,itisapoorestimatorbecauseitsvariancecanbemuchlargerthanthatofthesamplemean.OnenotablefeatureofY1isthatithasthesamevarianceforanysamplesize.Itseemsreasonabletorequireanyestimationproceduretoimproveasthesamplesizeincreases.Forestimatingapopulationmean,Y¯improvesinthesensethatitsvariancegetssmallerasngetslarger;Y1doesnotimproveinthissense.Wecanruleoutcertainsillyestimatorsbystudyingtheasymptoticorlargesamplepropertiesofestimators.Inaddition,wecansaysomethingpositiveaboutestimatorsthatarenotunbiasedandwhosevariancesarenoteasilyfound.Asymptoticanalysisinvolvesapproximatingthefeaturesofthesamplingdistribu-tionofanestimator.Theseapproximationsdependonthesizeofthesample.Unfortunately,wearenecessarilylimitedinwhatwecansayabouthow“large”asam-plesizeisneededforasymptoticanalysistobeappropriate;thisdependsontheunder-lyingpopulationdistribution.Butlargesampleapproximationshavebeenknowntoworkwellforsamplesizesassmallasn20.ConsistencyThefirstasymptoticpropertyofestimatorsconcernshowfartheestimatorislikelytobefromtheparameteritissupposedtobeestimatingasweletthesamplesizeincreaseindefinitely.708\nxd7/14/999:21PMPage709AppendixCFundamentalsofMathematicalStatisticsCONSISTENCYLetWnbeanestimatorofbasedonasampleY1,Y2,…,Ynofsizen.Then,Wnisaconsistentestimatorof,ifforevery0,P(Wn)*0asn*.(C.7)IfWnisnotconsistentfor,thenwesayitisinconsistent.WhenWnisconsistent,wealsosaythatistheprobabilitylimitofWn,writtenasplim(Wn).Unlikeunbiasedness—whichisafeatureofanestimatorforagivensamplesize—consistencyinvolvesthebehaviorofthesamplingdistributionoftheestimatorasthesamplesizengetslarge.Toemphasizethis,wehaveindexedtheestimatorbythesam-plesizeinstatingthisdefinition,andwewillcontinuewiththisconventionthroughoutthissection.Equation(C.7)lookstechnical,anditcanberatherdifficulttoestablishbasedonfundamentalprobabilityprinciples.Bycontrast,interpreting(C.7)isstraightforward.ItmeansthatthedistributionofWnbecomesmoreandmoreconcentratedabout,whichroughlymeansthatforlargersamplesizes,Wnislessandlesslikelytobeveryfarfrom.ThistendencyisillustratedinFigureC.3.FigureC.3Thesamplingdistributionsofaconsistentestimatorforthreesamplesizes.fW(w)nn=40n=16n=4w709\nxd7/14/999:21PMPage710AppendixCFundamentalsofMathematicalStatisticsIfanestimatorisnotconsistent,thenitdoesnothelpustolearnabout,evenwithanunlimitedamountofdata.Forthisreason,consistencyisaminimalrequirementofanestimatorusedinstatisticsoreconometrics.Wewillencounterestimatorsthatareconsistentundercertainassumptionsandinconsistentwhenthoseassumptionsfail.Whenestimatorsareinconsistent,wecanusuallyfindtheirprobabilitylimits,anditwillbeimportanttoknowhowfartheseprobabilitylimitsarefrom.Aswenotedearlier,unbiasedestimatorsarenotnecessarilyconsistent,butthosewhosevariancesshrinktozeroasthesamplesizegrowsareconsistent.Thiscanbestatedformally:IfWnisanunbiasedestimatorofandVar(Wn)*0asn*,thenplim(Wn).Unbiasedestimatorsthatusetheentiredatasamplewillusuallyhaveavariancethatshrinkstozeroasthesamplesizegrows,therebybeingconsistent.Agoodexampleofaconsistentestimatoristheaverageofarandomsampledrawn2fromapopulationwithandvariance.Wehavealreadyshownthatthesampleaver-2ageisunbiasedfor.Inequation(C.6),wederivedVar(Y¯n)/nforanysamplesizen.Therefore,Var(Y¯n)*0asn*,andsoY¯nisaconsistentestimatorof(inaddi-tiontobeingunbiased).TheconclusionthatY¯nisconsistentforholdsevenifVar(Y¯n)doesnotexist.Thisclassicresultisknownasthelawoflargenumbers(LLN).LAWOFLARGENUMBERSLetY1,Y2,…,Ynbeindependent,identicallydistributedrandomvariableswithmean.Then,plim(Y¯n).(C.8)Thelawoflargenumbersmeansthat,ifweareinterestedinestimatingthepopulationaverage,wecangetarbitrarilyclosetobychoosingasufficientlylargesample.Thisfundamentalresultcanbecombinedwithbasicpropertiesofplimstoshowthatfairlycomplicatedestimatorsareconsistent.PROPERTYPLIM.1Letbeaparameteranddefineanewparameter,g(),forsomecontinuousfunc-tiong().Supposethatplim(Wn).DefineanestimatorofbyGng(Wn).Then,plim(Gn).(C.9)Thisisoftenstatedasplimg(Wn)g(plimWn)(C.10)foracontinuousfunctiong().Theassumptionthatg()iscontinuousisatechnicalrequirementthathasoftenbeendescribednontechnicallyas“afunctionthatcanbegraphedwithoutliftingyourpencilfromthepaper.”Sinceallofthefunctionsweencounterinthistextarecontinuous,wedonotprovideaformaldefinitionofacontinuousfunction.Examplesofcontinuous710\nxd7/14/999:21PMPage711AppendixCFundamentalsofMathematicalStatistics2functionsareg()abforconstantsaandb,g(),g()1/,g(),g()exp(),andmanyvariantsonthese.Wewillnotneedtomentionthecontinuityassumptionagain.Asanimportantexampleofaconsistentbutbiasedestimator,considerestimating2thestandarddeviation,,fromapopulationwithmeanandvariance.Wealreadyn1222claimedthatthesamplevarianceSn(YiY¯)isunbiasedfor.Usingn1i12thelawoflargenumbersandsomealgebra,Sncanalsobeshowntobeconsistentfor222.ThenaturalestimatorofisSnSn(wherethesquarerootisalwaysthepositivesquareroot).Sn,whichiscalledthesamplestandarddeviation,isnotanunbiasedestimatorbecausetheexpectedvalueofthesquarerootisnotthesquarerootoftheexpectedvalue(seeSectionB.3).Nevertheless,byPLIM.1,plimSn22plimSn,soSnisaconsistentestimatorof.Herearesomeotherusefulpropertiesoftheprobabilitylimit:PROPERTYPLIM.2Ifplim(Tn)andplim(Un),then(i)plim(TnUn);(ii)plim(TnUn);(iii)plim(Tn/Un)/,provided0.Thesethreefactsaboutprobabilitylimitsallowustocombineconsistentestimatorsinavarietyofwaystogetotherconsistentestimators.Forexample,let{Y1,…,Yn}bearandomsampleofsizenonannualearningsfromthepopulationofworkerswithahighschooleducationanddenotethepopulationmeanbyY.Let{Z1,…,Zn}bearandomsampleonannualearningsfromthepopulationofworkerswithacollegeeducationanddenotethepopulationmeanbyZ.Wewishtoestimatethepercentagedifferenceinannualearningsbetweenthetwogroups,whichis100(ZY)/Y.(Thisisthepercentbywhichaverageearningsforcollegegraduatesdiffersfromaverageearningsforhighschoolgraduates.)SinceY¯nisconsistentforY,andZ¯nisconsistentforZ,itfollowsfromPLIM.1andpart(iii)ofPLIM.2thatGn100(Z¯nY¯n)/Y¯nisaconsistentestimatorof.GnisjustthepercentagedifferencebetweenZ¯nandY¯ninthesample,soitisanaturalestimator.Gnisnotanunbiasedestimatorof,butitisstillagoodestimatorunlessnissmall.AsymptoticNormalityConsistencyisapropertyofpointestimators.Whileitdoestellusthatthedistributionoftheestimatoriscollapsingaroundtheparameterasthesamplesizegetslarge,ittellsusessentiallynothingabouttheshapeofthatdistributionforagivensamplesize.Forconstructingintervalestimatorsandtestinghypotheses,weneedawaytoapproximatethedistributionofourestimators.Mosteconometricestimatorshavedistributionsthatarewell-approximatedbyanormaldistributionforlargesamples,whichmotivatesthefollowingdefinition.711\nxd7/14/999:21PMPage712AppendixCFundamentalsofMathematicalStatisticsASYMPTOTICNORMALITYLet{Zn:n1,2,…}beasequenceofrandomvariables,suchthatforallnumbersz,P(Znz)*(z)asn*,(C.11)where(z)isthestandardnormalcumulativedistributionfunction.Then,Znissaidtohaveanasymptoticstandardnormaldistribution.Inthiscase,weoftenwriteZn~ªNormal(0,1).(The“a”abovethetildastandsfor“asymptotically”or“approximately.”)Property(C.11)meansthatthecumulativedistributionfunctionforZngetscloserandclosertothecdfofthestandardnormaldistribution,asthesamplesizengetslarge.Whenasymptoticnormalityholds,forlargen,wehavetheapproximationP(Znz)(z).Thus,probabilitiesconcerningZncanbeapproximatedbystandardnormalprobabilities.Thecentrallimittheorem(CLT)isoneofthemostpowerfulresultsinprobabil-ityandstatistics.Itstatesthattheaveragefromarandomsampleforanypopulation(withfinitevariance),whenstandardized,hasanasymptoticstandardnormaldistribu-tion.CENTRALLIMITTHEOREM2Let{Y1,Y2,…,Yn}bearandomsamplewithmeanandvariance.Then,Y¯nZn,(C.12)/nhasanasymptoticstandardnormaldistribution.ThevariableZnin(C.12)isthestandardizedversionofY¯n:wehavesubtractedoffE(Y¯n)anddividedbysd(Y¯n)/n.Thus,regardlessofthepopulationdistribu-tionofY,Znhasmeanzeroandvarianceone,whichcoincideswiththemeanandvari-anceofthestandardnormaldistribution.Remarkably,theentiredistributionofZngetsarbitrarilyclosetothestandardnormaldistributionasngetslarge.Mostestimatorsencounteredinstatisticsandeconometricscanbewrittenasfunc-tionsofsampleaverages,inwhichcase,wecanapplythelawoflargenumbersandthecentrallimittheorem.Whentwoconsistentestimatorshaveasymptoticnormaldistrib-utions,wechoosetheestimatorwiththesmallestasymptoticvariance.Inadditiontothestandardizedsampleaveragein(C.12),manyotherstatisticsthatdependonsampleaveragesturnouttobeasymptoticallynormal.AnimportantoneisobtainedbyreplacingwithitsconsistentestimatorSninequation(C.12):Y¯n(C.13)S/nnalsohasanapproximatestandardnormaldistributionforlargen.Theexact(finitesam-ple)distributionsof(C.12)and(C.13)aredefinitelynotthesame,butthedifferenceisoftensmallenoughtobeignoredforlargen.712\nxd7/14/999:21PMPage713AppendixCFundamentalsofMathematicalStatisticsThroughoutthissection,eachestimatorhasbeensubscriptedbyntoemphasizethenatureofasymptoticorlargesampleanalysis.Continuingthisconventioncluttersthenotationwithoutprovidingadditionalinsight,oncethefundamentalsofasymptoticanalysisareunderstood.Henceforth,wedropthensubscriptandrelyonyoutoremem-berthatestimatorsdependonthesamplesize,andpropertiessuchasconsistencyandasymptoticnormalityrefertothegrowthofthesamplesizewithoutbound.C.4GENERALAPPROACHESTOPARAMETERESTIMATIONUptothispoint,wehaveusedthesampleaveragetoillustratethefiniteandlargesam-plepropertiesofestimators.Itisnaturaltoask:Aretheregeneralapproachestoesti-mationthatproduceestimatorswithgoodproperties,suchasunbiasedness,consistency,andefficiency?Theanswerisyes.Adetailedtreatmentofvariousapproachestoestimationisbeyondthescopeofthistext;here,weprovideonlyaninformaldiscussion.AthoroughdiscussionisgiveninLarsenandMarx(1986,Chapter5).MethodofMomentsGivenaparameterappearinginapopulationdistribution,thereareusuallymanywaystoobtainunbiasedandconsistentestimatorsof.TryingalldifferentpossibilitiesandcomparingthemonthebasisofthecriteriainSectionsC.2andC.3isnotpractical.Fortunately,somemethodshavebeenshowntohavegoodgeneralproperties,andforthemostpart,thelogicbehindthemisintuitivelyappealing.Intheprevioussections,wehaveseensomeexamplesofmethodofmomentspro-cedures.Basically,methodofmomentsestimationproceedsasfollows.TheparameterisshowntoberelatedtosomeexpectedvalueinthedistributionofY,usuallyE(Y)or2E(Y)(althoughmoreexoticchoicesaresometimesused).Suppose,forexample,thattheparameterofinterest,,isrelatedtothepopulationmeanasg()forsomefunctiong.SincethesampleaverageY¯isanunbiasedandconsistentestimatorof,itisnaturaltoreplacewithY¯,whichgivesustheestimatorg(Y¯)of.Theestimatorg(Y¯)isconsistentfor,andifg()isalinearfunctionof,theng(Y¯)isunbiasedaswell.Whatwehavedoneisreplacethepopulationmoment,,withitssamplecoun-terpart,Y¯.Thisiswherethename“methodofmoments”comesfrom.Wecovertwoadditionalmethodofmomentsestimatorsthatwillbeusefulforourdiscussionofregressionanalysis.Recallthatthecovariancebetweentworandomvari-ablesXandYisdefinedasXYE[(XX)(YY)].Themethodofmomentsn1suggestsestimatingXYbyn(XiX¯)(YiY¯).Thisisaconsistentestimatori1ofXY,butitturnsouttobebiasedforessentiallythesamereasonthatthesamplevari-anceisbiasedifn,ratherthann1,isusedasthedivisor.Thesamplecovarianceisdefinedasn1SXY(XiX¯)(YiY¯).(C.14)n1i1713\nxd7/14/999:21PMPage714AppendixCFundamentalsofMathematicalStatisticsItcanbeshownthatthisisanunbiasedestimatorofXY(andreplacingnwithn1makesnodifferenceasthesamplesizegrowsindefinitely,sothisestimatorisstillcon-sistent).AswediscussedinSectionB.4,thecovariancebetweentwovariablesisoftendif-ficulttointerpret.Usually,wearemoreinterestedincorrelation.SincethepopulationcorrelationisXYXY/(XY),themethodofmomentssuggestsestimatingXYasnS(XiX¯)(YiY¯)XYi1RXYSSn1/2n1/2,(C.15)XY22(XiX¯)(YiY¯)i1i1whichiscalledthesamplecorrelationcoefficient(orsamplecorrelationforshort).Noticethatwehavecanceledthedivisionbyn1inthesamplecovarianceandthesamplestandarddeviations.Infact,wecoulddivideeachofthesebyn,andwewouldarriveatthesamefinalformula.Itcanbeshownthatthesamplecorrelationcoefficientisalwaysintheinterval[1,1],asitshouldbe.BecauseSXY,SX,andSYareconsistentforthecorrespondingpopulationparameter,RXYisaconsistentestimatorofthepopulationcorrelation,XY.However,RXYisabiasedestimatorfortworeasons.First,SXandSYarebiasedestima-torsofXandY,respectively.Second,RXYisaratioofestimators,andsoitwouldnotbeunbiased,evenifSXandSYwere.Forourpurposes,thisisnotimportant,althoughthefactthatnounbiasedestimatorofXYexistsisaclassicalresultinmathematicalsta-tistics.MaximumLikelihoodAnothergeneralapproachtoestimationisthemethodofmaximumlikelihood,atopiccoveredinmanyintroductorystatisticscourses.Abriefsummaryinthesimplestcasewillsufficehere.Let{Y1,Y2,…,Yn}bearandomsamplefromthepopulationdistribu-tionf(y;).Becauseoftherandomsamplingassumption,thejointdistributionof{Y1,Y2,…,Yn}issimplytheproductofthedensities:f(y1;)f(y2;)f(yn;).Inthediscretecase,thisisP(Y1y1,Y2y2,…,Ynyn).Now,definethelikelihoodfunc-tionasL(;Y1,…,Yn)f(Y1;)f(Y2;)f(Yn;),(C.16)whichisarandomvariablebecauseitdependsontheoutcomeoftherandomsample{Y1,Y2,…,Yn}.Themaximumlikelihoodestimatorof,callitW,isthevalueofthatmaximizesthelikelihoodfunction(thisiswhywewriteLasafunctionof,fol-lowedbytherandomsample).Clearly,thisvaluedependsontherandomsample.Themaximumlikelihoodprinciplesaysthat,outofallthepossiblevaluesfor,thevaluethatmakesthelikelihoodoftheobserveddatalargestshouldbechosen.Intuitively,thisisareasonableapproachtoestimating.Maximumlikelihoodestimation(MLE)isusuallyconsistentandsometimesunbi-ased.Butsoaremanyotherestimators.ThewidespreadappealofMLEisthatitisgen-714\nxd7/14/999:21PMPage715AppendixCFundamentalsofMathematicalStatisticserallythemostasymptoticallyefficientestimatorwhenthepopulationmodelf(y;)iscorrectlyspecified.Inaddition,theMLEissometimestheminimumvarianceunbi-asedestimator;thatis,ithasthesmallestvarianceamongallunbiasedestimatorsof.[SeeLarsenandMarx(1986,Chapter5)forverificationoftheseclaims.]WeonlyneedtorelyonMLEforsomeoftheadvancedtopicsinPart3ofthetext.LeastSquaresAthirdkindofestimator,andonethatplaysamajorrolethroughoutthetext,iscalledaleastsquaresestimator.Wehavealreadyseenanexampleofleastsquares:thesam-plemean,Y¯,isaleastsquaresestimatorofthepopulationmean,.WealreadyknowY¯isamethodofmomentsestimator.Whatmakesitaleastsquaresestimator?Itcanbeshownthatthevalueofmwhichmakesthesumofsquareddeviationsn2(Yim)i1assmallaspossibleismY¯.Showingthisisnotdifficult,butweomitthealgebra.Forsomeimportantdistributions,includingthenormalandtheBernoulli,thesam-pleaverageY¯isalsothemaximumlikelihoodestimatorofthepopulationmean.Thus,theprinciplesofleastsquares,methodofmoments,andmaximumlikelihoodoftenresultinthesameestimator.Inothercases,theestimatorsaresimilarbutnotidentical.C.5INTERVALESTIMATIONANDCONFIDENCEINTERVALSTheNatureofIntervalEstimationApointestimateobtainedfromaparticularsampledoesnot,byitself,provideenoughinformationfortestingeconomictheoriesorforinformingpolicydiscussions.Apointestimatemaybetheresearcher’sbestguessatthepopulationvalue,but,byitsnature,itprovidesnoinformationabouthowclosetheestimateis“likely”tobetothepopula-tionparameter.Asanexample,supposearesearcherreports,onthebasisofarandomsampleofworkers,thatjobtraininggrantsincreasehourlywageby6.4%.Howarewetoknowwhetherornotthisisclosetotheeffectinthepopulationofworkerswhocouldhavebeentrained?Sincewedonotknowthepopulationvalue,wecannotknowhowcloseanestimateisforaparticularsample.However,wecanmakestatementsinvolv-ingprobabilities,andthisiswhereintervalestimationcomesin.Wealreadyknowonewayofassessingtheuncertaintyinanestimator:finditssamplingstandarddeviation.Reportingthestandarddeviationoftheestimator,alongwiththepointestimate,providessomeinformationontheaccuracyofourestimate.However,eveniftheproblemofthestandarddeviation’sdependenceonunknownpopulationparametersisignored,reportingthestandarddeviationalongwiththepointestimatemakesnodirectstatementaboutwherethepopulationvalueislikelytolieinrelationtotheestimate.Thislimitationisovercomebyconstructingaconfidenceinterval.Weillustratetheconceptofaconfidenceintervalwithanexample.SupposethepopulationhasaNormal(,1)distributionandlet{Y1,…,Yn}bearandomsamplefrom715\nxd7/14/999:21PMPage716AppendixCFundamentalsofMathematicalStatisticsthispopulation.(Weassumethatthevarianceofthepopulationisknownandequaltounityforthesakeofillustration;wethenshowwhattodointhemorerealisticcasethatthevarianceisunknown.)Thesampleaverage,Y¯,hasanormaldistributionwithmeanandvariance1/n:Y¯~Normal(,1/n).Fromthis,wecanstandardizeY¯,andsincethestandardizedversionofY¯hasastandardnormaldistribution,wehaveY¯P1.961.96.95.1/nTheeventinparenthesesisidenticaltotheeventY¯1.96/nY¯1.96/n,andsoP(Y¯1.96/nY¯1.96/n).95.(C.17)Equation(C.17)isinterestingbecauseittellsusthattheprobabilitythattherandominterval[Y¯1.96/n,Y¯1.96/n]containsthepopulationmeanis.95,or95%.Thisinformationallowsustoconstructanintervalestimateof,whichisobtainedbyplugginginthesampleoutcomeoftheaverage,y¯.Thus,[y¯1.96/n,y¯1.96/n](C.18)isanexampleofanintervalestimateof.Itisalsocalleda95%confidenceinterval.Ashorthandnotationforthisintervalisy¯1.96/n.Theconfidenceintervalinequation(C.18)iseasytocompute,oncethesampledata{y1,y2,…,yn}areobserved;y¯istheonlyfactorthatdependsonthedata.Forexample,supposethatn16andtheaverageofthe16datapointsis7.3.Then,the95%confi-denceintervalforis7.31.96/167.3.49,whichwecanwriteinintervalformas[6.81,7.79].Byconstruction,y¯7.3isinthecenterofthisinterval.Unlikeitscomputation,themeaningofaconfidenceintervalismoredifficulttounderstand.Whenwesaythatequation(C.18)isa95%confidenceintervalfor,wemeanthattherandominterval[Y¯1.96/n,Y¯1.96/n](C.19)containswithprobability.95.Inotherwords,beforetherandomsampleisdrawn,thereisa95%chancethat(C.19)contains.Equation(C.19)isanexampleofanin-tervalestimator.Itisarandominterval,sincetheendpointschangewithdifferentsamples.Aconfidenceintervalisofteninterpretedasfollows:“Theprobabilitythatisintheinterval(C.18)is.95.”Thisisincorrect.Oncethesamplehasbeenobservedandy¯hasbeencomputed,thelimitsoftheconfidenceintervalaresimplynumbers(6.81and7.79intheexamplejustgiven).Thepopulationparameter,,whileunknown,isalsojustsomenumber.Therefore,eitherisorisnotintheinterval(C.18)(andwewillneverknowwithcertaintlywhichisthecase).Probabilityplaysnorole,oncethecon-fidenceintervaliscomputedfortheparticulardataathand.Theprobabilisticinterpre-tationcomesfromthefactthatfor95%ofallrandomsamples,theconstructedconfidenceintervalwillcontain.716\nxd7/14/999:21PMPage717AppendixCFundamentalsofMathematicalStatisticsToemphasizethemeaningofaconfidenceinterval,TableC.2containscalculationsfor20randomsamples(orreplications)fromtheNormal(2,1)distributionwithsamplesizen10.Foreachofthe20samples,y¯isobtained,and(C.18)iscomputedasy¯1.96/10y¯.62(eachroundedtotwodecimals).Asyoucansee,theintervalchangeswitheachrandomsample.Nineteenofthe20intervalscontainthepopulationvalueof.Onlyforreplicationnumber19isnotintheconfidenceinterval.Inotherwords,95%ofthesamplesresultinaconfidenceintervalthatcontains.Thisdidnothavetobethecasewithonly20replications,butitworkedoutthatwayforthispartic-ularsimulation.TableC.2SimulatedConfidenceIntervalsfromaNormal(,1)Distributionwith2Replicationy¯95%IntervalContains?11.98(1.36,2.60)Yes21.43(0.81,2.05)Yes31.65(1.03,2.27)Yes41.88(1.26,2.50)Yes52.34(1.72,2.96)Yes62.58(1.96,3.20)Yes71.58(0.96,2.20)Yes82.23(1.61,2.85)Yes91.96(1.34,2.58)Yes102.11(1.49,2.73)Yes112.15(1.53,2.77)Yes121.93(1.31,2.55)Yes132.02(1.40,2.64)Yes142.10(1.48,2.72)Yes152.18(1.56,2.80)Yescontinued717\nxd7/14/999:21PMPage718AppendixCFundamentalsofMathematicalStatisticsTableC.2(concluded)Replicationy¯95%IntervalContains?162.10(1.48,2.72)Yes171.94(1.32,2.56)Yes182.21(1.59,2.83)Yes191.16(0.54,1.78)No201.75(1.13,2.37)YesConfidenceIntervalsfortheMeanfromaNormallyDistributedPopulationTheconfidenceintervalderivedinequation(C.18)helpsillustratehowtoconstructandinterpretconfidenceintervals.Inpractice,equation(C.18)isnotveryusefulforthemeanofanormalpopulationbecauseitassumesthatthevarianceisknowntobeunity.Itiseasytoextend(C.18)tothecasewherethestandarddeviationisknowntobeanyvalue:the95%confidenceintervalis[y¯1.96/n,y¯1.96/n].(C.20)Therefore,providedisknown,aconfidenceintervalforisreadilyconstructed.Toallowforunknown,wemustuseanestimate.Let1n1/22sn1(yiy¯)(C.21)i1denotethesamplestandarddeviation.Then,weobtainaconfidenceintervalthatdependsentirelyontheobserveddatabyreplacinginequation(C.20)withitsesti-mate,s.Unfortunately,thisdoesnotpreservethe95%levelofconfidencebecausesdependsontheparticularsample.Inotherwords,therandominterval[Y¯1.96(S/n)]nolongercontainswithprobability.95becausetheconstanthasbeenreplacedwiththerandomvariableS.Howshouldweproceed?Ratherthanusingthestandardnormaldistribution,wemustrelyonthetdistribution.ThetdistributionarisesfromthefactthatY¯~tn1,(C.22)S/n718\nxd7/14/999:21PMPage719AppendixCFundamentalsofMathematicalStatisticswhereY¯isthesampleaverage,andSisthesamplestandarddeviationoftherandomsample{Y1,…,Yn}.Wewillnotprove(C.22);acarefulproofcanbefoundinavarietyofplaces[forexample,LarsenandMarx(1988,Chapter7)].thToconstructa95%confidenceinterval,letcdenotethe97.5percentileinthetn1distribution.Inotherwords,cisthevaluesuchthat95%oftheareainthetn1isbetweencandc:P(ctn1c).95.(Thevalueofcdependsonthedegreesoffreedomn1,butwedonotmakethisexplicit.)ThechoiceofcisillustratedinFigureC.4.Oncechasbeenproperlychosen,therandominterval[YcS/n,YcS/n]containswithprobability.95.Foraparticularsample,the95%confidenceintervaliscalculatedas[y¯cs/n,y¯cs/n].(C.23)ThevaluesofcforvariousdegreesoffreedomcanbeobtainedfromTableG.2inAppendixG.Forexample,ifn20,sothatthedfisn119,thenc2.093.Thus,the95%confidenceintervalis[y¯2.093(s/20)],wherey¯andsarethevaluesobtainedfromthesample.Evenifs(whichisveryunlikely),theconfidenceinter-valin(C.23)iswiderthanthatin(C.20)becausec1.96.Forsmalldegreesoffree-dom,(C.23)ismuchwider.FigureC.4The97.5thpercentile,c,inatdistribution.Area=.95Area=.025Area=.025–c0c719\nxd7/14/999:21PMPage720AppendixCFundamentalsofMathematicalStatisticsMoregenerally,letcdenotethe100(1)percentileinthetn1distribution.Then,a100(1)%confidenceintervalisobtainedas[y¯c/2s/n,y¯c/2s/n].(C.24)Obtainingc/2requireschoosingandknowingthedegreesoffreedomn1;then,TableG.2canbeused.Forthemostpart,wewillconcentrateon95%confidenceinter-vals.Thereisasimplewaytorememberhowtoconstructaconfidenceintervalforthemeanofanormaldistribution.Recallthatsd(Y¯)/n.Thus,s/nisthepointesti-mateofsd(Y¯).Theassociatedrandomvariable,S/n,issometimescalledthestan-darderrorofY.Sincewhatshowsupinformulasisthepointestimates/n,wedefinethestandarderrorofy¯asse(y¯)s/n.Then,(C.24)canbewritteninshorthandas[y¯c/2se(y¯)].(C.25)Thisequationshowswhythenotionofthestandarderrorofanestimateplaysanimpor-tantroleineconometrics.EXAMPLEC.2(EffectofJobTrainingGrantsonWorkerProductivity)Holzer,Block,Cheatham,andKnott(1993)studiedtheeffectsofjobtraininggrantsonworkerproductivitybycollectinginformationon“scraprates”forasampleofMichiganmanufacturingfirmsreceivingjobtraininggrantsin1988.TableC.3liststhescraprates—measuredasnumberofitemsper100producedthatarenotusableandthereforeneedtobescrapped—for20firms.Eachofthesefirmsreceivedajobtraininggrantin1988;therewerenograntsawardedin1987.Weareinterestedincontructingaconfidenceintervalforthechangeinthescrapratefrom1987to1988forthepopulationofallmanufacturingfirmsthatcouldhavereceivedgrants.TableC.3ScrapRatesfor20MichiganManufacturingFirmsFirm19871988Change11037211036514.45.5.05continued720\nxd7/14/999:21PMPage721AppendixCFundamentalsofMathematicalStatisticsTableC.3(concluded)Firm19871988Change51.251.54.2961.31.5.271.06.8.26832198.18.677.51101.671.17.511.98.51.47121.5.513.45.61.16145.036.71.671584416972171819118.28.2.0819752203.973.83.14Average4.383.231.15Weassumethatthechangeinscraprateshasanormaldistribution.Sincen20,a95%confidenceintervalforthemeanchangeinscrapratesis[y2.093se(y¯)],wherese(y¯)ths/n.Thevalue2.093isthe97.5percentileinat19distribution.Fortheparticularsamplevalues,y¯1.15andse(y¯).54(eachroundedtotwodecimals),andsothe95%confi-denceintervalis[2.28,.02].Thevaluezeroisexcludedfromthisinterval,soweconcludethat,with95%confidence,theaveragechangeinscrapratesinthepopulationisnotzero.721\nxd7/14/999:21PMPage722AppendixCFundamentalsofMathematicalStatisticsAtthispoint,ExampleC.2ismostlyillustrativebecauseithassomepotentiallyseriousflawsasaneconometricanalysis.Mostimportantly,itassumesthatanysys-tematicreductioninscrapratesisduetothejobtraininggrants.Butmanythingscanhappenoverthecourseoftheyeartochangeworkerproductivity.Fromthisanalysis,wehavenowayofknowingwhetherthefallinaveragescrapratesisattributabletothejobtraininggrantsorif,atleastpartly,someexternalforceisresponsible.ASimpleRuleofThumbfora95%ConfidenceIntervalTheconfidenceintervalin(C.25)canbecomputedforanysamplesizeandanyconfi-dencelevel.AswesawinSectionB.4,thetdistributionapproachesthestandardnor-maldistributionasthedegreesoffreedomgetslarge.Inparticular,for.05,c/2*1.96asn*,althoughc/2isalwaysgreaterthan1.96foreachn.Aruleofthumbforanapproximate95%confidenceintervalis[y¯2se(y¯)].(C.26)Inotherwords,weobtainy¯anditsstandarderrorandthencomputey¯plusandminustwiceitsstandarderrortoobtaintheconfidenceinterval.Thisisslightlytoowideforverylargen,anditistoonarrowforsmalln.AswecanseefromExampleC.2,evenfornassmallas20,(C.26)isintheballparkfora95%confidenceintervalforthemeanfromanormaldistribution.Thismeanswecangetprettyclosetoa95%confidenceintervalwithouthavingtorefertottables.AsymptoticConfidenceIntervalsforNonnormalPopulationsInsomeapplications,thepopulationisclearlynonnormal.AleadingcaseistheBernoullidistribution,wheretherandomvariabletakesononlythevalueszeroandone.Inothercases,thenonnormalpopulationhasnostandarddistribution.Thisdoesnotmatter,providedthesamplesizeissufficientlylargeforthecentrallimittheoremtogiveagoodapproximationforthedistributionofthesampleaverageY¯.Forlargen,anapproximate95%confidenceintervalis[y¯1.96se(y¯)],(C.27)thwherethevalue1.96isthe97.5percentileinthestandardnormaldistribution.Mechanically,computinganapproximateconfidenceintervaldoesnotdifferfromthenormalcase.Aslightdifferenceisthatthenumbermultiplyingthestandarderrorcomesfromthestandardnormaldistribution,ratherthanthetdistribution,becauseweareusingasymptotics.Becausethetdistributionapproachesthestandardnormalasthedfincreases,equation(C.25)isalsoperfectlylegitimateasanapproximate95%interval;somepreferthisto(C.27)becausetheformerisexactfornormalpop-ulations.722\nxd7/14/999:21PMPage723AppendixCFundamentalsofMathematicalStatisticsEXAMPLEC.3(RaceDiscriminationinHiring)TheUrbanInstituteconductedastudyin1988inWashingtonD.C.toexaminetheextentofracediscriminationinhiring.Fivepairsofpeopleinterviewedforseveraljobs.Ineachpair,onepersonwasblack,andtheotherpersonwaswhite.Theyweregivenresumesindicat-ingthattheywerevirtuallythesameintermsofexperience,education,andotherfactorsthatdeterminejobqualification.Theideawastomakeindividualsassimilaraspossiblewiththeexceptionofrace.Eachpersoninapairinterviewedforthesamejob,andtheresearchersrecordedwhichapplicantreceivedajoboffer.Thisisanexampleofamatchedpairsanalysis,whereeachtrialconsistsofdataontwopeople(ortwofirms,twocities,andsoon)thatarethoughttobesimilarinmanyrespectsbutdifferentinoneimportantchar-acteristic.LetBdenotetheprobabilitythattheblackpersonisofferedajobandletWbetheprobabilitythatthewhitepersonisofferedajob.Weareprimarilyinterestedinthediffer-ence,BW.LetBidenoteaBernoullivariableequaltooneiftheblackpersongetsajobofferfromemployeri,andzerootherwise.Similarly,Wi1ifthewhitepersongetsajobofferfromemployeri,andzerootherwise.Poolingacrossthefivepairsofpeople,therewereatotalofn241trials(pairsofinterviewswithemployees).UnbiasedestimatorsofBandWareB¯andW¯,thefractionsofinterviewsforwhichblacksandwhiteswereofferedjobs,respectively.Toputthisintotheframeworkofcomputingaconfidenceintervalforapopulationmean,defineanewvariableYiBiWi.Now,Yicantakeonthreevalues:1iftheblackpersondidnotgetthejobbutthewhitepersondid,0ifbothpeopleeitherdidordidnotgetthejob,and1iftheblackpersongotthejobandthewhitepersondidnot.Then,E(Yi)E(Bi)E(Wi)BW.ThedistributionofYiiscertainlynotnormal—itisdiscreteandtakesononlythreeval-ues.Nevertheless,anapproximateconfidenceintervalforBWcanbeobtainedbyusinglargesamplemethods.Usingthe241observeddatapoints,b¯.224andw¯.357,andsoy¯.224.357.133.Thus,22.4%ofblackapplicantswereofferedjobs,while35.7%ofwhiteapplicantswereofferedjobs.Thisisprimafacieevidenceofdiscriminationagainstblacks,butwecanlearnmuchmorebycomputingaconfidenceintervalfor.Tocomputeanapproximate95%confidenceinterval,weneedthesamplestandarddeviation.Thisturnsouttobes.482[usingequation(C.21)].Using(C.27),weobtaina95%CIforBWas.1331.96(.482/241).133.031[.164,.102].Theapproximate99%CIis.1332.58(.482/241)[.213,.053].Naturally,thiscontainsawiderrangeofvaluesthanthe95%CI.Buteventhe99%CIdoesnotcontainthevaluezero.Thus,weareveryconfidentthatthepopulationdifferenceBWisnotzero.Onefinalcommentneedstobemadebeforeweleaveconfidenceintervals.Becausethestandarderrorfory¯,se(y¯)s/n,shrinkstozeroasthesamplesizegrows,weseethat—allelseequal—alargersamplesizemeansasmallerconfidenceinterval.Thus,animportantbenefitofalargesamplesizeisthatitresultsinsmallerconfidenceintervals.723\nxd7/14/999:21PMPage724AppendixCFundamentalsofMathematicalStatisticsC.6HYPOTHESISTESTINGSofar,wehavereviewedhowtoevaluatepointestimators,andwehaveseen—inthecaseofapopulationmean—howtoconstructandinterpretconfidenceintervals.Butsometimesthequestionweareinterestedinhasadefiniteyesornoanswer.Herearesomeexamples:(1)Doesajobtrainingprogrameffectivelyincreaseaverageworkerproductivity?(seeExampleC.2);(2)Areblacksdiscriminatedagainstinhiring?(seeExampleC.3);(3)Dostifferstatedrunkdrivinglawsreducethenumberofdrunkdri-vingarrests?Devisingmethodsforansweringsuchquestions,usingasampleofdata,isknownashypothesistesting.FundamentalsofHypothesisTestingToillustratetheissuesinvolvedwithhypothesistesting,consideranelectionexample.Supposetherearetwocandidatesinanelection,CandidatesAandB.CandidateAisreportedtohavereceived42%ofthepopularvote,whileCandidateBreceived58%.Thesearesupposedtorepresentthetruepercentagesinthevotingpopulation,andwetreatthemassuch.CandidateAisconvincedthatmorepeoplemusthavevotedforhim,andsohewouldliketoinvestigatewhethertheelectionwasrigged.Knowingsomethingaboutstatistics,CandidateAhiresaconsultingagencytorandomlysample100voterstorecordwhetherornoteachpersonvotedforhim.Supposethat,forthesamplecollected,53peoplevotedforCandidateA.Thissampleestimateof53%clearlyexceedsthereportedpopulationvalueof42%.ShouldCandidateAconcludethattheelectionwasindeedafraud?WhileitappearsthatthevotesforCandidateAwereundercounted,wecannotbecertain.Evenifonly42%ofthepopulationvotedforCandidateA,itispossiblethat,inasampleof100,weobserve53peoplewhodidvoteforCandidateA.Thequestionis:Howstrongisthesampleevidenceagainsttheofficiallyreportedpercentageof42%?Onewaytoproceedistosetupahypothesistest.LetdenotethetrueproportionofthepopulationvotingforCandidateA.ThehypothesisthatthereportedresultsareaccuratecanbestatedasH0:.42.(C.28)Thisisanexampleofanullhypothesis.WealwaysdenotethenullhypothesisbyH0.Inhypothesistesting,thenullhypothesisplaysarolesimilartothatofadefendentontrialinmanyjudicialsystems:justasadefendentispresumedtobeinnocentuntilprovenguilty,thenullhypothesisispresumedtobetrueuntilthedatastronglysuggestotherwise.Inthecurrentexample,CandidateAmustpresentfairlystrongevidenceagainst(C.28)inordertowinarecount.Thealternativehypothesisintheelectionexampleisthatthetrueproportionvot-ingforCandidateAintheelectionisgreaterthan.42:H1:.42.(C.29)InordertoconcludethatH0isfalseandthatH1istrue,wemusthaveevidence“beyondreasonabledoubt”againstH0.Howmanyvotesoutof100wouldbeneededbeforewe724\nxd7/14/999:21PMPage725AppendixCFundamentalsofMathematicalStatisticsfeeltheevidenceisstronglyagainstH0?Mostwouldagreethatobserving43votesoutofasampleof100isnotenoughtooverturntheoriginalelectionresults;suchanout-comeiswellwithintheexpectedsamplingvariation.Ontheotherhand,wedonotneedtoobserve100votesforCandidateAtocastdoubtonH0.Whether53outof100isenoughtorejectH0ismuchlessclear.Theanswerdependsonhowwequantify“beyondreasonabledoubt.”Inhypothesistesting,wecanmaketwokindsofmistakes.First,wecanrejectthenullhypothesiswhenitisinfacttrue.ThisiscalledaTypeIerror.Intheelectionexample,aTypeIoccursifwerejectH0whenthetrueproportionofpeoplevotingforCandidateAisinfact.42.ThesecondkindoferrorisfailingtorejectH0whenitisactuallyfalse.ThisiscalledaTypeIIerror.Intheelectionexample,aTypeIIerroroccursif.42butwefailtorejectH0.Afterwehavemadethedecisionofwhetherornottorejectthenullhypothesis,wehaveeitherdecidedcorrectlyorwehavecommittedanerror.Wewillneverknowwithcertaintywhetheranerrorwascommitted.However,wecancomputetheprobabilityofmakingeitheraTypeIoraTypeIIerror.HypothesistestingrulesareconstructedtomaketheprobabilityofcommittingaTypeIerrorfairlysmall.Generally,wedefinethesignificancelevel(orsimplythelevel)ofatestastheprobabilityofaTypeIerror;itistypicallydenotedby.Symbolically,wehaveP(RejectH0H0).(C.30)Theright-handsideisreadas:“TheprobabilityofrejectingH0giventhatH0istrue.”Classicalhypothesistestingrequiresthatweinitiallyspecifyasignificancelevelforatest.Whenwespecifyavaluefor,weareessentiallyquantifyingourtoleranceforaTypeIerror.Commonvaluesforare.10,.05,and.01.If.05,thentheresearcheriswillingtofalselyrejectH05%ofthetime,inordertodetectdeviationsfromH0.Oncewehavechosenthesignificancelevel,wewouldthenliketominimizetheprobabilityofaTypeIIerror.Alternatively,wewouldliketomaximizethepowerofatestagainstallrelevantalternatives.Thepowerofatestisjustone,minustheproba-bilityofaTypeIIerror.Mathematically,()P(RejectH0)1P(TypeII),wheredenotestheactualvalueoftheparameter.Naturally,wewouldlikethepowertoequalunitywheneverthenullhypothesisisfalse.Butthisisimpossibletoachievewhilekeepingthesignificancelevelsmall.Instead,wechooseourteststomaximizethepowerforagivensignificancelevel.TestingHypothesesAbouttheMeaninaNormalPopulationInordertotestanullhypothesisagainstanalternative,weneedtochooseateststatis-tic(orstatistic,forshort)andacriticalvalue.Thechoicesforthestatisticandcriticalvaluearebasedonconvenienceandonthedesiretomaximizepowergivenasignifi-cancelevelforthetest.Inthissubsection,wereviewhowtotesthypothesesforthemeanofanormalpopulation.725\nxd7/14/999:21PMPage726AppendixCFundamentalsofMathematicalStatisticsAteststatistic,denotedT,issomefunctionoftherandomsample.Whenwecom-putethestatisticforaparticularoutcome,weobtainanoutcomeoftheteststatistic,whichwewilldenotet.Givenateststatistic,wecandefinearejectionrulethatdetermineswhenH0isrejectedinfavorofH1.Inthistext,allrejectionrulesarebasedoncomparingthevalueofateststatistic,t,toacriticalvalue,c.Thevaluesoftthatresultinrejectionofthenullhypothesisarecollectivelyknownastherejectionregion.Inordertodeterminethecriticalvalue,wemustfirstdecideonasignificancelevelofthetest.Then,given,thecriticalvalueassociatedwithisdeterminedbythedistributionofT,assumingthatH0istrue.Wewillwritethiscriticalvalueasc,suppressingthefactthatitdependson.2TestinghypothesesaboutthemeanfromaNormal(,)populationisstraight-forward.ThenullhypothesisisstatedasH0:0,(C.31)where0isavaluethatwespecify.Inthemajorityofapplications,00,butthegen-eralcaseisnomoredifficult.Therejectionrulewechoosedependsonthenatureofthealternativehypothesis.ThethreealternativesofinterestareH1:0,(C.32)H1:0,(C.33)andH1:0.(C.34)Equation(C.32)givesaone-sidedalternative,asdoes(C.33).Whenthealternativehypothesisis(C.32),thenulliseffectivelyH0:0,sincewerejectH0onlywhen0.Thisisappropriatewhenweareinterestedinthevalueofbutonlywhenisatleastaslargeas0.Equation(C.34)isatwo-sidedalternative.Thisisacceptablewhenweareinterestedinanydeparturefromthenullhypothesis.Considerfirstthealternativein(C.32).Intuitively,weshouldrejectH0infavorofH1whenthevalueofthesampleaverage,y¯,is“sufficiently”greaterthan0.Buthowshouldwedeterminewheny¯islargeenoughforH0toberejectedatthechosensignif-icancelevel?Thisrequiresknowingtheprobabilityofrejectingthenullhypothesiswhenitistrue.Ratherthanworkingdirectlywithy¯,weuseitsstandardizedversion,whereisreplacedwiththesamplestandarddeviation,s:tn(y¯0)/s(y¯0)/se(y¯),(C.35)wherese(y¯)s/nisthestandarderrorofy¯.Giventhesampleofdata,itiseasytoobtaint.Thereasonweworkwithtisthat,underthenullhypothesis,therandomvari-ableTn(Y¯0)/S726\nxd7/14/999:21PMPage727AppendixCFundamentalsofMathematicalStatisticsFigureC.5Rejectionregionfora5%significanceleveltestagainsttheone-sidedalternative>0.Area=.95Area=.050crejectionhasatn1distribution.Now,supposewehavesettledona5%significancelevel.Then,thecriticalvaluecischosensothatP(TcH0).05;thatis,theprobabilityofaTypeIerroris5%.Oncewehavefoundc,therejectionruleistc,(C.36)wherecisthe100(1)percentileinatn1distribution;asapercent,thesignificancelevelis100%.Thisisanexampleofaone-tailedtestbecausetherejectionregionisthinonetailofthetdistribution.Fora5%significancelevel,cisthe95percentileinthetn1distribution;thisisillustratedinFigureC.5.Adifferentsignificancelevelleadstoadifferentcriticalvalue.Thestatisticinequation(C.35)isoftencalledthetstatisticfortestingH0:0.Thetstatisticmeasuresthedistancefromy¯to0relativetothestandarderrorofy¯,se(y¯).EXAMPLEC.4(EffectofEnterpriseZonesonBusinessInvestments)Inthepopulationofcitiesgrantedenterprisezonesinaparticularstate[seePapke(1994)forIndiana],letYdenotethepercentagechangeininvestmentfromtheyearbeforetothe727\nxd7/14/999:21PMPage728AppendixCFundamentalsofMathematicalStatistics2yearafteracitybecameanenterprisezone.AssumethatYhasaNormal(,)distribution.ThenullhypothesisthatenterprisezoneshavenoeffectonbusinessinvestmentisH0:0;thealternativethattheyhaveapositiveeffectisH1:0(weassumethattheydonothaveanegativeeffect).SupposethatwewishtotestH0atthe5%level.Theteststatisticinthiscaseisy¯y¯t.(C.37)s/nse(y¯)Supposethatwehaveasampleof36citieswhicharegrantedenterprisezones.Then,thecriticalvalueisc1.69(seeTableG.2),andwerejectH0infavorofH1ift1.69.Supposethatthesampleyieldsy¯8.2ands23.9.Then,t2.06,andH0isthereforerejectedatthe5%level.Thus,weconcludethat,atthe5%significancelevel,enterprisezoneshaveaneffectonaverageinvestment.The1%criticalvalueis2.44,andsoH0isnotrejectedatthe1%level.ThesamecaveatholdshereasinExampleC.2:wehavenotcontrolledforotherfactorsthatmightaffectinvestmentincitiesovertime,andsowecannotclaimthattheeffectiscausal.Therejectionruleissimilarfortheone-sidedalternative(C.32).Atestwithasig-nificancelevelof100%rejectsH0against(C.33)whenevertc;(C.38)inotherwords,wearelookingfornegativevaluesofthetstatistic—whichimpliesy¯0—thataresufficientlyfarfromzerotorejectH0.Fortwo-sidedalternatives,wemustbecarefultochoosethecriticalvaluesothatthesignificancelevelofthetestisstill.IfH1isgivenbyH1:0,thenwerejectH0ify¯isfarfrom0inabsolutevalue:ay¯muchlargerormuchsmallerthan0pro-videsevidenceagainstH0infavorofH1.A100%leveltestisobtainedfromtherejec-tionruletc,(C.39)wheretistheabsolutevalueofthetstatisticin(C.35).Thisgivesatwo-tailedtest.Wemustnowbecarefulinchoosingthecriticalvalue:cisthe100(1/2)percentileinththetn1distribution.Forexample,if.05,thenthecriticalvalueisthe97.5per-centileinthetn1distribution.ThisensuresthatH0isrejectedonly5%ofthetimewhenitistrue(seeFigureC.6).Forexample,ifn22,thenthecriticalvalueisc2.08,ththe97.5percentileinat21distribution(seeTableG.2).Theabsolutevalueofthetsta-tisticmustexceed2.08inordertorejectH0againstH1atthe5%level.Itisimportanttoknowtheproperlanguageofhypothesistesting.Sometimes,theappropriatephrase“wefailtorejectH0infavorofH1atthe5%significancelevel”isreplacedwith“weacceptH0atthe5%significancelevel.”Thelatterwordingisincor-rect.Withthesamesetofdatathereareusuallymanyhypothesesthatcannotbe728\nxd7/14/999:21PMPage729AppendixCFundamentalsofMathematicalStatisticsFigureC.6Rejectionregionfora5%significanceleveltestagainstthetwo-sidedalternativeH1:0.Area=.95Area=.025Area=.0250rejectionrejectionregion–ccregionrejected.Intheearlierelectionexample,itwouldbelogicallyinconsistenttosaythatH0:.42andH0:.43areboth“accepted,”sinceonlyoneofthesecanbetrue.Butitisentirelypossiblethatneitherofthesehypothesesisrejected.Forthisreason,wealwayssay“failtorejectH0”ratherthan“acceptH0.”AsymptoticTestsforNonnormalPopulationsIfthesamplesizeislargeenoughtoinvokethecentrallimittheorem(seeSectionC.3),themechanicsofhypothesistestingforpopulationmeansarethesamewhetherornotthepopulationdistributionisnormal.Thetheoreticaljustificationcomesfromthefactthat,underthenullhypothesis,Tn(Y¯0)/S~ªNormal(0,1).Therefore,withlargen,wecancomparethetstatisticin(C.35)withthecriticalvaluesfromastandardnormaldistribution.Sincethetn1distributionconvergestothestan-dardnormaldistributionasngetslarge,thetandstandardnormalcriticalvalueswillbeverycloseforextremelylargen.Sinceasymptotictheoryisbasedonnincreasingwithoutbound,itcannottelluswhetherthestandardnormalortcriticalvaluesarebet-ter.Formoderatevaluesofn,saybetween30and60,itistraditionaltousethetdistri-butionbecauseweknowthisiscorrectfornormalpopulations.Forn120,thechoice729\nxd7/14/999:21PMPage730AppendixCFundamentalsofMathematicalStatisticsbetweenthetandstandardnormaldistributionsislargelyirrelevantbecausethecriticalvaluesarepracticallythesame.Becausethecriticalvalueschosenusingeitherthestandardnormalortdistributionareonlyapproximatelyvalidfornonnormalpopulations,ourchosensignificancelevelsarealsoonlyapproximate;thus,fornonnormalpopulationsoursignificancelevelsarereallyasymptoticsignificancelevels.Thus,ifwechoosea5%significancelevel,butourpopulationisnonnormal,thentheactualsignificancelevelwillbelargerorsmallerthan5%(andwecannotknowwhichisthecase).Whenthesamplesizeislarge,theactualsignificancelevelwillbeverycloseto5%.Practicallyspeaking,thedistinctionisnotimportant,andsowewillnowdropthequalifier“asymptotic.”EXAMPLEC.5(RaceDiscriminationinHiring)IntheUrbanInstitutestudyofdiscriminationinhiring(seeExampleC.3),weareprimarilyinterestedintestingH0:0againstH1:0,whereBWisthedifferenceinprobabilitiesthatblacksandwhitesreceivejoboffers.RecallthatisthepopulationmeanofthevariableYBW,whereBandWarebinaryindicators.Usingthen241pairedcomparisons,weobtainedy¯.133andse(y¯).482/241.031.ThetstatisticfortestingH0:0ist.133/.0314.29.YouwillrememberfromAppendixBthatthestandardnormaldistributionis,forpracticalpurposes,indistinguishablefromthetdis-tributionwith240degreesoffreedom.Thevalue4.29issofaroutinthelefttailofthedistributionthatwerejectH0atanyreasonablesignificancelevel.Infact,the.005(one-halfofapercent)criticalvalue(fortheone-sidedtest)isabout2.58.Atvalueof4.29isverystrongevidenceagainstH0infavorofH1.Thus,weconcludethatthereisdiscriminationinhiring.ComputingandUsingp-ValuesThetraditionalrequirementofchoosingasignificancelevelaheadoftimemeansthatdifferentresearchers,usingthesamedataandsameproceduretotestthesamehypoth-esis,couldwindupwithdifferentconclusions.Reportingthesignificancelevelatwhichwearecarryingoutthetestsolvesthisproblemtosomedegree,butitdoesnotcom-pletelyremovetheproblem.Toprovidemoreinformation,wecanaskthefollowingquestion:Whatisthelargestsignificancelevelatwhichwecouldcarryoutthetestandstillfailtorejectthenullhypothesis?Thisvalueisknownasthep-valueofatest(sometimescalledtheprob-value).Comparedwithchoosingasignificancelevelaheadoftimeandobtainingacrit-icalvalue,computingap-valueissomewhatmoredifficult.Butwiththeadventofquickandinexpensivecomputing,p-valuesarenowfairlyeasytoobtain.2Asanillustration,considertheproblemoftestingH0:0inaNormal(,)population.OurteststatisticinthiscaseisTnY¯/S,andweassumethatnislargeenoughtotreatTashavingastandardnormaldistributionunderH0.SupposethattheobservedvalueofTforoursampleist1.52(notehowwehaveskippedthestepofchoosingasignificancelevel).Nowthatwehaveseenthevaluet,wecanfindthe730\nxd7/14/999:21PMPage731AppendixCFundamentalsofMathematicalStatisticslargestsignificancelevelatwhichwewouldfailtorejectH0.Thisisthesignificancelevelassociatedwithusingtasourcriticalvalue.SinceourteststatisticThasastan-dardnormaldistributionunderH0,wehavep-valueP(T1.52H0)1(1.52).065,(C.40)where()denotesthestandardnormalcdf.Inotherwords,thep-valueinthisexam-pleissimplytheareatotherightof1.52,theobservedvalueoftheteststatistic,inastandardnormaldistribution.SeeFigureC.7forillustration.Sincep-value.065,thelargestsignificancelevelatwhichwecancarryoutthistestandfailtorejectis6.5%.Ifwecarryoutthetestatalevelbelow6.5%(suchasat5%),wefailtorejectH0.Ifwecarryoutthetestatalevellargerthan6.5%(suchas10%),werejectH0.Withthep-valueathand,wecancarryoutthetestatanylevel.Thep-valueinthisexamplehasanotherusefulinterpretation:itistheprobabilitythatweobserveavalueofTaslargeas1.52whenthenullhypothesisistrue.Ifthenullhypothesisisactuallytrue,wewouldobserveavalueofTaslargeas1.52duetochanceonly6.5%ofthetime.WhetherthisissmallenoughtorejectH0dependsonourtoler-anceforaTypeIerror.Thep-valuehasasimilarinterpretationinallothercases,aswewillsee.FigureC.7Thep-valuewhent=1.52fortheone-sidedalternative0.area=.065=p-value01.52731\nxd7/14/999:21PMPage732AppendixCFundamentalsofMathematicalStatisticsGenerally,smallp-valuesareevidenceagainstH0,sincetheyindicatethattheoutcomeofthedataoccurswithsmallprobabilityifH0istrue.Inthepreviousexam-ple,ifthadbeenalargervalue,sayt2.85,thenthep-valuewouldbe1(2.85).002.Thismeansthat,ifthenullhypothesisweretrue,wewouldobserveavalueofTaslargeas2.85withprobability.002.Howdoweinterpretthis?Eitherweobtainedaveryunusualsampleorthenullhypothesisisfalse.UnlesswehaveaverysmalltoleranceforTypeIerror,wewouldrejectthenullhypothesis.Ontheotherhand,alargep-valueisweakevidenceagainstH0.Ifwehadgottent.47inthepreviousexample,thenp-value1(.47).32.ObservingavalueofTlargerthan.47happenswithprobability.32,evenwhenH0istrue;thisislargeenoughsothatthereisinsufficientdoubtaboutH0,unlesswehaveaveryhightoler-anceforTypeIerror.Forhypothesistestingaboutapopulationmeanusingthetdistribution,weneeddetailedtablesinordertocomputep-values.TableG.2onlyallowsustoputboundsonp-values.Fortunately,manystatisticsandeconometricspackagesnowcomputep-valuesroutinely,andtheyalsoprovidecalculationofcdfsforthetandotherdistributionsusedforcomputingp-values.EXAMPLEC.6(EffectofJobTrainingGrantsonWorkerProductivity)ConsideragaintheHolzeretal.(1993)datainExampleC.2.Fromapolicyperspective,therearetwoquestionsofinterest.First,whatisourbestestimateofthemeanchangeinscraprates,?Wehavealreadyobtainedthisforthesampleof20firmslistedinTableC.3:thesampleaverageofthechangeinscrapratesis1.15.Relativetotheinitialaveragescrapratein1987,thisrepresentsafallinthescraprateofabout26.3%(1.15/4.38.263),whichisanontrivialeffect.Wewouldalsoliketoknowwhetherthesampleprovidesstrongevidenceforaneffectinthepopulationofmanufacturingfirmsthatcouldhavereceivedgrants.Thenullhypoth-esisisH0:0,andwetestthisagainstH1:0,whereistheaveragechangeinscraprates.Underthenull,thejobtraininggrantshavenoeffectonaveragescraprates.Thealternativestatesthatthereisaneffect.Wedonotcareaboutthealternative0;thenullhypothesisiseffectivelyH0:0.Sincey¯1.15andse(y¯).54,t1.15/.542.13.Thisisbelowthe5%crit-icalvalueof1.73(fromat19distribution)butabovethe1%criticalvalue,2.54.Thep-valueinthiscaseiscomputedasp-valueP(T192.13),(C.41)whereT19representsatdistributedrandomvariablewith19degreesoffreedom.Theinequalityisreversedfrom(C.40)becausethealternativehastheform(C.33),not(C.32).Theprobabilityin(C.41)istheareatotheleftof2.13inat19distribution(seeFig-ureC.8).732\nxd7/14/999:21PMPage733AppendixCFundamentalsofMathematicalStatisticsFigureC.8Thep-valuewhent2.13with19degreesoffreedomfortheone-sidedalternative0.area=p-value=.023–2.130UsingTableG.2,themostwecansayisthatthep-valueisbetween.025and.01,butthitiscloserto.025(sincethe97.5percentileisabout2.09).Usingastatisticalpackage,suchasStata,wecancomputetheexactp-value.Itturnsouttobeabout.023,whichisreasonableevidenceagainstH0.Thisiscertainlyenoughevidencetorejectthenullhypoth-esisthatthetraininggrantshadnoeffectatthe2.5%significancelevel(andthereforeatthe5%level).Computingap-valueforatwo-sidedtestissimilar,butwemustaccountforthetwo-sidednatureoftherejectionrule.Forttestingaboutpopulationmeans,thep-valueiscomputedasP(Tn1t)2P(Tn1t),(C.42)wheretisthevalueoftheteststatistic,andTn1isatrandomvariable.(Forlargen,replaceTn1withastandardnormalrandomvariable.)Thus,tocomputetheabsolutevalueofthetstatistic,findtheareatotherightofthisvalueinatn1distributionandmultiplytheareabytwo.733\nxd7/14/999:21PMPage734AppendixCFundamentalsofMathematicalStatisticsFornonnormalpopulations,theexactp-valuecanbedifficulttoobtain.Nevertheless,wecanfindasymptoticp-valuesbyusingthesamecalculations.Thesep-valuesarevalidforlargesamplesizes.Fornlargerthan,say,120,wemightaswellusethestandardnormaldistribution.TableG.1isdetailedenoughtogetaccuratep-values,butwecanalsouseastatisticsoreconometricsprogram.EXAMPLEC.7(RaceDiscriminationinHiring)UsingthematchedpairdatafromtheUrbanInstitute(n241),weobtainedt4.29.IfZisastandardnormalrandomvariable,P(Z4.29)is,forpracticalpurposes,zero.Inotherwords,the(asymptotic)p-valueforthisexampleisessentiallyzero.ThisisverystrongevidenceagainstH0.SUMMARYOFHOWTOUSEp-VALUES(i)ChooseateststatisticTanddecideonthenatureofthealternative.Thisdeter-mineswhethertherejectionruleistc,tc,ortc.(ii)Usetheobservedvalueofthetstatisticasthecriticalvalueandcomputethecor-respondingsignificancelevelofthetest.Thisisthep-value.Iftherejectionruleisoftheformtc,thenp-valueP(Tt).Iftherejectionruleistc,thenp-valueP(Tt);iftherejectionruleistc,thenp-valueP(Tt).(iii)Ifasignificancelevelhasbeenchosen,thenwerejectH0atthe100%levelifp-value.Ifp-value,thenwefailtorejectH0atthe100%level.Thus,itisasmallp-valuethatleadstorejection.TheRelationshipBetweenConfidenceIntervalsandHypothesisTestingSincecontructingconfidenceintervalsandhypothesistestsbothinvolveprobabilitystatements,itisnaturaltothinkthattheyaresomehowlinked.Itturnsoutthattheyare.Afteraconfidenceintervalhasbeenconstructed,wecancarryoutavarietyofhypoth-esistests.Theconfidenceintervalswehavediscussedarealltwo-sidedbynature.(Inthistext,wewillhavenoneedtoconstructone-sidedconfidenceintervals.)Thus,confidenceintervalscanbeusedtotestagainsttwo-sidedalternatives.Inthecaseofapopulationmean,thenullisgivenby(C.31),andthealternativeis(C.34).Supposewehavecon-structeda95%confidenceintervalfor.Then,ifthehypothesizedvalueofunderH0,0,isnotintheconfidenceinterval,thenH0:0isrejectedagainstH1:0atthe5%level.If0liesinthisinterval,thenwefailtorejectH0atthe5%level.Noticehowanyvaluefor0canbetestedonceaconfidenceintervalisconstructed,andsinceaconfidenceintervalcontainsmorethanonevalue,therearemanynullhypothe-sesthatwillnotberejected.734\nxd7/14/999:21PMPage735AppendixCFundamentalsofMathematicalStatisticsEXAMPLEC.8(TrainingGrantsandWorkerProductivity)IntheHolzeretal.example,weconstructeda95%confidenceintervalforthemeanchangeinscraprateas[2.28,.02].Sincezeroisexcludedfromthisinterval,werejectH0:0againstH1:0atthe5%level.This95%confidenceintervalalsomeansthatwefailtorejectH0:2atthe5%level.Infact,thereisacontinuumofnullhypothe-sesthatarenotrejectedgiventhisconfidenceinterval.PracticalVersusStatisticalSignificanceIntheexamplescoveredsofar,wehaveproducedthreekindsofevidenceconcerningpopulationparameters:pointestimates,confidenceintervals,andhypothesistests.Thesetoolsforlearningaboutpopulationparametersareequallyimportant.Thereisanunderstandabletendencyforstudentstofocusonconfidenceintervalsandhypothesistestsbecausethesearethingstowhichwecanattachconfidenceorsignificancelevels.Butinanystudy,wemustalsointerpretthemagnitudesofpointestimates.Statisticalsignificancedependsonthesizeofthetstatisticandnotjustonthesizeofy¯.FortestingH0:0,ty¯/se(y¯).Thus,statisticalsignificancedependsontheratioofy¯toitsstandarderror.Atstatisticcanbelargeeitherbecausey¯islargeorbecausese(y¯)issmall.EXAMPLEC.9(EffectofFreewayWidthonCommuteTime)LetYdenotethechangeincommutetime,measuredinminutes,forcommutersinamet-ropolitanareafrombeforeafreewaywaswidenedtoafterthefreewaywaswidened.2AssumethatY~Normal(,).Thenullhypothesisthatthewideningdidnotreduceaver-agecommutetimeisH0:0;thealternativethatitreducedaveragecommutetimeisH1:0.Supposearandomsampleofcommutersofsizen300isobtainedtodeter-minetheeffectivenessofthefreewayproject.Theaveragechangeincommutetimeiscom-putedtobey¯3.6,andthesamplestandarddeviationiss18.7;thus,se(y¯)18.7/3001.08.Thetstatisticist3.6/1.083.33,whichisverystatisticallysig-nificant;thep-valueisessentiallyzero.Thus,weconcludethatthefreewaywideninghadastatisticallysignificanteffectonaveragecommutetime.Iftheoutcomeofthehypothesistestisallthatwerereportedfromthestudy,itwouldbemisleading.Reportingonlystatisticalsignificancemasksthefactthattheestimatedreductioninaveragecommutetime,3.6minutes,isprettymeager.Tobeupfront,weshouldreportthepointestimateof3.6,alongwiththesignificancetest.Whilethemagnitudeandsignofthetstatisticdeterminestatisticalsignificance,thepointestimatey¯determineswhatwemightcallpracticalsignificance.Anestimatecanbestatisticallysignificantwithoutbeingespeciallylarge.Weshouldalwaysdiscussthe735\nxd7/14/999:21PMPage736AppendixCFundamentalsofMathematicalStatisticspracticalsignificancealongwiththestatisticalsignificanceofpointestimates;thisthemewillariseofteninthetext.Findingpointestimatesthatarestatisticallysignificantwithoutbeingpracticallysignificantoftenoccurswhenweareworkingwithlargesamples.Todiscusswhythishappens,itisusefultohavethefollowingdefinition.TESTCONSISTENCYAconsistenttestrejectsH0withprobabilityapproachingoneasthesamplesizegrows,wheneverH1istrue.Anotherwaytosaythatatestisconsistentisthat,asthesamplesizetendstoinfin-ity,thepowerofthetestgetscloserandclosertounity,wheneverH1istrue.Allofthetestswecoverinthistexthavethisproperty.Inthecaseoftestinghypothesesaboutapopulationmean,testconsistencyfollowsbecausethevarianceofY¯convergestozeroasthesamplesizegetslarge.ThetstatisticfortestingH0:0isTY¯/(S/n).Sinceplim(Y¯)andplim(S),itfollowsthatif,say,0,thenTgetslargerandlarger(withhighprobability)asn*.Inotherwords,nomatterhowcloseistozero,wecanbealmostcertaintorejectH0:0,givenalargeenoughsamplesize.Thissaysnothingaboutwhetherislargeinapracticalsense.C.7REMARKSONNOTATIONInourreviewofprobabilityandstatisticshereandinAppendixB,wehavebeencare-fultousestandardconventionstodenoterandomvariables,estimators,andteststatis-tics.Forexample,wehaveusedWtoindicateanestimator(randomvariable)andwtodenoteaparticularestimate(outcomeoftherandomvariableW).Distinguishingbetweenanestimatorandanestimateisimportantforunderstandingvariousconceptsinestimationandhypothesistesting.However,makingthisdistinctionquicklybecomesaburdenineconometricanalysisbecausethemodelsaremorecomplicated:manyran-domvariablesandparameterswillbeinvolved,andbeingtruetotheusualconventionsfromprobabilityandstatisticsrequiresmanyextrasymbols.Inthemaintext,weuseasimplerconventionthatiswidelyusedineconometrics.Ifisapopulationparameter,thenotationˆ(“thetahat”)willbeusedtodenotebothanestimatorandanestimateof.Thisnotationisusefulinthatitprovidesasimplewayofattachinganestimatortothepopulationparameteritissupposedtobeestimat-ing.Thus,ifthepopulationparameteris,thenˆdenotesanestimatororestimateof222;iftheparameteris,ˆisanestimatororestimateof;andsoon.Sometimes,wewilldiscusstwoestimatorsofthesameparameter,inwhichcase,wewillneedadif-ferentnotation,suchas˜(“thetatilda”).Whiledroppingtheconventionsfromprobabilityandstatisticstoindicateestima-tors,randomvariables,andteststatisticsputsadditionalresponsibilityonyou,itisnotabigdeal,oncethedifferencebetweenanestimatorandanestimateisunderstood.Ifwearediscussingstatisticalpropertiesofˆ—suchasderivingwhetherornotitisunbi-asedorconsistent—thenwearenecessarilyviewingˆasanestimator.Ontheotherhand,ifwewritesomethinglikeˆ1.73,thenweareclearlydenotingapointestimate736\nxd7/14/999:21PMPage737AppendixCFundamentalsofMathematicalStatisticsfromagivensampleofdata.Theconfusionthatcanarisebyusingˆtodenotebothshouldbeminimal,onceyouhaveagoodunderstandingofprobabilityandstatistics.SUMMARYWehavediscussedtopicsfrommathematicalstatisticsthatareheavilyreliedonineconometricanalysis.Thenotionofanestimator,whichissimplyaruleforcombiningdatatoestimateapopulationparameter,isfundamental.Wehavecoveredvariousprop-ertiesofestimators.Themostimportantsmallsamplepropertiesareunbiasednessandefficiency,thelatterofwhichdependsoncomparingvarianceswhenestimatorsareunbiased.Largesamplepropertiesconcernthesequenceofestimatorsobtainedasthesamplesizegrows,andtheyarealsoheavilyreliedonineconometrics.Anyusefulesti-matorisconsistent.Thecentrallimittheoremimpliesthat,inlargesamples,thesam-plingdistributionofmostestimatorsisapproximatelynormal.Thesamplingdistributionofanestimatorcanbeusedtoconstructconfidenceinter-vals.Wesawthisforestimatingthemeanfromanormaldistributionandforcomput-ingapproximateconfidenceintervalsinnonnormalcases.Classicalhypothesistesting,whichrequiresspecifyinganullhypothesis,analternativehypothesis,andasignifi-cancelevel,iscarriedoutbycomparingateststatistictoacriticalvalue.Alternatively,ap-valuecanbecomputedthatallowsustocarryoutatestatanysignificancelevel.KEYTERMSAlternativeHypothesisPowerofaTestAsymptoticNormalityPracticalSignificanceBiasProbabilityLimitCentralLimitTheorem(CLT)p-ValueConfidenceIntervalRandomSampleConsistentEstimatorRejectionRegionConsistentTestSampleAverageCriticalValueSampleCorrelationCoefficientEstimateSampleCovarianceEstimatorSampleStandardDeviationHypothesisTestSampleVarianceInconsistentSamplingDistributionIntervalEstimatorSamplingVarianceLawofLargeNumbers(LLN)SignificanceLevelLeastSquaresEstimatorStandardErrorMaximumLikelihoodEstimatortStatisticMeanSquaredError(MSE)TestStatisticMethodofMomentsTwo-SidedAlternativeMinimumVarianceUnbiasedEstimatorTwo-TailedTestNullHypothesisTypeIErrorOne-SidedAlternativeTypeIIErrorOne-TailedTestUnbiasednessPopulation737\nxd7/14/999:21PMPage738AppendixCFundamentalsofMathematicalStatisticsPROBLEMSC.1LetY1,Y2,Y3,andY4beindependent,identicallydistributedrandomvariables12fromapopulationwithmeanandvariance.LetY¯(Y1Y2Y3Y4)denote4theaverageofthesefourrandomvariables.2(i)WhataretheexpectedvalueandvarianceofY¯intermsofand?(ii)Now,consideradifferentestimatorof:1111WY1Y2Y3Y4.8842ThisisanexampleofaweightedaverageoftheYi.ShowthatWisalsoanunbiasedestimatorof.FindthevarianceofW.(iii)Basedonyouranswerstoparts(i)and(ii),whichestimatorofdoyouprefer,Y¯orW?(iv)Now,consideramoregeneralestimatorof,definedbyWaa1Y1a2Y2a3Y3a4Y4,wheretheaiareconstants.WhatconditionisneededontheaiforWatobeanunbiasedestimatorof?(v)ComputethevarianceoftheestimatorWafrompart(iv).C.2ThisisamoregeneralversionofProblemC.1.LetY1,Y2,…,Ynbenpairwise2uncorrelatedrandomvariableswithcommonmeanandcommonvariance.LetY¯denotethesampleaverage.(i)DefinetheclassoflinearestimatorsofbyWaa1Y1a2Y2…anYn,wheretheaiareconstants.WhatrestrictionontheaiisneededforWatobeanunbiasedestimatorof?(ii)FindVar(Wa).(iii)Foranynumbersa1,a2,…,an,thefollowinginequalityholds:(a12222a2…an)/na1a2…an.Usethis,alongwithparts(i)and(ii),toshowthatVar(Wa)Var(Y¯)wheneverWaisunbiased,sothatY¯isthebestlinearunbiasedestimator.[Hint:Whatdoestheinequalitybecomewhentheaisatisfytherestrictionfrompart(i)?]C.3LetYdenotethesampleaveragefromarandomsamplewithmeanandvariance2.Considertwoalternativeestimatorsof:W1[(n1)/n]Y¯andW2Y¯/2.(i)ShowthatW1andW2arebothbiasedestimatorsofandfindthebiases.Whathappenstothebiasesasn*?Commentonanyimpor-tantdifferencesinbiasforthetwoestimatorsasthesamplesizegetslarge.(ii)FindtheprobabilitylimitsofW1andW2.{Hint:UsepropertiesPLIM.1andPLIM.2;forW1,notethatplim[(n1)/n]1.}Whichestimatorisconsistent?(iii)FindVar(W1)andVar(W2).738\nxd7/14/999:21PMPage739AppendixCFundamentalsofMathematicalStatistics(iv)ArguethatW1isabetterestimatorthanY¯ifis“close”tozero.(Considerbothbiasandvariance.)C.4ForpositiverandomvariablesXandY,supposetheexpectedvalueofYgivenXisE(YX)X.TheunknownparametershowshowtheexpectedvalueofYchangeswithX.(i)DefinetherandomvariableZY/X.ShowthatE(Z).[Hint:UsePropertyCE.2alongwiththelawofiteratedexpectations,PropertyCE.4.Inparticular,firstshowthatE(ZX)andthenuseCE.4.]n1(ii)Usepart(i)toprovethattheestimatorWn(Yi/Xi)isunbiasedi1forW,where{(Xi,Yi):i1,2,…,n}isarandomsample.(iii)ThefollowingtablecontainsdataoncornyieldsforseveralcountiesinIowa.TheUSDApredictsthenumberofhectaresofcornineachcountybasedonsatellitephotos.Researcherscountthenumberof“pixels”ofcorninthesatellitepicture(asopposedto,forexample,thenumberofpixelsofsoybeansorofuncultivatedland)andusethesetopredicttheactualnumberofhectares.Todevelopapredictionequationtobeusedforcountiesingeneral,theUSDAsurveyedfarmersinselectedcoun-tiestoobtaincornyieldsinhectares.LetYicornyieldincountyiandletXinumberofcornpixelsinthesatellitepictureforcountyi.Therearen17observationsforeightcounties.Usethissampletocomputetheestimateofdevisedinpart(ii).PlotCornYieldCornPixels1165.76374296.32209376.082534185.354325116.433676162.083617152.042888161.75369992.88206continued739\nxd7/14/999:21PMPage740AppendixCFundamentalsofMathematicalStatisticsPlotCornYieldCornPixels10149.943161164.7514512127.0735513133.552951477.7022315206.3945916108.3329017118.17307C.5LetYdenoteaBernoulli()randomvariablewith01.Supposeweareinterestedinestimatingtheoddsratio,/(1),whichistheprobabilityofsuc-cessovertheprobabilityoffailure.Givenarandomsample{Y1,…,Yn},weknowthatanunbiasedandconsistentestimatorofisY¯,theproportionofsuccessesinntrials.AnaturalestimatorofisG{Y¯/(1Y¯)},theproportionofsuccessesoverthepro-portionoffailuresinthesample.(i)WhyisGnotanunbiasedestimatorof?(ii)UsePLIM.2(iii)toshowthatGisaconsistentestimatorof.C.6Youarehiredbythegovernortostudywhetherataxonliquorhasdecreasedaver-ageliquorconsumptioninyourstate.Youareabletoobtain,forasampleofindividu-alsselectedatrandom,thedifferenceinliquorconsumption(inounces)fortheyearsbeforeandafterthetax.Forpersoniwhoissampledrandomlyfromthepopulation,Yidenotesthechangeinliquorconsumption.Treattheseasarandomsamplefroma2Normal(,)distribution.(i)Thenullhypothesisisthattherewasnochangeinaverageliquorcon-sumption.Statethisformallyintermsof.(ii)Thealternativeisthattherewasadeclineinliquorconsumption;statethealternativeintermsof.(iii)Now,supposeyoursamplesizeisn900andyouobtaintheestimatesy¯32.8ands466.4.CalculatethetstatisticfortestingH0againstH1;obtainthep-valueforthetest.(Becauseofthelargesamplesize,justusethestandardnormaldistributiontabulatedinTableG.1.)DoyourejectH0atthe5%level?atthe1%level?(iv)Wouldyousaythattheestimatedfallinconsumptionislargeinmag-nitude?Commentonthepracticalversusstatisticalsignificanceofthisestimate.740\nxd7/14/999:21PMPage741AppendixCFundamentalsofMathematicalStatistics(v)Whathasbeenimplicitlyassumedinyouranalysisaboutotherdeter-minantsofliquorconsumptionoverthetwo-yearperiodinordertoinfercausalityfromthetaxchangetoliquorconsumption?C.7Thenewmanagementatabakeryclaimsthatworkersarenowmoreproductivethantheywereunderoldmanagement,whichiswhywageshave“generallyincreased.”baLetWibeWorkeri’swageundertheoldmanagementandletWibeWorkeri’swageabafterthechange.ThedifferenceisDiWiWi.AssumethattheDiarearandom2samplefromaNormal(,)distribution.(i)Usingthefollowingdataon15workers,constructanexact95%confi-denceintervalfor.(ii)Formallystatethenullhypothesisthattherehasbeennochangeinaver-agewages.Inparticular,whatisE(Di)underH0?Ifyouarehiredtoexaminethevalidityofthenewmanagement’sclaim,whatistherele-vantalternativehypothesisintermsofE(Di)?(iii)Testthenullhypothesisfrompart(ii)againstthestatedalternativeatthe5%and1%levels.(iv)Obtainthep-valueforthetestinpart(iii).WorkerWageBeforeWageAfter18.309.2529.409.0039.009.25410.5010.00511.4012.0068.759.50710.0010.2589.509.50910.8011.501012.5513.101112.0011.50128.659.00continued741\nxd7/14/999:21PMPage742AppendixCFundamentalsofMathematicalStatisticsWorkerWageBeforeWageAfter137.757.751411.2511.501512.6513.00C.8TheNewYorkTimes(2/5/90)reportedthree-pointshootingperformanceforthetoptenthree-pointshootersintheNBA.Thefollowingtablesummarizesthesedata:PlayerFGA-FGMMarkPrice429-188TrentTucker833-345DaleEllis1,149-472CraigHodges1,016-396DannyAinge1,051-406ByronScott676-260ReggieMiller416-159LarryBird1,206-455JonSundvold440-166BrianTaylor417-157Note:FGAfieldgoalsattemptedandFGMfieldgoalsmade.Foragivenplayer,theoutcomeofaparticularshotcanbemodeledasaBernoulli(zero-one)variable:ifYiistheoutcomeofshoti,thenYi1iftheshotismade,andYi0iftheshotismissed.Letdenotetheprobabilityofmakinganyparticularthree-pointshotattempt.ThenaturalestimatorofisY¯FGM/FGA.(i)EstimateforMarkPrice.(ii)FindthestandarddeviationoftheestimatorY¯intermsofandthenumberofshotattempts,n.742\nxd7/14/999:21PMPage743AppendixCFundamentalsofMathematicalStatistics(iii)Theasymptoticdistributionof(Y¯)/se(Y¯)isstandardnormal,wherese(Y¯)Y¯(1Y¯)/n.UsethisfacttotestH0:.5againstH1:.5forMarkPrice.Usea1%significancelevel.C.9Supposethatamilitarydictatorinanunnamedcountryholdsaplebiscite(ayes/novoteofconfidence)andclaimsthathewassupportedby65%ofthevoters.Ahumanrightsgroupsuspectsfoulplayandhiresyoutotestthevalidityofthedictator’sclaim.Youhaveabudgetthatallowsyoutorandomlysample200votersfromthecountry.(i)LetXbethenumberofyesvotesobtainedfromarandomsampleof200outoftheentirevotingpopulation.WhatistheexpectedvalueofXif,infact,65%ofallvoterssupportedthedictator?(ii)WhatisthestandarddeviationofX,againassumingthatthetruefrac-tionvotingyesintheplebisciteis.65?(iii)Now,youcollectyoursampleof200,andyoufindthat115peopleactu-allyvotedyes.UsetheCLTtoapproximatetheprobabilitythatyouwouldfind115orfeweryesvotesfromarandomsampleof200if,infact,65%oftheentirepopulationvotedyes.(iv)Howwouldyouexplaintherelevanceofthenumberinpart(iii)tosomeonewhodoesnothavingtraininginstatistics?C.10Beforeastrikeprematurelyendedthe1994majorleaguebaseballseason,TonyGwynnoftheSanDiegoPadreshad165hitsin419atbats,fora.394battingaverage.TherewasdiscussionaboutwhetherGwynnwasapotential.400hitterthatyear.ThisissuecanbecouchedintermsofGwynn’sprobabilityofgettingahitonaparticularatbat,callit.LetYibetheBernoulli()indicatorequaltounityifGwynngetsahitdur-thinghisiatbat,andzerootherwise.Then,Y1,Y2,…,YnisarandomsamplefromaBernoulli()distribution,whereistheprobabilityofsuccess,andn419.OurbestpointestimateofisGwynn’sbattingaverage,whichisjustthepropor-tionofsuccesses:y¯.394.Usingthefactthatse(y¯)y¯(1y¯)/n,constructanapproximate95%confidenceintervalfor,usingthestandardnormaldistribution.WouldyousaythereisstrongevidenceagainstGwynn’sbeingapotential.400hitter?Explain.743\n\nxd7/14/999:28PMPage744AppendixDSummaryofMatrixAlgebrahisappendixsummarizesthematrixalgebraconcepts,includingthealgebraofTprobability,neededforthestudyofmultiplelinearregressionmodelsusingmatricesinAppendixE.Noneofthismaterialisusedinthemaintext.D.1BASICDEFINITIONSDEFINITIOND.1(Matrix)Amatrixisarectangulararrayofnumbers.Moreprecisely,anmnmatrixhasmrowsandncolumns.Thepositiveintegermiscalledtherowdimension,andniscalledthecolumndimension.Weuseuppercaseboldfaceletterstodenotematrices.Wecanwriteanmnmatrixgenericallyasa11a12a13...a1na21a22a23...a2n.A[aij]..am1am2am3...amnththwhereaijrepresentstheelementintheirowandthejcolumn.Forexample,a25standsforthenumberinthesecondrowandthefifthcolumnofA.Aspecificexampleofa23matrixis217A450(D.1)wherea137.TheshorthandA[aij]isoftenusedtodefinematrixoperations.DEFINITIOND.2(SquareMatrix)Asquarematrixhasthesamenumberofrowsandcolumns.Thedimensionofasquarematrixisitsnumberofrowsandcolumns.744\nxd7/14/999:28PMPage745AppendixDSummaryofMatrixAlgebraDEFINITIOND.3(Vectors)(i)A1mmatrixiscalledarowvector(ofdimensionm)andcanbewrittenasx(x1,x2,…,xm).(ii)Ann1matrixiscalledacolumnvectorandcanbewrittenasy1y2.y...ynDEFINITIOND.4(DiagonalMatrix)AsquarematrixAisadiagonalmatrixwhenallofitsdiagonalelementsarezero,thatis,aij0forallij.Wecanalwayswriteadiagonalmatrixasa1100...00a220...0.A...000...annDEFINITIOND.5(IdentityandZeroMatrices)(i)Thennidentitymatrix,denotedI,orsometimesIntoemphasizeitsdimension,isthediagonalmatrixwithunity(one)ineachdiagonalposition,andzeroelsewhere:100...0010...0..IIn.....000...1(ii)Themnzeromatrix,denoted0,isthemnmatrixwithzeroforallentries.Thisneednotbeasquarematrix.D.2MATRIXOPERATIONSMatrixAdditionTwomatricesAandB,eachhavingdimensionmn,canbeaddedelementbyele-ment:AB[aijbij].Moreprecisely,a11b11a12b12...a1nb1na21b21a22b22...a2nb2n.AB...am1bm1am2bm2...amnbmn745\nxd7/14/999:28PMPage746AppendixDSummaryofMatrixAlgebraForexample,217104313450423073.Matricesofdifferentdimensionscannotbeadded.ScalarMultiplicationGivenanyrealnumber(oftencalledascalar),scalarmultiplicationisdefinedasA[aij],ora11a12...a1na21a22...a2n.A...am1am2...amnForexample,if2andAisthematrixinequation(D.1),then4214A8100.MatrixMultiplicationTomultiplymatrixAbymatrixBtoformtheproductAB,thecolumndimensionofAmustequaltherowdimensionofB.Therefore,letAbeanmnmatrixandletBbeannpmatrix.ThenmatrixmultiplicationisdefinedasnABaikbkj.k1thInotherwords,the(i,j)elementofthenewmatrixABisobtainedbymultiplyingeachththelementintheirowofAbythecorrespondingelementinthejcolumnofBandaddingthesenproductstogether.Aschematicmayhelpmakethisprocessmoretrans-parent:ABABb1jb2jnthirow*ai1ai2ai3...ainb3jaikbkj,k1...+‹bnj‹‹+‹‹‹ththjcolumn(i,j)element746\nxd7/14/999:28PMPage747AppendixDSummaryofMatrixAlgebrawhere,bythedefinitionofthesummationoperatorinAppendixA,naikbkjai1b1jai2b2j…ainbnj.k1Forexample,016021010121410120112241.3000Wecanalsomultiplyamatrixandavector.IfAisannmmatrixandyisanm1vector,thenAyisann1vector.Ifxisa1nvector,thenxAisa1mvector.Matrixaddition,scalarmultiplication,andmatrixmultiplicationcanbecombinedinvariousways,andtheseoperationssatisfyseveralrulesthatarefamiliarfrombasicoperationsonnumbers.Inthefollowinglistofproperties,A,B,andCarematriceswithappropriatedimensionsforapplyingeachoperation,andandarerealnumbers.Mostofthesepropertiesareeasytoillustratefromthedefinitions.PROPERTIESOFMATRIXMULTIPLICATION:(1)()AAA;(2)(AB)AB;(3)()A(A);(4)(AB)(A)B;(5)ABBA;(6)(AB)CA(BC);(7)(AB)CA(BC);(8)A(BC)ABAC;(9)(AB)CACBC;(10)IAAIA;(11)A00AA;(12)AA0;(13)A00A0;(14)ABBA,evenwhenbothproductsaredefined.Thelastpropertydeservesfurthercomment.IfAisnmandBismp,thenABisdefined,butBAisdefinedonlyifnp(therowdimensionofAequalsthecolumndimensionofB).IfAismnandBisnm,thenABandBAarebothdefined,buttheyarenotusuallythesame;infact,theyhavedifferentdimensions,unlessAandBarebothsquarematrices.EvenwhenAandBarebothsquare,ABBA,exceptunderspecialcircumstances.TransposeDEFINITIOND.6(Transpose)LetA[aij]beanmnmatrix.ThetransposeofA,denotedA(calledAprime),isthenmmatrixobtainedbyinterchangingtherowsandcolumnsofA.WecanwritethisasA[aji].Forexample,24217A,A15.45070PROPERTIESOFTRANSPOSE:(1)(A)A;(2)(A)Aforanyscalar;(3)n2(AB)A+B;(4)(AB)BA,whereAismnandBisnk;(5)xxxi,i1747\nxd7/14/999:28PMPage748AppendixDSummaryofMatrixAlgebrawherexisann1vector;(6)IfAisannkmatrixwithrowsgivenbythe1kvectorsa1,a2,…,an,sothatwecanwritea1a2.A.,.anthenA(a1a2...an).DEFINITIOND.7(SymmetricMatrix)AsquarematrixAisasymmetricmatrixifandonlyifAA.IfXisanynkmatrix,thenXXisalwaysdefinedandisasymmetricmatrix,ascanbeseenbyapplyingthefirstandfourthtransposeproperties(seeProblemD.3).PartitionedMatrixMultiplicationLetAbeannkmatrixwithrowsgivenbythe1kvectorsa1,a2,…,an,andletBbeannmmatrixwithrowsgivenby1mvectorsb1,b2,…,bn:a1b1a2b2..A.,B....anbnThen,nABaibi,i1whereforeachi,aibiisakmmatrix.Therefore,ABcanbewrittenasthesumofnmatrices,eachofwhichiskm.Asaspecialcase,wehavenAAaiai,i1whereaiaiisakkmatrixforalli.TraceThetraceofamatrixisaverysimpleoperationdefinedonlyforsquarematrices.DEFINITIOND.8(Trace)ForanynnmatrixA,thetraceofamatrixA,denotedtr(A),isthesumofitsdiag-onalelements.Mathematically,748\nxd7/14/999:28PMPage749AppendixDSummaryofMatrixAlgebrantr(A)aii.i1PROPERTIESOFTRACE:(1)tr(In)n;(2)tr(A)tr(A);(3)tr(AB)tr(A)tr(B);(4)tr(A)tr(A),foranyscalar;(5)tr(AB)tr(BA),whereAismnandBisnm.InverseThenotionofamatrixinverseisveryimportantforsquarematrices.DEFINITIOND.9(Inverse)111AnnnmatrixAhasaninverse,denotedA,providedthatAAInandAAIn.Inthiscase,Aissaidtobeinvertibleornonsingular.Otherwise,itissaidtobenon-invertibleorsingular.1PROPERTIESOFINVERSE:(1)Ifaninverseexists,itisunique;(2)(A)1111(1/)A,if0andAisinvertible;(3)(AB)BA,ifAandBareboth11nnandinvertible;(4)(A)(A).Wewillnotbeconcernedwiththemechanicsofcalculatingtheinverseofamatrix.Anymatrixalgebratextcontainsdetailedexamplesofsuchcalculations.D.3LINEARINDEPENDENCE.RANKOFAMATRIXForasetofvectorshavingthesamedimension,itisimportanttoknowwhetheronevectorcanbeexpressedasalinearcombinationoftheremainingvectors.DEFINITIOND.10(LinearIndependence)Let{x1,x2,…,xr}beasetofn1vectors.Thesearelinearlyindependentvectorsifandonlyif1x12x2…rxr0(D.2)impliesthat12…r0.If(D.2)holdsforasetofscalarsthatarenotallzero,then{x1,x2,…,xr}islinearlydependent.Thestatementthat{x1,x2,…,xr}islinearlydependentisequivalenttosayingthatatleastonevectorinthissetcanbewrittenasalinearcombinationoftheothers.DEFINITIOND.11(Rank)(i)LetAbeannmmatrix.TherankofamatrixA,denotedrank(A),isthemaxi-mumnumberoflinearlyindependentcolumnsofA.(ii)IfAisnmandrank(A)m,thenAhasfullcolumnrank.749\nxd7/14/999:28PMPage750AppendixDSummaryofMatrixAlgebraIfAisnm,itsrankcanbeatmostm.Amatrixhasfullcolumnrankifitscolumnsformalinearlyindependentset.Forexample,the32matrix132600canhaveatmostranktwo.Infact,itsrankisonlyonebecausethesecondcolumnisthreetimesthefirstcolumn.PROPERTIESOFRANK:(1)rank(A)rank(A);(2)IfAisnk,thenrank(A)min(n,k);(3)IfAiskkandrank(A)k,thenAisnonsingular.D.4QUADRATICFORMSANDPOSITIVEDEFINITEMATRICESDEFINITIOND.12(QuadraticForm)LetAbeannnsymmetricmatrix.ThequadraticformassociatedwiththematrixAisthereal-valuedfunctiondefinedforalln1vectorsx:nn2f(x)xAxaiixi2aijxixj.i1i1jiDEFINITIOND.13(PositiveDefiniteandPositiveSemi-Definite)(i)AsymmetricmatrixAissaidtobepositivedefinite(p.d.)ifxAx0foralln1vectorsxexceptx0.(ii)AsymmetricmatrixAispositivesemi-definite(p.s.d.)ifxAx0foralln1vectors.Ifamatrixispositivedefiniteorpositivesemi-definite,itisautomaticallyassumedtobesymmetric.PROPERTIESOFPOSITIVEDEFINITEANDPOSITIVESEMI-DEFINITEMATRICES:(1)Apositivedefinitematrixhasdiagonalelementsthatarestrictlypositive,whilea1p.s.d.matrixhasnonnegativediagonalelements;(2)IfAisp.d.,thenAexistsandisp.d.;(3)IfXisnk,thenXXandXXarep.s.d.;(4)IfXisnkandrank(X)k,thenXXisp.d.(andthereforenonsingular).D.5IDEMPOTENTMATRICESDEFINITIOND.14(IdempotentMatrix)LetAbeannnsymmetricmatrix.ThenAissaidtobeanidempotentmatrixifandonlyifAAA.Forexample,750\nxd7/14/999:28PMPage751AppendixDSummaryofMatrixAlgebra100000001isanidempotentmatrix,asdirectmultiplicationverifies.PROPERTIESOFIDEMPOTENTMATRICES:LetAbeannnidempotentmatrix.(1)rank(A)tr(A);(2)Aispositivesemi-definite.Wecanconstructidempotentmatricesverygenerally.LetXbeannkmatrixwithrank(X)k.Define1PX(XX)X1MInX(XX)XInP.ThenPandMaresymmetric,idempotentmatriceswithrank(P)kandrank(M)1nk.TheranksaremosteasilyobtainedbyusingProperty1:tr(P)tr[(XX)XX](fromProperty5fortrace)tr(Ik)k(byProperty1fortrace).Iteasilyfollowsthattr(M)tr(In)tr(P)nk.D.6DIFFERENTIATIONOFLINEARANDQUADRATICFORMSForagivenn1vectora,considerthelinearfunctiondefinedbyf(x)ax,foralln1vectorsx.Thederivativeoffwithrespecttoxisthe1nvectorofpartialderivatives,whichissimplyf(x)/xa.ForannnsymmetricmatrixA,definethequadraticformg(x)xAx.Then,g(x)/x2xA,whichisa1nvector.D.7MOMENTSANDDISTRIBUTIONSOFRANDOMVECTORSInordertoderivetheexpectedvalueandvarianceoftheOLSestimatorsusingmatri-ces,weneedtodefinetheexpectedvalueandvarianceofarandomvector.Asitsnamesuggests,arandomvectorissimplyavectorofrandomvariables.Wealsoneedtodefinethemultivariatenormaldistribution.TheseconceptsaresimplyextensionsofthosecoveredinAppendixB.751\nxd7/14/999:28PMPage752AppendixDSummaryofMatrixAlgebraExpectedValueDEFINITIOND.15(ExpectedValue)(i)Ifyisann1randomvector,theexpectedvalueofy,denotedE(y),isthevectorofexpectedvalues:E(y)[E(y1),E(y2),…,E(yn)].(ii)IfZisannmrandommatrix,E(Z)isthenmmatrixofexpectedvalues:E(Z)[E(zij)].PROPERTIESOFEXPECTEDVALUE:(1)IfAisanmnmatrixandbisann1vector,wherebotharenonrandom,thenE(Ayb)AE(y)b;(2)IfAispnandBismk,wherebotharenonrandom,thenE(AZB)AE(Z)B.Variance-CovarianceMatrixDEFINITIOND.16(Variance-CovarianceMatrix)Ifyisann1randomvector,itsvariance-covariancematrix,denotedVar(y),isdefinedas2112...1n2212...2n.Var(y).,.2n1n2...n2wherejVar(yj)andijCov(yi,yj).Inotherwords,thevariance-covariancematrixhasthevariancesofeachelementofydownitsdiagonal,withcovariancetermsintheoffdiagonals.BecauseCov(yi,yj)Cov(yj,yi),itimmediatelyfollowsthatavariance-covariancematrixissymmetric.PROPERTIESOFVARIANCE:(1)Ifaisann1nonrandomvector,thenVar(ay)a[Var(y)]a0;(2)IfVar(ay)0foralla0,Var(y)ispositivedefinite;(3)Var(y)E[(y)(y)],whereE(y);(4)Iftheelementsofyareuncorre-2lated,Var(y)isadiagonalmatrix.If,inaddition,Var(yj)forj1,2,…,n,then2Var(y)In;(5)IfAisanmnnonrandommatrixandbisann1nonrandomvector,thenVar(Ayb)A[Var(y)]A.MultivariateNormalDistributionThenormaldistributionforarandomvariablewasdiscussedatsomelengthinAppendixB.Weneedtoextendthenormaldistributiontorandomvectors.Wewillnotprovideanexpressionfortheprobabilitydistributionfunction,aswedonotneedit.Itisimportanttoknowthatamultivariatenormalrandomvectoriscompletelycharacter-izedbyitsmeananditsvariance-covariancematrix.Therefore,ifyisann1multi-variatenormalrandomvectorwithmeanandvariance-covariancematrix,wewritey~Normal(,).Wenowstateseveralusefulpropertiesofthemultivariatenormaldistribution.752\nxd7/14/999:28PMPage753AppendixDSummaryofMatrixAlgebraPROPERTIESOFTHEMULTIVARIATENORMALDISTRIBUTION:(1)Ify~Normal(,),theneachelementofyisnormallydistributed;(2)Ify~Normal(,),thenyiandyj,anytwoelementsofy,areindependentifandonlyiftheyareuncorre-lated,thatis,ij0;(3)Ify~Normal(,),thenAyb~Normal(Ab,AA),whereAandbarenonrandom;(4)Ify~Normal(0,),then,fornonrandommatrices2AandB,AyandByareindependentifandonlyifAB0.Inparticular,ifIn,thenAB0isnecessaryandsufficientforindependenceofAyandBy;(5)Ify~2Normal(0,In),Aisaknnonrandommatrix,andBisannnsymmetric,idem-potentmatrix,thenAyandyByareindependentifandonlyifAB0;(6)Ify~2Normal(0,In)andAandBarenonrandomsymmetric,idempotentmatrices,thenyAyandyByareindependentifandonlyifAB0.Chi-SquareDistributionInAppendixB,wedefinedachi-squarerandomvariableasthesumofsquaredinde-pendentstandardnormalrandomvariables.Invectornotation,ifu~Normal(0,In),then2uu~n.PROPERTIESOFTHECHI-SQUAREDISTRIBUTION:(1)Ifu~Normal(0,In)andAis2annnsymmetric,idempotentmatrixwithrank(A)q,thenuAu~q;(2)Ifu~Normal(0,In)andAandBarennsymmetric,idempotentmatricessuchthatAB0,thenuAuanduBuareindependent,chi-squarerandomvariables.tDistributionWealsodefinedthetdistributioninAppendixB.Nowweaddanimportantproperty.PROPERTYOFTHEtDISTRIBUTION:Ifu~Normal(0,In),cisann1nonrandomvector,Aisanonrandomnnsymmetric,idempotentmatrixwithrankq,andAc1/21/20,then{cu/(cc)}/(uAu)~tq.FDistributionRecallthatanFrandomvariableisobtainedbytakingtwoindependentchi-squarerandomvariablesandfindingtheratioofeachstandardizedbydegreesoffreedom.PROPERTYOFTHEFDISTRIBUTION:Ifu~Normal(0,In)andAandBarennnon-randomsymmetric,idempotentmatriceswithrank(A)k1,rank(B)k2,andAB0,then(uAu/k1)/(uBu/k2)~Fk1,k2.SUMMARYThisappendixcontainsacondensedformofthebackgroundinformationneededtostudytheclassicallinearmodelusingmatrices.Whilethematerialhereisself-contained,itisprimarilyintendedasareviewforreaderswhoarefamiliarwithmatrixalgebraandmultivariatestatistics,anditwillbeusedextensivelyinAppendixE.753\nxd7/14/999:28PMPage754AppendixDSummaryofMatrixAlgebraKEYTERMSChi-SquareRandomVariablePositiveSemi-DefiniteColumnVectorQuadraticFormDiagonalMatrixRandomVectorExpectedValueRankofaMatrixFRandomVariableRowVectorIdempotentMatrixScalarMultiplicationIdentityMatrixSquareMatrixInverseSymmetricMatrixLinearlyIndependentVectorstDistributionMatrixTraceofaMatrixMatrixMultiplicationTransposeMultivariateNormalDistributionVariance-CovarianceMatrixPositiveDefiniteZeroMatrixPROBLEMSD.1i(i)FindtheproductABusing016217A,B180.450300(ii)DoesBAexist?D.2IfAandBarenndiagonalmatrices,showthatABBA.D.3LetXbeanynkmatrix.ShowthatXXisasymmetricmatrix.D.4(i)iUsethepropertiesoftracetoarguethattr(AA)tr(AA)foranynmma-trixA.201(ii)ForA030,verifythattr(AA)tr(AA).D.5(i)iUsethedefinitionofinversetoprovethefollowing:ifAandBarenn111nonsingularmatrices,then(AB)BA.1(ii)IfA,B,andCareallnnnonsingularmatrices,find(ABC)intermsof111A,B,andC.D.6(i)iShowthatifAisannnsymmetric,positivedefinitematrix,thenAmusthavestrictlypositivediagonalelements.(ii)Writedowna22symmetricmatrixwithstrictlypositivediagonalele-mentsthatisnotpositivedefinite.D.7LetAbeannnsymmetric,positivedefinitematrix.ShowthatifPisanynnnonsingularmatrix,thenPAPispositivedefinite.D.8ProveProperty5ofvariancesforvectors,usingProperty3.754\n\nxd7/14/999:31PMPage755AppendixETheLinearRegressionModelinMatrixFormhisappendixderivesvariousresultsforordinaryleastsquaresestimationoftheTmultiplelinearregressionmodelusingmatrixnotationandmatrixalgebra(seeAppendixDforasummary).Thematerialpresentedhereismuchmoread-vancedthanthatinthetext.E.1THEMODELANDORDINARYLEASTSQUARESESTIMATIONThroughoutthisappendix,weusethetsubscripttoindexobservationsandanntodenotethesamplesize.Itisusefultowritethemultiplelinearregressionmodelwithkparametersasfollows:yt12xt23xt3…kxtkut,t1,2,…,n,(E.1)whereytisthedependentvariableforobservationt,andxtj,j2,3,…,k,aretheinde-pendentvariables.Noticehowourlabelingconventionherediffersfromthetext:wecalltheintercept1andlet2,…,kdenotetheslopeparameters.Thisrelabelingisnotimportant,butitsimplifiesthematrixapproachtomultipleregression.Foreacht,definea1kvector,xt(1,xt2,…,xtk),andlet(1,2,…,k)bethek1vectorofallparameters.Then,wecanwrite(E.1)asytxtut,t1,2,…,n.(E.2)[Someauthorsprefertodefinextasacolumnvector,inwhichcase,xtisreplacedwithxtin(E.2).Mathematically,itmakesmoresensetodefineitasarowvector.]Wecanwrite(E.2)infullmatrixnotationbyappropriatelydefiningdatavectorsandthmatrices.Letydenotethen1vectorofobservationsony:thetelementofyisyt.LetXbethenkvectorofobservationsontheexplanatoryvariables.Inotherththwords,thetrowofXconsistsofthevectorxt.Equivalently,the(t,j)elementofXissimplyxtj:755\nxd7/14/999:31PMPage756AppendixETheLinearRegressionModelinMatrixFormx11x12x13...x1kx21x22x23...x2kX.....nk..xn1xn2xn3...xnkFinally,letubethen1vectorofunobservabledisturbances.Then,wecanwrite(E.2)forallnobservationsinmatrixnotation:yXu.(E.3)Remember,becauseXisnkandisk1,Xisn1.Estimationofproceedsbyminimizingthesumofsquaredresiduals,asinSection3.2.Definethesumofsquaredresidualsfunctionforanypossiblek1parametervec-torbasn2SSR(b)(ytxtb).t1Thek1vectorofordinaryleastsquaresestimates,ˆ(ˆ1,ˆ2,…,ˆk),minimizesSSR(b)overallpossiblek1vectorsb.Thisisaprobleminmultivariablecalculus.Forˆtominimizethesumofsquaredresiduals,itmustsolvethefirstorderconditionSSR(ˆ)/b0.(E.4)2Usingthefactthatthederivativeof(ytxtb)withrespecttobisthe1kvector2(ytxtb)xt,(E.4)isequivalenttonxt(ytxtˆ)0.(E.5)t1(Wehavedividedby2andtakenthetranspose.)Wecanwritethisfirstordercondi-tionasn(ytˆ1ˆ2xt2…ˆkxtk)0t1nxt2(ytˆ1ˆ2xt2…ˆkxtk)0t1...nxtk(ytˆ1ˆ2xt2…ˆkxtk)0,t1which,apartfromthedifferentlabelingconvention,isidenticaltothefirstordercondi-tionsinequation(3.13).Wewanttowritetheseinmatrixformtomakethemmoreuse-ful.UsingtheformulaforpartitionedmultiplicationinAppendixD,weseethat(E.5)isequivalentto756\nxd7/14/999:31PMPage757AppendixETheLinearRegressionModelinMatrixFormX(yXˆ)0(E.6)or(XX)ˆXy.(E.7)Itcanbeshownthat(E.7)alwayshasatleastonesolution.Multiplesolutionsdonothelpus,aswearelookingforauniquesetofOLSestimatesgivenourdataset.AssumingthatthekksymmetricmatrixXXisnonsingular,wecanpremultiplyboth1sidesof(E.7)by(XX)tosolvefortheOLSestimatorˆ:1ˆ(XX)Xy.(E.8)Thisisthecriticalformulaformatrixanalysisofthemultiplelinearregressionmodel.TheassumptionthatXXisinvertibleisequivalenttotheassumptionthatrank(X)k,whichmeansthatthecolumnsofXmustbelinearlyindependent.Thisisthematrixver-sionofMLR.4inChapter3.Beforewecontinue,(E.8)warrantsawordofwarning.Itistemptingtosimplifytheformulaforˆasfollows:1111ˆ(XX)XyX(X)XyXy.TheflawinthisreasoningisthatXisusuallynotasquarematrix,andsoitcannotbe111inverted.Inotherwords,wecannotwrite(XX)X(X)unlessnk,acasethatvirtuallyneverarisesinpractice.Then1vectorsofOLSfittedvaluesandresidualsaregivenbyyˆXˆ,uˆyyˆyXˆ.From(E.6)andthedefinitionofuˆ,wecanseethatthefirstorderconditionforˆisthesameasXuˆ0.(E.9)BecausethefirstcolumnofXconsistsentirelyofones,(E.9)impliesthattheOLSresidualsalwayssumtozerowhenaninterceptisincludedintheequationandthatthesamplecovariancebetweeneachindependentvariableandtheOLSresidualsiszero.(WediscussedbothofthesepropertiesinChapter3.)Thesumofsquaredresidualscanbewrittenasn2SSRuˆtuˆuˆ(yXˆ)(yXˆ).(E.10)t1AllofthealgebraicpropertiesfromChapter3canbederivedusingmatrixalgebra.Forexample,wecanshowthatthetotalsumofsquaresisequaltotheexplainedsumofsquaresplusthesumofsquaredresiduals[see(3.27)].Theuseofmatricesdoesnotpro-videasimplerproofthansummationnotation,sowedonotprovideanotherderivation.757\nxd7/14/999:31PMPage758AppendixETheLinearRegressionModelinMatrixFormThematrixapproachtomultipleregressioncanbeusedasthebasisforageometri-calinterpretationofregression.ThisinvolvesmathematicalconceptsthatareevenmoreadvancedthanthosewecoveredinAppendixD.[SeeGoldberger(1991)orGreene(1997).]E.2FINITESAMPLEPROPERTIESOFOLSDerivingtheexpectedvalueandvarianceoftheOLSestimatorˆisfacilitatedbymatrixalgebra,butwemustshowsomecareinstatingtheassumptions.ASSUMPTIONE.1(LINEARINPARAMETERS)Themodelcanbewrittenasin(E.3),whereyisanobservedn1vector,Xisannkobservedmatrix,anduisann1vectorofunobservederrorsordisturbances.ASSUMPTIONE.2(ZEROCONDITIONALMEAN)ConditionalontheentirematrixX,eacherroruthaszeromean:E(utX)0,t1,2,…,n.Invectorform,E(uX)0.(E.11)ThisassumptionisimpliedbyMLR.3undertherandomsamplingassumption,MLR.2.Intimeseriesapplications,AssumptionE.2imposesstrictexogeneityontheexplana-toryvariables,somethingdiscussedatlengthinChapter10.Thisrulesoutexplanatoryvariableswhosefuturevaluesarecorrelatedwithut;inparticular,iteliminateslaggeddependentvariables.UnderAssumptionE.2,wecanconditiononthextjwhenwecom-putetheexpectedvalueofˆ.ASSUMPTIONE.3(NOPERFECTCOLLINEARITY)ThematrixXhasrankk.Thisisacarefulstatementoftheassumptionthatrulesoutlineardependenciesamongtheexplanatoryvariables.UnderAssumptionE.3,XXisnonsingular,andsoˆisuniqueandcanbewrittenasin(E.8).THEOREME.1(UNBIASEDNESSOFOLS)UnderAssumptionsE.1,E.2,andE.3,theOLSestimatorˆisunbiasedfor.PROOF:UseAssumptionsE.1andE.3andsimplealgebratowrite11ˆ(XX)Xy(XX)X(Xu)(E.12)111(XX)(XX)(XX)Xu(XX)Xu,1whereweusethefactthat(XX)(XX)Ik.TakingtheexpectationconditionalonXgives758\nxd7/14/999:31PMPage759AppendixETheLinearRegressionModelinMatrixForm1E(ˆX)(XX)XE(uX)1(XX)X0,becauseE(uX)0underAssumptionE.2.Thisargumentclearlydoesnotdependonthevalueof,sowehaveshownthatˆisunbiased.Toobtainthesimplestformofthevariance-covariancematrixofˆ,weimposetheassumptionsofhomoskedasticityandnoserialcorrelation.ASSUMPTIONE.4(HOMOSKEDASTICITYANDNOSERIALCORRELATION)2(i)Var(utX),t1,2,…,n.(ii)Cov(ut,usX)0,forallts.Inmatrixform,wecanwritethesetwoassumptionsas2Var(uX)In,(E.13)whereInisthennidentitymatrix.Part(i)ofAssumptionE.4isthehomoskedasticityassumption:thevarianceofutcan-notdependonanyelementofX,andthevariancemustbeconstantacrossobservations,t.Part(ii)isthenoserialcorrelationassumption:theerrorscannotbecorrelatedacrossobservations.Underrandomsampling,andinanyothercross-sectionalsamplingschemeswithindependentobservations,part(ii)ofAssumptionE.4automaticallyholds.Fortimeseriesapplications,part(ii)rulesoutcorrelationintheerrorsovertime(bothconditionalonXandunconditionally).Becauseof(E.13),weoftensaythatuhasscalarvariance-covariancematrixwhenAssumptionE.4holds.Wecannowderivethevariance-covariancematrixoftheOLSestimator.THEOREME.2(VARIANCE-COVARIANCEMATRIXOFTHEOLSESTIMATOR)UnderAssumptionsE.1throughE.4,21Var(ˆX)(XX).(E.14)PROOF:Fromthelastformulainequation(E.12),wehave111Var(ˆX)Var[(XX)XuX](XX)X[Var(uX)]X(XX).Now,weuseAssumptionE.4toget121Var(ˆX)(XX)X(In)X(XX)21121(XX)XX(XX)(XX).759\nxd7/14/999:31PMPage760AppendixETheLinearRegressionModelinMatrixFormFormula(E.14)meansthatthevarianceofˆj(conditionalonX)isobtainedbymulti-2th1plyingbythejdiagonalelementof(XX).Fortheslopecoefficients,wegaveaninterpretableformulainequation(3.51).Equation(E.14)alsotellsushowtoobtainthe2covariancebetweenanytwoOLSestimates:multiplybytheappropriateoffdiago-1nalelementof(XX).InChapter4,weshowedhowtoavoidexplicitlyfindingcovariancesforobtainingconfidenceintervalsandhypothesestestsbyappropriatelyrewritingthemodel.TheGauss-MarkovTheorem,initsfullgenerality,canbeproven.THEOREME.3(GAUSS-MARKOVTHEOREM)UnderAssumptionsE.1throughE.4,ˆisthebestlinearunbiasedestimator.PROOF:Anyotherlinearestimatorofcanbewrittenas˜Ay,(E.15)whereAisannkmatrix.Inorderfor˜tobeunbiasedconditionalonX,AcanconsistofnonrandomnumbersandfunctionsofX.(Forexample,Acannotbeafunctionofy.)ToseewhatfurtherrestrictionsonAareneeded,write˜A(Xu)(AX)Au.(E.16)Then,E(˜X)AXE(AuX)AXAE(uX)sinceAisafunctionofXAXsinceE(uX)0.For˜tobeanunbiasedestimatorof,itmustbetruethatE(˜X)forallk1vec-tors,thatis,AXforallk1vectors.(E.17)BecauseAXisakkmatrix,(E.17)holdsifandonlyifAXIk.Equations(E.15)and(E.17)characterizetheclassoflinear,unbiasedestimatorsfor.Next,from(E.16),wehave2Var(˜X)A[Var(uX)]AAA,byAssumptionE.4.Therefore,21Var(˜X)Var(ˆX)[AA(XX)]21[AAAX(XX)XA]becauseAXIk21A[InX(XX)X]A2AMA,1whereMInX(XX)X.BecauseMissymmetricandidempotent,AMAispositivesemi-definiteforanynkmatrixA.ThisestablishesthattheOLSestimatorˆisBLUE.How760\nxd7/14/999:31PMPage761AppendixETheLinearRegressionModelinMatrixFormisthissignificant?Letcbeanyk1vectorandconsiderthelinearcombinationcc11c22…ckk,whichisascalar.Theunbiasedestimatorsofcarecˆandc˜.ButVar(c˜X)Var(cˆX)c[Var(˜X)Var(ˆX)]c0,because[Var(˜X)Var(ˆX)]isp.s.d.Therefore,whenitisusedforestimatinganylinearcombinationof,OLSyieldsthesmallestvariance.Inparticular,Var(ˆjX)Var(˜jX)foranyotherlinear,unbiasedestimatorofj.2Theunbiasedestimatoroftheerrorvariancecanbewrittenas2ˆuˆuˆ/(nk),wherewehavelabeledtheexplanatoryvariablessothattherearektotalparameters,includingtheintercept.2THEOREME.4(UNBIASEDNESSOFˆ)22222UnderAssumptionsE.1throughE.4,ˆisunbiasedfor:E(ˆX)forall0.1PROOF:WriteuˆyXˆyX(XX)XyMyMu,whereMIn1X(XX)X,andthelastequalityfollowsbecauseMX0.BecauseMissymmetricandidempotent,uˆuˆuMMuuMu.BecauseuMuisascalar,itequalsitstrace.Therefore,E(uMuX)E[tr(uMu)X]E[tr(Muu)X]tr[E(Muu|X)]tr[ME(uu|X)]222tr(MIn)tr(M)(nk).11Thelastequalityfollowsfromtr(M)tr(In)tr[X(XX)X]ntr[(XX)XX]ntr(Ik)nk.Therefore,22E(ˆX)E(uMuX)/(nk).E.3STATISTICALINFERENCEWhenweaddthefinalclassicallinearmodelassumption,ˆhasamultivariatenormaldistribution,whichleadstothetandFdistributionsforthestandardteststatisticscov-eredinChapter4.ASSUMPTIONE.5(NORMALITYOFERRORS)2ConditionalonX,theutareindependentandidenticallydistributedasNormal(0,).Equivalently,ugivenXisdistributedasmultivariatenormalwithmeanzeroandvariance-22covariancematrixIn:u~Normal(0,In).761\nxd7/14/999:31PMPage762AppendixETheLinearRegressionModelinMatrixFormUnderAssumptionE.5,eachutisindependentoftheexplanatoryvariablesforallt.Inatimeseriessetting,thisisessentiallythestrictexogeneityassumption.THEOREME.5(NORMALITYOFˆ)UndertheclassicallinearmodelAssumptionsE.1throughE.5,ˆconditionalonXisdis-21tributedasmultivariatenormalwithmeanandvariance-covariancematrix(XX).TheoremE.5isthebasisforstatisticalinferenceinvolving.Infact,alongwiththepropertiesofthechi-square,t,andFdistributionsthatwesummarizedinAppendixD,wecanuseTheoremE.5toestablishthattstatisticshaveatdistributionunderAssumptionsE.1throughE.5(underthenullhypothesis)andlikewiseforFstatistics.Weillustratewithaproofforthetstatistics.THEOREME.6UnderAssumptionsE.1throughE.5,(ˆjj)/se(ˆj)~tnk,j1,2,…,k.PROOF:Theproofrequiresseveralsteps;thefollowingstatementsareinitiallyconditionalonX.First,byTheoremE.5,(ˆjj)/sd(ˆ)~Normal(0,1),wheresd(ˆj)th1cjj,andcjjisthejdiagonalelementof(XX).Next,underAssumptionsE.1throughE.5,conditionalonX,222(nk)ˆ/~nk.(E.18)22Thisfollowsbecause(nk)ˆ/(u/)M(u/),whereMisthennsymmetric,idem-potentmatrixdefinedinTheoremE.4.Butu/~Normal(0,In)byAssumptionE.5.Itfollows2fromProperty1forthechi-squaredistributioninAppendixDthat(u/)M(u/)~nk(becauseMhasranknk).21Wealsoneedtoshowthatˆandˆareindependent.Butˆ(XX)Xu,and21ˆuMu/(nk).Now,[(XX)X]M0becauseXM0.Itfollows,fromProperty5ofthemultivariatenormaldistributioninAppendixD,thatˆandMuareindependent.22SinceˆisafunctionofMu,ˆandˆarealsoindependent.Finally,wecanwrite221/2(ˆjj)/se(ˆj)[(ˆjj)/sd(ˆj)]/(ˆ/),whichistheratioofastandardnormalrandomvariableandthesquarerootofa2nk/(nk)randomvariable.Wejustshowedthattheseareindependent,andso,bydef-initionofatrandomvariable,(ˆjj)/se(ˆj)hasthetnkdistribution.Becausethisdistri-butiondoesnotdependonX,itistheunconditionaldistributionof(ˆjj)/se(ˆj)aswell.Fromthistheorem,wecanpluginanyhypothesizedvalueforjandusethetstatisticfortestinghypotheses,asusual.UnderAssumptionsE.1throughE.5,wecancomputewhatisknownastheCramer-Raolowerboundforthevariance-covariancematrixofunbiasedestimatorsof(again762\nxd7/14/999:31PMPage763AppendixETheLinearRegressionModelinMatrixForm21conditionalonX)[seeGreene(1997,Chapter4)].Thiscanbeshowntobe(XX),whichisexactlythevariance-covariancematrixoftheOLSestimator.Thisimpliesthatˆistheminimumvarianceunbiasedestimatorof(conditionalonX):Var(˜X)Var(ˆX)ispositivesemi-definiteforanyotherunbiasedestimator˜;wenolongerhavetorestrictourattentiontoestimatorslineariny.ItiseasytoshowthattheOLSestimatorisinfactthemaximumlikelihoodestima-torofunderAssumptionE.5.Foreacht,thedistributionofytgivenXis2Normal(xt,).BecausetheytareindependentconditionalonX,thelikelihoodfunc-tionforthesampleisobtainedfromtheproductofthedensities:n21/222(2)exp[(ytxt)/(2)].t12Maximizingthisfunctionwithrespecttoandisthesameasmaximizingitsnat-urallogarithm:n222[(1/2)log(2)(ytxt)/(2)].t1n22Forobtainingˆ,thisisthesameasminimizing(ytxt)—thedivisionby2t1doesnotaffecttheoptimization—whichisjusttheproblemthatOLSsolves.Theesti-22matorofthatwehaveused,SSR/(nk),turnsoutnottobetheMLEof;the2MLEisSSR/n,whichisabiasedestimator.BecausetheunbiasedestimatorofresultsintandFstatisticswithexacttandFdistributionsunderthenull,itisalwaysusedinsteadoftheMLE.SUMMARYThisappendixhasprovidedabriefdiscussionofthelinearregressionmodelusingmatrixnotation.Thismaterialisincludedformoreadvancedclassesthatusematrixalgebra,butitisnotneededtoreadthetext.Ineffect,thisappendixprovessomeoftheresultsthatweeitherstatedwithoutproof,provedonlyinspecialcases,orprovedthroughamorecumbersomemethodofproof.Othertopics—suchasasymptoticprop-erties,instrumentalvariablesestimation,andpaneldatamodels—canbegivenconcisetreatmentsusingmatrices.Advancedtextsineconometrics,includingDavidsonandMacKinnon(1993),Greene(1997),andWooldridge(1999),canbeconsultedfordetails.KEYTERMSFirstOrderConditionScalarVariance-CovarianceMatrixMatrixNotationVariance-CovarianceMatrixoftheOLSMinimumVarianceUnbiasedEstimator763\nxd7/14/999:31PMPage764AppendixETheLinearRegressionModelinMatrixFormPROBLEMSE.1Letxtbethe1kvectorofexplanatoryvariablesforobservationt.ShowthattheOLSestimatorˆcanbewrittenasn1nˆxtxtxtyt.t1t1Dividingeachsummationbynshowsthatˆisafunctionofsampleaverages.E.2Letˆbethek1vectorofOLSestimates.(i)Showthatforanyk1vectorb,wecanwritethesumofsquaredresidualsasSSR(b)uˆuˆ(ˆb)XX(ˆb).[Hint:Write(yXb)(yXb)[uˆX(ˆb)][uˆX(ˆb)]andusethefactthatXuˆ0.](ii)ExplainhowtheexpressionforSSR(b)inpart(i)provesthatˆuniquelyminimizesSSR(b)overallpossiblevaluesofb,assumingXhasrankk.E.3LetˆbetheOLSestimatefromtheregressionofyonX.LetAbeakknon-singularmatrixanddefineztxtA,t1,…,n.Therefore,ztis1kandisanon-singularlinearcombinationofxt.LetZbethenkmatrixwithrowszt.Let˜denotetheOLSestimatefromaregressionofyonZ.1(i)Showthat˜Aˆ.(ii)Letyˆtbethefittedvaluesfromtheoriginalregressionandlety˜tbethefittedvaluesfromregressingyonZ.Showthaty˜tyˆt,forallt1,2,…,n.Howdotheresidualsfromthetworegressionscompare?2111(iii)Showthattheestimatedvariancematrixfor˜isˆA(XX)A,2whereˆistheusualvarianceestimatefromregressingyonX.(iv)LettheˆjbetheOLSestimatesfromregressingyton1,xt2,…,xtk,andletthe˜jbetheOLSestimatesfromtheregressionofyton1,a2xt2,…,akxtk,whereaj0,j2,…,k.Usetheresultsfrompart(i)tofindtherelationshipbetweenthe˜jandtheˆj.(v)Assumingthesetupofpart(iv),usepart(iii)toshowthatse(˜j)se(ˆj)/aj.(vi)Assumingthesetupofpart(iv),showthattheabsolutevaluesofthetstatisticsfor˜jandˆjareidentical.764\nxd7/14/999:34PMPage765AppendixFAnswerstoChapterQuestionsCHAPTER2QUESTION2.1Whenstudentability,motivation,age,andotherfactorsinuarenotrelatedtoatten-dance,(2.6)wouldhold.Thisseemsunlikelytobethecase.QUESTION2.2About$9.64.Toseethis,fromtheaveragewagesmeasuredin1976and1997dollars,wecangettheCPIdeflatoras16.64/5.902.82.Whenwemultiply3.42by2.82,weobtainabout9.64.QUESTION2.359.26,ascanbeseenbypluggingshareA60intoequation(2.28).Thisisnotunrea-sonable:ifCandidateAspends60%ofthetotalmoneyspent,heorsheispredictedtoreceivejustover59%ofthevote.QUESTION2.4Theequationwillbesalaˆryhun9,631.91185.01roe,asiseasilyseenbymulti-plyingequation(2.39)by10.QUESTION2.5nn2122Equation(2.58)canbewrittenasVar(ˆ0)(n)xi(xix¯),wherei1i121thetermmultiplyingnisgreaterthanorequaltoone,butitisequaltooneifand2onlyifx¯0.Inthiscase,thevarianceisassmallasitcanpossiblybe:Var(ˆ0)/n.CHAPTER3QUESTION3.1Justafewfactorsincludeageandgenderdistribution,sizeofthepoliceforce(or,moregenerally,resourcesdevotedtocrimefighting),population,andgeneralhistoricalfac-tors.Thesefactorscertainlymightbecorrelatedwithprbconvandavgsen,whichmeans(3.5)wouldnothold.Forexample,sizeofthepoliceforceispossiblycorrelatedwith765\nxd7/14/999:34PMPage766AppendixFAnswerstoChapterQuestionsbothprbconandavgsen,assomecitiesputmoreeffortintocrimepreventionandenforcement.Weshouldtrytobringasmanyofthesefactorsintotheequationaspos-sible.QUESTION3.2WeusethethirdpropertyofOLSconcerningpredictedvaluesandresiduals:whenweplugtheaveragevaluesofallindependentvariablesintotheOLSregressionline,weobtaintheaveragevalueofthedependentvariable.SocolGPA1.29.453hsGPA.0094ACT1.29.453(3.4).0094(24.2)3.06.YoucanchecktheaverageofcolGPAinGPA1.RAWtoverifythistotheseconddecimalplace.QUESTION3.3No.ThevariableshareAisnotanexactlinearfunctionofexpendAandexpendB,eventhoughitisanexactnonlinearfunction:shareA100[expendA/(expendAexpendB)].Therefore,itislegitimatetohaveexpendA,expendB,andshareAasexplana-toryvariables.QUESTION3.4AswediscussedinSection3.4,ifweareinterestedintheeffectofx1ony,correla-tionamongtheotherexplanatoryvariables(x2,x3,andsoon)doesnotaffectVar(ˆ1).Thesevariablesareincludedascontrols,andwedonothavetoworryaboutthiskindofcollinearity.Ofcourse,wearecontrollingforthemprimarilybecausewethinktheyarecorrelatedwithattendance,butthisisnecessarytoperformaceterisparibusanalysis.CHAPTER4QUESTION4.1Undertheseassumptions,theGauss-Markovassumptionsaresatisfied:uisindepen-dentoftheexplanatoryvariables,soE(ux1,…,xk)E(u),andVar(ux1,…,xk)Var(u).Further,itiseasilyseenthatE(u)0.Therefore,MLR.3andMLR.5hold.Theclassicallinearmodelassumptionsarenotsatisfied,becauseuisnotnormallydistrib-uted(whichisaviolationofMLR.6).QUESTION4.2H0:10,H1:10.QUESTION4.3Becauseˆ1.560andwearetestingagainstH1:10,theone-sidedp-valueisone-halfofthetwo-sidedp-value,or.043.QUESTION4.4H0:56780.k8andq4.Therestrictedversionofthemodelisscore01classize2expend3tchcomp4enrollu.766\nxd7/14/999:34PMPage767AppendixFAnswerstoChapterQuestionsQUESTION4.5TheFstatisticfortestingexclusionofACTis[(.291.183)/(1.291)](6803)103.13.Therefore,theabsolutevalueofthetstatisticisabout10.16.ThetstatisticonACTisnegative,becauseˆACTisnegative,sotACT10.16.QUESTION4.6Notbymuch.TheFtestforjointsignificanceofdroprateandgradrateiseasilycom-putedfromtheR-squaredsinthetable:F[(.361.353)/(1.361)](402/2)2.52.The10%criticalvalueisobtainedfromTableG.3(a)as2.30,whilethe5%criticalvaluefromTableG.3(b)is3.Thep-valueisabout.082.Thus,droprateandgradratearejointlysignificantatthe10%level,butnotatthe5%level.Inanycase,controllingforthesevariableshasaminoreffectontheb/scoefficient.CHAPTER5QUESTION5.1Thisrequiressomeassumptions.Itseemsreasonabletoassumethat20(scoredependspositivelyonpriGPA)andCov(skipped,priGPA)0(skippedandpriGPAarenegativelycorrelated).Thismeansthat210,whichmeansthatplim˜11.Because1isthoughttobenegative(oratleastnonpositive),asimpleregressionislikelytooverestimatetheimportanceofskippingclasses.QUESTION5.2ˆj1.96se(ˆj)istheasymptotic95%confidenceinterval.Or,wecanreplace1.96with2.CHAPTER6QUESTION6.1Becausefincdol1,000faminc,thecoefficientonfincdolwillbethecoefficientonfamincdividedby1,000,or.0927/1,000.0000927.Thestandarderroralsodropsbyafactorof1,000,andsothetstatisticdoesnotchange,nordoanyoftheotherOLSstatistics.Forreadability,itisbettertomeasurefamilyincomeinthousandsofdollars.QUESTION6.2Wecandothisgenerally.Theequationislog(y)01log(x1)2x2…,wherex2isaproportionratherthanapercentage.Then,ceterisparibus,log(y)2x2,100log(y)2(100x2),or%y2(100x2).Now,becausex2isthechangeintheproportion,100x2isapercentagepointchange.Inparticular,ifx2.01,then100x21,whichcorrespondstoaonepercentagepointchange.Butthen2isthepercentagechangeinywhen100x21.767\nxd7/14/999:34PMPage768AppendixFAnswerstoChapterQuestionsQUESTION6.3Thenewmodelwouldbestndfnl01atndrte2priGPA3ACT224priGPA5ACT6priGPAatndrte7ACTatndrteu.Therefore,thepar-tialeffectofatndrteonstndfnlis16priGPA7ACT.Thisiswhatwemultiplybyatndrtetoobtaintheceterisparibuschangeinstndfnl.QUESTION6.422Fromequation(6.21),R¯1ˆ/[SST/(n1)].Foragivensampleandagivendependentvariable,SST/(n1)isfixed.Whenweusedifferentsetsofexplanatory222variables,onlyˆchanges.Asˆdecreases,R¯increases.Ifwemakeˆ,andtherefore22ˆ,assmallaspossible,wearemakingR¯aslargeaspossible.QUESTION6.5Onepossibilityistocollectdataonannualearningsforasampleofactors,alongwithprofitabilityofthemoviesinwhichtheyeachappeared.Inasimpleregressionanaly-sis,wecouldrelateearningstoprofitability.Butweshouldprobablycontrolforotherfactorsthatmayaffectsalary,suchasage,gender,andthekindsofmoviesinwhichtheactorsperformed.MethodsforincludingqualitativefactorsinregressionmodelsareconsideredinChapter7.CHAPTER7QUESTION7.1No,becauseitwouldnotbeclearwhenpartyisoneandwhenitiszero.AbetternamewouldbesomethinglikeDem,whichisoneforDemocraticcandidates,andzeroforRepublicans.Or,Rep,whichisoneforRepublicans,andzeroforDemocrats.QUESTION7.2Withoutfieldasthebasegroup,wewouldincludethedummyvariablesfrstbase,scndbase,thrdbase,shrtstop,andcatcher.QUESTION7.3ThenullinthiscaseisH0:12340,sothattherearefourrestrictions.Asusual,wewoulduseanFtest(whereq4andkdependsonthenumberofotherexplanatoryvariables).QUESTION7.4Becausetenureappearsasaquadratic,weshouldallowseparatequadraticsformenandwomen.Thatis,wewouldaddtheexplanatoryvariablesfemaletenureand2femaletenure.QUESTION7.5Weplugpcnv0,avgsen0,tottime0,ptime860,qemp860,black1,andhispan0into(7.31):arrˆ86.380.038(4).170.398,oralmost.4.Itishardtoknowwhetherthisis“reasonable.”Forsomeonewithnopriorconvictionswhowas768\nxd7/14/999:34PMPage769AppendixFAnswerstoChapterQuestionsemployedthroughouttheyear,thisestimatemightseemhigh,butrememberthatthepopulationconsistsofmenwhowerealreadyarrestedatleastoncepriorto1986.CHAPTER8QUESTION8.1Thisstatementisclearlyfalse.Forexample,inequation(8.7),theusualstandarderrorforblackis.147,whiletheheteroskedasticity-robuststandarderroris.118.QUESTION8.22TheFtestwouldbeobtainedbyregressinguˆonmarrmale,marrfem,andsingfem(singmaleisthebasegroup).Withn526andthreeindependentvariablesinthisregression,thedfare3and522.QUESTION8.3Notreally.Becausethisisasimpleregressionmodel,heteroskedasticityonlymattersifitisrelatedtoinc.ButtheBreusch-Pagantestinthiscaseisequivalenttoatstatisticin2regressinguˆoninc.Atstatisticof.96isnotlargeenoughtorejectthehomoskedas-ticityassumption.QUESTION8.4Wecanuseweightedleastsquaresbutcomputetheheteroskedasticity-robuststandarderrors.Inequation(8.26),ifourvariancemodelisincorrect,westillhavehet-eroskedasticity.Thus,wecanmakeaguessattheformofheteroskedasticityandper-formWLS,butouranalysiscanbemaderobusttoincorrectformsofheteroskedasticity.Unfortunately,weprobablyhavetoexplicityobtainthetransformedvariables.CHAPTER9QUESTION9.12Thesearebinaryvariables,andsquaringthemhasnoeffect:blackblack,and2hispanhispan.QUESTION9.2WheneducIQisintheequation,thecoefficientoneduc,say1,measurestheeffectofeduconlog(wage)whenIQ0.(Thepartialeffectofeducationis19IQ.)ThereisnooneinthepopulationofinterestwithanIQclosetozero.Attheaveragepopula-tionIQ,whichis100,theestimatedreturntoeducationfromcolumn(3)is.018.00034(100).052,whichisalmostwhatweobtainasthecoefficientoneducincol-umn(2).QUESTION9.3No.Ifeduc*isaninteger—whichmeanssomeonehasnoeducationpastthepreviousgradecompleted—themeasurementerroriszero.Ifeduc*isnotaninteger,educ769\nxd7/14/999:34PMPage770AppendixFAnswerstoChapterQuestionseduc*,andsothemeasurementerrorisnegative.Ataminimum,e1cannothavezeromean,ande1andeduc*areprobablycorrelated.QUESTION9.4Anincumbent’sdecisionnottorunmaybesystematicallyrelatedtohowheorsheexpectstodointheelection.Therefore,wemayonlyhaveasampleofincumbentswhoarestronger,onaverage,thanallpossibleincumbentswhocouldrun.Thisresultsinasampleselectionproblemifthepopulationofinterestincludesallincumbents.Ifweareonlyinterestedintheeffectsofcampaignexpendituresonelectionoutcomesforincum-bentswhoseekreelection,thereisnosampleselectionproblem.CHAPTER10QUESTION10.1Theimpactpropensityis.48,whilethelong-runpropensityis.48.15.32.65.QUESTION10.2Theexplanatoryvariablesarext1ztandxt2zt1.Theabsenceofperfectcollinear-itymeansthatthesecannotbeconstant,andtherecannotbeanexactlinearrelationshipbetweentheminthesample.Thisrulesoutthepossibilitythatallthez1,…,zntakeonthesamevalueorthatthez0,z1,…,zn1takeonthesamevalue.Butiteliminatesotherpatternsaswell.Forexample,ifztabtforconstantsaandb,thenzt1ab(t1)(abt)bztb,whichisaperfectlinearfunctionofzt.QUESTION10.3If{zt}isslowlymovingovertime—asisthecaseforthelevelsorlogsofmanyeco-nomictimeseries—thenztandzt1canbehighlycorrelated.Forexample,thecorrela-tionbetweenunemtandunemt1inPHILLIPS.RAWis.74.QUESTION10.4No,becausealineartimetrendwith10becomesmoreandmorenegativeastgetslarge.Sincegfrcannotbenegative,alineartimetrendwithanegativetrendcoefficientcannotrepresentgfrinallfuturetimeperiods.QUESTION10.5TheinterceptforMarchis02.Seasonaldummyvariablesarestrictlyexogenousbecausetheyfollowadeterministicpattern.Forexample,themonthsdonotchangebaseduponwhethereithertheexplanatoryvariablesorthedependentvariablechange.CHAPTER11QUESTION11.1(i)No,becauseE(yt)01tdependsont.(ii)Yes,becauseytE(yt)etisani.i.d.sequence.770\nxd7/14/999:34PMPage771AppendixFAnswerstoChapterQuestionsQUESTION11.2eeWepluginft(1/2)inft1(1/2)inft2intoinftinft1(unemt0)etandrearrange:inft(1/2)(inft1inft2)01unemtet,where010,asbefore.Therefore,wewouldregressytonunemt,whereytinft(1/2)(inft1inft2).Notethatwelosethefirsttwoobservationsinconstructingyt.QUESTION11.3No,becauseutandut1arecorrelated.Inparticular,Cov(ut,ut1)E[(et221et1)(et11et2)]1E(et1)1e0if10.Iftheerrorsareseriallycorrelated,themodelcannotbedynamicallycomplete.CHAPTER12QUESTION12.1Weuseequation(12.4).Now,onlyadjacenttermsarecorrelated.Inparticular,the2covariancebetweenxtutandxt1ut1isxtxt1Cov(ut,ut1)xtxt1 e.Therefore,theformulaisnn122Var(ˆ1)SSTxxtVar(ut)2xtxt1E(utut1)t1t1n1222/SSTx(2/SSTx) extxt1t1n1222/SSTx e(2/SSTx)xtxt1t1222222whereVar(ut)e1ee(11).Unlessxtandxt1areuncorrelatedinthesample,thesecondtermisnonzerowhenever0.Noticethatifxtandxt1arepositivelycorrelatedand0,thetruevarianceisactuallysmallerthantheusualvari-ance.Whentheequationisinlevels(asopposedtobeingdifferenced),thetypicalcaseis0,withpositivecorrelationbetweenxtandxt1.QUESTION12.2ˆ1.96se(ˆ),wherese(ˆ)isthestandarderrorreportedintheregression.Or,wecouldusetheheteroskedasticity-robuststandarderror.ShowingthatthisisasymptoticallyvalidiscomplicatedbecausetheOLSresidualsdependonˆj,butitcanbedone.QUESTION12.3Themodelwehaveinmindisut1ut14ut4et,andwewanttotestH0:10,40againstthealternativethatH0isfalse.Wewouldruntheregressionofuˆtonuˆt1anduˆt4toobtaintheusualFstatisticforjointsignificanceofthetwolags.(Wearetestingtworestrictions.)QUESTION12.4Wewouldprobablyestimatetheequationusingfirstdifferences,asˆ.92iscloseenoughtoonetoraisequestionsaboutthelevelsregression.SeeChapter18formorediscussion.771\nxd7/14/999:34PMPage772AppendixFAnswerstoChapterQuestionsQUESTION12.5Becausethereisonlyoneexplanatoryvariable,theWhitetestiseasytocompute.22Simplyregressuˆtonreturnt1andreturnt1(withanintercept,asalways)andcom-2putetheFtestforjointsignificanceofreturnt1andreturnt1.Ifthesearejointlysig-nificantatasmallenoughsignificancelevel,werejectthenullofhomoskedasticity.CHAPTER13QUESTION13.1Yes,assumingthatwehavecontrolledforallrelevantfactors.Thecoefficientonblackis1.076,and,withastandarderrorof.174,itisnotstatisticallydifferentfromone.The95%confidenceintervalisfromabout.735to1.417.QUESTION13.2Thecoefficientonhighearnshowsthat,intheabsenceofanychangeintheearningscap,highearnersspendmuchmoretime—ontheorderof29.2%onaverage[becauseexp(.256)1.292]—onworkers’compensation.QUESTION13.3First,E(vi1)E(aiui1)E(ai)E(vi1)0.Similarly,E(vi2)0.Therefore,the2covariancebetweenvi1andvi2issimplyE(vi1vi2)E[(aiui1)(aiui2)]E(ai)2E(aiui1)E(aiui2)E(ui1ui2)E(ai),becauseallofthecovariancetermsarezeroby2assumption.ButE(ai)Var(ai),becauseE(ai)0.Thiscausespositiveserialcorre-lationacrosstimeintheerrorswithineachi,whichbiasestheusualOLSstandarderrorsinapooledcross-sectionalregression.QUESTION13.4Becauseadmnadmn90admn85isthedifferenceinbinaryindicators,itcanbe1ifandonlyifadmn900andadmn851.Inotherwords,Washingtonstatehadanadministrativeperselawin1985butitwasrepealedby1990.QUESTION13.5No,justasitdoesnotcausebiasandinconsistencyinatimeseriesregressionwithstrictlyexogenousexplanatoryvariables.Therearetworeasonsitisaconcern.First,serialcorrelationintheerrorsinanyequationgenerallybiasestheusualOLSstandarderrorsandteststatistics.Second,itmeansthatpooledOLSisnotasefficientasesti-matorsthataccountfortheserialcorrelation(asinChapter12).CHAPTER14QUESTION14.1Whetherweusefirstdifferencingorthewithintransformation,wewillhavetroubleestimatingthecoefficientonkidsit.Forexample,usingthewithintransformation,ifkidsitdoesnotvaryforfamilyi,thenki¨dsitkidsitkidsi0fort1,2,3.Aslongassomefamilieshavevariationinkidsit,thenwecancomputethefixedeffectsestima-772\nxd7/14/999:34PMPage773AppendixFAnswerstoChapterQuestionstor,butthekidscoefficientcouldbeveryimpreciselyestimated.Thisisaformofmul-ticollinearityinfixedeffectsestimation(orfirst-differencingestimation).QUESTION14.2Ifafirmdidnotreceiveagrantinthefirstyear,itmayormaynotreceiveagrantinthesecondyear.Butifafirmdidreceiveagrantinthefirstyear,itcouldnotgetagrantinthesecondyear.Thatis,ifgrant11,thengrant0.Thisinducesanegativecor-relationbetweengrantandgrant1.Wecanverifythisbycomputingaregressionofgrantongrant1,usingthedatainJTRAIN.RAWfor1989.Usingallfirmsinthesam-ple,wegetgraˆnt(.248)(.248)grant1.graˆnt(.035)(.072)grant1.2n157,R.070.Thecoefficientongrant1mustbethenegativeoftheintercept,becausegraˆnt0whengrant11.QUESTION14.3Itsuggeststhattheunobservedeffectaiispositivelycorrelatedwithunionit.Remember,pooledOLSleavesaiintheerrorterm,whilefixedeffectsremovesai.Bydefinition,aihasapositiveeffectonlog(wage).Bythestandardomittedvariablesanalysis(seeChapter3),OLShasanupwardbiaswhentheexplanatoryvariable(union)ispositivelycorrelatedwiththeomittedvariable(ai).Thus,belongingtoaunionappearstobepos-itivelyrelatedtotime-constant,unobservedfactorsthataffectwage.QUESTION14.4Notifallsisterswithinafamilyhavethesamemotherandfather.Then,becausetheparents’racevariableswouldnotchangebysister,theywouldbedifferencedawayin(14.13).CHAPTER15QUESTION15.1Probablynot.Inthesimpleequation(15.18),yearsofeducationispartoftheerrorterm.Ifsomemenwhowereassignedlowdraftlotterynumbersobtainedadditionalschool-ing,thenlotterynumberandeducationarenegativelycorrelated,whichviolatesthefirstrequirementforaninstrumentalvariableinequation(15.4).QUESTION15.2(i)For(15.27),werequirethathighschoolpeergroupeffectscarryovertocollege.Namely,foragivenSATscore,astudentwhowenttoahighschoolwheresmokingmarijuanawasmorepopularwouldsmokemoremarijuanaincollege.Eveniftheiden-tificationcondition(15.27)holds,thelinkmightbeweak.(ii)Wehavetoassumethatpercentofstudentsusingmarijuanaatastudent’shighschoolisnotcorrelatedwithunobservedfactorsthataffectcollegegradepointaverage.773\nxd7/14/999:34PMPage774AppendixFAnswerstoChapterQuestionsWhilewearesomewhatcontrollingforhighschoolqualitybyincludingSATintheequation,thismightnotbeenough.Perhapshighschoolsthatdidabetterjobofprepar-ingstudentsforcollegealsohadfewerstudentssmokingmarijuana.Or,marijuanausagecouldbecorrelatedwithaverageincomelevels.Theseare,ofcourse,empiricalquestionsthatwemayormaynotbeabletoanswer.QUESTION15.3WhileprevalenceoftheNRAandsubscriberstogunmagazinesareprobablycorrelatedwiththepresenceofguncontrollegislation,itisnotobviousthattheyareuncorrelatedwithunobservedfactorsthataffecttheviolentcrimerate.Infact,wemightarguethatapopulationinterestedingunsisareflectionofhighcrimerates,andcontrollingforeconomicanddemographicvariablesisnotsufficienttocapturethis.Itwouldbehardtoarguepersuasivelythatthesearetrulyexogenousintheviolentcrimeequation.QUESTION15.4Asusual,therearetworequirements.First,itshouldbethecasethatgrowthingovern-mentspendingissystematicallyrelatedtothepartyofthepresident,afternettingouttheinvestmentrateandgrowthinthelaborforce.Inotherwords,theinstrumentmustbepartiallycorrelatedwiththeendogenousexplanatoryvariable.WhilewemightthinkthatgovernmentspendinggrowsmoreslowlyunderRepublicanpresidents,thiscer-tainlyhasnotalwaysbeentrueintheUnitedStatesandwouldhavetobetestedusingthetstatisticonREPt1inthereducedformgGOVt01REPt12INVRATt3gLABtvt.WemustassumethatthepartyofthepresidenthasnoseparateeffectongGDP.Thiswouldbeviolatedif,forexample,monetarypolicydifferssystematicallybypresidentialpartyandhasaseparateeffectonGDPgrowth.CHAPTER16QUESTION16.1Probablynot.Itisbecausefirmschoosepriceandadvertisingexpendituresjointlythatwearenotinterestedintheexperimentwhere,say,advertisingchangesexogenouslyandwewanttoknowtheeffectonprice.Instead,wewouldmodelpriceandadvertis-ingeachasafunctionofdemandandcostvariables.Thisiswhatfallsoutoftheeco-nomictheory.QUESTION16.2Wemustassumetwothings.First,moneysupplygrowthshouldappearinequation(16.22),sothatitispartiallycorrelatedwithinf.Second,wemustassumethatmoneysupplygrowthdoesnotappearinequation(16.23).Ifwethinkwemustincludemoneysupplygrowthinequation(16.23),thenwearestillshortaninstrumentforinf.Ofcourse,theassumptionthatmoneysupplygrowthisexogenouscanalsobequestioned.QUESTION16.3UsetheHausmantestfromChapter15.Inparticular,letvˆ2betheOLSresidualsfromthereducedformregressionofopenonlog(pcinc)andlog(land).Then,useanOLS774\nxd7/14/999:34PMPage775AppendixFAnswerstoChapterQuestionsregressionofinfonopen,log(pcinc),andvˆ2andcomputethetstatisticforsignificanceofvˆ2.Ifvˆ2issignificant,the2SLSandOLSestimatesarestatisticallydifferent.QUESTION16.4Thedemandequationlookslikelog(fisht)01log(prcfisht)2log(inct)3log(prcchickt)4log(prcbeeft)ut1,wherelogarithmsareusedsothatallelasticitiesareconstant.Byassumption,thedemandfunctioncontainsnoseasonality,sotheequationdoesnotcontainmonthlydummyvariables(sayfebt,mart,…,dect,withJanuaryasthebasemonth).Also,byassumption,thesupplyoffishisseasonal,whichmeansthatthesupplyfunctiondoesdependonatleastsomeofthemonthlydummyvariables.Evenwithoutsolvingthereducedformforlog(prcfish),weconcludethatitdependsonthemonthlydummyvari-ables.Sincetheseareexogenous,theycanbeusedasinstrumentsforlog(prcfish)inthedemandequation.Therefore,wecanestimatethedemand-for-fishequationusingmonthlydummiesastheIVsforlog(prcfish).Identificationrequiresthatatleastonemonthlydummyvariableappearswithanonzerocoefficientinthereducedformforlog(prcfish).CHAPTER17QUESTION17.1H0:4560,sothattherearethreerestrictionsandthereforethreedfintheLRorWaldtest.QUESTION17.22Weneedthepartialderivativeof(ˆ0ˆ1nwifeincˆ2educˆ3experˆ4exper…)withrespecttoexper,whichis()(ˆ32ˆ4exper),where()isevaluatedatthegivenvaluesandtheinitiallevelofexperience.Therefore,weneedtoevaluatethestandardnormalprobabilitydensityat.270.012(20.13).131(12.3).123(10)2.0019(10).053(42.5).868(0).036(1).463,whereweplugintheinitiallevel1/22ofexperience(10).But(.463)(2)exp[(.463)/2].358.Next,wemultiplythisbyˆ32ˆ4exper,whichisevaluatedatexper10.Thepartialeffectusingthecalculusapproximationis.358[.1232(.0019)(10)].030.Inotherwords,atthegivenvaluesoftheexplanatoryvariablesandstartingatexper10,thenextyearofexperienceincreasestheprobabilityoflaborforceparticipationbyabout.03.QUESTION17.3No.Thenumberofextramaritalaffairsisanonnegativeinteger,whichpresumablytakesonzeroorsmallnumbersforasubstantialfractionofthepopulation.Itisnotreal-istictouseaTobitmodel,which,whileallowingapileupatzero,treatsyasbeingcon-tinuouslydistributedoverpositivevalues.Formally,assumingthatymax(0,y*),wherey*isnormallydistributed,isatoddswiththediscretenessofthenumberofextra-maritalaffairswheny0.775\nxd7/14/999:34PMPage776AppendixFAnswerstoChapterQuestionsQUESTION17.4TheadjustedstandarderrorsaretheusualPoissonMLEstandarderrorsmultipliedbyˆ21.41,sotheadjustedstandarderrorswillbeabout41%higher.Thequasi-2LRstatisticistheusualLRstatisticdividedbyˆ,soitwillbeone-halfoftheusualLRstatistic.QUESTION17.5Byassumption,mvpi0xiui,where,asusual,xidenotesalinearfunctionoftheexogenousvariables.Now,observedwageisthelargestoftheminimumwageandthemarginalvalueproduct,sowageimax(minwagei,mvpi),whichisverysimilartoequation(17.34),exceptthatthemaxoperatorhasreplacedtheminoperator.CHAPTER18QUESTION18.1Wecanplugthesevaluesdirectlyintoequation(18.1)andtakeexpectations.First,becausezs0,foralls0,y1u1.Then,z01,soy00u0.Forh1,yhh1huh.Becausetheerrorshavezeroexpectedvalues,E(y1),E(y0)0,andE(yh)h1h,forallh1.Ash*,h*0.ItfollowsthatE(yh)*ash*,thatis,theexpectedvalueofyhreturnstotheexpectedvaluebeforetheincreaseinz,attimezero.Thismakessense:whiletheincreaseinzlastedfortwoperiods,itisstillatemporaryincrease.QUESTION18.2Underthedescribedsetup,ytandxtarei.i.d.sequencesthatareindependentofoneanother.Inparticular,ytandxtareuncorrelated.Ifˆ1istheslopecoefficientfromregressingytonxt,t1,2,…,n,thenplimˆ10.Thisisasitshouldbe,asweareregressingoneI(0)processonanotherI(0)process,andtheyareuncorrelated.Wewritetheequationyt01xtet,where010.Because{et}isindependentof{xt},thestrictexogeneityassumptionholds.Moreover,{et}isseriallyuncorrelatedandhomoskedastic.ByTheorem11.2inChapter11,thetstatisticforˆ1hasanapprox-imatestandardnormaldistribution.Ifetisnormallydistributed,theclassicallinearmodelassumptionshold,andthetstatistichasanexacttdistribution.QUESTION18.3Writextxt1at,where{at}isI(0).Byassumption,thereisalinearcombination,saystytxt,whichisI(0).Now,ytxt1yt(xtat)stat.BecausestandatareI(0)byassumption,soisstat.QUESTION18.4JustusethesumofsquaredresidualsformoftheFtestandassumehomoskedasticity.TherestrictedSSRisobtainedbyregressinghy6thy3t1(hy6t1hy3t2)onaconstant.Noticethat0istheonlyparametertoestimateinhy6t00hy3t1(hy6t1hy3t2)whentherestrictionsareimposed.Theunrestrictedsumofsquaredresidualsisobtainedfromequation(18.39).776\nxd7/14/999:34PMPage777AppendixFAnswerstoChapterQuestionsQUESTION18.5Wearefittingtwoequations:yˆtˆˆtandyˆtˆˆyeart.Wecanobtaintherela-tionshipbetweentheparametersbynotingthatyeartt49.Pluggingthisintothesecondequationgivesyˆtˆˆ(t49)(ˆ49ˆ)ˆt.Matchingtheslopeandinterceptwiththefirstequationgivesˆˆ—sothattheslopesontandyeartareiden-tical—andˆˆ49ˆ.Generally,whenweuseyearratherthant,theinterceptwillchange,buttheslopewillnot.(Youcanverifythisbyusingoneofthetimeseriesdatasets,suchasHSEINV.RAWorINVEN.RAW.)Whetherweusetorsomemeasureofyeardoesnotchangefittedvalues,and,naturally,itdoesnotchangeforecastsoffuturevalues.Theinterceptsimplyadjustsappropriatelytodifferentwaysofincludingatrendintheregression.777\n\nxd7/14/999:37PMPage778AppendixGStatisticalTablesTABLEG.1CumulativeAreasUndertheStandardNormalDistributionz01234567893.00.00130.00130.00130.00120.00120.00110.00110.00110.00100.00102.90.00190.00180.00180.00170.00160.00160.00150.00150.00140.00142.80.00260.00250.00240.00230.00230.00220.00210.00210.00200.00192.70.00350.00340.00330.00320.00310.00300.00290.00280.00270.00262.60.00470.00450.00440.00430.00410.00400.00390.00380.00370.00362.50.00620.00600.00590.00570.00550.00540.00520.00510.00490.00482.40.00820.00800.00780.00750.00730.00710.00690.00680.00660.00642.30.01070.01040.01020.00990.00960.00940.00910.00890.00870.00842.20.01390.01360.01320.01290.01250.01220.01190.01160.01130.01102.10.01790.01740.01700.01660.01620.01580.01540.01500.01460.01432.00.02280.02220.02170.02120.02070.02020.01970.01920.01880.01831.90.02870.02810.02740.02680.02620.02560.02500.02440.02390.02331.80.03590.03510.03440.03360.03290.03220.03140.03070.03010.02941.70.04460.04360.04270.04180.04090.04010.03920.03840.03750.03671.60.05480.05370.05260.05160.05050.04950.04850.04750.04650.04551.50.06680.06550.06430.06300.06180.06060.05940.05820.05710.05591.40.08080.07930.07780.07640.07490.07350.07210.07080.06940.06811.30.09680.09510.09340.09180.09010.08850.08690.08530.08380.08231.20.11510.11310.11120.10930.10750.10560.10380.10200.10030.09851.10.13570.13350.13140.12920.12710.12510.12300.12100.11900.11701.00.15870.15620.15390.15150.14920.14690.14460.14230.14010.13790.90.18410.18140.17880.17620.17360.17110.16850.16600.16350.16110.80.21190.20900.20610.20330.20050.19770.19490.19220.18940.18670.70.24200.23890.23580.23270.22960.22660.22360.22060.21770.2148continued778\nxd7/14/999:37PMPage779AppendixGStatisticalTablesTABLEG.1(concluded)z01234567890.60.27430.27090.26760.26430.26110.25780.25460.25140.24830.24510.50.30850.30500.30150.29810.29460.29120.28770.28430.28100.27760.40.34460.34090.33720.33360.33000.32640.32280.31920.31560.31210.30.38210.37830.37450.37070.36690.36320.35940.35570.35200.34830.20.42070.41680.41290.40900.40520.40130.39740.39360.38970.38590.10.46020.45620.45220.44830.44430.44040.43640.43250.42860.42470.00.50000.49600.49200.48800.48400.48010.47610.47210.46810.46410.00.50000.50400.50800.51200.51600.51990.52390.52790.53190.53590.10.53980.54380.54780.55170.55570.55960.56360.56750.57140.57530.20.57930.58320.58710.59100.59480.59870.60260.60640.61030.61410.30.61790.62170.62550.62930.63310.63680.64060.64430.64800.65170.40.65540.65910.66280.66640.67000.67360.67720.68080.68440.68790.50.69150.69500.69850.70190.70540.70880.71230.71570.71900.72240.60.72570.72910.73240.73570.73890.74220.74540.74860.75170.75490.70.75800.76110.76420.76730.77040.77340.77640.77940.78230.78520.80.78810.79100.79390.79670.79950.80230.80510.80780.81060.81330.90.81590.81860.82120.82380.82640.82890.83150.83400.83650.83891.00.84130.84380.84610.84850.85080.85310.85540.85770.85990.86211.10.86430.86650.86860.87080.87290.87490.87700.87900.88100.88301.20.88490.88690.88880.89070.89250.89440.89620.89800.89970.90151.30.90320.90490.90660.90820.90990.91150.91310.91470.91620.91771.40.91920.92070.92220.92360.92510.92650.92790.92920.93060.93191.50.93320.93450.93570.93700.93820.93940.94060.94180.94290.94411.60.94520.94630.94740.94840.94950.95050.95150.95250.95350.95451.70.95540.95640.95730.95820.95910.95990.96080.96160.96250.96331.80.96410.96490.96560.96640.96710.96780.96860.96930.96990.97061.90.97130.97190.97260.97320.97380.97440.97500.97560.97610.97672.00.97720.97780.97830.97880.97930.97980.98030.98080.98120.98172.10.98210.98260.98300.98340.98380.98420.98460.98500.98540.98572.20.98610.98640.98680.98710.98750.98780.98810.98840.98870.98902.30.98930.98960.98980.99010.99040.99060.99090.99110.99130.99162.40.99180.99200.99220.99250.99270.99290.99310.99320.99340.99362.50.99380.99400.99410.99430.99450.99460.99480.99490.99510.99522.60.99530.99550.99560.99570.99590.99600.99610.99620.99630.99642.70.99650.99660.99670.99680.99690.99700.99710.99720.99730.99742.80.99740.99750.99760.99770.99770.99780.99790.99790.99800.99812.90.99810.99820.99820.99830.99840.99840.99850.99850.99860.99863.00.99870.99870.99870.99880.99880.99890.99890.99890.99900.9990Examples:IfZ~Normal(0,1)thenP(Z1.32).0934andP(Z1.84).9671.®Source:ThistablewasgeneratedusingtheStatafunctionnormd.779\nxd7/14/999:37PMPage780AppendixGStatisticalTablesTABLEG.2CriticalValuesofthetDistributionSignificanceLevel1-Tailed:.10.05.025.01.0052-Tailed:.20.10.050.02.01013.0786.31412.70631.82163.65721.8862.9204.3036.9659.92531.6382.3533.1824.5415.84141.5332.1322.7763.7474.60451.4762.0152.5713.3654.03261.4401.9432.4473.1433.70771.4151.8952.3652.9983.499D81.3971.8602.3062.8963.355e91.3831.8332.2622.8213.250g101.3721.8122.2282.7643.169re111.3631.7962.2012.7183.106121.3561.7822.1792.6813.055e131.3501.7712.1602.6503.012s141.3451.7612.1452.6242.977151.3411.7532.1312.6022.947of161.3371.7462.1202.5832.921171.3331.7402.1102.5672.898F181.3301.7342.1012.5522.878r191.3281.7292.0932.5392.861e201.3251.7252.0862.5282.845e211.3231.7212.0802.5182.831d221.3211.7172.0742.5082.819o231.3191.7142.0692.5002.807m241.3181.7112.0642.4922.797251.3161.7082.0602.4852.787261.3151.7062.0562.4792.779271.3141.7032.0522.4732.771281.3131.7012.0482.4672.763291.3111.6992.0452.4622.756301.3101.6972.0422.4572.750401.3031.6842.0212.4232.704601.2961.6712.0002.3902.660901.2911.6621.9872.3682.6321201.2891.6581.9802.3582.6171.2821.6451.9602.3262.576Examples:The1%criticalvalueforaone-tailedtestwith25dfis2.485.The5%criticalforatwo-tailedtestwithlarge(120)dfis1.96.®Source:ThistablewasgeneratedusingtheStatafunctioninvt.780\nxd7/14/999:37PMPage781AppendixGStatisticalTablesTABLEG.3a10%CriticalValuesoftheFDistributionNumeratorDegreesofFreedom12345678910103.292.922.732.612.522.462.412.382.352.32D113.232.862.662.542.452.392.342.302.272.25e123.182.812.612.482.392.332.282.242.212.19n133.142.762.562.432.352.282.232.202.162.14o143.102.732.522.392.312.242.192.152.122.10mi153.072.702.492.362.272.212.162.122.092.06na163.052.672.462.332.242.182.132.092.062.03t173.032.642.442.312.222.152.102.062.032.00o183.012.622.422.292.202.132.082.042.001.98r192.992.612.402.272.182.112.062.021.981.96D202.972.592.382.252.162.092.042.001.961.94eg212.962.572.362.232.142.082.021.981.951.92r222.952.562.352.222.132.062.011.971.931.90e232.942.552.342.212.112.051.991.951.921.89e242.932.542.332.192.102.041.981.941.911.88s252.922.532.322.182.092.021.971.931.891.87of262.912.522.312.172.082.011.961.921.881.86272.902.512.302.172.072.001.951.911.871.85F282.892.502.292.162.062.001.941.901.871.84r292.892.502.282.152.061.991.931.891.861.83ee302.882.492.282.142.051.981.931.881.851.82do402.842.442.232.092.001.931.871.831.791.76m602.792.392.182.041.951.871.821.771.741.71902.762.362.152.011.911.841.781.741.701.671202.752.352.131.991.901.821.771.721.681.652.712.302.081.941.851.771.721.671.631.60Example:The10%criticalvaluefornumeratordf2anddenominatordf40is2.44.®Source:ThistablewasgeneratedusingtheStatafunctioninvfprob.781\nxd7/14/999:37PMPage782AppendixGStatisticalTablesTABLEG.3b5%CriticalValuesoftheFDistributionNumeratorDegreesofFreedom12345678910104.964.103.713.483.333.223.143.073.022.98114.843.983.593.363.203.093.012.952.902.85De124.753.893.493.263.113.002.912.852.802.75n134.673.813.413.183.032.922.832.772.712.67o144.603.743.343.112.962.852.762.702.652.60mi154.543.683.293.062.902.792.712.642.592.54n164.493.633.243.012.852.742.662.592.542.49at174.453.593.202.962.812.702.612.552.492.45o184.413.553.162.932.772.662.582.512.462.41r194.383.523.132.902.742.632.542.482.422.38D204.353.493.102.872.712.602.512.452.392.35e214.323.473.072.842.682.572.492.422.372.32gr224.303.443.052.822.662.552.462.402.342.30e234.283.423.032.802.642.532.442.372.322.27e244.263.403.012.782.622.512.422.362.302.25s254.243.392.992.762.602.492.402.342.282.24o264.233.372.982.742.592.472.392.322.272.22f274.213.352.962.732.572.462.372.312.252.20F284.203.342.952.712.562.452.362.292.242.19r294.183.332.932.702.552.432.352.282.222.18ee304.173.322.922.692.532.422.332.272.212.16d404.083.232.842.612.452.342.252.182.122.08om604.003.152.762.532.372.252.172.102.041.99903.953.102.712.472.322.202.112.041.991.941203.923.072.682.452.292.172.092.021.961.913.843.002.602.372.212.102.011.941.881.83Example:The5%criticalvaluefornumeratordf4andlargedenominatordf()is2.37.®Source:ThistablewasgeneratedusingtheStatafunctioninvfprob.782\nxd7/14/999:37PMPage783AppendixGStatisticalTablesTABLEG.3c1%CriticalValuesoftheFDistributionNumeratorDegreesofFreedom123456789101010.047.566.555.995.645.395.205.064.944.85119.657.216.225.675.325.074.894.744.634.54D129.336.935.955.415.064.824.644.504.394.30e139.076.705.745.214.864.624.444.304.194.10no148.866.515.565.044.694.464.284.144.033.94m158.686.365.424.894.564.324.144.003.893.80in168.536.235.294.774.444.204.033.893.783.69a178.406.115.184.674.344.103.933.793.683.59t188.296.015.094.584.254.013.843.713.603.51or198.185.935.014.504.173.943.773.633.523.43208.105.854.944.434.103.873.703.563.463.37De218.025.784.874.374.043.813.643.513.403.31g227.955.724.824.313.993.763.593.453.353.26r237.885.664.764.263.943.713.543.413.303.21ee247.825.614.724.223.903.673.503.363.263.17s257.775.574.684.183.853.633.463.323.223.13o267.725.534.644.143.823.593.423.293.183.09f277.685.494.604.113.783.563.393.263.153.06287.645.454.574.073.753.533.363.233.123.03Fr297.605.424.544.043.733.503.333.203.093.00e307.565.394.514.023.703.473.303.173.072.98ed407.315.184.313.833.513.293.122.992.892.80o607.084.984.133.653.343.122.952.822.722.63m906.934.854.013.543.233.012.842.722.612.521206.854.793.953.483.172.962.792.662.562.476.634.613.783.323.022.802.642.512.412.32Example:The1%criticalvaluefornumeratordf3anddenominatordf60is4.13.®Source:ThistablewasgeneratedusingtheStatafunctioninvfprob.783\nxd7/14/999:37PMPage784AppendixGStatisticalTablesTABLEG.4CriticalValuesoftheChi-SquareDistributionSignificanceLevel.10.05.0112.713.846.6324.615.999.2136.257.8111.3447.789.4913.2859.2411.0715.09610.6412.5916.81712.0214.0718.48813.3615.5120.09De914.6816.9221.67g1015.9918.3123.21re1117.2819.6824.72e1218.5521.0326.22s1319.8122.3627.691421.0623.6829.14o1522.3125.0030.58f1623.5426.3032.00F1724.7727.5933.41re1825.9928.8734.81e1927.2030.1436.19d2028.4131.4137.57om2129.6232.6738.932230.8133.9240.292332.0135.1741.642433.2036.4242.982534.3837.6544.312635.5638.8945.642736.7440.1146.962837.9241.3448.282939.0942.5649.593040.2643.7750.89Example:The5%criticalvaluewithdf8is15.51.®functionSource:ThistablewasgeneratedusingtheStatainvchi.784\n\nxd7/14/999:40PMPage785REFERENCESAngrist,J.D.(1990),“LifetimeEarningsandtheVietnamJ.S.Long,292–324.NewburyPark,CA:SageEraDraftLottery:EvidencefromSocialSecurityPublications.AdministrativeRecords,”AmericanEconomicReviewBetts,J.R.(1995),“DoesSchoolQualityMatter?80,313–336.EvidencefromtheNationalLongitudinalSurveyofAngrist,J.D.,andA.B.Krueger(1991),“DoesYouth,”ReviewofEconomicsandStatistics77,CompulsorySchoolAttendanceAffectSchoolingand231–250.Earnings?”QuarterlyJournalofEconomics106,Biddle,J.E.,andD.S.Hamermesh(1990),“Sleepandthe979–1014.AllocationofTime,”JournalofPoliticalEconomy98,Ashenfelter,O.,andA.B.Krueger(1994),“Estimatesof922–943.theEconomicReturntoSchoolingfromaNewSampleBiddle,J.E.,andD.S.Hamermesh(1998),“Beauty,ofTwins,”AmericanEconomicReview84,1157–1173.Productivity,andDiscrimination:Lawyers’LooksandAverett,S.,andS.Korenman(1996),“TheEconomicLucre,”JournalofLaborEconomics16,172–201.RealityoftheBeautyMyth,”JournalofHumanBlackburn,M.,andS.Korenman(1994),“TheDecliningResources31,304–330.Marital-StatusEarningsDifferential,”JournalofAyers,I.,andS.D.Levitt(1998),“MeasuringPositivePopulationEconomics7,247–270.ExternalitiesfromUnobservableVictimPrecaution:Blackburn,M.,andD.Neumark(1992),“UnobservedAnEmpiricalAnalysisofLojack,”QuarterlyJournalAbility,EfficiencyWages,andInterindustryWageofEconomics108,43–77.Differentials,”QuarterlyJournalofEconomics107,Banerjee,A.,J.Dolado,J.W.Galbraith,andD.F.Hendry1421–1436.(1993),Co-Integration,Error-Correction,andtheBlömstrom,M.,R.E.Lipsey,andM.Zejan(1996),“IsEconometricAnalysisofNon-StationaryData.FixedInvestmenttheKeytoEconomicGrowth?”Oxford:OxfordUniversityPress.QuarterlyJournalofEconomics111,269–276.Bartik,T.J.(1991),“TheEffectsofPropertyTaxesandBollerslev,T.,R.Y.Chou,andK.F.Kroner(1992),OtherLocalPublicPoliciesontheIntrametropolitan“ARCHModelinginFinance:AReviewoftheTheoryPatternofBusinessLocation,”inIndustryLocationandEmpiricalEvidence,”JournalofEconometrics52,andPublicPolicy.Ed.H.W.HerzogandA.M.5–59.Schlottmann,57–80.Knoxville:UniversityofTen-Bollerslev,T.,R.F.Engle,andD.B.Nelson(1994),nesseePress.“ARCHModels,”Chapter49inHandbookofBecker,G.S.(1968),“CrimeandPunishment:AnEconometrics,Volume4.Ed.R.F.EngleandEconomicApproach,”JournalofPoliticalEconomyD.L.McFadden,2959–3038.Amsterdam:North-76,169–217.Holland.Belsley,D.,E.Kuh,andR.Welsch(1980),RegressionBound,J.,D.A.Jaeger,andR.M.Baker(1995),Diagnostics:IdentifyingInfluentialDataandSources“ProblemswithInstrumentalVariablesEstimationofCollinearity.NewYork:Wiley.WhentheCorrelationBetweentheInstrumentsandBerk,R.A.(1990),“APrimeronRobustRegression,”inEndogenousExplanatoryVariablesisWeak,”JournalModernMethodsofDataAnalysis.Ed.J.FoxandoftheAmericanStatisticalAssociation90,443–450.785\nxd7/14/999:40PMPage786ReferencesBreusch,T.S.,andA.R.Pagan(1979),“ASimpleTestforDavidson,R.,andJ.G.MacKinnon(1981),“SeveralTestsHeteroskedasticityandRandomCoefficientVariation,”ofModelSpecificationinthePresenceofAlternativeEconometrica50,987–1007.Hypotheses,”Econometrica49,781–793.Cameron,A.C.,andP.K.Trivedi(1998),RegressionDavidson,R.,andJ.G.MacKinnon(1993),EstimationAnalysisofCountData.Cambridge:CambridgeandInferenceinEconometrics.NewYork:OxfordUniversityPress.UniversityPress.Campbell,J.Y.,andN.G.Mankiw(1990),“PermanentDeLong,J.B.,andL.H.Summers(1991),“EquipmentIncome,CurrentIncome,andConsumption,”JournalInvestmentandEconomicGrowth,”QuarterlyJournalofBusinessandEconomicStatistics8,265–279.ofEconomics106,445–502.Card,D.(1995),“UsingGeographicVariationinCollegeDickey,D.A.,andW.A.Fuller(1979),“DistributionsofProximitytoEstimatetheReturntoSchooling,”intheEstimatorsforAutoregressiveTimeSerieswithaAspectsofLabourMarketBehavior:EssaysinHonourUnitRoot,”JournaloftheAmericanStatisticalofJohnVanderkamp.Ed.L.N.Christophides,E.K.Association74,427–431.Grant,andR.Swidinsky,201–222.Toronto:Diebold,F.X.(1998),ElementsofForecasting.Cin-UniversityofTorontoPress.cinnati,OH:South-Western.Card,D.,andA.Krueger(1992),“DoesSchoolQualityDownes,T.A.,andS.M.Greenstein(1996),“Under-Matter?ReturnstoEducationandtheCharacteristicsstandingtheSupplyDecisionsofNonprofits:ofPublicSchoolsintheUnitedStates,”JournalofModelingtheLocationofPrivateSchools,”RandPoliticalEconomy100,1–40.JournalofEconomics27,365–390.Castillo-Freeman,A.J.,andR.B.Freeman(1992),Draper,N.,andH.Smith(1981),AppliedRegression“WhentheMinimumWageReallyBites:TheEffectAnalysis.2ded.NewYork:Wiley.oftheU.S.-LevelMinimumonPuertoRico,”inDurbin,J.(1970),“TestingforSerialCorrelationinLeastImmigrationandtheWorkForce.Ed.G.J.BorjasandSquaresRegressionsWhenSomeoftheRegressorsareR.B.Freeman,177–211.Chicago:UniversityofLaggedDependentVariables,”Econometrica38,ChicagoPress.410–421.Clark,K.B.(1984),“UnionizationandFirmPerformance:Durbin,J.,andG.S.Watson(1950),“TestingforSerialTheImpactonProfits,Growth,andProductivity,”CorrelationinLeastSquaresRegressionsI,”Bio-AmericanEconomicReview74,893–919.metrika37,409–428.Cloninger,D.O.(1991),“LethalPoliceResponseasaEicker,F.(1967),“LimitTheoremsforRegressionswithCrimeDeterrent:57-CityStudySuggestsaDecreaseUnequalandDependentErrors,”ProceedingsoftheinCertainCrimes,”AmericanJournalofEconomicsFifthBerkeleySymposiumonMathematicalStatisticsandSociology50,59–69.andProbability1,59–82.Berkeley:UniversityofCloninger,D.O.,andL.C.Sartorius(1979),“CrimeCaliforniaPress.Rates,ClearanceRatesandEnforcementEffort:TheEide,E.(1994),EconomicsofCrime:DeterrenceandtheCaseofHouston,Texas,”AmericanJournalofRationalOffender.Amsterdam:NorthHolland.EconomicsandSociology38,389–402.Engle,R.F.(1982),“AutoregressiveConditionalHetero-Cochrane,J.H.(1997),“WhereistheMarketGoing?Un-skedasticitywithEstimatesoftheVarianceofUnitedcertainFactsandNovelTheories,”EconomicPerspec-KingdomInflation,”Econometrica50,987–1007.tives21,FederalReserveBankofChicago,3–37.Engle,R.F.,andC.W.J.Granger(1987),“Co-integrationCornwell,C.,andW.N.Trumbull(1994),“EstimatingtheandErrorCorrection:Representation,Estimation,andEconomicModelofCrimeUsingPanelData,”ReviewTesting,”Econometrica55,251–276.ofEconomicsandStatistics76,360–366.Evans,W.N.,andR.M.Schwab(1995),“FinishingHighCurrie,J.(1995),WelfareandtheWell-BeingofChildren.SchoolandStartingCollege:DoCatholicSchoolsChur,Switzerland:HarwoodAcademicPublishers.MakeaDifference?”QuarterlyJournalofEconomicsCurrie,J.,andN.Cole(1993),“WelfareandChildHealth:110,941–974.TheLinkBetweenAFDCParticipationandBirthFair,R.C.(1996),“EconometricsandPresidentialElec-Weight,”AmericanEconomicReview83,971–983.tions,”JournalofEconomicPerspectives10,89–102.Currie,J.,andD.Thomas(1995),“DoesHeadStartMakeFriedman,B.M.,andK.N.Kuttner(1992),“Money,aDifference?”AmericanEconomicReview85,Income,Prices,andInterestRates,”AmericanEco-341–364.nomicReview82,472–492.786\nxd7/14/999:40PMPage787ReferencesGaren,J.E.(1994),“ExecutiveCompensationandHolzer,H.(1991),“TheSpatialMismatchHypothesis:Principal-AgentTheory,”JournalofPoliticalEconomyWhatHastheEvidenceShown?”UrbanStudies28,102,175–1199.105–122.Geronimus,A.T.,andS.Korenman(1992),“TheHolzer,H.,R.Block,M.Cheatham,andJ.Knott(1993),SocioeconomicConsequencesofTeenChildbearing“AreTrainingSubsidiesEffective?TheMichiganReconsidered,”QuarterlyJournalofEconomics107,Experience,”IndustrialandLaborRelationsReview1187–1214.46,625–636.Goldberger,A.S.(1991),ACourseinEconometrics.Hoxby,C.M.(1994),“DoPrivateSchoolsProvideCambridge,MA:HarvardUniversityPress.CompetitionforPublicSchools?”NationalBureauofGranger,C.W.J.,andP.Newbold(1974),“SpuriousEconomicResearchWorkingPaperNumber4978.RegressionsinEconometrics,”JournalofEcono-Huber,P.J.(1967),“TheBehaviorofMaximummetrics2,111–120.LikelihoodEstimatesUnderNonstandardConditions,”Greene,W.(1997),EconometricAnalysis.3rdedition.ProceedingsoftheFifthBerkeleySymposiumonNewYork:MacMillan.MathematicalStatisticsandProbability1,221–233.Griliches,Z.(1957),“SpecificationBiasinEstimatesofBerkeley:UniversityofCaliforniaPress.ProductionFunctions,”JournalofFarmEconomicsHunter,W.C.,andM.B.Walker(1996),“TheCultural39,8–20.AffinityHypothesisandMortgageLendingDeci-Grogger,J.(1990),“TheDeterrentEffectofCapitalsions,”JournalofRealEstateFinanceandEconomicsPunishment:AnAnalysisofDailyHomicideCounts,”13,57–70.JournaloftheAmericanStatisticalAssociation410,Hylleberg,S.(1991),ModellingSeasonality.Oxford:295–303.OxfordUniversityPress.Grogger,J.(1991),“Certaintyvs.SeverityofPunish-Kane,T.J.,andC.E.Rouse(1995),“Labor-Marketment,”EconomicInquiry29,297–309.ReturnstoTwo-andFour-YearColleges,”AmericanHall,R.J.(1988),“TheRelationBetweenPriceandEconomicReview85,600–614.MarginalCostinU.S.Industry,”JournalofPoliticalKiel,K.A.,andK.T.McClain(1995),“HousePricesEconomy96,921–948.DuringSitingDecisionStages:TheCaseofanInciner-Hamermesh,D.S.,andJ.E.Biddle(1994),“BeautyandatorfromRumorThroughOperation,”JournaloftheLaborMarket,”AmericanEconomicReview84,EnvironmentalEconomicsandManagement28,1174–1194.241–255.Hamilton,J.D.(1994),TimeSeriesAnalysis.Princeton,Kleck,G.,andE.B.Patterson(1993),“TheImpactofGunNJ:PrincetonUniversityPress.ControlOwnershipLevelsonViolenceRates,”JournalHanushek,E.(1986),“TheEconomicsofSchooling:ofQuantitativeCriminology9,249–287.ProductionandEfficiencyinPublicSchools,”JournalKoenker,R.(1981),“ANoteonStudentizingaTestforofEconomicLiterature,1141–1177.Heteroskedasticity,”JournalofEconometrics17,Harvey,A.(1990),TheEconometricAnalysisofEconomic107–112.TimeSeries.2ded.Cambridge,MA:MITPress.Korenman,S.,andD.Neumark(1991),“DoesMarriageHausman,J.A.(1978),“SpecificationTestsinEcono-ReallyMakeMenMoreProductive?”Journalofmetrics,”Econometrica46,1251–1271.HumanResources26,282–307.Hausman,J.A.,andD.A.Wise(1977),“SocialExperi-Korenman,S.,andD.Neumark(1992),“Marriage,mentation,TruncatedDistributions,andEfficientMotherhood,andWages,”JournalofHumanRe-Estimation,”Econometrica45,319–339.sources27,233–255.Herrnstein,R.J.,andC.Murray(1994),TheBellCurve:Krueger,A.B.(1993),“HowComputersHaveChangedtheIntelligenceandClassStructureinAmericanLife.WageStructure:EvidencefromMicrodata,1984–1989,”NewYork:FreePress.QuarterlyJournalofEconomics108,33–60.Hersch,J.,andL.S.Stratton(1997),“Housework,FixedKrupp,C.M.,andP.S.Pollard(1996),“MarketRe-Effects,andWagesofMarriedWorkers,”JournalofsponsestoAntidumpingLaws:SomeEvidencefromHumanResources32,285–307.theU.S.ChemicalIndustry,”CanadianJournalofHines,J.R.(1996),“AlteredStates:TaxesandtheEconomics29,199–227.LocationofForeignDirectInvestmentinAmerica,”Kwiatkowski,D.,P.C.B.Phillips,P.Schmidt,andAmericanEconomicReview86,1076–1094.Y.Shin(1992),“TestingtheNullHypothesisof787\nxd7/14/999:40PMPage788ReferencesStationarityAgainsttheAlternativeofaUnitRoot:RegressioninRationalDistributedLagModels,”HowSureAreWethatEconomicTimeSeriesHaveaEconomicsLetters48,235–240.UnitRoot?”JournalofEconometrics54,159–178.McCormick,R.E.,andM.Tinsley(1987),“Athleticsver-Larsen,R.J.,andM.L.Marx(1986),AnIntroductiontosusAcademics:EvidencefromSATScores,”JournalMathematicalStatisticsandItsApplications.2ndedi-ofPoliticalEconomy95,1103–1116.tion.EnglewoodCliffs,NJ:Prentice-Hall.McFadden,D.L.(1974),“ConditionalLogitAnalysisofLeamer,E.E.(1983),“Let’sTaketheConOutofQualitativeChoiceAnalysis,”inFrontiersinEconometrics,”AmericanEconomicReview73,Econometrics.Ed.P.Zarembka,105–142.NewYork:31–43.AcademicPress.Levine,P.B.,A.B.Trainor,andD.J.Zimmerman(1996),Meyer,B.D.(1995),“NaturalandQuasi-Experimentsin“TheEffectofMedicaidAbortionFundingRestric-Economics,”JournalofBusinessandEconomictionsonAbortions,Pregnancies,andBirths,”JournalStatistics13,151–161.ofHealthEconomics15,555–578.Meyer,B.D.,W.K.Viscusi,andD.L.Durbin(1995),Levine,P.B.,andD.J.Zimmerman(1995),“TheBenefit“Workers’CompensationandInjuryDuration:Evi-ofAdditionalHigh-SchoolMathandScienceClassesdencefromaNaturalExperiment,”AmericanEco-forYoungMenandWomen,”JournalofBusinessandnomicReview85,322–340.EconomicsStatistics13,137–149.Mizon,G.E.,andJ.F.Richard(1986),“TheLevitt,S.D.(1994),“UsingRepeatChallengerstoEncompassingPrincipleandItsApplicationtoTest-EstimatetheEffectofCampaignSpendingonElectioningNonnestedHypotheses,”Econometrica54,OutcomesintheU.S.House,”JournalofPolitical657–678.Economy102,777–798.Mroz,T.A.(1987),“TheSensitivityofanEmpiricalLevitt,S.D.(1996),“TheEffectofPrisonPopulationSizeModelofMarriedWomen’sHoursofWorktoonCrimeRates:EvidencefromPrisonOvercrowdingEconomicandStatisticalAssumptions,”EconometricaLegislation,”QuarterlyJournalofEconomics111,55,765–799.319–351.Mullahy,J.,andP.R.Portney(1990),“AirPollution,Low,S.A.,andL.R.McPheters(1983),“WageCigaretteSmoking,andtheProductionofRespiratoryDifferentialsandtheRiskofDeath:AnEmpiricalHealth,”JournalofHealthEconomics9,193–205.Analysis,”EconomicInquiry21,271–280.Mullahy,J.,andJ.L.Sindelar(1994),“DoDrinkersKnowLynch,L.M.(1992),“PrivateSectorTrainingandtheWhentoSayWhen?AnEmpiricalAnalysisofDrunkEarningsofYoungWorkers,”AmericanEconomicDriving,”EconomicInquiry32,383–394.Review82,299–312.Netzer,D.(1992),“DifferencesinRelianceonUserMacKinnon,J.G.,andH.White(1985),“SomeChargesbyAmericanStateandLocalGovernments,”HeteroskedasticityConsistentCovarianceMatrixPublicFinanceQuarterly20,499–511.EstimatorswithImprovedFiniteSampleProperties,”Neumark,D.,andW.Wascher(1995),“MinimumWageJournalofEconometrics29,305–325.EffectsonEmploymentandSchoolEnrollment,”Maddala,G.S.(1983),LimitedDependentandJournalofBusinessandEconomicStatistics13,QualitativeVariablesinEconometrics.Cambridge:199–206.CambridgeUniversityPress.Newey,W.K.,andK.D.West(1987),“ASimple,PositiveMaloney,M.T.,andR.E.McCormick(1993),“AnSemi-DefiniteHeteroskedasticityandAutocorrelationExaminationoftheRolethatIntercollegiateAthleticConsistentCovarianceMatrix,”Econometrica55,ParticipationPlaysinAcademicAchievement:Ath-703–708.letes’FeatsintheClassroom,”JournalofHumanRe-Papke,L.E.(1987),“SubnationalTaxationandCapitalsources28,555–570.Mobility:EstimatesofTax-PriceElasticities,”Na-Mankiw,N.G.(1994),Macroeconomics.2ded.NewtionalTaxJournal40,191–203.York:Worth.Papke,L.E.(1994),“TaxPolicyandUrbanDevelopment:McCarthy,P.S.(1994),“RelaxedSpeedLimitsandEvidencefromtheIndianaEnterpriseZoneProgram,”HighwaySafety:NewEvidenceFromCalifornia,”JournalofPublicEconomics54,37–49.EconomicsLetters46,173–179.Papke,L.E.(1995),“ParticipationinandContributionstoMcClain,K.T.,andJ.M.Wooldridge(1995),“ASimple401(k)PensionPlans:EvidencefromPlanData,”TestfortheConsistencyofDynamicLinearJournalofHumanResources30,311–325.788\nxd7/14/999:40PMPage789ReferencesPapke,L.E.(1999),“Are401(k)PlansReplacingOtherSolon,G.(1985),“TheMinimumWageandTeen-ageEmployer-ProvidedPensions?EvidencefromPanelEmployment:ARe-analysiswithAttentiontoSerialData,”JournalofHumanResources,34,346–368.CorrelationandSeasonality,”JournalofHumanPark,R.(1966),“EstimationwithHeteroskedasticErrorResources20,292–297.Terms,”Econometrica34,888.Stigler,S.M.(1986),TheHistoryofStatistics.Pavlik,E.L.,andA.Belkaoui(1991),DeterminantsofCambridge,MA:HarvardUniversityPress.ExecutiveCompensation.NewYork:QuorumBooks.Stock,J.H.,andM.W.Watson(1989),“InterpretingthePeek,J.(1982),“InterestRates,IncomeTaxes,andEvidenceonMoney-IncomeCausality,”JournalofAnticipatedInflation,”AmericanEconomicReview72,Econometrics40,161–181.980–991.Stock,J.H.,andM.W.Watson(1993),“ASimplePindyck,R.S.,andD.L.Rubinfeld(1992),Micro-EstimatorofCointegratingVectorsinHigherOrdereconomics.2ded.NewYork:MacMillan.IntegratedSystems,”Econometrica61,783–820.Ram,R.(1986),“GovernmentSizeandEconomicVella,F.,andM.Verbeek(1998),“WhoseWagesDoGrowth:ANewFrameworkandSomeEvidencefromUnionsRaise?ADynamicModelofUnionismandCross-SectionandTime-SeriesData,”AmericanWageRateDeterminationforYoungMen,”JournalofEconomicsReview76,191–203.AppliedEconometrics13,163–183.Ramanathan,R.(1995),IntroductoryEconometricswithWald,A.(1940),“TheFittingofStraightLinesifBothApplications.3ded.FortWorth:DrydenPress.VariablesAreSubjecttoError,”AnnalsofMathe-Ramey,V.(1991),“NonconvexCostsandtheBehaviorofmaticalStatistics11,284–300.Inventories,”JournalofPoliticalEconomy99,Wallis,K.F.(1972),“TestingforFourth-OrderAuto-306–334.correlationinQuarterlyRegressionEquations,”Ramsey,J.B.(1969),“TestsforSpecificationErrorsinEconometrica40,617–636.ClassicalLinearLeast-SquaresAnalysis,JournalofWhite,H.(1980),“AHeteroskedasticity-ConsistenttheRoyalStatisticalAssociation,SeriesB,71,CovarianceMatrixEstimatorandaDirectTestfor350–371.Heteroskedasticity,”Econometrica48,817–838.Romer,D.(1993),“OpennessandInflation:TheoryandWhite,H.(1984),AsymptoticTheoryforEcono-Evidence,”QuarterlyJournalofEconomics108,metricians.Orlando:AcademicPress.869–903.White,M.J.(1986),“PropertyTaxesandFirmLocation:Rose,N.L.(1985),“TheIncidenceofRegulatoryRentsinEvidencefromProposition13,”inStudiesinStateandtheMotorCarrierIndustry,”RandJournalofEco-LocalPublicFinance.Ed.H.S.Rosen,83–112.nomics16,299–318.Chicago:UniversityofChicagoPress.Rose,N.L.,andA.Shepard(1997),“FirmDiversificationWhittington,L.A.,J.Alm,andH.E.Peters(1990),andCEOCompensation:ManagerialAbilityor“FertilityandthePersonalExemption:ImplicitExecutiveEntrenchment?”RandJournalofEco-PronatalistPolicyintheUnitedStates,”Americannomics28,489–514.EconomicReview80,545–556.Rouse,C.E.(1998),“PrivateSchoolVouchersandWooldridge,J.M.(1989),“AComputationallySimpleStudentAchievement:AnEvaluationoftheHeteroskedasticityandSerialCorrelation-RobustMilwaukeeParentalChoiceProgram,”QuarterlyStandardErrorfortheLinearRegressionModel,”JournalofEconomics113,553–602.EconomicsLetters31,239–243.Sander,W.(1992),“TheEffectofWomen’sSchoolingonWooldridge,J.M.(1991a),“ANoteonComputingFertility,”EconomicLetters40,229–233.R-SquaredandAdjustedR-SquaredforTrendingandSavin,N.E.,andK.J.White(1977),“TheDurbin-WatsonSeasonalData,”EconomicsLetters36,49–54.TestforSerialCorrelationwithExtremeSampleSizesWooldridge,J.M.(1991b),“OntheApplicationoforManyRegressors,”Econometrica45,1989–1996.Robust,Regression-BasedDiagnosticstoModelsofShea,J.(1993),“TheInput-OutputApproachtoConditionalMeansandConditionalVariances,”InstrumentSelection,”JournalofBusinessandJournalofEconometrics47,5–46.EconomicStatistics11,145–155.Wooldridge,J.M.(1994a),“ASimpleSpecificationShugart,W.F.,andR.D.Tollison(1984),“TheRandomTestforthePredictiveAbilityofTransformationCharacterofMergerActivity,”RandJournalofModels,”ReviewofEconomicsandStatistics76,Economics15,500–509.59–65.789\nxd7/14/999:40PMPage790ReferencesWooldridge,J.M.(1994b),“EstimationandInferenceforAdvancesinEconometricsandQuantitativeDependentProcesses,”Chapter45inHandbookofEconomics.Ed.G.S.Maddala,P.C.B.Phillips,andEconometrics,Volume4.Ed.R.F.EngleandD.L.T.N.Srinivasan,66–87.Oxford:Blackwell.McFadden,2639–2738.Amsterdam:North-Holland.Wooldridge,J.M.(1999),EconometricAnalysisofCrossWooldridge,J.M.(1995),“ScoreDiagnosticsforLinearSectionandPanelData.Forthcoming,Cambridge,ModelsEstimatedbyTwoStageLeastSquares,”inMA:MITPress.790\nry.qxd7/14/999:45PMPage791GLOSSARYAwithattenuationbiasislessinmagnitudethantheabsolutevalueoftheparameter.AugmentedDickey-FullerTest:AtestforaunitrootAdjustedR-Squared:Agoodness-of-fitmeasureinthatincludeslaggedchangesofthevariableasregres-multipleregressionanalysisthatpenalizesadditionalsors.explanatoryvariablesbyusingadegreesoffreedomAutocorrelation:Seeserialcorrelation.adjustmentinestimatingtheerrorvariance.AutoregressiveConditionalHeteroskedasticity(ARCH):AlternativeHypothesis:ThehypothesisagainstwhichAmodelofdynamicheteroskedasticitywherethevari-thenullhypothesisistested.anceoftheerrorterm,givenpastinformation,dependsAR(1)SerialCorrelation:Theerrorsinatimeserieslinearlyonthepastsquarederrors.regressionmodelfollowanAR(1)model.AutoregressiveProcessofOrderOne[AR(1)]:AtimeAsymptoticBias:Seeinconsistency.seriesmodelwhosecurrentvaluedependslinearlyonAsymptoticConfidenceInterval:Aconfidenceintervalitsmostrecentvalueplusanunpredictabledistur-thatisapproximatelyvalidinlargesamplesizes.bance.AsymptoticNormality:ThesamplingdistributionofaAuxiliaryRegression:Aregressionusedtocomputeaproperlynormalizedestimatorconvergestothestan-teststatistic—suchastheteststatisticsforhet-dardnormaldistribution.eroskedasticityandserialcorrelation—oranyotherAsymptoticProperties:Propertiesofestimatorsandtestregressionthatdoesnotestimatethemodelofprimarystatisticsthatapplywhenthesamplesizegrowswith-interest.outbound.Average:Thesumofnnumbersdividedbyn.AsymptoticStandardError:Astandarderrorthatisvalidinlargesamples.AsymptotictStatistic:Atstatisticthathasanapproxi-matestandardnormaldistributioninlargesamples.BAsymptoticVariance:ThesquareofthevaluewemustdivideanestimatorbyinordertoobtainanasymptoticBalancedPanel:Apaneldatasetwhereallyears(orstandardnormaldistribution.periods)ofdataareavailableforallcross-AsymptoticallyEfficient:Forconsistentestimatorswithsectionalunits.asymptoticallynormaldistributions,theestimatorwithBaseGroup:Thegrouprepresentedbytheoverallinter-thesmallestasymptoticvariance.ceptinamultipleregressionmodelthatincludesAsymptoticallyUncorrelated:Atimeseriesprocessindummyexplanatoryvariables.whichthecorrelationbetweenrandomvariablesatBasePeriod:Forindexnumbers,suchaspriceorpro-twopointsintimetendstozeroasthetimeintervalductionindices,theperiodagainstwhichallothertimebetweenthemincreases.(Seealsoweaklydepen-periodsaremeasured.dent.)BaseValue:ThevalueassignedtothebaseperiodforAttenuationBias:Biasinanestimatorthatisalwaysconstructinganindexnumber;usuallythebasevalueistowardzero;thus,theexpectedvalueofanestimatoroneor100.791\nry.qxd7/14/999:45PMPage792GlossaryBenchmarkGroup:Seebasegroup.CeterisParibus:Allotherrelevantfactorsareheldfixed.BernoulliRandomVariable:ArandomvariablethatChi-SquareDistribution:Aprobabilitydistributiontakesonthevalueszeroorone.obtainedbyaddingthesquaresofindependentstan-BestLinearUnbiasedEstimator(BLUE):Amongalldardnormalrandomvariables.Thenumberoftermsinlinear,unbiasedestimators,theestimatorwiththethesumequalsthedegreesoffreedominthedistribu-smallestvariance.OLSisBLUE,conditionalonthetion.samplevaluesoftheexplanatoryvariables,undertheChowStatistic:AnFstatisticfortestingtheequalityofGauss-Markovassumptions.regressionparametersacrossdifferentgroups(say,menBetaCoefficients:Seestandardizedcoefficients.andwomen)ortimeperiods(say,beforeandafteraBias:Thedifferencebetweentheexpectedandthepopu-policychange).lationparametervaluesofanestimator.ClassicalErrors-in-Variables(CEV):AmeasurementBiasedEstimator:Anestimatorwhoseexpectation,orerrormodelwheretheobservedmeasureequalsthesamplingmean,isdifferentfromthepopulationvalueactualvariableplusanindependent,oratleastanitissupposedtobeestimating.uncorrelated,measurementerror.BiasedTowardsZero:AdescriptionofanestimatorClassicalLinearModel:Themultiplelinearregressionwhoseexpectationinabsolutevalueislessthanthemodelunderthefullsetofclassicallinearmodelabsolutevalueofthepopulationparameter.assumptions.BinaryResponseModel:Amodelforabinary(dummy)ClassicalLinearModel(CLM)Assumptions:Theidealdependentvariable.setofassumptionsformultipleregressionanalysis:forBinaryVariable:Seedummyvariable.cross-sectionalanalysis,AssumptionsMLR.1throughBinomialDistribution:TheprobabilitydistributionofMLR.6andfortimeseriesanalysis,AssumptionsTS.1thenumberofsuccessesoutofnindependentBernoullithroughTS.6.Theassumptionsincludelinearityinthetrials,whereeachtrialhasthesameprobabilityofsuc-parameters,noperfectcollinearity,thezeroconditionalcess.meanassumption,homoskedasticity,noserialcorrela-BivariateRegressionModel:Seesimplelinearregres-tion,andnormalityoftheerrors.sionmodel.ClusterEffect:AnunobservedeffectthatiscommontoBLUE:Seebestlinearunbiasedestimator.allunits,usuallypeople,inthecluster.Breusch-GodfreyTest:AnasymptoticallyjustifiedtestClusterSample:AsampleofnaturalclustersorgroupsforAR(p)serialcorrelation,withAR(1)beingthemostwhichusuallyconsistofpeople.popular;thetestallowsforlaggeddependentvariablesCochrane-Orcutt(CO)Estimation:Amethodofesti-aswellasotherregressorsthatarenotstrictlyexoge-matingamultiplelinearregressionmodelwithAR(1)nous.errorsandstrictlyexogenousexplanatoryvariables;Breusch-PaganTest:AtestforheteroskedasticitywhereunlikePrais-Winsten,Cochrane-OrcuttdoesnotusethesquaredOLSresidualsareregressedonthetheequationforthefirsttimeperiod.explanatoryvariablesinthemodel.CoefficientofDetermination:SeeR-squared.Cointegration:ThenotionthatalinearcombinationofCtwoseries,eachofwhichisintegratedoforderone,isintegratedoforderzero.CausalEffect:AceterisparibuschangeinonevariableCompositeError:Inapaneldatamodel,thesumofthehasaneffectonanothervariable.timeconstantunobservedeffectandtheidiosyncraticCensoredRegressionModel:Amultipleregressionerror.modelwherethedependentvariablehasbeencensoredConditionalDistribution:Theprobabilitydistributionofaboveorbelowsomeknownthreshold.onerandomvariable,giventhevaluesofoneormoreCentralLimitTheorem:Akeyresultfromprobabilityotherrandomvariables.theorywhichimpliesthatthesumofindependentran-ConditionalExpectation:Theexpectedoraveragevaluedomvariables,orevenweaklydependentrandomvari-ofonerandomvariable,calledthedependentorables,whenstandardizedbyitsstandarddeviation,hasexplainedvariable,thatdependsonthevaluesofoneoradistributionthattendstostandardnormalasthesam-moreothervariables,calledtheindependentorexplana-plesizegrows.toryvariables.792\nry.qxd7/14/999:45PMPage793GlossaryConditionalForecast:Aforecastthatassumesthefuturewhichateststatisticiscomparedtodeterminewhethervaluesofsomeexplanatoryvariablesareknownwithornotthenullhypothesisisrejected.certainty.Cross-SectionalDataSet:AdatasetcollectedfromaConditionalVariance:Thevarianceofonerandomvari-populationatagivenpointintime.able,givenoneormoreotherrandomvariables.CumulativeDistributionFunction(cdf):AfunctionConfidenceInterval(CI):Aruleusedtoconstructaran-thatgivestheprobabilityofarandomvariablebeingdomintervalsothatacertainpercentageofalldatalessthanorequaltoanyspecifiedrealnumber.sets,determinedbytheconfidencelevel,yieldsanintervalthatcontainsthepopulationvalue.ConfidenceLevel:ThepercentageofsamplesinwhichDwewantourconfidenceintervaltocontainthepopula-tionvalue;95%isthemostcommonconfidencelevel,DataCensoring:Asituationthatariseswhenwedonotbut90%and99%arealsoused.alwaysobservetheoutcomeonthedependentvariableConsistentEstimator:Anestimatorthatconvergesinbecauseatanupper(orlower)thresholdweonlyknowprobabilitytothepopulationparameterasthesamplethattheoutcomewasabove(orbelow)thethreshold.sizegrowswithoutbound.(Seealsocensoredregressionmodel.)ConsistentTest:Atestwhere,underthealternativeDataFrequency:Theintervalatwhichtimeseriesdatahypothesis,theprobabilityofrejectingthenullhypoth-arecollected.Yearly,quarterly,andmonthlyaretheesisconvergestooneasthesamplesizegrowswithoutmostcommondatafrequencies.bound.DataMining:ThepracticeofusingthesamedatasettoConstantElasticityModel:Amodelwheretheelasticityestimatenumerousmodelsinasearchtofindthe“best”ofthedependentvariable,withrespecttoanexplana-model.toryvariable,isconstant;inmultipleregression,bothDavidson-MacKinnonTest:Atestthatisusedfortest-variablesappearinlogarithmicform.ingamodelagainstanonnestedalternative;itcanbeContemporaneouslyExogenousRegressor:Intimeimplementedasattestonthefittedvaluesfromtheseriesorpaneldataapplications,aregressorthatiscompetingmodel.uncorrelatedwiththeerrorterminthesametimeDegreesofFreedom(df):Inmultipleregressionanaly-period,butnotnecessarilyinothertimeperiods.sis,thenumberofobservationsminusthenumberofContinuousRandomVariable:Arandomvariablethatestimatedparameters.takesonanyparticularvaluewithprobabilityzero.DenominatorDegreesofFreedom:InanFtest,theControlGroup:Inprogramevaluation,thegroupthatdegreesoffreedomintheunrestrictedmodel.doesnotparticipateintheprogram.DependentVariable:ThevariabletobeexplainedinControlVariable:Seeexplanatoryvariable.amultipleregressionmodel(andavarietyofotherCornerSolution:Anonnegativedependentvariablethatmodels).isroughlycontinuousoverstrictlypositivevaluesbutDescriptiveStatistic:Astatisticusedtosummarizeasettakesonthevaluezerowithsomeregularity.ofnumbers;thesampleaverage,samplemedian,andCorrelationCoefficient:Ameasureoflineardepen-samplestandarddeviationarethemostcommon.dencebetweentworandomvariablesthatdoesnotDeseasonalizing:Theremovingoftheseasonalcompo-dependonunitsofmeasurementandisboundednentsfromamonthlyorquarterlytimeseries.between1and1.Detrending:ThepracticeofremovingthetrendfromaCountVariable:Avariablethattakesonnonnegativetimeseries.integervalues.Dickey-FullerDistribution:ThelimitingdistributionofCovariance:Ameasureoflineardependencebetweenthetstatisticintestingthenullhypothesisofaunittworandomvariables.root.CovarianceStationary:Atimeseriesprocesswithcon-Dickey-Fuller(DF)Test:AttestoftheunitrootnullstantmeanandvariancewherethecovariancebetweenhypothesisinanAR(1)model.(SeealsoaugmentedanytworandomvariablesinthesequencedependsonlyDickey-Fullertest.)onthedistancebetweenthem.DifferenceinSlopes:AdescriptionofamodelwhereCovariate:Seeexplanatoryvariable.someslopeparametersmaydifferbygrouportimeCriticalValue:Inhypothesistesting,thevalueagainstperiod.793\nry.qxd7/14/999:45PMPage794GlossaryDifference-in-DifferencesEstimator:AnestimatorthatEconomicModel:Arelationshipderivedfromeconomicarisesinpolicyanalysiswithdatafortwotimeperiods.theoryorlessformaleconomicreasoning.OneversionoftheestimatorappliestoindependentlyEconomicSignificance:Seepracticalsignificance.pooledcrosssectionsandanothertopaneldatasets.Elasticity:Thepercentchangeinonevariablegivena1%DiminishingMarginalEffect:Themarginaleffectofanceterisparibusincreaseinanothervariable.explanatoryvariablebecomessmallerasthevalueofEmpiricalAnalysis:Astudythatusesdatainaformaltheexplanatoryvariableincreases.econometricanalysistotestatheory,estimatearela-DiscreteRandomVariable:Arandomvariablethattionship,ordeterminetheeffectivenessofapolicy.takesonatmostafiniteorcountablyinfinitenumberofEndogeneity:Atermusedtodescribethepresenceofanvalues.endogenousexplanatoryvariable.DistributedLagModel:AtimeseriesmodelthatrelatesEndogenousExplanatoryVariable:Anexplanatorythedependentvariabletocurrentandpastvaluesofanvariableinamultipleregressionmodelthatiscorre-explanatoryvariable.latedwiththeerrorterm,eitherbecauseofanomittedDisturbance:Seeerrorterm.variable,measurementerror,orsimultaneity.DownwardBias:TheexpectedvalueofanestimatorisEndogenousSampleSelection:Nonrandomsamplebelowthepopulationvalueoftheparameter.selectionwheretheselectionisrelatedtothedependentDummyDependentVariable:Seebinaryresponsevariable,eitherdirectlyorthroughtheerrorterminthemodel.equation.DummyVariable:AvariablethattakesonthevaluezeroEndogenousVariables:Insimultaneousequationsmod-orone.els,variablesthataredeterminedbytheequationsinDummyVariableRegression:Inapaneldatasetting,thethesystem.regressionthatincludesadummyvariableforeachEngle-GrangerTwo-StepProcedure:Atwo-stepmethodcross-sectionalunit,alongwiththeremainingexplana-forestimatingerrorcorrectionmodelswherebythecoin-toryvariables.Itproducesthefixedeffectsestimator.tegratingparameterisestimatedinthefirststage,andtheDummyVariableTrap:Themistakeofincludingtooerrorcorrectionparametersareestimatedinthesecond.manydummyvariablesamongtheindependentvari-ErrorCorrectionModel:Atimeseriesmodelinfirstables;itoccurswhenanoverallinterceptisinthedifferencesthatalsocontainsanerrorcorrectionterm,modelandadummyvariableisincludedforeachwhichworkstobringtwoI(1)seriesbackintolong-rungroup.equilibrium.DurationAnalysis:AnapplicationofthecensoredErrorTerm:Thevariableinasimpleormultipleregres-regressionmodel,wherethedependentvariableistimesionequationthatcontainsunobservedfactorsthatelapseduntilacertaineventoccurs,suchasthetimeaffectthedependentvariable.Theerrortermmayalsobeforeanunemployedpersonbecomesreemployed.includemeasurementerrorsintheobserveddependentDurbin-Watson(DW)Statistic:Astatisticusedtotestorindependentvariables.forfirstorderserialcorrelationintheerrorsofatimeErrorVariance:Thevarianceoftheerrorterminamul-seriesregressionmodelundertheclassicallineartipleregressionmodel.modelassumptions.Errors-in-Variables:Asituationwhereeitherthedepen-DynamicallyCompleteModel:Atimeseriesmodeldentvariableorsomeindependentvariablesaremea-wherenofurtherlagsofeitherthedependentvariablesuredwitherror.ortheexplanatoryvariableshelptoexplainthemeanofEstimate:Thenumericalvaluetakenonbyanestimatorthedependentvariable.foraparticularsampleofdata.Estimator:Aruleforcombiningdatatoproduceanumer-icalvalueforapopulationparameter;theformoftheEruledoesnotdependontheparticularsampleobtained.EventStudy:AneconometricanalysisoftheeffectsofEconometricModel:Anequationrelatingthedependentanevent,suchasachangeingovernmentregulationorvariabletoasetofexplanatoryvariablesandunob-economicpolicy,onanoutcomevariable.serveddisturbances,whereunknownpopulationparame-ExcludingaRelevantVariable:Inmultipleregressiontersdeterminetheceterisparibuseffectofeachex-analysis,leavingoutavariablethathasanonzeropar-planatoryvariable.tialeffectonthedependentvariable.794\nry.qxd7/14/999:45PMPage795GlossaryExclusionRestrictions:Restrictionswhichstatethatcer-FirstDifference:Atransformationonatimeseriescon-tainvariablesareexcludedfromthemodel(orhavestructedbytakingthedifferenceofadjacenttimeperi-zeropopulationcoefficients).ods,wheretheearliertimeperiodissubtractedfromExogenousExplanatoryVariable:Anexplanatoryvari-thelatertimeperiod.ablethatisuncorrelatedwiththeerrorterm.First-DifferencedEquation:IntimeseriesorpaneldataExogenousSampleSelection:Sampleselectionthatmodels,anequationwherethedependentandindepen-eitherdependsonexogenousexplanatoryvariablesorisdentvariableshaveallbeenfirst-differenced.independentoftheerrortermintheequationofinterest.First-DifferencedEstimator:Inapaneldatasetting,theExogenousVariable:AnyvariablethatisuncorrelatedpooledOLSestimatorappliedtofirstdifferencesofthewiththeerrorterminthemodelofinterest.dataacrosstime.ExpectedValue:AmeasureofcentraltendencyintheFirstOrderConditions:Thesetoflinearequationsuseddistributionofarandomvariable,includinganestima-tosolvefortheOLSestimates.tor.FittedValues:TheestimatedvaluesofthedependentExperiment:Inprobability,ageneraltermusedtodenotevariablewhenthevaluesoftheindependentvariablesaneventwhoseoutcomeisuncertain.IneconometricforeachobservationarepluggedintotheOLSregres-analysis,itdenotesasituationwheredataarecollectedsionline.byrandomlyassigningindividualstocontrolandtreat-FixedEffect:Seeunobservedeffect.mentgroups.FixedEffectsEstimator:FortheunobservedeffectsExperimentalData:Datathathavebeenobtainedbypaneldatamodel,theestimatorobtainedbyapplyingrunningacontrolledexperiment.pooledOLStoatime-demeanedequation.ExperimentalGroup:Seetreatmentgroup.FixedEffectsTransformation:Forpaneldata,thetime-ExplainedSumofSquares(SSE):Thetotalsamplevari-demeaneddata.ationofthefittedvaluesinamultipleregressionmodel.ForecastError:Thedifferencebetweentheactualout-ExplainedVariable:Seedependentvariable.comeandtheforecastoftheoutcome.ExplanatoryVariable:Inregressionanalysis,avariableForecastInterval:Inforecasting,aconfidenceintervalthatisusedtoexplainvariationinthedependentvariable.forayetunrealizedfuturevalueofatimeseriesvari-ExponentialFunction:Amathematicalfunctiondefinedable.(Seealsopredictioninterval.)forallvaluesthathaveanincreasingslopebutacon-FunctionalFormMisspecification:Aproblemthatstantproportionatechange.occurswhenamodelhasomittedfunctionsoftheExponentialSmoothing:Asimplemethodofforecast-explanatoryvariables(suchasquadratics)orusestheingavariablethatinvolvesaweightingofallpreviouswrongfunctionsofeitherthedependentvariableoroutcomesonthatvariable.someexplanatoryvariables.ExponentialTrend:Atrendwithaconstantgrowthrate.FGFDistribution:TheprobabilitydistributionobtainedbyGauss-MarkovAssumptions:Thesetofassumptionsformingtheratiooftwoindependentchi-squareran-(AssumptionsMLR.1throughMLR.5orTS.1throughdomvariables,whereeachhasbeendividedbyitsTS.5)underwhichOLSisBLUE.degreesoffreedom.Gauss-MarkovTheorem:ThetheoremwhichstatesFStatistic:Astatisticusedtotestmultiplehypothesesthat,underthefiveGauss-Markovassumptions(forabouttheparametersinamultipleregressionmodel.cross-sectionalortimeseriesmodels),theOLSestima-FeasibleGLS(FGLS)Estimator:AGLSproceduretorisBLUE(conditionalonthesamplevaluesofthewherevarianceorcorrelationparametersareunknownexplanatoryvariables).andthereforemustfirstbeestimated.(Seealsogener-GeneralizedLeastSquares(GLS)Estimator:Anesti-alizedleastsquaresestimator.)matorthataccountsforaknownstructureoftheerrorFiniteDistributedLag(FDL)Model:Adynamicmodelvariance(heteroskedasticity),serialcorrelationpatternwhereoneormoreexplanatoryvariablesareallowedtointheerrors,orboth,viaatransformationoftheorigi-havelaggedeffectsonthedependentvariable.nalmodel.795\nry.qxd7/14/999:45PMPage796GlossaryGeometric(orKoyck)DistributedLag:Aninfinitedis-Itributedlagmodelwherethelagcoefficientsdeclineatageometricrate.IdentifiedEquation:AnequationwhoseparameterscanGoodness-of-FitMeasure:Astatisticthatsummarizesbeconsistentlyestimated,especiallyinmodelswithhowwellasetofexplanatoryvariablesexplainsaendogenousexplanatoryvariables.dependentorresponsevariable.IdiosyncraticError:Inpaneldatamodels,theerrorthatGrangerCausality:Alimitednotionofcausalitywherechangesovertimeaswellasacrossunits(say,individ-pastvaluesofoneseries(xt)areusefulforpredictinguals,firms,orcities).futurevaluesofanotherseries(yt),afterpastvaluesofImpactElasticity:Inadistributedlagmodel,theimme-ythavebeencontrolledfor.diatepercentagechangeinthedependentvariableGrowthRate:Theproportionatechangeinatimegivena1%increaseintheindependentvariable.seriesfromthepreviousperiod.Itmaybeapproxi-ImpactMultiplier:Seeimpactpropensity.matedasthedifferenceinlogsorreportedinper-ImpactPropensity:Inadistributedlagmodel,theimme-centageform.diatechangeinthedependentvariablegivenaone-unitincreaseintheindependentvariable.IncidentalTruncation:AsampleselectionproblemHwherebyonevariable,usuallythedependentvariable,isonlyobservedforcertainoutcomesofanothervari-able.HeckitMethod:Aneconometricprocedureusedtocor-InclusionofanIrrelevantVariable:Theincludingofanrectforsampleselectionbiasduetoincidentaltrun-explanatoryvariableinaregressionmodelthathasacationorsomeotherformofnonrandomlymissingzeropopulationparameterinestimatinganequationbydata.OLS.HeterogeneityBias:ThebiasinOLSduetoomittedhet-Inconsistency:Thedifferencebetweentheprobabilityerogeneity(oromittedvariables).limitofanestimatorandtheparametervalue.Heteroskedasticity:Thevarianceoftheerrorterm,givenIndependentRandomVariables:Randomvariablestheexplanatoryvariables,isnotconstant.whosejointdistributionistheproductofthemarginalHeteroskedasticityofUnknownForm:Hetero-distributions.skedasticitythatmaydependontheexplanatoryvari-IndependentVariable:Seeexplanatoryvariable.ablesinanunknown,arbitraryfashion.IndependentlyPooledCrossSection:AdatasetHeteroskedasticity-RobustFStatistic:AnF-typesta-obtainedbypoolingindependentrandomsamplesfromtisticthatis(asymptotically)robusttoheteroskedastic-differentpointsintime.ityofunknownform.Heteroskedasticity-RobustLMStatistic:AnLMstatis-IndexNumber:Astatisticthataggregatesinformationticthatisrobusttoheteroskedasticityofunknownoneconomicactivity,suchasproductionorprices.form.InfiniteDistributedLag(IDL)Model:AdistributedlagHeteroskedasticity-RobustStandardError:Astan-modelwhereachangeintheexplanatoryvariablecandarderrorthatis(asymptotically)robusttohet-haveanimpactonthedependentvariableintotheeroskedasticityofunknownform.indefinitefuture.Heteroskedasticity-RobusttStatistic:AtstatisticthatisInfluentialObservations:Seeoutliers.(asymptotically)robusttoheteroskedasticityofInformationSet:Inforecasting,thesetofvariablesthatunknownform.wecanobservepriortoformingourforecast.HighlyPersistentProcess:AtimeseriesprocesswhereIn-SampleCriteria:Criteriaforchoosingforecastingoutcomesinthedistantfuturearehighlycorrelatedmodelsthatarebasedongoodness-of-fitwithinthewithcurrentoutcomes.sampleusedtoobtaintheparameterestimates.Homoskedasticity:TheerrorsinaregressionmodelhaveInstrumentalVariable(IV):Inanequationwithanconstantvariance,conditionalontheexplanatoryvari-endogenousexplanatoryvariable,anIVisavariableables.thatdoesnotappearintheequation,isuncorrelatedHypothesisTest:Astatisticaltestofthenull,ormain-withtheerrorintheequation,andis(partially)corre-tained,hypothesisagainstanalternativehypothesis.latedwiththeendogenousexplanatoryvariable.796\nry.qxd7/14/999:45PMPage797GlossaryInstrumentalVariables(IV)Estimator:Anestimatorinmodel,thelagcoefficientsgraphedasafunctionofthealinearmodelusedwheninstrumentalvariablesarelaglength.availableforoneormoreendogenousexplanatoryvari-LaggedDependentVariable:Anexplanatoryvariableables.thatisequaltothedependentvariablefromanearlierIntegratedofOrderOne[I(1)]:Atimeseriesprocesstimeperiod.thatneedstobefirst-differencedinordertoproduceanLaggedEndogenousVariable:Inasimultaneousequa-I(0)process.tionsmodel,alaggedvalueofoneoftheendogenousIntegratedofOrderZero[I(0)]:Astationary,weaklyvariables.dependenttimeseriesprocessthat,whenusedinLagrangeMultiplierStatistic:Ateststatisticwithlargeregressionanalysis,satisfiesthelawoflargenumberssamplejustificationthatcanbeusedtotestforomittedandthecentrallimittheorem.variables,heteroskedasticity,andserialcorrelation,InteractionEffect:Inmultipleregression,thepartialamongothermodelspecificationproblems.effectofoneexplanatoryvariabledependsonthevalueLargeSampleProperties:Seeasymptoticproperties.ofadifferentexplanatoryvariable.LatentVariableModel:AmodelwheretheobservedInteractionTerm:Anindependentvariableinaregres-dependentvariableisassumedtobeafunctionofansionmodelthatistheproductoftwoexplanatoryvari-underlyinglatent,orunobserved,variable.ables.LawofIteratedExpectations:AresultfromprobabilityInterceptParameter:Theparameterinamultiplelinearthatrelatesunconditionalandconditionalexpectations.regressionmodelthatgivestheexpectedvalueoftheLawofLargeNumbers(LLN):Atheoremwhichsaysdependentvariablewhenalltheindependentvariablesthattheaveragefromarandomsampleconvergesinequalzero.probabilitytothepopulationaverage;theLLNalsoInterceptShift:Theinterceptinaregressionmodeldif-holdsforstationaryandweaklydependenttimeseries.fersbygrouportimeperiod.LeadsandLagsEstimator:Anestimatorofacointegrat-Internet:AglobalcomputernetworkthatcanbeusedtoingparameterinaregressionwithI(1)variables,whereaccessinformationanddownloaddatabases.thecurrent,somepast,andsomefuturefirstdifferencesIntervalEstimator:Arulethatusesdatatoobtainlowerintheexplanatoryvariableareincludedasregressors.andupperboundsforapopulationparameter.(SeealsoLevel-LevelModel:Aregressionmodelwheretheconfidenceinterval.)dependentvariableandtheindependentvariablesareinInverseMillsRatio:Atermthatcanbeaddedtoamul-level(ororiginal)form.tipleregressionmodeltoremovesampleselectionbias.Level-LogModel:Aregressionmodelwherethedepen-dentvariableisinlevelformand(atleastsomeof)theJindependentvariablesareinlogarithmicform.LikelihoodRatioStatistic:AstatisticthatcanbeusedJointDistribution:Theprobabilitydistributiondeter-totestsingleormultiplehypotheseswhenthecon-miningtheprobabilitiesofoutcomesinvolvingtwoorstrainedandunconstrainedmodelshavebeenesti-morerandomvariables.matedbymaximumlikelihood.ThestatisticistwiceJointHypothesisTest:Atestinvolvingmorethanonethedifferenceintheunconstrainedandconstrainedrestrictionontheparametersinamodel.log-likelihoods.JointlyStatisticallySignificant:ThenullhypothesisthatLimitedDependentVariable:Adependentorresponsetwoormoreexplanatoryvariableshavezeropopulationvariablewhoserangeisrestrictedinsomeimportantcoefficientsisrejectedatthechosensignificancelevel.way.JustIdentifiedEquation:FormodelswithendogenousLinearFunction:Afunctionwherethechangeintheexplanatoryvariables,anequationthatisidentifiedbutdependentvariable,givenaone-unitchangeinaninde-wouldnotbeidentfiedwithonefewerinstrumentalpendentvariable,isconstant.variable.LinearProbabilityModel(LPM):AbinaryresponsemodelwheretheresponseprobabilityislinearinitsLparameters.LinearTimeTrend:AtrendthatisalinearfunctionofLagDistribution:Inafiniteorinfinitedistributedlagtime.797\nry.qxd7/14/999:45PMPage798GlossaryLinearUnbiasedEstimator:InmultipleregressionMatrix:Anarrayofnumbers.analysis,anunbiasedestimatorthatisalinearfunctionMatrixNotation:Aconvenientmathematicalnotation,oftheoutcomesonthedependentvariable.groundedinmatrixalgebra,forexpressingandmanip-LogarithmicFunction:Amathematicalfunctiondefinedulatingthemultipleregressionmodel.forpositiveargumentsthathasapositive,butdimin-MaximumLikelihoodEstimation(MLE):Abroadlyishing,slope.applicableestimationmethodwheretheparameteresti-Log-LevelModel:Aregressionmodelwherethedepen-matesarechosentomaximizethelog-likelihoodfunc-dentvariableisinlogarithmicformandtheindepen-tion.dentvariablesareinlevel(ororiginal)form.Mean:Seeexpectedvalue.Log-LogModel:Aregressionmodelwherethedepen-MeanAbsoluteError(MAE):Aperformancemeasuredentvariableand(atleastsomeof)theexplanatoryinforecasting,computedastheaverageoftheabsolutevariablesareinlogarithmicform.valuesoftheforecasterrors.LogitModel:AmodelforbinaryresponsewheretheMeanSquaredError:Theexpectedsquareddistancethatresponseprobabilityisthelogitfunctionevaluatedataanestimatorisfromthepopulationvalue;itequalsthelinearfunctionoftheexplanatoryvariables.varianceplusthesquareofanybias.Log-LikelihoodFunction:Thesumofthelog-likelihoods,MeasurementError:Thedifferencebetweenanwherethelog-likelihoodforeachobservationisthelogobservedvariableandthevariablethatbelongsinaofthedensityofthedependentvariablegiventhemultipleregressionequation.explanatoryvariables;thelog-likelihoodfunctionisMedian:Inaprobabilitydistribution,itisthevalueviewedasafunctionoftheparameterstobeestimated.wherethereisa50%chanceofbeingbelowthevalueLong-RunElasticity:Thelong-runpropensityinadis-anda50%chanceofbeingaboveit.Inasampleoftributedlagmodelwiththedependentandindependentnumbers,itisthemiddlevalueafterthenumbershavevariablesinlogarithmicform;thus,thelong-runelas-beenordered.ticityistheeventualpercentageincreaseintheMethodofMomentsEstimator:Anestimatorobtainedexplainedvariable,givenapermanent1%increaseinbyusingthesampleanalogofpopulationmoments;theexplanatoryvariable.ordinaryleastsquaresandtwostageleastsquaresareLong-RunMultiplier:Seelong-runpropensity.bothmethodofmomentsestimators.Long-RunPropensity:Inadistributedlagmodel,theMicronumerosity:AtermintroducedbyArthureventualchangeinthedependentvariablegivenaper-Goldbergertodescribepropertiesofeconometricesti-manent,one-unitincreaseintheindependentvariable.matorswithsmallsamplesizes.MinimumVarianceUnbiasedEstimator:AnestimatorLongitudinalData:Seepaneldata.withthesmallestvarianceintheclassofallunbiasedLossFunction:Afunctionthatmeasuresthelosswhenaestimators.forecastdiffersfromtheactualoutcome;themostcom-MissingData:Adataproblemthatoccurswhenwedomonexamplesareabsolutevaluelossandsquaredloss.notobservevaluesonsomevariablesforcertainobser-vations(individuals,cities,timeperiods,andsoon)inMthesample.MovingAverageProcessofOrderOne[MA(1)]:AMarginalEffect:Theeffectonthedependentvariabletimeseriesprocessgeneratedasalinearfunctionofthethatresultsfromchanginganindependentvariablebycurrentvalueandonelaggedvalueofazero-mean,asmallamount.constantvariance,uncorrelatedstochasticprocess.Martingale:Atimeseriesprocesswhoseexpectedvalue,Multicollinearity:Atermthatreferstocorrelationamonggivenallpastoutcomesontheseries,simplyequalsthetheindependentvariablesinamultipleregressionmostrecentvalue.model;itisusuallyinvokedwhensomecorrelationsareMartingaleDifferenceSequence:Thefirstdifferenceof“large,”butanactualmagnitudeisnotwell-defined.amartingale.Itisunpredictable(orhasazeromean),MultipleHypothesisTest:Atestofanullhypothesisgivenpastvaluesofthesequence.involvingmorethanonerestrictionontheparameters.MatchedPairsSample:Asamplewhereeachobserva-MultipleLinearRegression(MLR)Model:Amodeltionismatchedwithanother,asinasampleconsistinglinearinitsparameters,wherethedependentvariableisofahusbandandwifeorasetoftwosiblings.afunctionofindependentvariablesplusanerrorterm.798\nry.qxd7/14/999:45PMPage799GlossaryMultipleRegressionAnalysis:AtypeofanalysisthatisOusedtodescribeestimationofandinferenceinthemul-tiplelinearregressionmodel.ObservationalData:Seenonexperimentaldata.MultipleRestrictions:MorethanonerestrictionontheOLS:Seeordinaryleastsquares.parametersinaneconometricmodel.OLSInterceptEstimate:TheinterceptinanOLSMultipleStep-AheadForecast:Atimeseriesforecastofregressionline.morethanoneperiodintothefuture.OLSRegressionLine:Theequationrelatingthepre-MultiplicativeMeasurementError:Measurementerrordictedvalueofthedependentvariabletotheindepen-wheretheobservedvariableistheproductofthetruedentvariables,wheretheparameterestimateshaveunobservedvariableandapositivemeasurementerror.beenobtainedbyOLS.OLSSlopeEstimate:AslopeinanOLSregressionline.OmittedVariableBias:ThebiasthatarisesintheOLSNestimatorswhenarelevantvariableisomittedfromtheregression.n-R-SquaredStatistic:SeeLagrangemultiplierstatistic.OmittedVariables:Oneormorevariables,whichweNaturalExperiment:Asituationwheretheeconomicwouldliketocontrolfor,havebeenomittedinestimat-environment—sometimessummarizedbyanexplana-ingaregressionmodel.toryvariable—exogenouslychanges,perhapsinadver-One-SidedAlternative:Analternativehypothesiswhichtently,duetoapolicyorinstitutionalchange.statesthattheparameterisgreaterthan(orlessthan)NaturalLogarithm:Seelogarithmicfunction.thevaluehypothesizedunderthenull.NominalVariable:AvariablemeasuredinnominalorOne-Step-AheadForecast:Atimeseriesforecastonecurrentdollars.periodintothefuture.NonexperimentalData:DatathathavenotbeenOne-TailedTest:Ahypothesistestagainstaone-sidedobtainedthroughacontrolledexperiment.alternative.NonlinearFunction:Afunctionwhoseslopeisnotcon-On-LineDataBases:Databasesthatcanbeaccessedviastant.acomputernetwork.NonnestedModels:Two(ormore)modelswherenoOn-LineSearchServices:ComputersoftwarethatmodelcanbewrittenasaspecialcaseoftheotherbyallowstheInternetordatabasesontheInternettobeimposingrestrictionsontheparameters.searchedbytopic,name,title,orkeywords.NonrandomSampleSelection:AsampleselectionOrderCondition:Anecessaryconditionforidentifyingprocessthatcannotbecharacterizedasdrawingran-theparametersinamodelwithoneormoreendoge-domlyfromthepopulationofinterest.nousexplanatoryvariables:thetotalnumberofexoge-NonstationaryProcess:Atimeseriesprocesswhosenousvariablesmustbeatleastasgreatasthetotaljointdistributionsarenotconstantacrossdifferentnumberofexplanatoryvariables.epochs.OrdinalVariable:AvariablewheretheorderingoftheNormalDistribution:Aprobabilitydistributioncom-valuesconveysinformationbutthemagnitudeofthemonlyusedinstatisticsandeconometricsformodelingvaluesdoesnot.apopulation.ItsprobabilitydistributionfunctionhasaOrdinaryLeastSquares(OLS):Amethodforestimatingbellshape.theparametersofamultiplelinearregressionmodel.NormalityAssumption:TheclassicallinearmodelTheordinaryleastsquaresestimatesareobtainedbyassumptionwhichstatesthattheerror(ordependentminimizingthesumofsquaredresiduals.variable)hasanormaldistribution,conditionalontheOutliers:Observationsinadatasetthataresubstantiallyexplanatoryvariables.differentfromthebulkofthedata,perhapsbecauseofNullHypothesis:Inclassicalhypothesistesting,wetakeerrorsorbecausesomedataaregeneratedbyadiffer-thishypothesisastrueandrequirethedatatoprovideentmodelthanmostoftheotherdata.substantialevidenceagainstit.Out-of-SampleCriteria:Criteriausedforchoosingfore-NumeratorDegreesofFreedom:InanFtest,thenum-castingmodelsthatarebasedonapartofthesampleberofrestrictionsbeingtested.thatwasnotusedinobtainingparameterestimates.799\nry.qxd7/14/999:45PMPage800GlossaryOverallSignificanceofaRegression:AtestofthejointPointForecast:Theforecastedvalueofafutureout-significanceofallexplanatoryvariablesappearinginacome.multipleregressionequation.PoissonDistribution:AprobabilitydistributionforOverdispersion:Inmodelingacountvariable,thevari-countvariables.anceislargerthanthemean.PoissonRegressionModel:Amodelforacountdepen-OveridentifiedEquation:Inmodelswithendogenousdentvariablewherethedependentvariable,conditionalexplanatoryvariables,anequationwherethenumberofontheexplanatoryvariables,isnominallyassumedtoinstrumentalvariablesisstrictlygreaterthanthenum-haveaPoissondistribution.berofendogenousexplanatoryvariables.PolicyAnalysis:Anempiricalanalysisthatusesecono-OveridentifyingRestrictions:Theextramomentcondi-metricmethodstoevaluatetheeffectsofacertainpol-tionsthatcomefromhavingmoreinstrumentalvariablesicy.thanendogenousexplanatoryvariablesinalinearmodel.PooledCrossSection:Adataconfigurationwhereinde-OverspecifyingaModel:Seeinclusionofanirrelevantpendentcrosssections,usuallycollectedatdifferentvariable.pointsintime,arecombinedtoproduceasingledataset.PooledOLSEstimation:OLSestimationwithindepen-Pdentlypooledcrosssections,paneldata,orclustersam-ples,wheretheobservationsarepooledacrosstime(orp-value:Thesmallestsignificancelevelatwhichthenullgroup)aswellasacrossthecross-sectionalunits.hypothesiscanberejected.Equivalently,thelargestPopulation:Awell-definedgroup(ofpeople,firms,significancelevelatwhichthenullhypothesiscannotcities,andsoon)thatisthefocusofastatisticalorberejected.econometricanalysis.PanelData:AdatasetconstructedfromrepeatedcrossPopulationModel:Amodel,especiallyamultiplelinearsectionsovertime.Withabalancedpanel,thesameregressionmodel,thatdescribesapopulation.unitsappearineachtimeperiod.WithanunbalancedPopulationR-Squared:Inthepopulation,thefractionofpanel,someunitsdonotappearineachtimeperiod,thevariationinthedependentvariablethatisexplainedoftenduetoattrition.bytheexplanatoryvariables.PairwiseUncorrelatedRandomVariables:AsetoftwoPopulationRegressionFunction:Seeconditionalormorerandomvariableswhereeachpairisuncorre-expectation.lated.PowerofaTest:Theprobabilityofrejectingthenullhy-Parameter:Anunknownvaluethatdescribesapopula-pothesiswhenitisfalse;thepowerdependsontheval-tionrelationship.uesofthepopulationparametersunderthealternative.ParsimoniousModel:AmodelwithasfewparametersPracticalSignificance:Thepracticaloreconomicimpor-aspossibleforcapturinganydesiredfeatures.tanceofanestimate,whichismeasuredbyitssignandPartialEffect:Theeffectofanexplanatoryvariableonmagnitude,asopposedtoitsstatisticalsignificance.thedependentvariable,holdingotherfactorsinthePrais-Winsten(PW)Estimation:Amethodofestimat-regressionmodelfixed.ingamultiplelinearregressionmodelwithAR(1)PercentCorrectlyPredicted:Inabinaryresponseerrorsandstrictlyexogenousexplanatoryvariables;model,thepercentageoftimesthepredictionofzeroorunlikeCochrane-Orcutt,Prais-Winstenusestheequa-onecoincideswiththeactualoutcome.tionforthefirsttimeperiodinestimation.PercentageChange:Theporportionatechangeinavari-PredeterminedVariable:Inasimultaneousequationsable,multipliedby100.model,eitheralaggedendogenousvariableoralaggedPercentagePointChange:Thechangeinavariablethatexogenousvariable.ismeasuredasapercent.PredictedVariable:Seedependentvariable.PerfectCollinearity:Inmultipleregression,oneinde-Prediction:Theestimateofanoutcomeobtainedbyplug-pendentvariableisanexactlinearfunctionofoneorgingspecificvaluesoftheexplanatoryvariablesintoanmoreotherindependentvariables.estimatedmodel,usuallyamultipleregressionmodel.Plug-InSolutiontotheOmittedVariablesProblem:APredictionError:Thedifferencebetweentheactualout-proxyvariableissubstitutedforanunobservedomittedcomeandapredictionofthatoutcome.variableinanOLSregression.PredictionInterval:Aconfidenceintervalforan800\nry.qxd7/14/999:45PMPage801Glossaryunknownoutcomeonadependentvariableinamulti-Rpleregressionmodel.PredictorVariable:Seeexplanatoryvariable.R-BarSquared:SeeadjustedR-squared.ProbabilityDensityFunction(pdf):Afunctionthat,forR-Squared:Inamultipleregressionmodel,thepropor-discreterandomvariables,givestheprobabilitythatthetionofthetotalsamplevariationinthedependentvari-randomvariabletakesoneachvalue;forcontinuousablethatisexplainedbytheindependentvariable.randomvariables,theareaunderthepdfgivestheprob-R-SquaredFormoftheFStatistic:TheFstatisticforabilityofvariousevents.testingexclusionrestrictionsexpressedintermsoftheProbabilityLimit:Thevaluetowhichanestimatorcon-R-squaredsfromtherestrictedandunrestrictedmodels.vergesasthesamplesizegrowswithoutbound.RandomEffectsEstimator:AfeasibleGLSestimatorinProbitModel:Amodelforbinaryresponseswherethetheunobservedeffectsmodelwheretheunobservedresponseprobabilityisthestandardnormalcdfevalu-effectisassumedtobeuncorrelatedwiththeexplana-atedatalinearfunctionoftheexplanatoryvariables.toryvariablesineachtimeperiod.ProgramEvaluation:AnanalysisofaparticularprivateRandomEffectsModel:Theunobservedeffectspanelorpublicprogramusingeconometricmethodstoobtaindatamodelwheretheunobservedeffectisassumedtothecausaleffectoftheprogram.beuncorrelatedwiththeexplanatoryvariablesineachProportionateChange:Thechangeinavariablerelativetimeperiod.toitsinitialvalue;mathematically,thechangedividedRandomSampling:Asamplingschemewherebyeachbytheinitialvalue.observationisdrawnatrandomfromthepopulation.InProxyVariable:Anobservedvariablethatisrelatedbutparticular,nounitismorelikelytobeselectedthananynotidenticaltoanunobservedexplanatoryvariableinotherunit,andeachdrawisindependentofallothermultipleregressionanalysis.draws.RandomVariable:Avariablewhoseoutcomeisuncer-Qtain.RandomWalk:AtimeseriesprocesswherenextQuadraticFunctions:Functionsthatcontainsquaresofperiod’svalueisobtainedasthisperiod’svalue,plusoneormoreexplanatoryvariables;theycapturedimin-anindependent(oratleastanuncorrelated)errorishingorincreasingeffectsonthedependentvariable.term.QualitativeVariable:Avariabledescribinganon-RandomWalkwithDrift:Arandomwalkthathasaquantitativefeatureofanindividual,afirm,acity,andconstant(ordrift)addedineachperiod.soon.RankCondition:AsufficientconditionforidentificationQuasi-DemeanedData:Inrandomeffectsestimationforofamodelwithoneormoreendogenousexplanatorypaneldata,itistheoriginaldataineachtimeperiodvariables.minusafractionofthetimeaverage;thesecalculationsRationalDistributedLag(RDL)Model:Atypeofinfi-aredoneforeachcross-sectionalobservation.nitedistributedlagmodelwherethelagdistributionQuasi-DifferencedData:Inestimatingaregressiondependsonrelativelyfewparameters.modelwithAR(1)serialcorrelation,itisthedifferenceRealVariable:Amonetaryvaluemeasuredintermsofabetweenthecurrenttimeperiodandamultipleofthebaseperiod.previoustimeperiod,wherethemultipleistheparam-ReducedFormEquation:AlinearequationwhereaneterintheAR(1)model.endogenousvariableisafunctionofexogenousvari-Quasi-Experiment:Seenaturalexperiment.ablesandunobservederrors.Quasi-LikelihoodRatioStatistic:AmodificationoftheReducedFormError:Theerrortermappearinginalikelihoodratiostatisticthataccountsforpossibledis-reducedformequation.tributionalmisspecification,asinaPoissonregressionReducedFormParameters:Theparametersappearingmodel.inareducedformequation.Quasi-MaximumLikelihoodEstimation:MaximumRegressand:Seedependentvariable.likelihoodestimationbutwherethelog-likelihoodRegressionErrorSpecificationTest(RESET):Agen-functionmaynotcorrespondtotheactualconditionaleraltestforfunctionalforminamultipleregressiondistributionofthedependentvariable.model;itisanFtestofjointsignificanceofthe801\nry.qxd7/14/999:45PMPage802Glossarysquares,cubes,andperhapshigherpowersofthefittedSamplingDistribution:TheprobabilitydistributionofvaluesfromtheinitialOLSestimation.anestimatoroverallpossiblesampleoutcomes.RegressionThroughtheOrigin:RegressionanalysisSamplingVariance:Thevarianceinthesamplingdistri-wheretheinterceptissettozero;theslopesarebutionofanestimator;itmeasuresthespreadintheobtainedbyminimizingthesumofsquaredresiduals,samplingdistribution.asusual.ScoreStatistic:SeeLagrangemultiplierstatistic.Regressor:Seeexplanatoryvariable.SeasonalDummyVariables:AsetofdummyvariablesRejectionRegion:Thesetofvaluesofateststatisticthatusedtodenotethequartersormonthsoftheyear.leadstorejectingthenullhypothesis.Seasonality:AfeatureofmonthlyorquarterlytimeseriesRejectionRule:Inhypothesistesting,therulethatdeter-wheretheaveragevaluedifferssystematicallybysea-mineswhenthenullhypothesisisrejectedinfavorofsonoftheyear.thealternativehypothesis.SeasonallyAdjusted:MonthlyorquarterlytimeseriesResidual:Thedifferencebetweentheactualvalueanddatawheresomestatisticalprocedure—possiblythefitted(orpredicted)value;thereisaresidualforregressiononseasonaldummyvariables—hasbeeneachobservationinthesampleusedtoobtainanOLSusedtoremovetheseasonalcomponent.regressionline.SelectedSample:Asampleofdataobtainednotbyran-ResidualAnalysis:Atypeofanalysisthatstudiesthedomsamplingbutbyselectingonthebasisofsomesignandsizeofresidualsforparticularobservationsobservedorunobservedcharacteristic.afteramultipleregressionmodelhasbeenestimated.Semi-Elasticity:ThepercentagechangeinthedependentResidualSumofSquares:Seesumofsquaredresiduals.variablegivenaone-unitincreaseinanindependentResponseProbability:Inabinaryresponsemodel,thevariable.probabilitythatthedependentvariabletakesontheSensitivityAnalysis:Theprocessofcheckingwhethervalueone,conditionalonexplanatoryvariables.theestimatedeffectsandstatisticalsignificanceofkeyResponseVariable:Seedependentvariable.explanatoryvariablesaresensitivetoinclusionofotherRestrictedModel:Inhypothesistesting,themodelexplanatoryvariables,functionalform,droppingofobtainedafterimposingalloftherestrictionsrequiredpotentiallyoutlyingobservations,ordifferentmethodsunderthenull.ofestimation.RootMeanSquaredError(RMSE):AnothernameforSerialCorrelation:Inatimeseriesorpaneldatamodel,thestandarderroroftheregressioninmultipleregres-correlationbetweentheerrorsindifferenttimeperiods.sionanalysis.SerialCorrelation-RobustStandardError:Astandarderrorforanestimatorthatis(asymptotically)validwhetherornottheerrorsinthemodelareseriallycor-related.SSeriallyUncorrelated:Theerrorsinatimeseriesorpaneldatamodelarepairwiseuncorrelatedacrosstime.SampleAverage:Thesumofnnumbersdividedbyn;aShort-RunElasticity:Theimpactpropensityinadistrib-measureofcentraltendency.utedlagmodelwhenthedependentandindependentSampleCorrelation:Foroutcomesontworandomvari-variablesareinlogarithmicform.ables,thesamplecovariancedividedbytheproductofSignificanceLevel:TheprobabilityofTypeIerrorinthesamplestandarddeviations.hypothesistesting.SampleCovariance:Anunbiasedestimatorofthepopu-SimpleLinearRegressionModel:Amodelwherethelationcovariancebetweentworandomvariables.dependentvariableisalinearfunctionofasingleinde-SampleRegressionFunction:SeeOLSregressionline.pendentvariable,plusanerrorterm.SampleSelectionBias:BiasintheOLSestimatorwhichSimultaneity:Atermthatmeansatleastoneexplanatoryisinducedbyusingdatathatarisefromendogenousvariableinamultiplelinearregressionmodelisdeter-sampleselection.minedjointlywiththedependentvariable.SampleStandardDeviation:AconsistentestimatorofSimultaneityBias:ThebiasthatarisesfromusingOLStothepopulationstandarddeviation.estimateanequationinasimultaneousequationsmodel.SampleVariance:Anunbiased,consistentestimatorofSimultaneousEquationsModel(SEM):Amodelthatthepopulationvariance.jointlydeterminestwoormoreendogenousvariables,802\nry.qxd7/14/999:45PMPage803GlossarywhereeachendogenousvariablecanbeafunctionofStatisticalInference:Theactoftestinghypothesesaboutotherendogenousvariablesaswellasofexogenouspopulationparameters.variablesandanerrorterm.StatisticallyDifferentfromZero:Seestatisticallysig-SlopeParameter:Thecoefficientonanindependentnificant.variableinamultipleregressionmodel.StatisticallyInsignificant:FailuretorejectthenullSpreadsheet:Computersoftwareusedforenteringandhypothesisthatapopulationparameterisequaltozero,manipulatingdata.atthechosensignificancelevel.SpuriousCorrelation:Acorrelationbetweentwovari-StatisticallySignificant:Rejectingthenullhypothesisablesthatisnotduetocausality,butperhapstothethataparameterisequaltozeroagainstthespecifieddependenceofthetwovariablesonanotherunobservedalternative,atthechosensignificancelevel.factor.StochasticProcess:AsequenceofrandomvariablesSpuriousRegressionProblem:Aproblemthatarisesindexedbytime.whenregressionanalysisindicatesarelationshipStrictExogeneity:Anassumptionthatholdsinatimebetweentwoormoreunrelatedtimeseriesprocessesseriesorpaneldatamodelwhentheexplanatoryvari-simplybecauseeachhasatrend,isanintegratedtimeablesarestrictlyexogenous.series(suchasarandomwalk),orboth.StrictlyExogenous:AfeatureofexplanatoryvariablesinStableAR(1)Process:AnAR(1)processwherethepa-atimeseriesorpaneldatamodelwheretheerrortermrameteronthelagislessthanoneinabsolutevalue.atanytimeperiodhaszeroexpectation,conditionalonThecorrelationbetweentworandomvariablesinthetheexplanatoryvariablesinalltimeperiods;alesssequencedeclinestozeroatageometricrateasthedis-restrictiveversionisstatedintermsofzerocorrelations.tancebetweentherandomvariablesincreases,andsoaStronglyDependent:Seehighlypersistentprocess.stableAR(1)processisweaklydependent.StructuralEquation:Anequationderivedfromeco-StandardDeviation:Acommonmeasureofspreadinnomictheoryorfromlessformaleconomicreasoning.thedistributionofarandomvariable.StructuralError:Theerrorterminastructuralequation,StandardDeviationofˆj:Acommonmeasureofspreadwhichcouldbeoneequationinasimultaneousequa-inthesamplingdistributionofˆj.tionsmodel.StandardErrorofˆj:Anestimateofthestandarddevi-StructuralParameters:Theparametersappearinginaationinthesamplingdistributionofˆj.structuralequation.StandardErroroftheEstimate:SeestandarderrorofSumofSquaredResiduals:Inmultipleregressiontheregression.analysis,thesumofthesquaredOLSresidualsacrossStandardErroroftheRegression(SER):Inmultipleallobservations.regressionanalysis,theestimateofthestandarddevia-SummationOperator:Anotation,denotedby,usedtotionofthepopulationerror,obtainedasthesquarerootdefinethesummingofasetofnumbers.ofthesumofsquaredresidualsoverthedegreesoffreedom.StandardNormalDistribution:ThenormaldistributionTwithmeanzeroandvarianceone.StandardizedCoefficient:AregressioncoefficientthattDistribution:Thedistributionoftheratioofastandardmeasuresthestandarddeviationchangeinthedepen-normalrandomvariableandthesquarerootofaninde-dentvariablegivenaonestandarddeviationincreaseinpendentchi-squarerandomvariable,wherethechi-anindependentvariable.squarerandomvariableisfirstdividedbyitsdf.StandardizedRandomVariable:ArandomvariabletRatio:Seetstatistic.transformedbysubtractingoffitsexpectedvalueandtStatistic:Thestatisticusedtotestasinglehypothesisdividingtheresultbyitsstandarddeviation;thenewran-abouttheparametersinaneconometricmodel.domvariablehasmeanzeroandstandarddeviationone.TestStatistic:AruleusedfortestinghypotheseswhereStaticModel:Atimeseriesmodelwhereonlycontem-eachsampleoutcomeproducesanumericalvalue.poraneousexplanatoryvariablesaffectthedependentTextEditor:Computersoftwarethatcanbeusedtoeditvariable.textfiles.StationaryProcess:Atimeseriesprocesswherethemar-Text(ASCII)File:Auniversalfileformatthatcanbetrans-ginalandalljointdistributionsareinvariantacrosstime.portedacrossnumerouscomputerplatforms.803\nry.qxd7/14/999:45PMPage804GlossaryTime-DemeanedData:Paneldatawhere,foreachcross-Usectionalunit,theaverageovertimeissubtractedfromthedataineachtimeperiod.UnbalancedPanel:ApaneldatasetwherecertainyearsTimeSeriesData:Datacollectedovertimeononeor(orperiods)ofdataaremissingforsomecross-morevariables.sectionalunits.TimeSeriesProcess:Seestochasticprocess.UnbiasedEstimator:AnestimatorwhoseexpectedTimeTrend:Afunctionoftimethatistheexpectedvaluevalue(ormeanofitssamplingdistribution)equalstheofatrendingtimeseriesprocess.populationvalue(regardlessofthepopulationvalue).TobitModel:AmodelforadependentvariablethattakesUnconditionalForecast:Aforecastthatdoesnotrelyononthevaluezerowithpositiveprobabilitybutisknowing,orassumingvaluesfor,futureexplanatoryroughlycontinuouslydistributedoverstrictlypositivevariables.values.(Seealsocornersolution.)UncorrelatedRandomVariables:RandomvariablesTopCoding:Aformofdatacensoringwherethevalueofthatarenotlinearlyrelated.avariableisnotreportedwhenitisaboveagivenUnderspecifyingaModel:Seeexcludingarelevantvari-threshold;weonlyknowthatitisatleastaslargeastheable.threshold.UnidentifiedEquation:AnequationwithoneormoreTotalSumofSquares(SST):Thetotalsamplevariationendogenousexplanatoryvariableswheresufficientinadependentvariableaboutitssampleaverage.instrumentalvariablesdonotexisttoidentifythepa-TreatmentGroup:Inprogramevaluation,thegroupthatrameters.participatesintheprogram.(SeealsoexperimentalUnitRootProcess:Ahighlypersistenttimeseriesgroup.)processwherethecurrentvalueequalslastperiod’sTrendingProcess:Atimeseriesprocesswhosevalue,plusaweaklydependentdisturbance.expectedvalueisanincreasingordecreasingfunctionUnobservedEffect:Inapaneldatamodel,anunob-oftime.servedvariableintheerrortermthatdoesnotchangeTrend-StationaryProcess:Aprocessthatisstationaryovertime.Forclustersamples,anunobservedvariableonceatimetrendhasbeenremoved;itisusuallythatiscommontoallunitsinthecluster.implicitthatthedetrendedseriesisweaklydepen-UnobservedEffectsModel:Amodelforpaneldataordent.clustersampleswheretheerrortermcontainsanunob-TruncatedRegressionModel:Aclassicallinearregres-servedeffect.sionmodelforcross-sectionaldatainwhichthesam-UnobservedHeterogeneity:Seeunobservedeffect.plingschemeentirelyexcludes,onthebasisofUnrestrictedModel:Inhypothesistesting,themodeloutcomesonthedependentvariable,partofthepopu-thathasnorestrictionsplacedonitsparameters.lation.UpwardBias:TheexpectedvalueofanestimatorisTrueModel:Theactualpopulationmodelrelatingthegreaterthanthepopulationparametervalue.dependentvariabletotherelevantindependentvari-ables,plusadisturbance,wherethezeroconditionalmeanassumptionholds.TwoStageLeastSquares(2SLS)Estimator:Aninstru-VmentalvariablesestimatorwheretheIVforanendoge-nousexplanatoryvariableisobtainedasthefittedvalueVariance:Ameasureofspreadinthedistributionofafromregressingtheendogenousexplanatoryvariableonrandomvariable.allexogenousvariables.VarianceofthePredictionError:ThevarianceintheTwo-SidedAlternative:Analternativewherethepop-errorthatariseswhenpredictingafuturevalueoftheulationparametercanbeeitherlessthanorgreaterthandependentvariablebasedonanestimatedmultiplethevaluestatedunderthenullhypothesis.regressionequation.Two-TailedTest:Atestagainstatwo-sidedalternative.VectorAutoregressive(VAR)Model:AmodelfortwoTypeIError:Arejectionofthenullhypothesiswhenitormoretimeserieswhereeachvariableismodeledasistrue.alinearfunctionofpastvaluesofallvariables,plusdis-TypeIIError:Thefailuretorejectthenullhypothesisturbancesthathavezeromeansgivenallpastvaluesofwhenitisfalse.theobservedvariables.804\nry.qxd7/14/999:45PMPage805GlossaryWWithinTransformation:Seefixedeffectstransforma-tion.WeaklyDependent:Atermthatdescribesatimeseriesprocesswheresomemeasureofdependencebetweenrandomvariablesattwopointsintime—suchascorre-Ylation—diminishesastheintervalbetweenthetwopointsintimeincreases.YearDummyVariables:FordatasetswithatimeseriesWeightedLeastSquares(WLS)Estimator:Anestima-component,dummy(binary)variablesequaltooneintorusedtoadjustforaknownformofheteroskedastic-therelevantyearandzeroinallotheryears.ity,whereeachsquaredresidualisweightedbytheinverseofthe(estimated)varianceoftheerror.WhiteTest:AtestforheteroskedasticitythatinvolvesregressingthesquaredOLSresidualsontheOLSfittedZvaluesandonthesquaresofthefittedvalues;initsmostgeneralform,thesquaredOLSresidualsareZeroConditionalMeanAssumption:Akeyassumptionregressedontheexplanatoryvariables,thesquaresofusedinmultipleregressionanalysiswhichstatesthat,theexplanatoryvariables,andallthenonredundantgivenanyvaluesoftheexplanatoryvariables,thecrossproductsoftheexplanatoryvariables.expectedvalueoftheerrorequalszero.(SeeWithinEstimator:Seefixedeffectsestimator.AssumptionsMLR.3,TS.2,andTS.2.)805\n查看更多